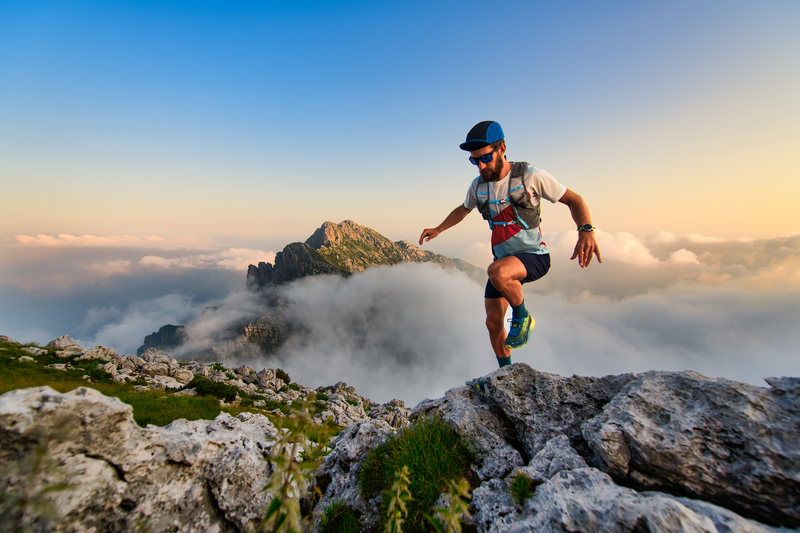
95% of researchers rate our articles as excellent or good
Learn more about the work of our research integrity team to safeguard the quality of each article we publish.
Find out more
REVIEW article
Front. Med. , 02 September 2024
Sec. Precision Medicine
Volume 11 - 2024 | https://doi.org/10.3389/fmed.2024.1467289
This article is part of the Research Topic Exploring Novel Peptide-Drug Conjugates for Overcoming Chemotherapeutic Resistance View all articles
Inadequate bioavailability of therapeutic drugs, which is often the consequence of their unacceptable solubility and dissolution rates, is an indisputable operational challenge of pharmaceutical companies due to its detrimental effect on the therapeutic efficacy. Over the recent decades, application of supercritical fluids (SCFs) (mainly SCCO2) has attracted the attentions of many scientists as promising alternative of toxic and environmentally-hazardous organic solvents due to possessing positive advantages like low flammability, availability, high performance, eco-friendliness and safety/simplicity of operation. Nowadays, application of different machine learning (ML) as a versatile, robust and accurate approach for the prediction of different momentous parameters like solubility and bioavailability has been of great attentions due to the non-affordability and time-wasting nature of experimental investigations. The prominent goal of this article is to review the role of different ML-based tools for the prediction of solubility/bioavailability of drugs using SCCO2. Moreover, the importance of solubility factor in the pharmaceutical industry and different possible techniques for increasing the amount of this parameter in poorly-soluble drugs are comprehensively discussed. At the end, the efficiency of SCCO2 for improving the manufacturing process of drug nanocrystals is aimed to be discussed.
Over the recent decades, the manufacturing process of innovative, affordable and effective therapeutic agents with optimum physicochemical and biological profiles has been the most important mission of scientists in the pharmaceutical industry (1–4). Despite great importance and significant endeavors, pharmaceutical companies have currently faced with different challenges like patent considerations, decrement in the number of novel marketed products, time/cost-intensiveness of R&D regulatory pathways toward the advancement of Active Pharmaceutical Ingredients (APIs) and their appropriate dosage of administration (5–9). It is momentous to note that some operational/functional parameters like bioavailability, solubility, dissolution rate, permeability and morphology can considerably influence the physicochemical property and therefore, the therapeutic efficacy of each determined drug (10–12). Nearly 65 to 75% of prevalent APIs are recently in solid structure or consists of solid APIs formulated as solid suspensions. Owing to the presence of important characteristics like simplicity of synthesis, higher physicochemical stability and crystallinity, formation of APIs in the solid state seems to be more appropriate. It is worth mentioning that safety, medical efficacy, physicochemical stabilities and sufficient solubility in aqueous media can be considered as momentous parameters for the development of novel API (13–16). In the current years, application of novel types of ionic liquids (ILs) in the pharmaceutical industry has paved the way for more efficient delivery of drugs. These novel, effective and green compounds have shown their great potential to deal with the challenges related to conventional dosage forms like insufficient solubility and low permeability in topical drug delivery systems (17, 18).
Drug formulation is usually implemented by the combination of inert excipients with active APIs for the production of highly-effective drug with favorable therapeutic efficacy. In the current years, serious endeavors are being made to improve/optimize the momentous parameters related to drug formation for decreasing side effects and enhancing API stability and patient compliance (19–22). On the basis of appropriate route of administration, formulation of APIs can be done via disparate set of materials like inert excipients (i.e., polymers, surfactants) and in an extensive type of delivery systems including microparticles (MPs) and nanoparticles (NPs) (7, 23–26). On-time innovation of highly-efficacious therapeutic agents to the market depends on the advancement of promising drug delivery systems. Despite the great success of conventional approaches for the formulation and discovery of effective drugs for patients, the emergence of several challenges like high cost and long process time has restricted their application in pharmacology (27–30). In doing so, finding novel, time-saving and affordable techniques for the prediction of drug properties is of great necessity.
Machine learning (ML) can be interpreted as a subdivision of artificial intelligence (AI) that has illustrated its brilliant potential of utilization for the prediction of momentous parameters in different processes via training computational models on the basis of a body of data (25, 31, 32). Over the last years, artificial intelligence (AI) has substantially improved the formulation /development of novel therapeutic agents. Using AI, scientists are now able to optimize the formulation of drugs and increase the accuracy of clinical trials (33–35). For example, ML can facilitate the stability prediction of a special drug formulation by the consideration of data from numerous experimental investigations that evaluated the stability of API formulations. Current developments in ML algorithms as well as the advancement of robust and cost-effective have substantially enhanced availability to precise ML-based models (36–38). The abovementioned advancements have eventuated in the emergence of unbelievable tendency in the real-world utilization of different ML/AI-based algorithms in several industrial aspects like gas separation, cancer diagnosis, membrane processes, absorption/adsorption, catalysis and pharmaceuticals (39–45). Other noteworthy utilization of ML-based algorithms are the use of supervised learning algorithms and deep reinforcement learning for the estimation of momentous parameters in chemical reactions and the employment of deep learning (DL) to specify the 3D structure of a protein from its amino acid sequence (46–50). Figure 1 schematically demonstrates various approaches of ML.
