- 1Department of Gastroenterology, The Second Affiliated Hospital of Guanzhou University of Chinese Medicine, Guangzhou, China
- 2The Fifth Affiliated Hospital of Guangzhou Medical University, Guangzhou, China
Background: Although large language models (LLMs) have demonstrated powerful capabilities in general domains, they may output information in the medical field that could be incorrect, incomplete, or fabricated. They are also unable to answer personalized questions related to departments or individual patient health. Retrieval-augmented generation technology (RAG) can introduce external knowledge bases and utilize the retrieved information to generate answers or text, thereby enhancing prediction accuracy.
Method: We introduced internal departmental data and 17 commonly used gastroenterology guidelines as a knowledge base. Based on RAG, we developed the Endo-chat medical chat application, which can answer patient questions related to gastrointestinal endoscopy. We then included 200 patients undergoing gastrointestinal endoscopy, randomly divided into two groups of 100 each, for a questionnaire survey. A comparative evaluation was conducted between the traditional manual methods and Endo-chat.
Results: Compared to ChatGPT, Endo-chat can accurately and professionally answer relevant questions after matching the knowledge base. In terms of response efficiency, completeness, and patient satisfaction, Endo-chat outperformed manual methods significantly. There was no statistical difference in response accuracy between the two. Patients showed a preference for AI services and expressed support for the introduction of AI. All participating nurses in the survey believed that introducing AI could reduce nursing workload.
Conclusion: In clinical practice, Endo-chat can be used as a highly effective auxiliary tool for digestive endoscopic care.
Introduction
With the rapid rise of OpenAI’s ChatGPT, large language models (LLMs) have attracted widespread attention in various fields. They have demonstrated significant capabilities in clinical information processing tasks, such as medical Q&A (1), data extraction (2), medical record summarization (3), content generation, and predictive modeling (4). However, commonly used large language models in the market, such as OpenAI’s ChatGPT, are trained using publicly available data and are not optimized for clinical use. This means that when prompted with clinical questions, publicly available LLMs may output incorrect, incomplete, or fabricated information and are unable to answer questions applicable to certain internal departmental data (5).
Despite these limitations, LLMs are believed to have enormous potential in biomedical and clinical applications. This is because modern medical practice is a highly complex task, with the volume of knowledge generated increasing annually. For example, it is estimated that in 2016, two papers were uploaded to PubMed every minute (6), which has surely increased over the past 7 years. The medical field continues to expand its clinical knowledge system and develop comprehensive practice guidelines, such as the 2022 Chinese guidelines for the treatment of Helicobacter pylori infection and the diagnosis and treatment of chronic gastritis. LLMs do not update in real-time or train on new medical guidelines, so they cannot answer more specialized questions in relevant disease areas. As shown in Figure 1, regarding the “eradication therapy for H. pylori,” ChatGPT’s response is vague and does not specifically address the specific scope and usage of dual, triple, or quadruple therapy regimens. Additionally, different hospitals and departments have different patient education content, such as where to pick up medications, how to schedule appointments with doctors, fee standards, etc., which LLMs cannot answer. As shown in Figure 2, ChatGPT cannot provide answers to this type of content. Currently, many studies have focused on how to use LLM to solve specific disease problems, but such research has found that the answers from LLM lack sufficient professionalism and cannot be compared to those from senior doctors (7–10). How to transform LLM to make its answers more domain-specific is currently a research hotspot.
There are three general methods and technologies to achieve the “specialization” of LLM: (1) Fine-tuning the original LLM model, which requires a large amount of computational resources that are generally unaffordable for hospital departments (11). (2) Using professional prompt words within LLM, which can only handle a small amount of data and requires users to constantly modify inputs (12–14). (3) Retrieval-augmented generation technology (RAG) (15–17).
