- 1Affiliated Hospital of Nanjing University of Chinese Medicine, Nanjing, China
- 2Department of Endocrinology, Nanjing University of Chinese Medicine, Nanjing, China
- 3Department of Endocrinology, Jiangsu Province Hospital of Chinese Medicine, Affiliated Hospital of Nanjing University of Chinese Medicine, Nanjing, China
- 4Department of Endocrinology, Nanjing Jiangning Hospital of Chinese Medicine, Affiliated Jiangning Hospital of Chinese Medicine, China Pharmaceutical University, Nanjing, China
Objective: Previous observational studies have suggested associations between various inflammatory cytokines with type 2 diabetes mellitus and diabetic nephropathy. However, the causal association remains uncertain.
Method: Summary statistics for type 2 diabetes mellitus and diabetic nephropathy were obtained from a publicly available genome-wide association study. Data on inflammatory cytokines were sourced from a genome-wide association study on protein quantitative trait loci. The inverse variance-weighted method was applied as the primary method for causal inference. MR-Egger, weighted mode, and weighted median method were employed as supplementary analyses. Sensitivity analyses were performed to detect heterogeneity and potential horizontal pleiotropy in the study.
Result: Genetic evidence indicated that elevated levels of fibroblast growth factor 19 levels promoted the occurrence of type 2 diabetes mellitus, and increased concentrations of fibroblast growth factor 21 levels, C-C motif chemokine 19 levels, eotaxin levels, and interleukin-10 mitigated the risk of developing type 2 diabetes mellitus, while type 2 diabetes mellitus did not exert a significant influence on said proteins. Elevated levels of tumor necrosis factor ligand superfamily member 14 and TNF-related activation-induced cytokine were associated with an increased risk of diabetic nephropathy, and increased concentrations of interleukin-1-alpha and transforming growth factor-alpha were potentially correlated with a diminished risk of diabetic nephropathy. Sensitivity analyses further ensure the robustness of our findings.
Conclusion: Mendelian randomization analysis highlights a causal association between inflammatory cytokines with type 2 diabetes mellitus and diabetic nephropathy, offering valuable evidence and reference for future research.
1 Introduction
Type 2 diabetes mellitus (T2DM) is a common chronic metabolic disease (1). Persistent hyperglycemia in the blood may cause multiple system complications, which may pose significant risks to the health and life span of patients. Diabetic nephropathy (DN) is a common microvascular complication of T2DM, affecting about 40% of patients with T2DM (2, 3). It is also a major cause of end-stage renal disease (ESRD). Despite advances in treatment, the molecular mechanisms underlying DN remain unclear, and current therapies, including angiotensin-converting enzyme inhibitors (ACEIs), angiotensin II receptor blockers (ARBs), and sodium-glucose cotransporter 2 inhibitors (SGLT-2i), are primarily symptomatic (4–6). The pathogenesis of DN involves multiple factors, including dysregulation of kidney signaling pathways that affect metabolism, hemodynamics, inflammation, and autophagy, ultimately leading to ESRD (7–10).
Immune response and inflammatory modulators are closely related to pancreatic β cell dysfunction and insulin resistance in patients with T2DM. Pro-inflammatory cytokines such as interleukin-1β (IL-1β) can inhibit insulin secretion and pancreatic β cell proliferation by increasing the transcription and secretion of chemokines (11). Inhibition of inflammatory factors in diabetic mice can effectively protect islet cells and delay the progress of hyperglycemia (12). Oxidative stress and inflammation are central to the progression of DN. Increased oxidative stress in DN arises from an imbalance between hyperglycemia-induced reactive oxygen species (ROS) production and the antioxidant defense mechanisms (13, 14). DN-induced hemodynamic or metabolic dysfunction triggers inflammatory processes, leading to the release of chemokines and pro-inflammatory cytokines, such as IL-1β, interleukin-6 (IL-6), interleukin-18 (IL-18), and adhesion molecules like vascular cell adhesion protein-1 (VCAM-1) and intercellular adhesion molecule-1 (ICAM-1) (15, 16). Furthermore, chemokine signaling recruits immune cells, including macrophages and T lymphocytes, which release additional pro-inflammatory mediators, exacerbating renal inflammation (17, 18). ROS not only cause oxidative tissue damage but also promote the aggregation of inflammatory cells, leading to the production of inflammatory cytokines, growth factors, and transcription factors linked to DN pathogenesis (19). The infiltration of inflammatory cells, such as lymphocytes, neutrophils, and macrophages, is a key driver of kidney damage in DN (20). The recruitment and differentiation of these immune cells are regulated by various inflammatory cytokines (6, 21, 22). For example, upon stimulation by ROS, NF-kB translocates to the nucleus, binds to NF-kB binding sites, and activates transcription. This leads to the release of adhesion molecules and pro-inflammatory factors, including monocyte chemoattractant protein-1 (MCP-1), ICAM-1, transforming growth factor-β1 (TGF-β1), IL-1β, IL-6, and tumor necrosis factor-α (TNF-α), which can also regulate ROS levels (23). High glucose (HG) can induce NF-kB receptor activators in podocytes, further promoting DN progression (24). The NOD-like receptor protein (NLRP) 3 inflammasome regulates inflammation by cleaving pro-inflammatory cytokines, such as pro-IL-1β and pro-IL-18, into their active forms (25). Bruton’s tyrosine kinase (BTK) is activated in the kidneys of DN patients, and its knockdown can reduce macrophage-induced inflammation in diabetic mice by inhibiting NLRP3 inflammasome activity (26). High-mobility group box 1 (HMGB1), a nuclear non-histone protein, can enter the nucleus through active secretion or passive release. Once in the cytoplasm, HMGB1 participates in immune responses. When released extracellularly, HMGB1 acts as a potent inflammatory mediator, either alone or as part of a pro-inflammatory cascade, stimulating the immune system (27). HMGB1 is regulated by ROS and the NLRP3 inflammasome, which facilitate its translocation from the nucleus to the cytoplasm. Once in the cytoplasm, HMGB1 binds to Toll-like receptor 4 (TLR4), activates NF-kB, and induces an inflammatory response (28). Overall, numerous studies demonstrate that oxidative stress and inflammation are interdependent processes that coexist within an inflammatory environment. Inflammatory cells release substantial amounts of ROS at inflammation sites, resulting in increased oxidative damage.
Current research indicates that inflammatory cytokines play a role in the treatment of T2DM and DN. In a long-term randomized controlled trial, it was observed that the plasma total FGF-21 level and biological activity level of patients with T2DM decreased (29). A cross-sectional study found that the serum concentration of hGDNF in patients with T2DM was lower than that in the control group, and the level of hGDNF in patients with poor blood sugar control was also significantly reduced (30). Zhang et al. (31) demonstrated that interleukin-17C (IL-17C) contributes to DN and suggested that inhibiting IL-17C could offer a therapeutic approach. Murakoshi et al. (32) found that IL-6 is essential for the proliferation of glomerular mesangial cells and the activation and expansion of B cells. As a pleiotropic cytokine, IL-6 has diverse effects on the body and directly impacts the inflammatory response in DN. Nakamura et al. (33) identified MCP-1 as an early marker of kidney function changes in DN, with its levels reflecting disease progression (34, 35). Additionally, research has shown that DN progression may be influenced by the activation of various signaling pathways mediated by inflammatory cytokines (36, 37) and other biomarkers (38, 39). However, the precise genetic impact of these cytokines on T2DM and DN remains unclear, underscoring the need for further investigation.
Mendelian randomization (MR) analysis is a powerful method for elucidating causal associations between exposures and outcomes, utilizing SNPs as instrumental variables (IVs) (40, 41). Recently, MR has become a valuable tool for assessing these causal relationships (42). In our study, we employed a two-sample bidirectional MR analysis to examine the causal association between inflammatory cytokines with T2DM and DN, providing genetic evidence for this association. The procedural framework of our study is illustrated in Figure 1A.