Figure 1. Various approaches of ML (106).
This paper aims to review the role of disparate ML-based tools for the prediction of solubility/bioavailability of drugs using SCCO2. Moreover, the importance of solubility factor in the pharmaceutical industry and different possible techniques for increasing the amount of this parameter in poorly-soluble drugs are comprehensively discussed. At the end, the efficiency of SCCO2 for improving the manufacturing process of drug nanocrystals is aimed to be discussed.
Solubility may be well considered as a momentous physical property and is defined as the ability of a specific solvent absorption in solvents. This factor is extensively employed in disparate scientific fields like liquid–liquid extraction, membrane-based separation, drug delivery, material science and medicine (51–56). Poor solubility of therapeutic medicines in water can be regarded as an important challenge in the pharmaceutical industry, which may lead to reducing the bioavailability and therapeutic performance. This challenge has motivated the researchers to develop novel ways to overcome this problem (56–58). Figure 2 schematically presents different common techniques for increasing the solubility of poorly-soluble drugs.
Figure 2. Schematic presentation of common techniques for enhancing the solubility of poorly-soluble drugs (57).
To conduct the scientific researches in the scientific scope of solubility, experimental analysis and computer simulation must be incorporated. Owing to the presence of various challenges during the conduction of investigational experiments under high temperature/pressure, particularly accompanying with stirring and vibration (with the aim of accelerating solubility), scientists have made their efforts to use accurate techniques for the prediction of momentous parameters related to drug development (59, 60). Over the last decades, development of predictive modeling tools has attracted the attentions of academic/industrial researchers. For instance, three eminent scientists (Martin Karplus, Michael Levitt, and Arieh Warshel) were the winner of the 2013 Nobel Prize in chemistry for the advancement of multiscale models for complicated chemical systems (61). The multiscale technique applies disparate time and space scales for the investigation of prevalent problem in all the micro-, meso- and microspatial scales (62–64). Figure 3 schematically demonstrates the employed models and methods of multiscale model.
Figure 3. Schematic demonstration of employed models and methods of multiscale model. Reprinted from (54) with permission from Elsevier.
It is of great importance to note the fact that the solubility of therapeutic drugs at the macroscale and its rate at the micro/meso scales results in the emergence of important challenges to the conventional research procedures (54, 65). The multiscale solubility investigation can be regarded as one of the most efficient procedures to show the inherence of dissolution process. This type of study has recently demonstrated great potential for the prepare scientific reference for process parameter selection at the multiscale level. This technique possesses great potential in various scientific scopes like self-assembly and dynamic evaluation of polymers (66, 67).
The ongoing approach toward the development of novel drugs must be on the basis of the quality by design principles (QbD) (68–70). The primary level toward the implementation of this aspect is the interpretation of the quality target product profile (QTPP) and the critical quality attributes (CQA) of the product. By the identification of the relationships, the appointment of design space takes place, which provides an appropriate opportunity to optimize/control the product’s quality (71–75). From the past till now, quantitative evaluation and appointment of the design space were on the basis of experimental design, regression procedures and prevalent statistical analysis. ML algorithms [particularly Artificial neural network (ANN)], have been applied in different complex examples of QbD-based pharmaceutical development. Extensive application of ANN in pharmaceutical industry is prominently due to their brilliant characteristics like non-linear nature and great capability to make complex relationships between CMAs or CPPs with CQAs for disparate pharmaceutical dosage forms (76–81). For instance, Simões et al. (77) have assessed the role of using ANN predictive model based on ML approach for the QbD-based advancement of a poorly soluble therapeutic medicine fabricated in industrial settings and its comparison with the reference product and bioequivalence investigations. To implement this research, ANN was constructed using only 5 hidden nodes in 1 hidden layer. Development of this predictive model was on the basis of the use of hyperbolic tangent functions and its validation was corroborated by a random holdback of 33% of the dataset. Application of this model resulted in the emergence of valid prediction formulas for all 3 responses, with R2 values higher than 0.94 for training and validation datasets (78).
In a comprehensive scientific investigation, Belič et al. employed ANNs and fuzzy models to evaluate the impact of particle size and tableting parameters on the tablet capping tendency. The results demonstrated the fact that the developed model-based expert systems can dramatically enhance the trial-and-error procedures (82). Lee et al. developed an ML-based approach for the identification of emerging techniques at early stages applying immediately-defined multiple patent indicators. They also successfully applied a feed-forward multilayer neural network to provide the nonlinear connection between input and output indicators. They concluded that the developed approach could appropriately facilitate the responsive technology forecasting and planning in the pharmaceutical industry (83, 84). In the current years, the supremacy of deep learning (DL) than commonly-applied ML approaches has been recently approved (50, 85, 86). DL has illustrated their great potential of application in different industrial approaches such as the prediction of solubility and drug release (28, 87, 88). Apart from DL technique, other cutting-edge and breakthrough ML-based algorithms such as Light gradient boosting machine algorithm (lightGBM) have illustrated their noteworthy efficiency in the prediction of functional/operational parameters and complexation between cyclodextrins and APIs (89, 90). Decision tree (DT)-based techniques have shown their great potential to predict particle size of solid lipid nanoparticles (91). Figure 4 schematically depicts the incorporation potential of AI with drug development and research.
Figure 4. Schematic demonstration of AI contribution with drug development and research (88).
The use of supercritical fluids for the formulation of drugs has recently attracted great attentions as an outstanding alternative for commonly-applied organic solvents owing to its brilliant positive points like non-toxicity, affordability and great efficiency (92–94). Rapid Expansion of Supercritical Solutions (RESS) is considered as the first technique of particle formation initially developed in the 1980’s (95). Despite the extensive application of disparate organic solvents (i.e., chlorodifluoromethane, trifluoromethane and ethane), A prevalent SCF in RESS is SCCO2 (96–98). Table 1 enlists the role of SCFs (especially CO2) solvents for the synthesis of the micro/nano amorphous/crystalline particles and micro/nano cocrystals using RESS method.