In the era of large language models, RAG specifically refers to models retrieving relevant information from a vast document library when answering questions or generating text. Subsequently, this retrieved information is utilized to generate answers or text, thereby enhancing prediction accuracy. The RAG method allows developers to avoid retraining the entire large model for each specific task. Instead, they can provide additional information input to the model by attaching a knowledge base, thereby improving the accuracy of its responses. The RAG method is particularly suitable for tasks that require a large amount of knowledge. In this study, we adopted the RAG approach, collecting guidelines and internal departmental data related to gastroenterology and gastrointestinal endoscopy as a knowledge base. We combined Microsoft’s Azure OpenAI service as the large model to build an application for answering patient questions related to gastrointestinal endoscopy, known as Endo-chat.
Method
Ontology construction
Commonly used gastroenterology guidelines were downloaded from Wanfang and CNKI databases, and then integrated with internal departmental data to establish a knowledge base consisting of 17 documents (18–23, 27–36). The specific composition is shown in Table 1. Since the input knowledge base length for large models was limited, we had to divide the documents into smaller text blocks. Python 3.10 and llama index version 0.9.8 were used as the programming language and tool library (24). The Sentence Window Node Parser method was employed to split the documents, yielding a total of 752 knowledge base entries. After data preprocessing, each guideline entering the knowledge base has approximately 12,000 characters, totaling 194,582 characters. The internal departmental information has a total of 54,813 characters, making the grand total about 200,065 characters.
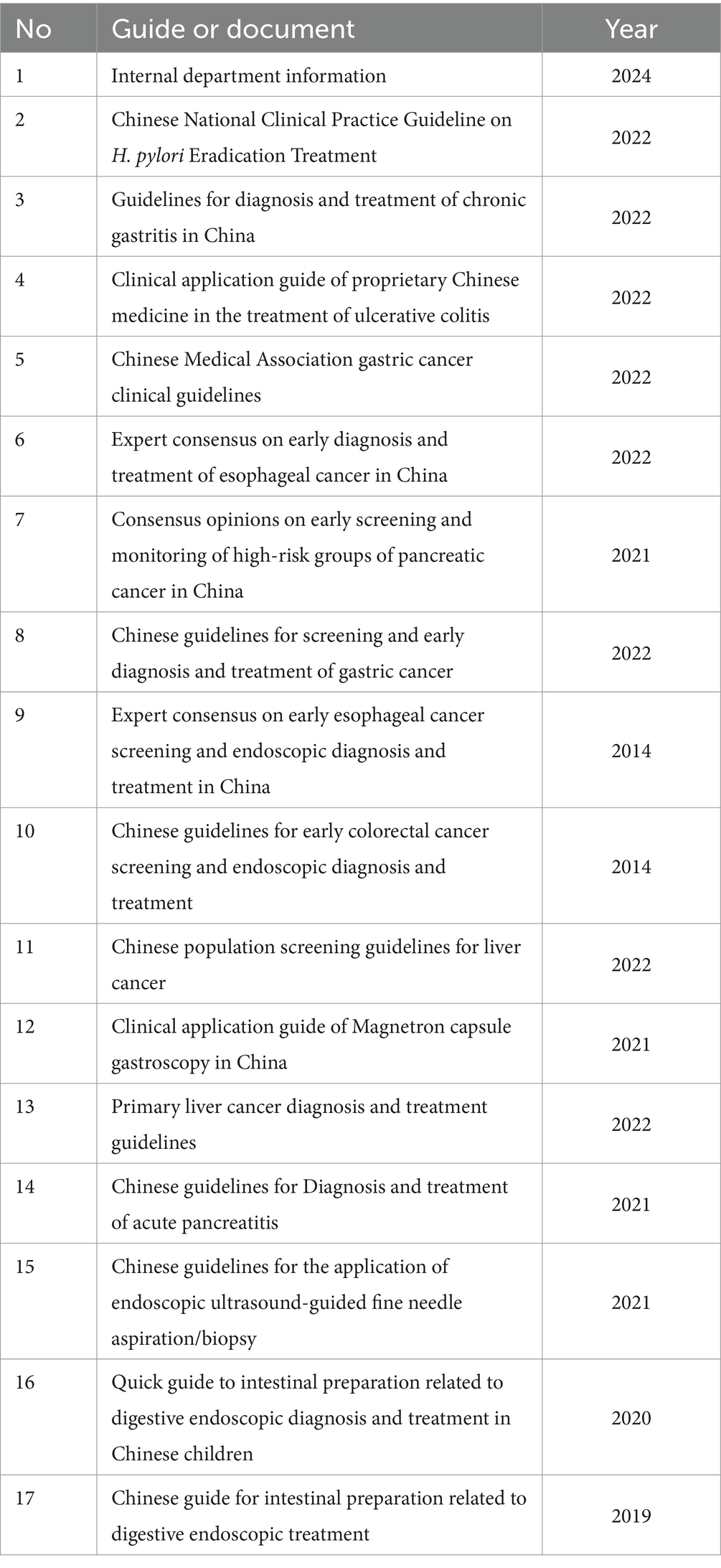
Table 1. Clinical guidelines and expert consensus related to digestive endoscopy included in the model.