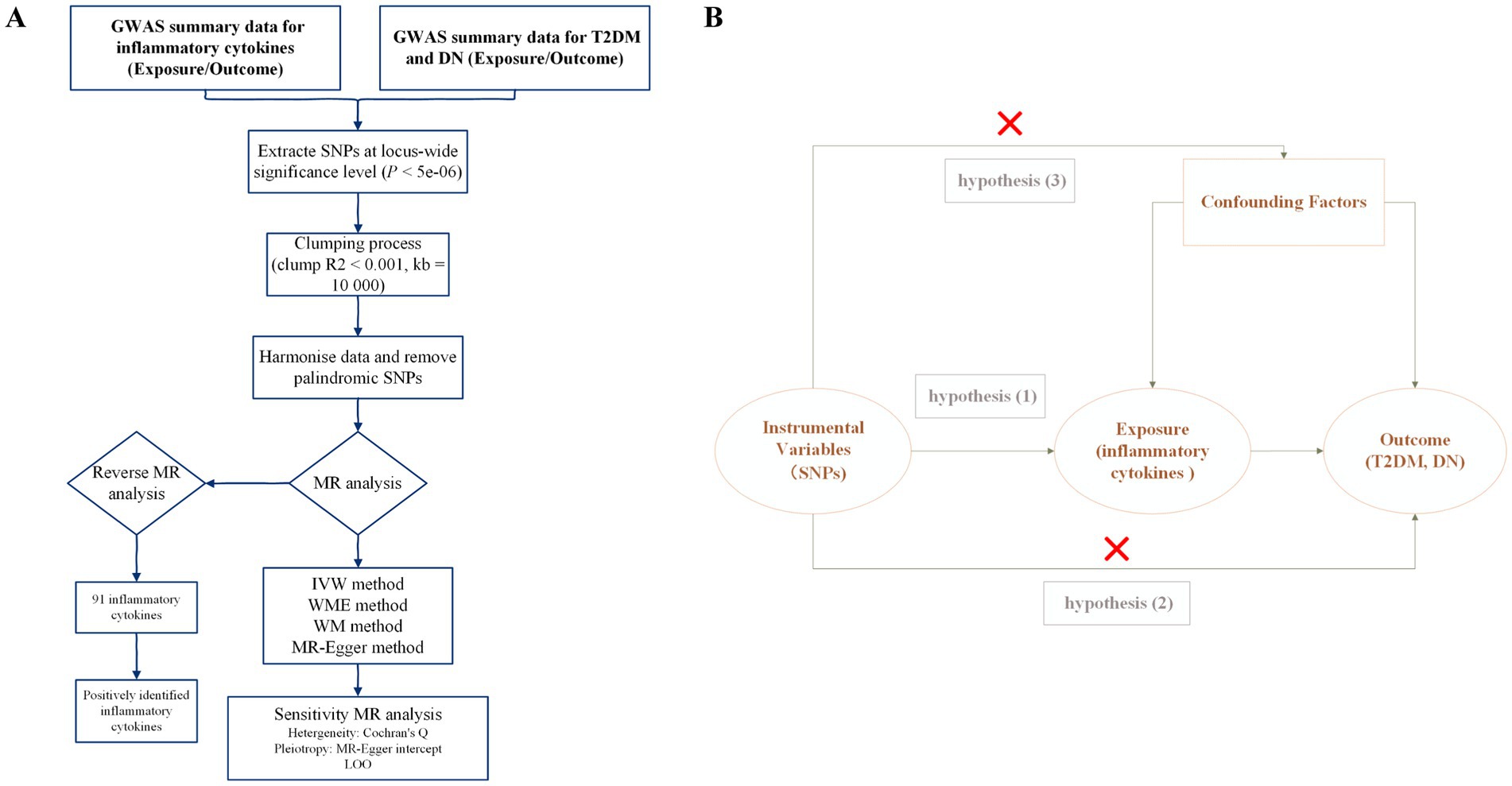
Figure 1. Study overview. (A) The protocol of our study procedure. T2DM, type 2 diabetes mellitus; DN, diabetic nephropathy; SNPs, single nucleotide polymorphisms; GWAS, genome-wide association study; LOO, leave-one-out; MR, Mendelian randomization; WM, weighted mode; WME, weighted median; IVW, inverse variance weighted. (B) The three hub hypothesis of the MR analysis.
2 Material and method
2.1 Exposure and outcome data sources
To estimate SNP effects associated with inflammatory cytokines, we used genome-wide association studies (GWAS) summary statistics (GSCT90274758–GSCT90274848) provided by Zhao et al. (43), which include data on 91 inflammatory cytokines from 14,824 individuals of European ancestry. Summary statistics for T2DM and DN were obtained from a publicly available GWAS analysis, which comprising 38,841 T2DM cases and 451,248 controls of European ancestry (ebi-a-GCST90018926), 1,032 DN cases and 451,248 controls of European ancestry (ebi-a-GCST90018832), 220 DN cases and 132,764 controls of East Asian ancestry (ebi-a-GCST90018612), sourced from the IEU OpenGWAS Project website.1 The dataset for T2DM European ancestry included 490,089 individuals and 24,167,560 SNPs (44). The dataset for DN European ancestry included 452,280 individuals and 24,190,738 SNPs, while the dataset for East Asian ancestry included 132,984 individuals and 12,447,074 SNPs (44).
Since this study relies on publicly available data, no additional ethical approval or consent was required. To minimize the impact of race-related confounding factors, the study population predominantly comprised individuals of European ancestry. Data from individuals of East Asian descent were also included to enhance the robustness and generalizability of the findings.
2.2 Instrumental variables selection
The selection of genetic variants as IVs for inflammatory cytokines followed the three-hub hypothesis (Figure 1B). First, SNPs were screened from the GWAS data, considering only those with a significance threshold of p < 5 × 10−6. A linkage disequilibrium test was then performed on these SNPs to ensure they met the independence criterion. SNP selection was carefully controlled with parameters set to R2 < 0.001 and a maximum distance of 10,000 kb to reduce linkage disequilibrium and identify independent SNPs (45). Second, the PhenoScanner database was used to validate whether the identified SNP loci were associated with other potential confounding factors (46). Finally, to evaluate the susceptibility of the selected SNPs to weak IV bias, F statistics were calculated with a threshold set at F > 10 (using the formula F = β2/SE2, where β represents the effect size and SE the standard error). SNPs with F < 10 were deemed susceptible to weak IV bias and excluded to avoid their influence on the results. Higher F statistics indicate that the genetic markers are likely to cause phenotypic variations, which can lead to divergence in results due to these variations (47, 48). The selection of genetic variants as IVs was associated with the exposure of inflammatory cytokines.
2.3 Statistical analysis
2.3.1 MR analysis
To uncover the causal association between inflammatory cytokines with T2DM and DN, we employed a range of analytical methods, including inverse variance weighted (IVW), weighted median (WME), weighted mode (WM), MR-Egger regression, and forest plot visualization (49, 50). Using multiple analytical approaches aimed to enhance the robustness and reliability of our findings. A significance threshold of p < 0.05 was used to determine causal associations between inflammatory cytokines with T2DM and DN (45, 51).
To address the potential for specific errors and account for multiple testing, we applied the q value to adjust for the false discovery rate (FDR). A q value <0.1 indicates a significant association, while a p < 0.05 but q value ≥0.1 suggests a more tentative association (52).
2.3.2 Sensitivity analysis
The p-value from Cochran’s Q statistics of the IVW method was used to assess the heterogeneity of the IVs. A p-value ≥0.05 indicates the absence of heterogeneity in the causal analysis (53, 54). Additionally, a funnel plot was employed as a visual tool to detect heterogeneity, with a symmetrical distribution of SNPs suggesting homogeneity in the results (55). Depending on the presence or absence of heterogeneity, either a random-effects model or a fixed-effects model was chosen.
Pleiotropy was assessed using MR-Egger regression, with the intercept of the MR-Egger regression in a scatter plot providing insights into potential pleiotropic effects (56, 57). Leave-one-out (LOO) analysis was also conducted to evaluate the stability of the results by identifying SNPs with significant influence when individually removed (58). The risk association between inflammatory cytokines with T2DM and DN was quantified using odds ratios (OR) and 95% confidence intervals (CI), with statistical significance defined as p < 0.05.