Table 1. Formation of micro/nano amorphous/crystalline particles and micro/nano cocrystals using RESS method.
In the recent decades, pharmaceutical industry has encountered with different challenges. One of the most important concerns of scientists is the unacceptable solubility of therapeutic agents in water, which significantly affects their bioavailability, dissolution rates and hence, reduces the therapeutic performance of approved drugs (99). There are two prominent approaches that are being extensively applied in current years to overcome inadequate drug solubility concerns. (1) Techniques related to particle size reduction of drugs (i.e., micronization/nanonization). (2) Modification of physicochemical/structural characteristics of poorly water-soluble drugs (5, 100, 101). Currently, application of SCFs (particularly SCCO2), have been of paramount attentions to enhance the solubility/bioavailability and therefore, the therapeutic efficiency of medicines. Great interest toward the employment of SCCO2 is owing to its disparate advantageous technical properties, as well as noteworthy technical features like greenness, safety, versatility, simplicity of operation and low flammability (58, 102–104). Another practical CO2-based methodology for the formulation/synthesis of medical-based nanoparticles/nanocrystals or co-precipitated drugs is the application of SCCO2 as namely as high mobility solutes or co-solvents. By changing the use of this SCF from solvents to antisolvents and additives, the required value of CO2 to prepare micro/nanoparticles and the size of equipment to allow the needed CO2 action is declined dramatically (105).
As mentioned in the manuscript, the integration of web innovation with medical science to enhance the precision and efficiency of predictive models in decision-making and deep learning algorithms. Thus, the motivation of scientists has recently been towards the use of different ML-based approaches in pharmaceutical industries. To reach this purpose, different ML-based approaches like support vector machine (SVM), multiple linear regression (MLR), radio frequency (RF) and deep learning techniques are being extensively implemented for the prediction of different momentous parameters like solubility and bioavailability. Over the last four decades, unique/tunable incorporation of brilliant and noteworthy physicochemical futures of SCCO2 with the growing regulatory needs and global requirements for more eco-friendly processes have increased the interest of researchers to develop and apply SCCO2-based bottom-up processes for enhancing the solubility and solubility of poorly-soluble therapeutic agents and forming drug-based nanoparticles/nanocrystals. This paper aimed to overview the role of different ML-based tools for the prediction of solubility/bioavailability of drugs using SCCO2. Moreover, the importance of solubility factor in the pharmaceutical industry and different possible techniques for increasing the amount of this parameter in poorly-soluble drugs are comprehensively discussed. At the end, the efficiency of SCCO2 for improving the manufacturing process of drug nanocrystals is aimed to be discussed. As the future perspective, different endeavors must be made to evaluate the rate of SCF-based reaction-mediated synthesis compared to conventional techniques. Moreover, the great potential of SCFs to manufacture environmentally-friendly and green extracts from plant substrates or industrial biowaste has motivated the researchers to pay more attention to the use of these solvents compared to organic solvents. Finding promising ways to facilitate the scale-up of these technologies can be another action, which can be under evaluation in the future for the production of pharmaceutical components, biowaste and different liquid extracts.
JA: Conceptualization, Data curation, Formal analysis, Investigation, Methodology, Writing – original draft.
The author(s) declare that no financial support was received for the research, authorship, and/or publication of this article.
The author declares that the research was conducted in the absence of any commercial or financial relationships that could be construed as a potential conflict of interest.
All claims expressed in this article are solely those of the authors and do not necessarily represent those of their affiliated organizations, or those of the publisher, the editors and the reviewers. Any product that may be evaluated in this article, or claim that may be made by its manufacturer, is not guaranteed or endorsed by the publisher.
1. Kostyrin, EV, Ponkratov, VV, and Al-Shati, AS. Development of machine learning model and analysis study of drug solubility in supercritical solvent for green technology development. Arab J Chem. (2022) 15:104346. doi: 10.1016/j.arabjc.2022.104346
2. Khoshmaram, A, Zabihi, S, Pelalak, R, Pishnamazi, M, Marjani, A, and Shirazian, S. Supercritical process for preparation of nanomedicine: oxaprozin case study. Chem Eng Technol. (2021) 44:208–12. doi: 10.1002/ceat.202000411
3. Pishnamazi, M, Taghvaie Nakhjiri, A, Sodagar Taleghani, A, Ghadiri, M, Marjani, A, and Shirazian, S. Molecular separation of ibuprofen and 4-isobutylacetophenone using octanol organic solution by porous polymeric membranes. PLoS One. (2020) 15:e0237271. doi: 10.1371/journal.pone.0237271
4. Yadav, S, Palei, NN, Dinda, SC, and Dhar, AK. Drug delivery in biotechnology: present and future In: S Bose, et al., editors. Concepts in pharmaceutical biotechnology and drug development. Singapore: Springer Nature Singapore (2024). 103–38.