RAG framework construction
Integrating documents (Figure 3) was achieved through the utilization of the application programming interface (API) provided by Microsoft Azure OpenAI (15). In the preprocessing stage, the 752 knowledge base entries were embedded using the text-embedding-ada-002 model to convert the text into vector numerical representations. These vector values were further stored in a vector database, with Faiss database being used in this study. When patients input questions, such as “How to prepare for a colonoscopy,” they also undergo text embedding using the text-embedding-ada-002 model. The vector values of the patient’s input question are matched for similarity with the vector numerical values of the knowledge base entries in the vector database, filtering out the most relevant knowledge base entries. For example, a matched entry could be: “The method for colonoscopy preparation is as follows, dietary preparation…” We will retrieve 10 relevant knowledge articles and then let the large model determine which content to use as background knowledge to answer the user’s question. Finally, the patient’s question and the retrieved knowledge base entry are sent to the LLM, which answers the patient’s question based on prompt words and the knowledge base. The LLM selected for our Endo-chat is the gpt-3.5-turbo-16 k model.
Interactive interface construction and effect evaluation
A user-friendly chat interface was designed using the streamlit tool library to enhance communication with patients (25). This interface, shown in Figure 4, enables patients to log in through a web link on their mobile phones or computers to inquire about any concerns they may have. To quantitatively evaluate the advantages of AI applications over traditional nurse manual responses to patient questions, we evaluate from the following aspects, as detailed in the supplement: (1) Efficiency Response time: comparing the average time required for AI robots and nurses to answer questions. (2) Accuracy Information accuracy: comparing the accuracy of the answers provided by the two methods through professional evaluation. Information completeness: evaluating whether the answers provided by the two methods comprehensively cover the patients’ inquiries. (3) Patient Satisfaction survey: assessing patient satisfaction with AI robots and manual services through a questionnaire survey. Preference test: asking patients which type of service they would prefer to use in the future. (4) Impact on nursing staff Workload: evaluating the changes in the workload of nursing staff after the implementation of AI robots. Job satisfaction: investigating the attitudes of nursing staff towards the introduction of AI robots and its impact on job satisfaction. 200 patients were randomly divided into two groups of 100 each to undergo gastrointestinal endoscopy examinations for a questionnaire survey.