2.3.3 Bidirectional MR analysis
A two-sample bidirectional MR analysis was conducted to explore the potential reverse causal association between T2DM, DN (exposure) and inflammatory cytokines (outcome). The procedural steps for this bidirectional MR analysis closely followed those outlined for the previous MR analysis.
2.3.4 Statistical software
All MR analyses were conducted using R (version 4.3.1) with the TwoSampleMR package.
3 Results
3.1 Instrumental variables selection
We selected 21 SNPs related to inflammatory cytokines in T2DM dataset, including 4 SNPs from Fibroblast growth factor 21 levels (FGF21), 4 SNPs from C-C motif chemokine 19 levels (CCL19), 3 SNPs from fibroblast growth factor 19 levels (FGF19), 6 SNPs from eotaxin levels (CCL11), 4 SNPs from Interleukin-10 (IL-10) (Figure 2A).
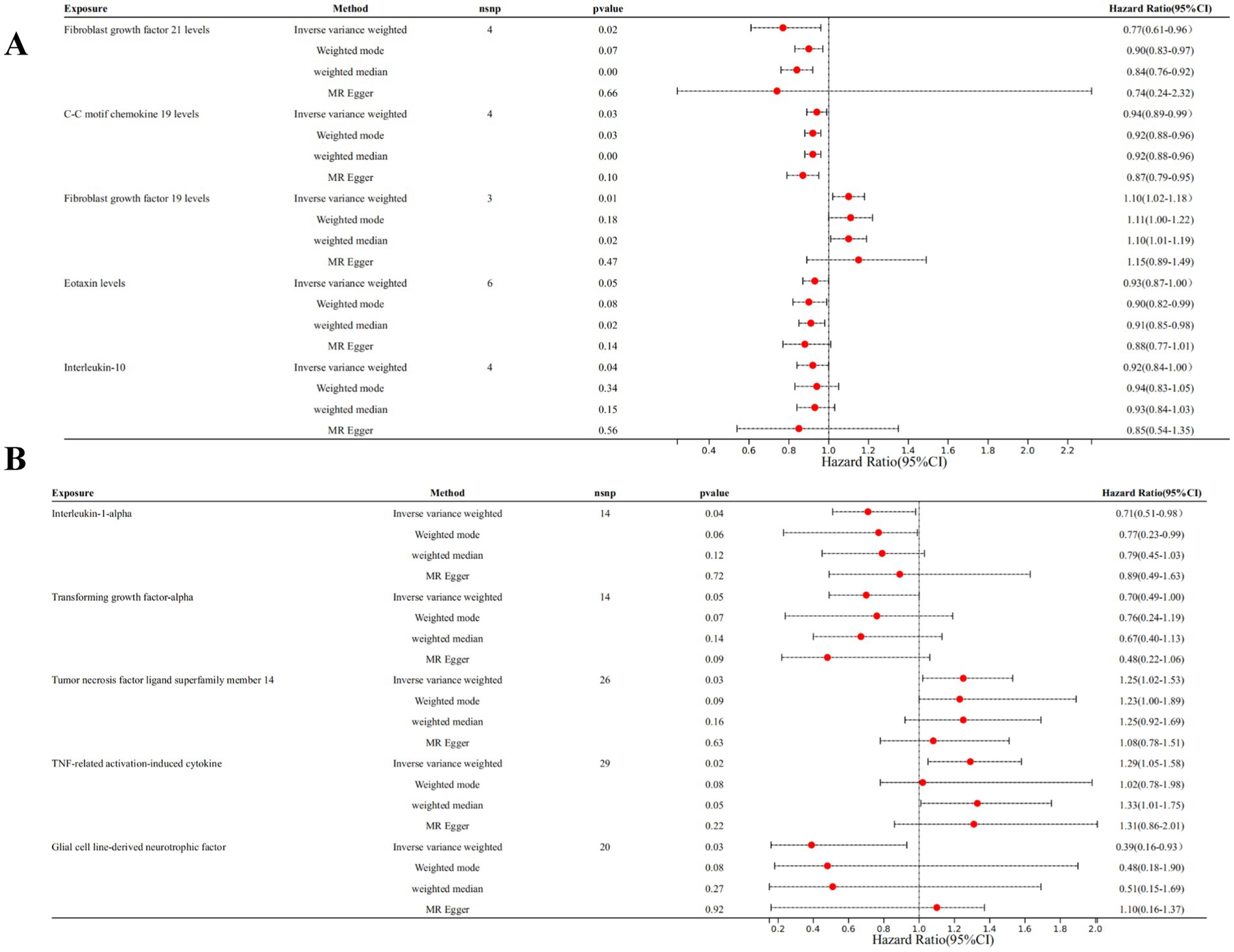
Figure 2. Forest plots of MR analysis. (A) Forest plot of the MR analysis results in T2DM dataset. (B) Forest plot of the MR analysis results in DN dataset. Exposure represents the inflammatory cytokines, nSNP represents the number of SNPs, and pval represents the p-value.
Among individuals of European ancestry in DN dataset, a total of 14 SNPs were extracted from tumor necrosis factor ligand superfamily member 14 (TNFSF14), 14 SNPs from TNF-related activation-induced cytokine (TRANCE), 26 SNPs from interleukin-1 alpha (IL-1α), and 29 SNPs from transforming growth factor alpha (TGF-α). For individuals of East Asian ancestry in DN dataset, 20 SNPs were extracted from glial cell line-derived neurotrophic factor (GDNF) (Figure 2B). Notably, the F statistics for the IVs used in this study exceeded 10, indicating minimal bias from weak IVs and ensuring the robustness of the results (Supplementary Table S1).
The substantial differences between European and East Asian populations stem from factors like genetic allele frequency variations, environmental factors, and lifestyle differences, which may result in distinct research outcomes. For instance, a particular SNP might be common in one population but rare in another. If racial differences are not considered, these frequency disparities could impact effect estimates in MR analyses, leading to biased conclusions. Beyond genetic differences, ethnic groups vary in terms of diet, lifestyle, culture, and socioeconomic status. These environmental distinctions could also influence outcomes through gene-environment interactions. To minimize race-related confounding, we focused primarily on individuals of European descent. However, data from those of East Asian ancestry were also included to improve the robustness and generalizability of our findings.
3.2 MR analysis
Genetic evidence indicated that elevated levels of FGF19 levels (OR = 1.100, 95% CI 1.020–1.180, p = 0.030) promoted the occurrence of T2DM, and increased concentrations of FGF21 levels (OR = 0.770, 95% CI 0.610–0.960, p = 0.020), CCL19 (OR = 0.050, 95% CI 0.930–0.990, p = 0.030) levels, CCL11 levels (OR = 0.930, 95% CI 0.870–1.000, p = 0.050), and IL-10 (OR = 0.920, 95% CI 0.840–1.000, p = 0.040) mitigated the risk of developing T2DM (Figures 3, 4).