5. Padrela, L, Rodrigues, MA, Duarte, A, Dias, AMA, Braga, MEM, and de Sousa, HC. Supercritical carbon dioxide-based technologies for the production of drug nanoparticles/nanocrystals–a comprehensive review. Adv Drug Deliv Rev. (2018) 131:22–78. doi: 10.1016/j.addr.2018.07.010
6. Douroumis, D, and Nokhodchi, A. Preface: engineering of pharmaceutical cocrystals, salts and polymorphs: advances and challenges. Adv Drug Deliv Rev. (2017) 117:1–2. doi: 10.1016/j.addr.2017.10.002
7. Vioglio, PC, Chierotti, MR, and Gobetto, R. Pharmaceutical aspects of salt and cocrystal forms of APIs and characterization challenges. Adv Drug Deliv Rev. (2017) 117:86–110. doi: 10.1016/j.addr.2017.07.001
8. Puhlmann, N, Vidaurre, R, and Kümmerer, K. Designing greener active pharmaceutical ingredients: insights from pharmaceutical industry into drug discovery and development. Eur J Pharm Sci. (2024) 192:106614. doi: 10.1016/j.ejps.2023.106614
9. Elveny, M, Khan, A, Nakhjiri, AT, and Albadarin, AB. A state-of-the-art review on the application of various pharmaceutical nanoparticles as a promising technology in cancer treatment. Arab J Chem. (2021) 14:103352. doi: 10.1016/j.arabjc.2021.103352
10. Pindelska, E, Sokal, A, and Kolodziejski, W. Pharmaceutical cocrystals, salts and polymorphs: advanced characterization techniques. Adv Drug Deliv Rev. (2017) 117:111–46. doi: 10.1016/j.addr.2017.09.014
11. Higashi, K, Ueda, K, and Moribe, K. Recent progress of structural study of polymorphic pharmaceutical drugs. Adv Drug Deliv Rev. (2017) 117:71–85. doi: 10.1016/j.addr.2016.12.001
12. Zhang, W, Taheri-Ledari, R, Ganjali, F, Mirmohammadi, SS, Qazi, FS, Saeidirad, M, et al. Effects of morphology and size of nanoscale drug carriers on cellular uptake and internalization process: a review. RSC Adv. (2023) 13:80–114. doi: 10.1039/D2RA06888E
13. Kale, DP, Zode, SS, and Bansal, AK. Challenges in translational development of pharmaceutical cocrystals. J Pharm Sci. (2017) 106:457–70. doi: 10.1016/j.xphs.2016.10.021
14. Kuminek, G, Cao, F, Bahia de Oliveira da Rocha, A, Gonçalves Cardoso, S, and Rodríguez-Hornedo, N. Cocrystals to facilitate delivery of poorly soluble compounds beyond-rule-of-5. Adv Drug Deliv Rev. (2016) 101:143–66. doi: 10.1016/j.addr.2016.04.022
15. Jennotte, O, Koch, N, Lechanteur, A, and Evrard, B. Three-dimensional printing technology as a promising tool in bioavailability enhancement of poorly water-soluble molecules: a review. Int J Pharm. (2020) 580:119200. doi: 10.1016/j.ijpharm.2020.119200
16. Taleghani, AS, Nakhjiri, AT, Khakzad, MJ, Rezayat, SM, Ebrahimnejad, P, Heydarinasab, A, et al. Mesoporous silica nanoparticles as a versatile nanocarrier for cancer treatment: a review. J Mol Liq. (2021) 328:115417. doi: 10.1016/j.molliq.2021.115417
17. Khan, O, Bhawale, R, Vasave, R, and Mehra, NK. Ionic liquid-based formulation approaches for enhanced transmucosal drug delivery. Drug Discov Today. (2024) 29:104109. doi: 10.1016/j.drudis.2024.104109
18. Bhat, AR, Padder, RA, Husain, M, and Patel, R. Development of Cholinium-based API ionic liquids with enhanced drug solubility: biological evaluation and interfacial properties. Mol Pharm. (2024) 21:535–49. doi: 10.1021/acs.molpharmaceut.3c00673
19. Bannigan, P, Aldeghi, M, Bao, Z, Häse, F, Aspuru-Guzik, A, and Allen, C. Machine learning directed drug formulation development. Adv Drug Deliv Rev. (2021) 175:113806. doi: 10.1016/j.addr.2021.05.016
20. Aulton, ME, and Taylor, K. Aulton's pharmaceutics: the design and manufacture of medicines Elsevier Health Sciences. London, United Kingdom: Churchill Livingstone-Elsevier (2013).
21. Barik, A, and Dhar, A. Introduction to different types of dosage forms and commonly used excipients In: Dosage forms, formulation developments and regulations. Elsevier (2024). 67–82.
22. Hyer, A, Gregory, D, Kay, K, le, Q, Turnage, J, Gupton, F, et al. Continuous manufacturing of active pharmaceutical ingredients: current trends and perspectives. Advanced Synthesis & Catalysis. (2024) 366:357–89. doi: 10.1002/adsc.202301137
23. Berry, DJ, and Steed, JW. Pharmaceutical cocrystals, salts and multicomponent systems; intermolecular interactions and property based design. Adv Drug Deliv Rev. (2017) 117:3–24. doi: 10.1016/j.addr.2017.03.003
24. Paccione, N, Guarnizo-Herrero, V, Ramalingam, M, Larrarte, E, and Pedraz, JL. Application of 3D printing on the design and development of pharmaceutical oral dosage forms. J Control Release. (2024) 373:463–80. doi: 10.1016/j.jconrel.2024.07.035
25. Babanezhad, M, Behroyan, I, Nakhjiri, AT, Marjani, A, and Shirazian, S. Computational modeling of transport in porous media using an adaptive network-based fuzzy inference system. ACS Omega. (2020) 5:30826–35. doi: 10.1021/acsomega.0c04497
26. Pishnamazi, M, Nakhjiri, AT, Taleghani, AS, Ghadiri, M, Marjani, A, and Shirazian, S. Computational modeling of drug separation from aqueous solutions using octanol organic solution in membranes. Sci Rep. (2020) 10:19133. doi: 10.1038/s41598-020-76189-w
27. Harrison, SA, Allen, AM, Dubourg, J, Noureddin, M, and Alkhouri, N. Challenges and opportunities in NASH drug development. Nat Med. (2023) 29:562–73. doi: 10.1038/s41591-023-02242-6
28. Askr, H, Elgeldawi, E, Aboul Ella, H, Elshaier, YAMM, Gomaa, MM, and Hassanien, AE. Deep learning in drug discovery: an integrative review and future challenges. Artif Intell Rev. (2023) 56:5975–6037. doi: 10.1007/s10462-022-10306-1
29. Babanezhad, M, Behroyan, I, Nakhjiri, AT, Rezakazemi, M, Marjani, A, and Shirazian, S. Thermal prediction of turbulent forced convection of nanofluid using computational fluid dynamics coupled genetic algorithm with fuzzy interface system. Sci Rep. (2021) 11:1308. doi: 10.1038/s41598-020-80207-2