Results
To analyze the responses of Endo-chat and ChatGPT, we posed some typical gastroenterology questions to both. The comparison of their answers is presented in Table 2, where we focused on 5 specific questions. For questions with clear personalization, such as “How much does a gastrointestinal endoscopy cost,” ChatGPT is very cautious and provides answers that lack useful information. The Endo-chat, after matching with the department’s internal knowledge base, can provide very accurate answers. For questions with some level of personalization, such as “How should individuals preparing for a morning colonoscopy proceed,” ChatGPT’s responses were vague and lack practical significance, while Endo-chat provided specific and accurate answers. As for questions with higher levels of expertise, such as “Please introduce the treatment plan for eradicating H. pylori” and “How often should follow-up visits be conducted for a diagnosis of atrophic gastritis” Endo-chat combined the latest guidelines to provide precise answers, with response quality far superior to ChatGPT. To more thoroughly evaluate the performance of Endo-chat and ChatGPT in this specific field, we selected 200 questions and had them answered using both AI methods. Experienced doctors were invited to assess the responses from the two AIs, focusing on the accuracy and level of detail of the answers. Since the approach was akin to a competition, it was not suitable to use the chi-square test. Instead, we assigned “Win,” “Tie,” or “Lose” judgments for each comparison. “Win” indicated that Endo-chat provided a superior response, “Lose” meant that ChatGPT offered a better response, and “Tie” implied that there was no significant difference between the two answers. According to Table 3, in terms of accuracy, Endo-chat won in 70% of the 200 cases, and tied with ChatGPT in 26.5% of the cases. In terms of the level of detail in the responses, Endo-chat won 94.5% of the cases, and tied in 4%. In both accuracy and the level of detail in responses, Endo-chat significantly outperformed ChatGPT.
A questionnaire evaluation was performed on two approaches, AI and manual, as outlined in Table 4. There were no statistically significant differences in age and gender between the two groups of patients. In terms of response efficiency, completeness, and patient satisfaction, AI was significantly superior to the manual method. In terms of response accuracy, the manual group, which was considered to have very accurate answers, accounted for 91%, while the AI group accounted for 82%. There was no significant difference between the two groups (p = 0.085). For each participant, we provided a detailed introduction to both the AI and manual methods, so participants had a certain degree of understanding of the other method. Regarding the question “which services do you prefer to use in the future?” we are investigating whether participants are dissatisfied with the method they are currently using, rather than which method they prefer. Among the participants in the manual group, 45% were willing to try the method of the AI group. However, only 20% of the participants in the AI group were willing to switch to the manual method. There was a significant statistical difference between the two (p < 0.001). Both groups of participants held a supportive attitude towards the introduction of AI, and there was no statistical difference between the two groups (p = 0.2485). All nurses participating in the questionnaire believed that introducing AI could reduce nursing workload. In open-ended questions, the most common suggestion from patients was to introduce a combination of AI and manual methods, where manual intervention could be provided for questions that AI could not answer or had doubts about. It is evident that the use of AI methods significantly helps improve nursing efficiency and enhance patient satisfaction.
Discussion
In the clinical work of digestive endoscopy, nurses often need to answer a large number of questions regarding preoperative, postoperative, diet, follow-up, etc., which consumes a significant amount of working time. For routine examination patients, these questions can be answered using standardized language or procedures, for example, the question “How should I prepare for a gastrointestinal endoscopy?” can be answered based on the department’s internal processes. However, for patients with different diagnoses, a certain level of medical expertise is required. For example, the question “How long should I wait for a follow-up colonoscopy?” would need personalized advice based on clinical guidelines. Research has shown that using large-scale language models (such as ChatGPT) for medical Q&A in clinical practice can improve the efficiency of medical and nursing work. However, there are two major obstacles to the use of large language models in the medical field: one is that these models may generate “fictional” or seemingly credible but incorrect answers, which is an inevitable issue with large models; the other is the inability to answer personalized questions related to specific departments, personal health information, etc. Due to the low tolerance for errors in medical Q&A, these two issues need to be addressed urgently. Therefore, we combined RAG with LLM to create the Endo-chat Q&A application, which not only alleviates the above two issues to a large extent but also significantly improves nursing work in clinical practice.
In our experiment, the specific practice method involved nurses replying to patient questions in a WeChat group, which consumed a significant amount of nursing effort. Additionally, nurses were unable to provide detailed answers to multiple questions from multiple patients simultaneously. In contrast, the advantages of the AI method are evident. Endo-chat is a 24/7 online robot that can provide immediate feedback to patient questions. Due to the use of the RAG technology framework, its response accuracy is comparable to that of humans, and it can provide more detailed and comprehensive answers than manual responses.