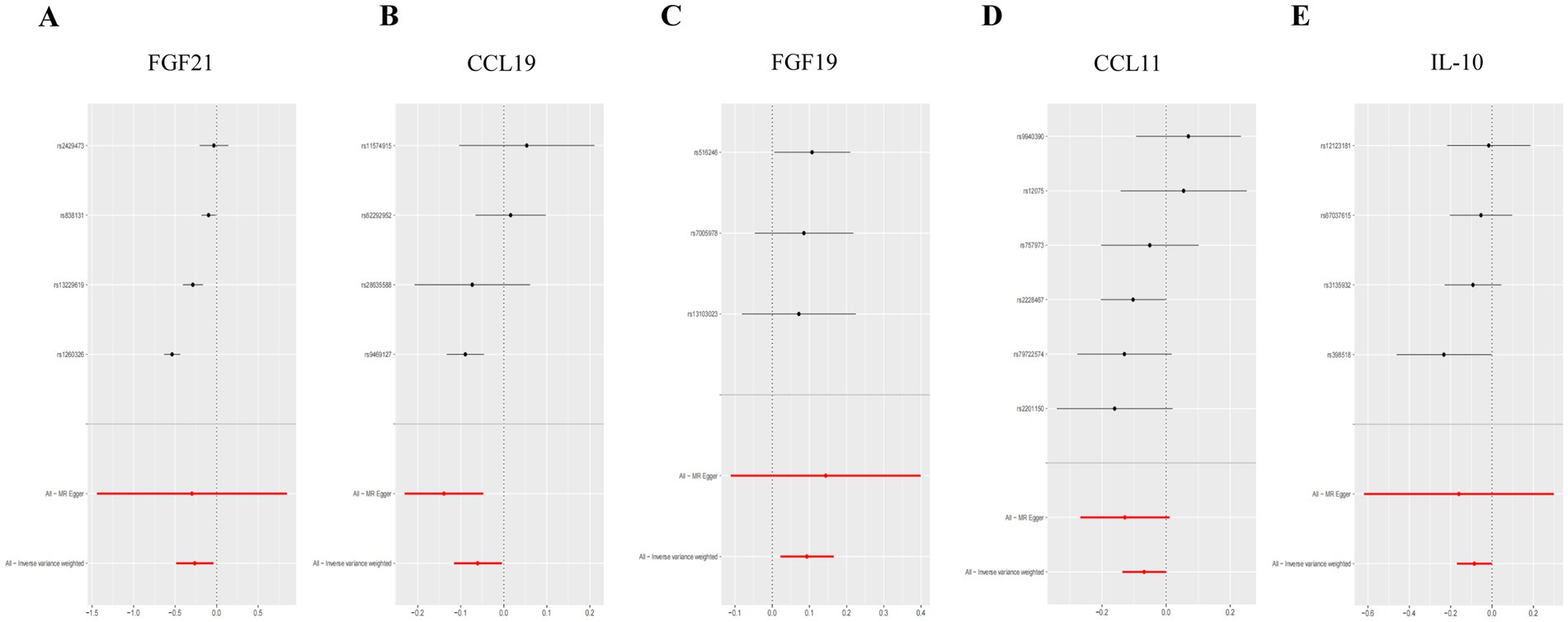
Figure 3. Forest plot of single SNP MR results in T2DM dataset. (A) Gene expression of FGF21. (B) Gene expression of CCL19. (C) Gene expression of FGF19. (D) Gene expression of CCL11. (E) Gene expression of IL-10. In this representation, each black dot symbolizes the T2DM with increased standard deviation (SD) in the inflammatory cytokine, generated by utilizing each SNP as an individual IV. Conversely, the red dot denotes the causal estimation derived from all SNP combinations using various MR methods. The horizontal line segment represents the 95% CI. Specifically, the IVW causal estimate illustrates how the overall estimate (depicted by the red horizontal line) might be disproportionately influenced by the removal of a single variant (indicated by the black horizontal line).
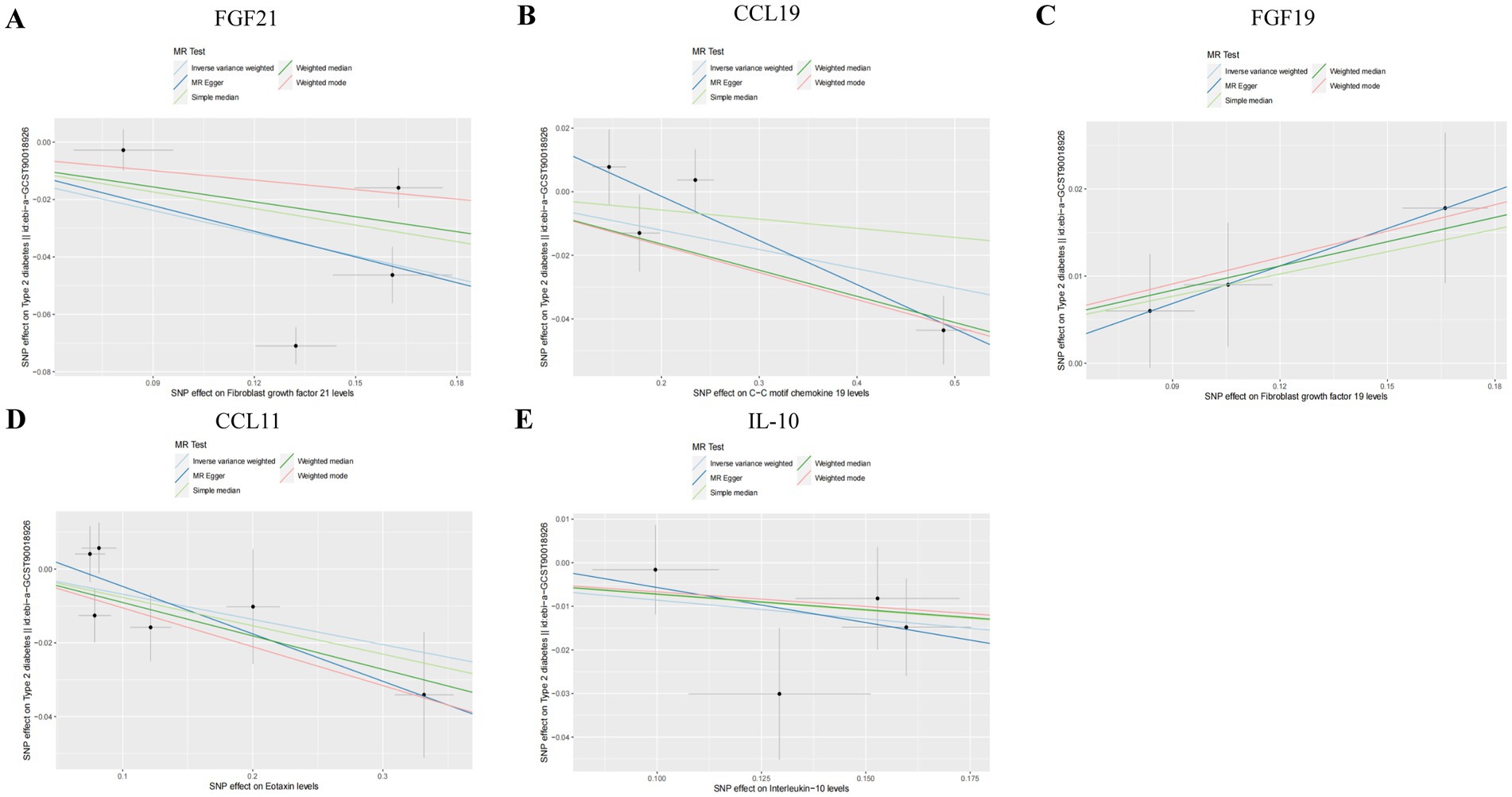
Figure 4. Scatter plots of SNP analysis in T2DM dataset. (A) Gene expression of FGF21. (B) Gene expression of CCL19. (C) Gene expression of FGF19. (D) Gene expression of CCL11. (E) Gene expression of IL-10. The X-axis denotes the impact of the SNP on the inflammatory cytokine, while the Y-axis represents the SNP’s influence on T2DM. Each black dot signifies a single SNP, with the line segment depicting the 95% CI. The slope of the straight line reflects the causal estimation derived from the MR method. In this visualization, the green line corresponds to the IVW method, the blue line represents the MR Egger method, the dark line signifies the WME method, the reseda green line represents the Simple median method and the red line represents the WM method.
Among individuals of European ancestry in DN dataset, the IVW analysis revealed a significant positive causal association between the gene expression of TNFSF14 (OR = 1.249, 95% CI 1.018–1.532, p = 0.033) and TRANCE (OR = 1.287, 95% CI 1.051–1.577, p = 0.015) with DN. In contrast, gene expression of IL-1α (OR = 0.712, 95% CI 0.514–0.984, p = 0.040) and TGF-α (OR = 0.701, 95% CI 0.493–0.998, p = 0.049) showed a significant negative causal association with DN. Among individuals of East Asian ancestry in DN dataset, gene expression of GDNF was significantly negatively associated with DN (OR = 0.391, 95% CI 0.163–0.931, p = 0.034) (Supplementary Figure S1).
Given that all SNPs functioned as effective IVs without horizontal pleiotropy and that the IVW analysis results remained unbiased, the IVW method was considered more reliable for providing effect estimates compared to alternative methodologies (59). This supports the conclusion that inflammatory cytokines have a causal association with T2DM and DN.