30. Zhuang, W, Hachem, K, Bokov, D, Javed Ansari, M, and Taghvaie Nakhjiri, A. Ionic liquids in pharmaceutical industry: a systematic review on applications and future perspectives. J Mol Liq. (2022) 349:118145. doi: 10.1016/j.molliq.2021.118145
31. An, F, Sayed, BT, Parra, RMR, Hamad, MH, Sivaraman, R, Zanjani Foumani, Z, et al. Machine learning model for prediction of drug solubility in supercritical solvent: modeling and experimental validation. J Mol Liq. (2022) 363:119901. doi: 10.1016/j.molliq.2022.119901
32. Ge, K, and Ji, Y. Novel computational approach by combining machine learning with molecular thermodynamics for predicting drug solubility in solvents. Ind Eng Chem Res. (2021) 60:9259–68. doi: 10.1021/acs.iecr.1c00998
33. Elbadawi, M, Li, H, Sun, S, Alkahtani, ME, Basit, AW, and Gaisford, S. Artificial intelligence generates novel 3D printing formulations. Appl Mater Today. (2024) 36:102061. doi: 10.1016/j.apmt.2024.102061
34. Babanezhad, M, Behroyan, I, Nakhjiri, AT, Marjani, A, Rezakazemi, M, Heydarinasab, A, et al. Investigation on performance of particle swarm optimization (PSO) algorithm based fuzzy inference system (PSOFIS) in a combination of CFD modeling for prediction of fluid flow. Sci Rep. (2021) 11:1505. doi: 10.1038/s41598-021-81111-z
35. Ali, KA, Mohin, SK, Mondal, P, Goswami, S, Ghosh, S, and Choudhuri, S. Influence of artificial intelligence in modern pharmaceutical formulation and drug development. Future J Pharm Sci. (2024) 10:53. doi: 10.1186/s43094-024-00625-1
36. Gormley, AJ . Machine learning in drug delivery. J Control Release. (2024) 373:23–30. doi: 10.1016/j.jconrel.2024.06.045
37. Haval, AM, Shwetabh, K, and Dash, SS. Machine learning-based enhanced drug delivery system and its applications–a systematic review. J Angiother. (2023) 642:123098. doi: 10.1016/j.ijpharm.2023.123098
38. Cao, Y, Taghvaie Nakhjiri, A, and Ghadiri, M. Membrane desalination for water treatment: recent developments, techno-economic evaluation and innovative approaches toward water sustainability. Eur Phys J Plus. (2022) 137:763. doi: 10.1140/epjp/s13360-022-02999-8
39. Hosny, A, Parmar, C, Quackenbush, J, Schwartz, LH, and Aerts, HJWL. Artificial intelligence in radiology. Nat Rev Cancer. (2018) 18:500–10. doi: 10.1038/s41568-018-0016-5
40. Wu, N, Phang, J, Park, J, Shen, Y, Huang, Z, Zorin, M, et al. Deep neural networks improve radiologists’ performance in breast cancer screening. IEEE Trans Med Imaging. (2019) 39:1184–94. doi: 10.1109/TMI.2019.2945514
41. Stokes, JM, Yang, K, Swanson, K, Jin, W, Cubillos-Ruiz, A, Donghia, NM, et al. A deep learning approach to antibiotic discovery. Cell. (2020) 180:688–702.e13. e13. doi: 10.1016/j.cell.2020.01.021
42. Wang, J, Tian, K, Li, D, Chen, M, Feng, X, Zhang, Y, et al. Machine learning in gas separation membrane developing: ready for prime time. Sep Purif Technol. (2023) 313:123493. doi: 10.1016/j.seppur.2023.123493
43. Jin, H, Andalib, V, Yasin, G, Bokov, DO, Kamal, M, Alashwal, M, et al. Computational simulation using machine learning models in prediction of CO2 absorption in environmental applications. J Mol Liq. (2022) 358:119159. doi: 10.1016/j.molliq.2022.119159
44. Rahimi, M, Moosavi, SM, Smit, B, and Hatton, TA. Toward smart carbon capture with machine learning. Cell Rep Phys Sci. (2021) 2:100396. doi: 10.1016/j.xcrp.2021.100396
45. Marjani, A, Nakhjiri, AT, Pishnamazi, M, and Shirazian, S. Evaluation of potassium glycinate, potassium lysinate, potassium sarcosinate and potassium threonate solutions in CO2 capture using membranes. Arab J Chem. (2021) 14:102979. doi: 10.1016/j.arabjc.2020.102979
46. Coley, CW, Jin, W, Rogers, L, Jamison, TF, Jaakkola, TS, Green, WH, et al. A graph-convolutional neural network model for the prediction of chemical reactivity. Chem Sci. (2019) 10:370–7. doi: 10.1039/C8SC04228D
47. Senior, AW, Evans, R, Jumper, J, Kirkpatrick, J, Sifre, L, Green, T, et al. Improved protein structure prediction using potentials from deep learning. Nature. (2020) 577:706–10. doi: 10.1038/s41586-019-1923-7
48. Zhou, Z, Li, X, and Zare, RN. Optimizing chemical reactions with deep reinforcement learning. ACS Centr Sci. (2017) 3:1337–44. doi: 10.1021/acscentsci.7b00492
49. Marjani, A, Nakhjiri, AT, Taleghani, AS, and Shirazian, S. Mass transfer modeling absorption using nanofluids in porous polymeric membranes. J Mol Liq. (2020) 318:114115. doi: 10.1016/j.molliq.2020.114115
50. Cao, Y, Taghvaie Nakhjiri, A, and Ghadiri, M. Different applications of machine learning approaches in materials science and engineering: comprehensive review. Eng Appl Artif Intell. (2024) 135:108783. doi: 10.1016/j.engappai.2024.108783
51. Guerin, G, Molev, G, Pichugin, D, Rupar, PA, Qi, F, Cruz, M, et al. Effect of concentration on the dissolution of one-dimensional polymer crystals: a TEM and NMR study. Macromolecules. (2018) 52:208–16. doi: 10.1021/acs.macromol.8b02126
52. Zhu, L, Honrao, S, Rijal, B, Hennig, RG, and Manuel, MV. Phase equilibria and diffusion coefficients in the Fe-Zn binary system. Mater Des. (2020) 188:108437. doi: 10.1016/j.matdes.2019.108437
53. del Pilar Sánchez-Camargo, A, Bueno, M, Parada-Alfonso, F, Cifuentes, A, and Ibáñez, E. Hansen solubility parameters for selection of green extraction solvents. TrAC Trends Anal Chem. (2019) 118:227–37. doi: 10.1016/j.trac.2019.05.046
54. Li, M, Zhang, J, Zou, Y, Wang, F, Chen, B, Guan, L, et al. Models for the solubility calculation of a CO2/polymer system: a review. Materials Today Commun. (2020) 25:101277. doi: 10.1016/j.mtcomm.2020.101277
55. Taghvaie Nakhjiri, A, Sanaeepur, H, Ebadi Amooghin, A, and Shirazi, MMA. Recovery of precious metals from industrial wastewater towards resource recovery and environmental sustainability: a critical review. Desalination. (2022) 527:115510. doi: 10.1016/j.desal.2021.115510