This study also has certain limitations. (1) The RAG framework may generate incorrect answers due to inaccurate retrieval of knowledge articles, although this possibility is small. As shown in Figure 3, the construction of Endo-chat can be divided into three parts: ① Creating a knowledge base using document segmentation; ② Creating a vector database; ③ Matching and retrieving questions with the knowledge base. The retrieved knowledge articles and questions are entered into the LLM for answer generation. If the retrieval of knowledge articles is inaccurate and LLM answers based on incorrect knowledge, it may lead to an incorrect answer. This is why there were 5 cases of inaccurate AI responses in the question “How accurate do you think the answers are?” in Table 4. To minimize the occurrence of inaccurate recalls, we drew inspiration from the Self-RAG (26) approach. We first recalled 10 texts based on the similarity between the question and the knowledge passage embeddings. We then allowed the LLM to analyze in parallel whether these 10 texts were relevant to the question, ultimately only incorporating the relevant knowledge passages as the background for Endo-chat’s responses. For example, if the user input is “Hello, who are you?” Endo-chat will not adopt any knowledge passages. Conversely, if all 10 passages recalled are closely related to the question, Endo-chat will adopt all of them. The question of how many texts need to be recalled to obtain the best response is an engineering problem, which depends on the total number of knowledge passages, the required response speed, and the context length limit of the LLM. Building a higher-quality knowledge base and achieving more precise recalls is an area worth exploring in the future. (2) LLM may also fail to follow instructions, even when the correct knowledge articles are input, LLM may not answer according to the knowledge articles. This may be due to the preference selection during LLM training. (3) This study only included 17 documents and cannot cover all content in the field of gastroenterology. LLM can only accurately answer the content contained in these documents. If a patient asks about content outside the documents, such as “What should I do for chronic diarrhea” LLM can only answer based on its own capabilities, resulting in a general response. (4) Due to the use of an external API, Endo-chat may not be able to respond when there are many concurrent requests. This can explain the question “How long does it take to receive an answer after asking the AI robot/nurse?” where in some cases, AI cannot respond promptly.
In conclusion, combining RAG technology and using LLM for medical vertical domain Q&A is a meaningful clinical practice. Our next step could involve optimizing the RAG framework, expanding the content of the knowledge base, and making Endo-chat responses more accurate and applicable.
Data availability statement
The raw data supporting the conclusions of this article will be made available by the authors, without undue reservation.
Ethics statement
The studies involving humans were approved by Ethics Committee of Guangdong Provincial Hospital of Chinese Medicine. The studies were conducted in accordance with the local legislation and institutional requirements. The participants provided their written informed consent to participate in this study.
Author contributions
ZF: Writing – original draft, Writing – review & editing. SF: Data curation, Methodology, Software, Writing – original draft. YH: Conceptualization, Data curation, Methodology, Writing – review & editing. WH: Investigation, Supervision, Writing – review & editing. ZZ: Data curation, Investigation, Methodology, Writing – review & editing. YG: Data curation, Methodology, Writing – original draft. YL: Resources, Supervision, Writing – review & editing.
Funding
The author(s) declare that no financial support was received for the research, authorship, and/or publication of this article.
Conflict of interest
The authors declare that the research was conducted in the absence of any commercial or financial relationships that could be construed as a potential conflict of interest.
Publisher’s note
All claims expressed in this article are solely those of the authors and do not necessarily represent those of their affiliated organizations, or those of the publisher, the editors and the reviewers. Any product that may be evaluated in this article, or claim that may be made by its manufacturer, is not guaranteed or endorsed by the publisher.