However, after FDR correction, only FGF21 (q value = 0.001) and CCL19 (q value = 0.025) levels showed a significant causal association with T2DM, while the gene expression of IL-1α showed a significant negative causal association with DN (q value = 0.050). The associations for the other inflammatory cytokines were considered suggestive of a link to T2DM and DN.
3.3 Sensitivity analysis
In both T2DM and DN datasets, Cochran’s Q test revealed no evidence of heterogeneity among the included IVs (p > 0.05). Additionally, the MR-Egger regression intercept test indicated that pleiotropy did not bias the results (p > 0.05) (Supplementary Table S2). Funnel plots visually confirmed that potential confounders were unlikely to affect the causal inferences (Figure 5; Supplementary Figure S2). The LOO sensitivity analysis showed that removing individual SNPs did not significantly alter the analysis results (Figure 6; Supplementary Figure S3).
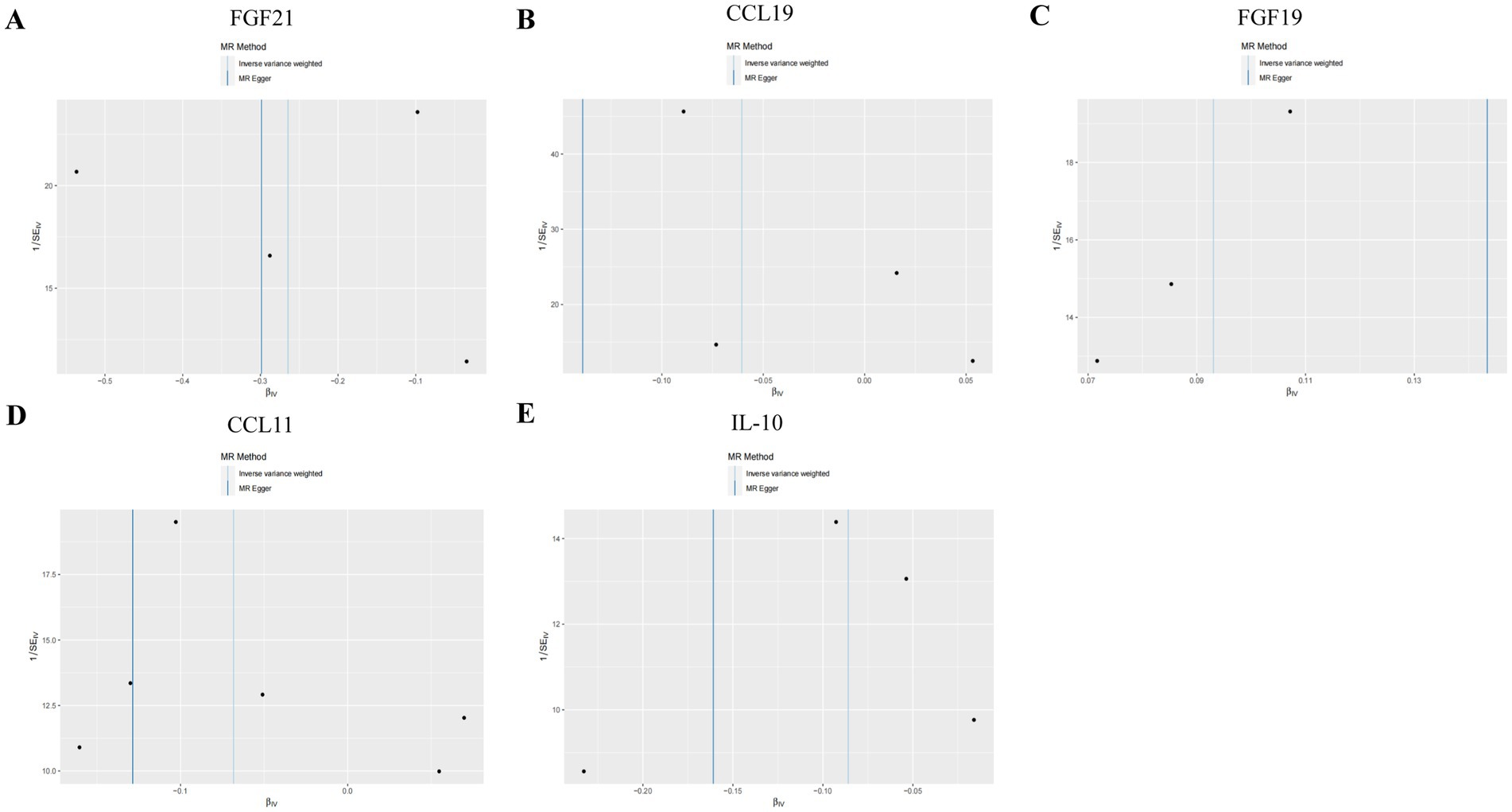
Figure 5. Funnel plots of sensitivity analysis in T2DM dataset. (A) Gene expression of FGF21. (B) Gene expression of CCL19. (C) Gene expression of FGF19. (D) Gene expression of CCL11. (E) Gene expression of IL-10. The light blue represents the IVW method, and the dark blue represents the MR Egger method.
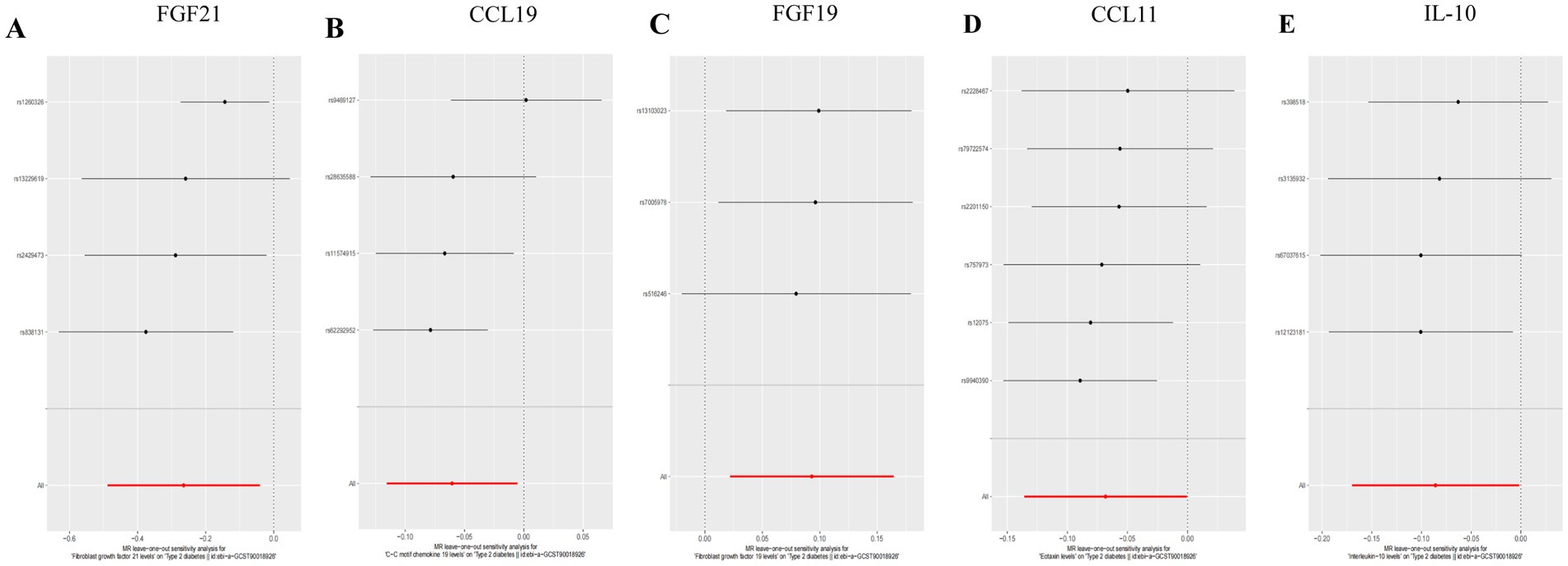
Figure 6. Forest plots of LOO analysis in T2DM dataset. (A) Gene expression of FGF21. (B) Gene expression of CCL19. (C) Gene expression of FGF19. (D) Gene expression of CCL11. (E) Gene expression of IL-10. In this representation, each black dot symbolizes the T2DM with increased standard deviation (SD) in the inflammatory cytokine, generated by utilizing each SNP as an individual IV. Conversely, the red dot denotes the causal estimation derived from all SNP combinations using various MR methods. The horizontal line segment represents the 95% CI. Specifically, the IVW causal estimate illustrates how the overall estimate (depicted by the red horizontal line) might be disproportionately influenced by the removal of a single variant (indicated by the black horizontal line).