56. Liu, X, Zhao, L, Wu, B, and Chen, F. Improving solubility of poorly water-soluble drugs by protein-based strategy: a review. Int J Pharm. (2023) 634:122704. doi: 10.1016/j.ijpharm.2023.122704
57. Kumari, L, Choudhari, Y, Patel, P, Gupta, GD, Singh, D, Rosenholm, JM, et al. Advancement in solubilization approaches: a step towards bioavailability enhancement of poorly soluble drugs. Life. (2023) 13:1099. doi: 10.3390/life13051099
58. MS, A, Rajesh, M, and Subramanian, L. Solubility enhancement techniques: a comprehensive review. World J Biol Pharm Health Sci. (2023) 13:141–9. doi: 10.30574/wjbphs.2023.13.3.0125
59. Li, S, Gu, H, Huang, A, Zou, Y, Yang, S, and Fu, L. Thermodynamic analysis and experimental verification of the direct reduction of iron ores with hydrogen at elevated temperature. J Mater Sci. (2022) 57:20419–34. doi: 10.1007/s10853-022-07855-9
60. Khatsee, S, Daranarong, D, Punyodom, W, and Worajittiphon, P. Electrospinning polymer blend of PLA and PBAT: Electrospinnability–solubility map and effect of polymer solution parameters toward application as antibiotic-carrier mats. J Appl Polym Sci. (2018) 135:46486. doi: 10.1002/app.46486
61. Karplus, M, Levitt, M, and Warshel, A. The nobel prize in chemistry 2013. Nobel Media AB. (2014)
62. Tsamopoulos, AJ, Katsarou, AF, Tsalikis, DG, and Mavrantzas, VG. Shear rheology of unentangled and marginally entangled ring polymer melts from large-scale nonequilibrium molecular dynamics simulations. Polymers. (2019) 11:1194. doi: 10.3390/polym11071194
63. Zhou, P, Hou, J, Yan, Y, Wang, J, and Chen, W. Effect of aggregation and adsorption behavior on the flow resistance of surfactant fluid on smooth and rough surfaces: a many-body dissipative particle dynamics study. Langmuir. (2019) 35:8110–20. doi: 10.1021/acs.langmuir.8b04278
64. Nborhani, T, Nabavi, SA, Hanak, DP, and Manovic, V. Thermodynamic models applied to CO2 absorption modelling. Rev Chem Eng. (2021) 37:931–57. doi: 10.1515/revce-2019-0058
65. Nguyen, M-L, Nakhjiri, AT, Kamal, M, Mohamed, A, Algarni, M, Yu, ST, et al. State-of-the-art review on the application of membrane bioreactors for molecular micro-contaminant removal from aquatic environment. Membranes. (2022) 12:429. doi: 10.3390/membranes12040429
66. Hao, Y, Smith, MM, and Carroll, SA. Multiscale modeling of CO2-induced carbonate dissolution: from core to meter scale. Int J Greenho Gas Control. (2019) 88:272–89. doi: 10.1016/j.ijggc.2019.06.007
67. Topol, EJ . High-performance medicine: the convergence of human and artificial intelligence. Nat Med. (2019) 25:44–56. doi: 10.1038/s41591-018-0300-7
68. Djuriš, J, Kurćubić, I, and Ibrić, S. Review of machine learning algorithms application in pharmaceutical technology. Arch Pharm. (2021) 71:302–17. doi: 10.5937/arhfarm71-32499
69. Djuris, J, Ibric, S, and Đurić, Z. Quality by design in the pharmaceutical development In: Computer-aided applications in pharmaceutical technology. Elsevier (2024). 1–21.
70. Comoglu, T, and Ozyilmaz, ED. Pharmaceutical product development: a “quality by design”(QbD) approach In: Dosage forms, formulation developments and regulations. Elsevier (2024). 285–310.
71. Amasya, G, Aksu, B, Badilli, U, Onay-Besikci, A, and Tarimci, N. QbD guided early pharmaceutical development study: production of lipid nanoparticles by high pressure homogenization for skin cancer treatment. Int J Pharm. (2019) 563:110–21. doi: 10.1016/j.ijpharm.2019.03.056
72. Koletti, AE, Tsarouchi, E, Kapourani, A, Kontogiannopoulos, KN, Assimopoulou, AN, and Barmpalexis, P. Gelatin nanoparticles for NSAID systemic administration: quality by design and artificial neural networks implementation. Int J Pharm. (2020) 578:119118. doi: 10.1016/j.ijpharm.2020.119118
73. Khuat, TT, Bassett, R, Otte, E, Grevis-James, A, and Gabrys, B. Applications of machine learning in antibody discovery, process development, manufacturing and formulation: current trends, challenges, and opportunities. Comput Chem Eng. (2024) 182:108585. doi: 10.1016/j.compchemeng.2024.108585
74. Peeters, M, Barrera Jiménez, AA, Matsunami, K, Ghijs, M, dos Santos Schultz, E, Roudgar, M, et al. Analysis of the effect of formulation properties and process parameters on granule formation in twin-screw wet granulation. Int J Pharm. (2024) 650:123671. doi: 10.1016/j.ijpharm.2023.123671
75. Makarov, DM, Kalikin, NN, and Budkov, YA. Prediction of drug-like compounds solubility in supercritical carbon dioxide: a comparative study between classical density functional theory and machine learning approaches. Ind Eng Chem Res. (2024) 63:1589–603. doi: 10.1021/acs.iecr.3c03208
76. Nguyen, CN, Tran, BN, do, TT, Nguyen, H, and Nguyen, TN. D-optimal optimization and data-analysis comparison between a DoE software and artificial neural networks of a chitosan coating process onto PLGA nanoparticles for lung and cervical cancer treatment. J Pharm Innov. (2019) 14:206–20. doi: 10.1007/s12247-018-9345-x
77. Simões, MF, Silva, G, Pinto, AC, Fonseca, M, Silva, NE, Pinto, RMA, et al. Artificial neural networks applied to quality-by-design: from formulation development to clinical outcome. Eur J Pharm Biopharm. (2020) 152:282–95. doi: 10.1016/j.ejpb.2020.05.012
78. Lou, H, Lian, B, and Hageman, MJ. Applications of machine learning in solid oral dosage form development. J Pharm Sci. (2021) 110:3150–65. doi: 10.1016/j.xphs.2021.04.013
79. Karalis, VD . Chapter 6 - artificial intelligence in drug discovery and clinical practice In: N Pippa, C Demetzos, and M Chountoulesi, editors. From current to future trends in pharmaceutical technology. Springer (2024). 215–55.