Supplementary material
The Supplementary material for this article can be found online at: https://www.frontiersin.org/articles/10.3389/fmed.2024.1500258/full#supplementary-material
References
1. Yeo, YH, Samaan, JS, Ng, WH, Ting, PS, Trivedi, H, Vipani, A, et al. Assessing the performance of ChatGPT in answering questions regarding cirrhosis and hepatocellular carcinoma. Clin Mol Hepatol. (2023) 29:721–32. doi: 10.3350/cmh.2023.0089
2. Ge, J, Li, M, Delk, MB, and Lai, JC. A comparison of a large language model vs manual chart review for the extraction of data elements from the electronic health record. Gastroenterol. (2024) 166:707–709. e3. doi: 10.1053/j.gastro.2023.12.019
3. Nayak, A, Alkaitis, MS, Nayak, K, Nikolov, M, Weinfurt, KP, and Schulman, K. Comparison of history of present illness summaries generated by a chatbot and senior internal medicine residents. JAMA Intern Med. (2023) 183:1026–7. doi: 10.1001/jamainternmed.2023.2561
4. Han, C, Kim, DW, Kim, S, You, S.C., Park, J.Y., Bae, S., et al. Evaluation of GPT-4 for 10-year cardiovascular risk prediction: Insights from the UK biobank and KoGES data. iScience. (2024) 27:109022. doi: 10.1016/j.isci.2024.109022
5. Ji, Z, Lee, N, Frieske, R, Yu, T, Su, D, Xu, Y, et al. Survey of hallucination in natural language generation. ACM Comput Surv. (2022) 55:1–38. doi: 10.1145/3571730
6. Landhuis, E. Scientific literature: information overload. Nature. (2016) 535:457–8. doi: 10.1038/nj7612-457a
7. Gravina, AG, Pellegrino, R, Cipullo, M, Palladino, G, Imperio, G, Ventura, A, et al. ChatGPT be a tool producing medical information for common inflammatory bowel disease patients’ questions? An evidence-controlled analysis. World J Gastroenterol. (2024) 30:17–33. doi: 10.3748/wjg.v30.i1.17
8. Gong, EJ, and Bang, CS. Evaluating the role of large language models in inflammatory bowel disease patient information. World J Gastroenterol. (2024) 30:3538–40. doi: 10.3748/wjg.v30.i29.3538
9. Hirosawa, T, and Shimizu, T. Enhancing clinical reasoning with chat generative pre-trained transformer: a practical guide. Diagnosi. (2024) 11:102–5. doi: 10.1515/dx-2023-0116
10. Aburumman, R, al Annan, K, Mrad, R, Brunaldi, VO, Gala, K, and Abu Dayyeh, BK. Assessing ChatGPT vs. standard medical resources for endoscopic sleeve Gastroplasty education: a medical professional evaluation study. Obes Surg. (2024) 34:2718–24. doi: 10.1007/s11695-024-07283-5
11. Gao, Y, Xiong, Y, Gao, X, Jia, K, Pan, J, Bi, Y, et al. Retrieval-augmented generation for large language models: a survey. arXiv. (2023) 10997. doi: 10.48550/arXiv.2312.10997
12. Kojima, T, Gu, SS, Reid, M, Matsuo, Y, and Iwasawa, Y. Large language models are zero-shot reasoners. arXiv. (2022)
14. Parnami, A, and Lee, M. Learning from few examples: a summary of approaches to few-shot learning. arXiv. (2022)
15. RAG and generative AI-Azure Cognitive Search|Microsoft learn. Available at: https://learn.microsoft.com/en-us/azure/search/retrieval-augmented-generation-overview (accessed November 8, 2023).