3.4 Bidirectional MR analysis
The reverse MR analysis revealed there is no causal association between T2DM, DN and the positive inflammatory cytokines previously identified (Figures 7A,B).
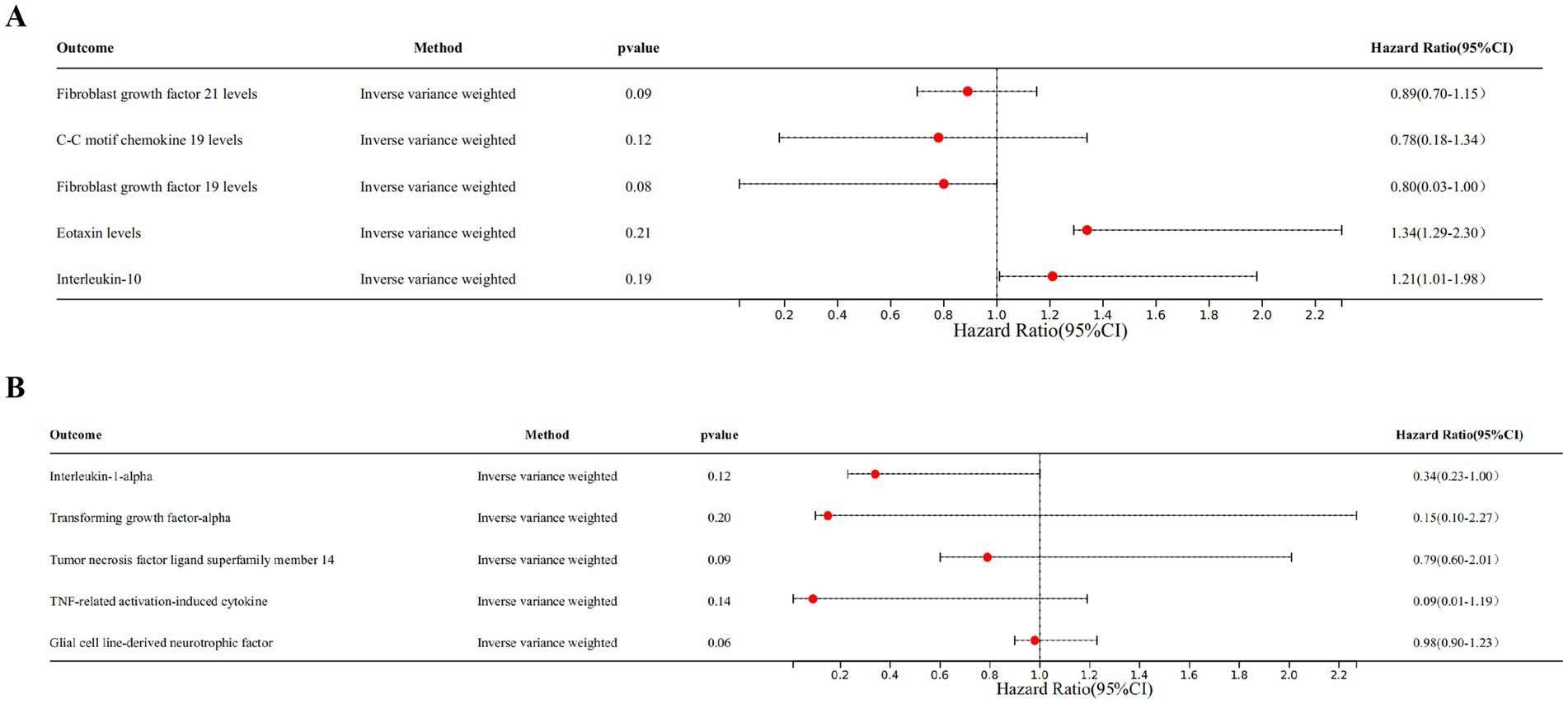
Figure 7. Forest plots of bidirectional MR analysis. (A) Forest plot of the MR analysis results in T2DM dataset. (B) Forest plot of the MR analysis results in DN dataset. Outcome represents the positive identified inflammatory cytokines, nSNP represents the number of SNPs, and pval represents the p-value.
4 Discussion
In this study, we conducted a comprehensive analysis using large-scale GWAS data to investigate the causal association between inflammatory cytokines with T2DM and DN. To address the three hypotheses, we first included only SNPs that met the criteria of p < 5 × 10−6, R2 < 0.001, and a maximum distance of 10,000 kb. Second, the PhenoScanner database was used to exclude potential confounding factors. Finally, only SNPs with F > 10 were included in the analysis. Genetic evidence indicated that elevated levels of FGF19 levels promoted the occurrence of T2DM, and increased concentrations of FGF21 levels, CCL19 levels, CCL11 levels, and IL-10 mitigated the risk of developing T2DM, while T2DM did not exert a significant influence on said proteins. Elevated levels of TNFSF14 and TRANCE were associated with an increased risk of DN, and increased concentrations of IL-1α and TGF-α were potentially correlated with a diminished risk of DN. Subsequent sensitivity analyses confirmed the robustness and consistency of these findings.
FGF19 is primarily secreted by the ileum and functions as an endocrine factor. It plays a crucial role in inhibiting bile acid synthesis and regulating hepatic metabolism (60). Previous studies have suggested that FGF19 may be involved in the regulation of glucose and lipid metabolism, as well as the maintenance of energy homeostasis. Additionally, it may play a protective role in preserving β-cell function (61). Zhang et al. (62) demonstrated in their study that FGF19 levels in subjects with normal glucose tolerance were significantly lower than those in subjects with impaired fasting glucose or impaired glucose tolerance. Moreover, when compared to patients with T2DM, FGF19 levels were markedly reduced in T2DM patients with metabolic syndrome. Human studies have found that serum FGF19 levels are lower in patients with T2DM compared to control groups. However, after weight loss induced by partial gastrectomy or gastroenterostomy, FGF19 levels increase, accompanied by improvements in glucose metabolism indicators (63). Previous studies have indicated that FGF19 acts as a protective factor against T2DM. However, the MR results suggest that FGF19 may serve as a risk predictor for T2DM. This discrepancy could be influenced by various factors, such as environmental conditions and innate genetic variations.
FGF21 is a key regulator of endocrine metabolism. It promotes glucose uptake in skeletal muscle by activating the phosphoinositide 3-kinase/protein kinase C (PI3K/PKC) signaling pathway, which mediates the translocation of glucose transporter 4 (GLUT4) (64). Additionally, FGF21 enhances glucose uptake in adipose tissue through the activation of the extracellular signal-regulated kinase 1/2 (ERK1/2) signaling pathway, thereby helping to maintain glucose and lipid homeostasis (65). FGF21 also induces the nuclear translocation of nuclear factor erythroid 2-related factor 2 (Nrf2), promoting the expression of antioxidant genes, which alleviates oxidative stress and improves insulin resistance (66).
CCL19 is a chemokine that plays a role in immune cell migration and inflammatory responses. Its relationship with the development of T2DM is linked to its involvement in chronic inflammation, a key factor in the pathogenesis of T2DM. In individuals with T2DM, persistent low-grade inflammation contributes to insulin resistance, pancreatic β-cell dysfunction, and metabolic disturbances (67). CCL19 is elevated in patients with T2DM and may promote the infiltration of immune cells into tissues such as adipose tissue, pancreas, and liver. This can exacerbate local inflammation and insulin resistance, contributing to the progression of T2DM. Moreover, CCL19 has been studied in relation to its effects on endothelial dysfunction and atherosclerosis, which are common complications of T2DM (68). The chemokine may accelerate vascular inflammation, increasing the risk of cardiovascular diseases in patients with diabetes. CCL19 is associated with T2DM through its role in promoting inflammation, contributing to insulin resistance, and possibly aggravating cardiovascular complications. CCL11 also known as eotaxin-1, is a chemokine primarily involved in the recruitment of eosinophils and other immune cells to sites of inflammation. Its relationship with T2DM has been increasingly recognized in recent years (69). CCL11 contributes to the development and progression of T2DM by enhancing inflammatory responses and promoting insulin resistance. A meta-analysis revealed that the expression levels of CCL11 are significantly higher in patients with T2DM compared to control groups (70). The upregulation of CCL19 and CCL11 have been confirmed in both T2DM datasets and high-glucose in vitro experiments. However, the MR results indicate that CCL19 and CCL11 act as a protective factor in T2DM, which may be attributed to genetic variations.