80. Saha, R, Chauhan, A, and Rastogi Verma, S. Machine learning: an advancement in biochemical engineering. Biotechnol Lett. (2024) 46:497–519. doi: 10.1007/s10529-024-03499-8
81. Wang, Z, Wan, Y, Li, H, and Wang, Y. Computational investigations on anti-cancer drug solubility in supercritical solvent for efficient cancer therapy. J Mol Liq. (2024) 404:124864. doi: 10.1016/j.molliq.2024.124864
82. Belič, A, Škrjanc, I, Božič, DZ, Karba, R, and Vrečer, F. Minimisation of the capping tendency by tableting process optimisation with the application of artificial neural networks and fuzzy models. Eur J Pharm Biopharm. (2009) 73:172–8. doi: 10.1016/j.ejpb.2009.05.005
83. Lee, C, Kwon, O, Kim, M, and Kwon, D. Early identification of emerging technologies: a machine learning approach using multiple patent indicators. Technol Forecast Soc Chang. (2018) 127:291–303. doi: 10.1016/j.techfore.2017.10.002
84. Cao, Y, Nakhjiri, AT, and Ghadiri, M. Numerical investigation of ibuprofen removal from pharmaceutical wastewater using adsorption process. Sci Rep. (2021) 11:24478. doi: 10.1038/s41598-021-04185-9
85. Yang, Y, Ye, Z, Su, Y, Zhao, Q, Li, X, and Ouyang, D. Deep learning for in vitro prediction of pharmaceutical formulations. Acta Pharm Sin B. (2019) 9:177–85. doi: 10.1016/j.apsb.2018.09.010
86. Babanezhad, M, Behroyan, I, Nakhjiri, AT, Marjani, A, and Shirazian, S. Simulation of liquid flow with a combination artificial intelligence flow field and Adams–Bashforth method. Sci Rep. (2020) 10:16719. doi: 10.1038/s41598-020-72602-6
87. Ekins, S . The next era: deep learning in pharmaceutical research. Pharm Res. (2016) 33:2594–603. doi: 10.1007/s11095-016-2029-7
88. Vora, LK, Gholap, AD, Jetha, K, Thakur, RRS, Solanki, HK, and Chavda, VP. Artificial intelligence in pharmaceutical technology and drug delivery design. Pharmaceutics. (2023) 15:1916. doi: 10.3390/pharmaceutics15071916
89. Zhao, Q, Ye, Z, Su, Y, and Ouyang, D. Predicting complexation performance between cyclodextrins and guest molecules by integrated machine learning and molecular modeling techniques. Acta Pharm Sin B. (2019) 9:1241–52. doi: 10.1016/j.apsb.2019.04.004
90. Gao, H, Ye, Z, Dong, J, Gao, H, Yu, H, Li, H, et al. Predicting drug/phospholipid complexation by the lightGBM method. Chem Phys Lett. (2020) 747:137354. doi: 10.1016/j.cplett.2020.137354
91. Öztürk, AA, Gündüz, AB, and Ozisik, O. Supervised machine learning algorithms for evaluation of solid lipid nanoparticles and particle size. Comb Chem High Throughput Screen. (2018) 21:693–9. doi: 10.2174/1386207322666181218160704
92. Chen, H, Hu, Y, Kang, Y, Wang, X, Liu, F, and Liu, Y. Advantages of supercritical CO2 compound fracturing in shale on fracture geometry, complexity and width. J Nat Gas Sci Eng. (2021) 93:104033. doi: 10.1016/j.jngse.2021.104033
93. Sodeifian, G, Alwi, RS, Razmimanesh, F, and Roshanghias, A. Solubility of pazopanib hydrochloride (PZH, anticancer drug) in supercritical CO2: experimental and thermodynamic modeling. J Supercrit Fluids. (2022) 190:105759. doi: 10.1016/j.supflu.2022.105759
94. Sodeifian, G, Surya Alwi, R, Razmimanesh, F, and Abadian, M. Solubility of Dasatinib monohydrate (anticancer drug) in supercritical CO2: experimental and thermodynamic modeling. J Mol Liq. (2022) 346:117899. doi: 10.1016/j.molliq.2021.117899
95. Smith, RD, and Udseth, HR. Mass spectrometry with direct supercritical fluid injection. Anal Chem. (1983) 55:2266–72. doi: 10.1021/ac00264a016
96. Brunner, G . Applications of supercritical fluids. Annu Rev Chem Biomol Eng. (2010) 1:321–42. doi: 10.1146/annurev-chembioeng-073009-101311
97. Reverchon, E, and Adami, R. Nanomaterials and supercritical fluids. J Supercrit Fluids. (2006) 37:1–22. doi: 10.1016/j.supflu.2005.08.003
98. Cansell, F, and Aymonier, C. Design of functional nanostructured materials using supercritical fluids. J Supercrit Fluids. (2009) 47:508–16. doi: 10.1016/j.supflu.2008.10.002
99. Ainurofiq, A, Putro, DS, Ramadhani, DA, Putra, GM, and do Espirito Santo, LDC. A review on solubility enhancement methods for poorly water-soluble drugs. J Rep Pharm Sci. (2021) 10:137–47. doi: 10.4103/jrptps.JRPTPS_134_19
100. Da Silva, FLO, Marques, MBDF, Kato, KC, and Carneiro, G. Nanonization techniques to overcome poor water-solubility with drugs. Expert Opin Drug Discov. (2020) 15:853–64. doi: 10.1080/17460441.2020.1750591
101. Khan, KU, Minhas, MU, Badshah, SF, Suhail, M, Ahmad, A, and Ijaz, S. Overview of nanoparticulate strategies for solubility enhancement of poorly soluble drugs. Life Sci. (2022) 291:120301. doi: 10.1016/j.lfs.2022.120301
102. Alshahrani, SM, Alshetaili, AS, Alfadhel, MM, Belal, A, Abourehab, MAS, al Saqr, A, et al. Optimization of tamoxifen solubility in carbon dioxide supercritical fluid and investigating other molecular targets using advanced artificial intelligence models. Sci Rep. (2023) 13:1313. doi: 10.1038/s41598-022-25562-y