16. Wang, Y, Ma, X, and Chen, W. Augmenting black-box LLMs with medical textbooks for clinical question answering. arXiv. (2023)
17. Lozano, A, Fleming, SL, Chiang, C-C, and Shah, N. Clinfo.Ai: an open-source retrieval-augmented large language model system for answering medical questions using scientific literature. arXiv. (2023)
18. Helicobacter pylori Group, Chinese Society of Gastroenterology. Chinese guidelines for the treatment of Helicobacter pylori infection. Chin J Gastroenterol. (2022) 27:150–62. doi: 10.1097/CM9.0000000000002546
19. Digestive System Tumor Collaboration Group, Chinese Society of Gastroenterology. Guidelines for diagnosis and treatment of chronic gastritis in China (2022, Shanghai). Chin J Dig. (2023) 415–28. doi: 10.1111/1751-2980.13193
20. Clinical Application Guidelines for the Treatment of Superior Diseases of Proprietary Chinese Medicine" Standardization Project Team. Clinical guidelines for the treatment of ulcerative colitis with proprietary Chinese medicines (abbreviated version, 2022). Chin J Dig. (2022) 42:17–26. doi: 10.3760/cma.j.cn311367-20220914-00448
21. Society of Oncology, Chinese Medical Association. Chinese Medical Association clinical diagnosis and treatment guidelines for gastric Cancer (2021 edition). Chin Med J. (2022) 102:21. doi: 10.3760/cma.j.cn112137-20220127-00197
22. Early diagnosis and Early Treatment Group, Society of Oncology, Chinese Medical Association. Expert consensus on early diagnosis and treatment of esophageal cancer in China. Chin J Oncol. (2022) 44:10. doi: 10.3760/cma.j.cn112152-20220220-00114
23. Pancreatic Disease Collaboration Group, Digestive Endoscopy Branch, Chinese Medical Association. Consensus opinion on early screening and surveillance of high-risk groups of pancreatic cancer in China (Nanjing, 2021). Chin J Gastroenterol. (2022) 27:415–28.
24. LlamaIndex, Data framework for LLM applications. Available at: https://www.llamaindex.ai/ (accessed March 5, 2024).
25. Streamlit. A faster way to build and share data apps. Available at: https://streamlit.io/ (accessed March 5, 2024).
26. Asai, A, Wu, Z, Wang, Y, et al. Self-rag: learning to retrieve, generate, and critique through self-reflection. arXiv. (2023) 2310:11511
27. He, J, Chen, WQ, Li, ZS, Li, N, Ren, JS, Tian, JH, et al. Chinese guidelines for screening and early diagnosis and treatment of gastric Cancer (Beijing, 2022). China Cancer. (2022) 31:40. doi: 10.3760/cma.j.cn115610-20220624-00370
28. Association of Digestive Endoscopy, Chinese Society of Medicine, Professional Committee of Tumor Endoscopy, Chinese Anticancer Association. Expert consensus on early esophageal cancer screening and endoscopic diagnosis and treatment in China (2014, Beijing). Chin J Dig Endosc. (2015) 84–92. doi: 10.3760/cma.j.issn.1007-5232.2015.04.001
29. Bo, Y, Yang, F, Ma, D, and Zou, WB. Guidelines for early colorectal Cancer screening and endoscopic diagnosis and treatment in China. Chin J Gastroenterol. (2015) 20:21. doi: 10.3760/cma.j.issn.1007-5232.2015.06.001
30. He, J, Chen, WQ, Shen, HB, Li, N, Qu, CF, Shi, JF, et al. Screening guidelines for liver Cancer in Chinese population (Beijing, 2022). China Cancer. (2022) 8:587–631. doi: 10.3760/cma.j.cn112152-20220720-00502
31. National Clinical Research Center for Digestive Diseases (Shanghai), National Digestive Endoscopy Quality Control Center, Capsule Endoscopy Collaboration Group, Digestive Endoscopy Society, Chinese Medical Association, et al. Guidelines for clinical application of magnetron capsule gastroscopy in China (condensed version,2021, Shanghai). Chin J Dig Endosc. (2021) 38:15. doi: 10.3760/cma.j.cn311367-20210522-00296
32. General Office of the National Health Commission of China. Primary liver cancer diagnosis and treatment guidelines. Chin J Clin Hepatol. (2022) 38:288–303. doi: 10.3969/j.issn.1001-5256.2022.02.009