IL-10 is a multifunctional inflammatory cytokine that primarily regulates the body’s inflammatory response and immune balance through mechanisms such as humoral immune stimulation (71). It is an inhibitory cytokine that can attenuate the apoptosis of β-cells, suppression of insulin secretion, and peripheral insulin resistance induced by pro-inflammatory cytokines such as IL-6 and TNF-α. By improving pancreatic signaling pathways, IL-10 helps reduce the risk of developing T2DM (72).
TNFSF14 is involved in promoting renal fibrosis (73). IL-1 is pivotal in immune regulation and inflammatory response orchestration. Elevated serum levels and gene expression of IL-1 are consistently observed in patients with DN, which correlates with studies linking IL-1 gene polymorphisms to increased risk of end-stage DN (74, 75). Clinical studies have demonstrated that inhibiting EGFR with TGF-α monoclonal antibodies significantly delays the onset and progression of DN (76). Experimental evidence shows that TNF-α induces the production of inflammatory mediators, including prostaglandins, leukotrienes, and IL-1, in cultured human mesangial cells. These mediators are implicated in the pathogenesis of DN (77). Diabetic mice exhibit decreased GDNF levels, but local administration or overexpression of the GDNF gene in these mice has been shown to reduce apoptosis, improve β-cell quality and proliferation, enhance insulin secretion, and improve local tissue function. Despite these benefits, the efficacy of GDNF in blood sugar control remains a topic of debate (78, 79).
The inflammatory cytokines identified in this study not only a unique risk for DN, but also associated with other systemic diseases. For example, Huang et al. (80) found that TNFSF14 mediates the impact of docosahexaenoic acid on atopic dermatitis. Yan et al. (81) found that a decrease in IL-1α levels has been associated with the development of intervertebral disc degeneration. TGF-α holds diagnostic value in various diseases, including endometrial cancer (82) and non-small cell lung cancer (83). Its expression levels can serve as a biomarker, aiding in the early detection and diagnosis of these malignancies. However, there is still a lack of MR research evidence linking these inflammatory cytokines to systemic diseases. Future studies are needed to further investigate these associations and establish more robust causal relationships.
Unlike previous MR analyses that explored the relationship between immune cells or inflammatory cytokines and DN, this study stands out in several ways. First, we applied a more stringent threshold of p < 5 ×10−6, whereas others set it at 1 ×10−5, which led to a more selective identification of instrumental variables and a lower risk of pleiotropy. Second, this study assessed 91 inflammatory cytokines, as opposed to the 41 analyzed in earlier research. Third, we used a larger GWAS dataset with a greater number of cases, and we validated our findings using other ethnic GWAS datasets, ensuring the robustness and lack of bias in our results. Fourth, differences in the exposure and outcome datasets led to conclusions that contrast with those of prior studies. Fifth, a FDR correction was implemented, reducing the probability of false positives in the MR results. Additionally, a bidirectional MR analysis was carried out to reliably estimate the causal connection between exposure and disease, thus addressing the reverse causality issue often seen in traditional observational research. Finally, the PhenoScanner database was employed to eliminate potential confounders related to DN. However, certain limitations need to be acknowledged. Firstly, the outcome data originate from European and East Asian populations, other populations should be validated using local data. Secondly, the lack of detailed information, such as type, severity, and duration of diabetes, hinders the possibility of conducting further subgroup analyses. Therefore, they must be validated in future large-sample clinical trials. Thirdly, while MR facilitates the evaluation of the enduring impacts of genetically predisposed inflammatory cytokines throughout an individual’s lifespan, it may not directly encapsulate the attenuation of these factors in adulthood due to the influence of diverse unreported regulators. Fourthly, while we employed various procedures in MR to effectively exclude confounders, there remain potential confounding factors that may affect the study’s accuracy. For example, certain genetic variants can simultaneously influence multiple phenotypes (84). Additionally, for inflammatory factors that did not show statistical significance in our analysis, it is still possible that a causal relationship with DN or T2DM exists, which may not have been detected due to the limited SNPs available for these inflammatory factors (85). Finally, to conduct sensitivity and horizontal pleiotropy analyses, more SNPs needed to be included as IVs, so instead of the traditional significance threshold (p < 5 ×10−8), we chose 5 ×10−6.
5 Conclusion
This study revealed pivotal contributions of FGF19, FGF21, CCL19, CCL11, and IL-10 in the advancement of T2DM. The importance of TNFSF14, TRANCE, IL-1α, TGF-α, and GDNF in the progress of DN. These findings offered favorable implications for the treatment and prevention of T2DM and DN, laying the groundwork for novel clinical approaches and management strategies. However, additional experimental and clinical investigations are necessary to elucidate the functions and molecular mechanisms of these inflammatory factors in future studies.
Data availability statement
The original contributions presented in the study are included in the article/Supplementary material, further inquiries can be directed to the corresponding authors.
Author contributions
SS: Writing – original draft, Writing – review & editing. JN: Writing – review & editing. YS: Writing – review & editing. QP: Writing – review & editing. LZ: Conceptualization, Writing – original draft. QY: Conceptualization, Data curation, Writing – original draft, Writing – review & editing. JY: Data curation, Writing – original draft, Writing – review & editing.
Funding
The author(s) declare that financial support was received for the research, authorship, and/or publication of this article. This study was supported by grants from the National Natural Science Foundation of China (Grant Nos. 82174293 and 82374355), Science and Technology Support Program of Jiangsu Province (ZD202208), Postgraduate Research and Practice Innovation Program of Jiangsu Province (SJCX24_0967), and the Jiangning District Science and Technology Benefiting the People Program (Grant Title: Investigation of the potential mechanisms of Kunkui Baoshen formula in the treatment of diabetic kidney disease based on multi-dimensional data. Grant No. 20244043S).
Acknowledgments
The authors thank all contributors to the IEU OpenGWAS Project.
Conflict of interest
The authors declare that the research was conducted in the absence of any commercial or financial relationships that could be construed as a potential conflict of interest.
Publisher’s note
All claims expressed in this article are solely those of the authors and do not necessarily represent those of their affiliated organizations, or those of the publisher, the editors and the reviewers. Any product that may be evaluated in this article, or claim that may be made by its manufacturer, is not guaranteed or endorsed by the publisher.
Supplementary material
The Supplementary material for this article can be found online at: https://www.frontiersin.org/articles/10.3389/fmed.2024.1459752/full#supplementary-material
Abbreviations
ACEIs, Angiotensin-converting enzyme inhibitors; ARBs, Angiotensin II receptor blockers; BTK, Bruton’s tyrosine kinase; CCL11, Eotaxin levels; CCL19, C-C motif chemokine 19; CCL28, C-C motif chemokine 28; CI, Confidence interval; DN, Diabetic nephropathy; ESRD, End-stage renal disease; FGF19, Fibroblast growth factor 19; FGF21, Fibroblast growth factor 21; ICAM-1, Intercellular cell adhesion molecule-1; IL-1α, Interleukin-1-alpha; IL-1β, Interleukin-1β; IL-6, Interleukin-6; IL-10, Interleukin-10; IL-18, Interleukin-18; IVW, Inverse variance weighted; IVs, Instrumental variables; GDNF, Glial cell line-derived neurotrophic factor; GWAS, Genome-wide association study; HG, High glucose; HMGB1, High-mobility group box 1; LIFR, Leukemia inhibitory factor receptor; LOO, Leave-one-out; MCP-1, Monocyte chemoattractant protein-1; MR, Mendelian randomization; NLRP, NOD-like receptor protein; OR, Odds ratio; PD-L1, Programmed cell death 1 ligand 1; ROS, Reactive oxygen species; SGLT-2i, Sodium-dependent glucose transporters 2 inhibitors; SNPs, Single nucleotide polymorphisms; T2DM, Type 2 diabetes mellitus; TGF-α, Transforming growth factor-alpha; TGF-β1, Transforming growth factor-β1; TLR4, Toll-like receptor 4; TNF-α, Tumor necrosis factor-α; TNF-β, TNF-beta; TNFSF14, Tumor necrosis factor ligand superfamily member 14; TRANCE, TNF-related activation-induced cytokine; TRAIL, TNF-related apoptosis-inducing ligand; VEGFA, Vascular endothelial growth factor A; VCAM-1, Vascular cell adhesion protein-1; WM, Weighted mode; WME, Weighted median.