103. Jagtap, S, et al. Solubility enhancement technique: a review. J Pharm Sci Res. (2018) 10:2205–11.
104. Savjani, KT, Gajjar, AK, and Savjani, JK. Drug solubility: importance and enhancement techniques. Int Schol Res Not. (2012) 2012:1–10. doi: 10.5402/2012/195727
105. Sodeifian, G, and Usefi, MMB. Solubility, extraction, and nanoparticles production in supercritical carbon dioxide: a Mini-review. ChemBioEng Rev. (2023) 10:133–66. doi: 10.1002/cben.202200020
106. Aldoseri, A, Al-Khalifa, KN, and Hamouda, AM. Re-thinking data strategy and integration for artificial intelligence: concepts, opportunities, and challenges. Appl Sci. (2023) 13:7082. doi: 10.3390/app13127082
107. Fattahi, A, Karimi-Sabet, J, Keshavarz, A, Golzary, A, Rafiee-Tehrani, M, and Dorkoosh, FA. Preparation and characterization of simvastatin nanoparticles using rapid expansion of supercritical solution (RESS) with trifluoromethane. J Supercrit Fluids. (2016) 107:469–78. doi: 10.1016/j.supflu.2015.05.013
108. Zhang, W, Sun, Y, Li, Y, Shen, R, Ni, H, and Hu, D. Preparation and influencing factors of sirolimus liposome by supercritical fluid. Artif Cells Blood Subst Biotechnol. (2012) 40:62–5. doi: 10.3109/10731199.2011.585618
109. Keshavarz, A, Karimi-Sabet, J, Fattahi, A, Golzary, AA, Rafiee-Tehrani, M, and Dorkoosh, FA. Preparation and characterization of raloxifene nanoparticles using rapid expansion of supercritical solution (RESS). J Supercrit Fluids. (2012) 63:169–79. doi: 10.1016/j.supflu.2011.12.005
110. Hezave, AZ, and Esmaeilzadeh, F. Fabrication of micron level particles of amoxicillin by rapid expansion of supercritical solution. J Dispers Sci Technol. (2012) 33:1419–28. doi: 10.1080/01932691.2011.620883
111. Türk, M, and Bolten, D. Formation of submicron poorly water-soluble drugs by rapid expansion of supercritical solution (RESS): results for naproxen. J Supercrit Fluids. (2010) 55:778–85. doi: 10.1016/j.supflu.2010.09.023
112. Herrmann, M, Förter-Barth, U, Kröber, H, Kempa, PB, Juez-Lorenzo, MM, and Doyle, S. Co-crystallization and characterization of pharmaceutical ingredients. Part Part Syst Charact. (2009) 26:151–6. doi: 10.1002/ppsc.200800046
113. Vemavarapu, C, Mollan, MJ, and Needham, TE. Crystal doping aided by rapid expansion of supercritical solutions. AAPS PharmSciTech. (2002) 3:17–31. doi: 10.1208/pt030429
114. Atila, C, Yıldız, N, and Çalımlı, A. Particle size design of digitoxin in supercritical fluids. J Supercrit Fluids. (2010) 51:404–11. doi: 10.1016/j.supflu.2009.10.006
115. Chiou, AH-J, Cheng, H-C, and Wang, D-P. Micronization and microencapsulation of felodipine by supercritical carbon dioxide. J Microencapsul. (2006) 23:265–76. doi: 10.1080/02652040500435071
Keywords: supercritical CO2, solubility enhancement, machine learning, therapeutic efficiency, artificial intelligence
Citation: Alamoudi JA (2024) Recent advancements toward the incremsent of drug solubility using environmentally-friendly supercritical CO2: a machine learning perspective. Front. Med. 11:1467289. doi: 10.3389/fmed.2024.1467289
Received: 19 July 2024; Accepted: 20 August 2024;
Published: 02 September 2024.
Edited by:
Syed Faheem Askari Rizvi, Lanzhou University, ChinaReviewed by:
Syeda Samar Mustafa, Government College University Lahore, PakistanCopyright © 2024 Alamoudi. This is an open-access article distributed under the terms of the Creative Commons Attribution License (CC BY). The use, distribution or reproduction in other forums is permitted, provided the original author(s) and the copyright owner(s) are credited and that the original publication in this journal is cited, in accordance with accepted academic practice. No use, distribution or reproduction is permitted which does not comply with these terms.
*Correspondence: Jawaher Abdullah Alamoudi, amFhbGFtb3VkaUBwbnUuZWR1LnNh
Disclaimer: All claims expressed in this article are solely those of the authors and do not necessarily represent those of their affiliated organizations, or those of the publisher, the editors and the reviewers. Any product that may be evaluated in this article or claim that may be made by its manufacturer is not guaranteed or endorsed by the publisher.
Research integrity at Frontiers
Learn more about the work of our research integrity team to safeguard the quality of each article we publish.