33. Pancreatic Surgery Group, Surgical Society of Chinese Medical Association. Chinese guidelines for diagnosis and treatment of acute pancreatitis. Chin J Surg. (2021) 59:10. doi: 10.3760/cma.j.cn112139-20210416-00172
34. Chinese Medical Doctor Association Endoscopic ultrasound expert Committee. Application guidelines for endoscopic ultrasound-guided fine needle aspiration/biopsy in China (2021, Shanghai). Chin J Dig Endosc. (2021) 38:24. doi: 10.3760/cma.j.cn321463-20210302-00143
35. Pediatric Collaboration Group, Digestive Endoscopy Society, Chinese Medical Association, Specialty Committee of Pediatric Digestive Endoscopy, Endoscopy Branch of Chinese Medical Doctor AssociationLinhu, EQ, et al. Quick guide for intestinal preparation related to the diagnosis and treatment of digestive endoscopy in Chinese children (Xi'an, 2020). Chin J Evid Based Med. (2021) 21:11. doi: 10.7507/1672-2531.202012004
36. Li, ZS, and Linhu, EQ. Chinese guide for intestinal preparation related to digestive endoscopic treatment (2019, Shanghai). Chin J Dig. (2019) 39:6. doi: 10.3760/cma.j.issn.1007-5232.2019.07.001
Appendix: Questionnaire survey scale
Section 1: Basic information
1. Your age:
• 18–30
• 31–45
• 46–60
• 61 and above
2. Your gender:
• Male
• Female
• Other
• Prefer not to say
3. Your colonoscopy date:
• ____ (Please fill in the date)
Section 2: Service efficiency
1. How long did it take for you to receive a response after asking a question to the AI robot/nurse?
• Immediately
• Less than 5 minutes
• 5–30 minutes
• More than 30 minutes
Section 3: Accuracy and completeness
1. How accurate do you think the answers were?
• Very accurate
• Accurate
• Somewhat accurate
• Inaccurate
2. Did the answer fully cover your questions?
• Completely covered
• Mostly covered
• Partially covered
• Hardly covered
Section 4: Patient satisfaction
1. How satisfied are you with the overall service?
• Very satisfied
• Satisfied
• Neutral
• Dissatisfied
2. Which service would you prefer to use in the future?
• AI robot
• Nurse manual
• No preference
• Undecided
Section 5: Impact on nursing staff (if applicable)
1. If you are a nursing staff, how has your workload changed after the introduction of the AI robot?
• Significantly reduced
• Slightly reduced
• No change
• Slightly increased
• Significantly increased
2. What is your attitude towards the introduction of the AI robot?
• Strongly support
• Support
• Neutral
• Oppose
• Strongly oppose
Section 6: Open-ended questions
1. What suggestions or comments do you have for the AI robot service?
• _____________________________________________
2. What suggestions or comments do you have for the nurse manual service?
• ______________________________________________
Keywords: large language model, retrieval enhanced generation technology, digestive endoscopic nursing, questionnaire survey scale, ChatGPT
Citation: Fu Z, Fu S, Huang Y, He W, Zhong Z, Guo Y and Lin Y (2024) Application of large language model combined with retrieval enhanced generation technology in digestive endoscopic nursing. Front. Med. 11:1500258. doi: 10.3389/fmed.2024.1500258
Edited by:
Antonietta G. Gravina, University of Campania Luigi Vanvitelli, ItalyReviewed by:
Raffaele Pellegrino, University of Campania Luigi Vanvitelli, ItalyZhichao Yang, University of Massachusetts Amherst, United States
Copyright © 2024 Fu, Fu, Huang, He, Zhong, Guo and Lin. This is an open-access article distributed under the terms of the Creative Commons Attribution License (CC BY). The use, distribution or reproduction in other forums is permitted, provided the original author(s) and the copyright owner(s) are credited and that the original publication in this journal is cited, in accordance with accepted academic practice. No use, distribution or reproduction is permitted which does not comply with these terms.
*Correspondence: Yanfeng Lin, bGlueWFuZmVuZzE5OTdAZ3p1Y20uZWR1LmNu
†These authors have contributed equally to this work and share first authorship