Footnotes
References
1. Harris, E. Meta-analysis: vitamin D therapy reduced type 2 diabetes. JAMA. (2023) 329:703. doi: 10.1001/jama.2023.1551
2. Liu, L, Chen, Y, Li, X, Wang, J, and Yang, L. Therapeutic potential: the role of mesenchymal stem cells from diverse sources and their derived exosomes in diabetic nephropathy. Biomed Pharmacother. (2024) 175:116672. doi: 10.1016/j.biopha.2024.116672
3. Mise, K, Long, J, Galvan, DL, Ye, Z, Fan, G, Sharma, R, et al. NDUFS4 regulates cristae remodeling in diabetic kidney disease. Nat Commun. (2024) 15:1965. doi: 10.1038/s41467-024-46366-w
5. Perkovic, V, Tuttle, KR, Rossing, P, Mahaffey, KW, Mann, JFE, Bakris, G, et al. Effects of semaglutide on chronic kidney disease in patients with type 2 diabetes. N Engl J Med. (2024) 391:109–21. doi: 10.1056/NEJMoa2403347
6. Pan, HC, Chen, JY, Chen, HY, Yeh, FY, Huang, TT, Sun, CY, et al. Sodium-glucose cotransport protein 2 inhibitors in patients with type 2 diabetes and acute kidney disease. JAMA Netw Open. (2024) 7:e2350050. doi: 10.1001/jamanetworkopen.2023.50050
7. Neuen, BL, Heerspink, HJL, Vart, P, Claggett, BL, Fletcher, RA, Arnott, C, et al. Estimated lifetime cardiovascular, kidney, and mortality benefits of combination treatment with SGLT2 inhibitors, GLP-1 receptor agonists, and nonsteroidal MRA compared with conventional care in patients with type 2 diabetes and albuminuria. Circulation. (2024) 149:450–62. doi: 10.1161/CIRCULATIONAHA.123.067584
8. Hu, S, Hang, X, Wei, Y, Wang, H, Zhang, L, and Zhao, L. Crosstalk among podocytes, glomerular endothelial cells and mesangial cells in diabetic kidney disease: an updated review. Cell Commun Signal. (2024) 22:136. doi: 10.1186/s12964-024-01502-3
9. Wu, XQ, Zhao, L, Zhao, YL, He, XY, Zou, L, Zhao, YY, et al. Traditional Chinese medicine improved diabetic kidney disease through targeting gut microbiota. Pharm Biol. (2024) 62:423–35. doi: 10.1080/13880209.2024.2351946
10. Luo, LP, Suo, P, Ren, LL, Liu, HJ, Zhang, Y, and Zhao, YY. Shenkang injection and its three anthraquinones ameliorates renal fibrosis by simultaneous targeting IkB/NF-kB and Keap 1/Nrf 2 signaling pathways. Front Pharmacol. (2021) 12:800522. doi: 10.3389/fphar.2021.800522
11. Fruhbeck, G, Gomez-Ambrosi, J, Ramirez, B, Becerril, S, Rodriguez, A, Mentxaka, A, et al. Decreased expression of the NLRP6 inflammasome is associated with increased intestinal permeability and inflammation in obesity with type 2 diabetes. Cell Mol Life Sci. (2024) 81:77. doi: 10.1007/s00018-024-05124-3
12. Chang, TT, Lin, LY, and Chen, JW. A novel resolution of diabetes: C-C chemokine motif ligand 4 is a common target in different types of diabetes by protecting pancreatic islet cell and modulating inflammation. Front Immunol. (2021) 12:650626. doi: 10.3389/fimmu.2021.650626
13. Antar, SA, Ashour, NA, Sharaky, M, Khattab, M, Ashour, NA, Zaid, RT, et al. Diabetes mellitus: classification, mediators, and complications; a gate to identify potential targets for the development of new effective treatments. Biomed Pharmacother. (2023) 168:115734. doi: 10.1016/j.biopha.2023.115734
14. Wang, N, and Zhang, C. Oxidative stress: a culprit in the progression of diabetic kidney disease. Antioxidants. (2024) 13:11–9. doi: 10.3390/antiox13040455
15. Rayego-Mateos, S, Rodrigues-Diez, RR, Fernandez-Fernandez, B, Mora-Fernandez, C, Marchant, V, Donate-Correa, J, et al. Targeting inflammation to treat diabetic kidney disease: the road to 2030. Kidney Int. (2023) 103:282–96. doi: 10.1016/j.kint.2022.10.030
16. Fu, J, Sun, Z, Wang, X, Zhang, T, Yuan, W, Salem, F, et al. The single-cell landscape of kidney immune cells reveals transcriptional heterogeneity in early diabetic kidney disease. Kidney Int. (2022) 102:1291–304. doi: 10.1016/j.kint.2022.08.026
17. Jiang, WJ, Xu, CT, Du, CL, Dong, JH, Xu, SB, Hu, BF, et al. Tubular epithelial cell-to-macrophage communication forms a negative feedback loop via extracellular vesicle transfer to promote renal inflammation and apoptosis in diabetic nephropathy. Theranostics. (2022) 12:324–39. doi: 10.7150/thno.63735
18. Tuttle, KR, Agarwal, R, Alpers, CE, Bakris, GL, Brosius, FC, Kolkhof, P, et al. Molecular mechanisms and therapeutic targets for diabetic kidney disease. Kidney Int. (2022) 102:248–60. doi: 10.1016/j.kint.2022.05.012
19. Wang, J, Xu, G, Ning, Y, Wang, X, and Wang, GL. Mitochondrial functions in plant immunity. Trends Plant Sci. (2022) 27:1063–76. doi: 10.1016/j.tplants.2022.04.007
20. Lin, DW, Yang, TM, Ho, C, Shih, YH, Lin, CL, and Hsu, YC. Targeting macrophages: therapeutic approaches in diabetic kidney disease. Int J Mol Sci. (2024) 25:44–8. doi: 10.3390/ijms25084350
21. Alicic, RZ, Rooney, MT, and Tuttle, KR. Diabetic kidney disease: challenges, progress, and possibilities. Clin J Am Soc Nephrol. (2017) 12:2032–45. doi: 10.2215/CJN.11491116
22. Jin, Q, Liu, T, Qiao, Y, Liu, D, Yang, L, Mao, H, et al. Oxidative stress and inflammation in diabetic nephropathy: role of polyphenols. Front Immunol. (2023) 14:1185317. doi: 10.3389/fimmu.2023.1185317
23. An, Y, Xu, BT, Wan, SR, Ma, XM, Long, Y, Xu, Y, et al. The role of oxidative stress in diabetes mellitus-induced vascular endothelial dysfunction. Cardiovasc Diabetol. (2023) 22:237. doi: 10.1186/s12933-023-01965-7
24. Ke, G, Chen, X, Liao, R, Xu, L, Zhang, L, Zhang, H, et al. Receptor activator of NF-kappa B mediates podocyte injury in diabetic nephropathy. Kidney Int. (2021) 100:377–90. doi: 10.1016/j.kint.2021.04.036
25. Wang, Y, Wang, J, Zheng, W, Zhang, J, Wang, J, Jin, T, et al. Identification of an IL-1 receptor mutation driving autoinflammation directs IL-1-targeted drug design. Immunity. (2023) 56:1485–1501.e7. doi: 10.1016/j.immuni.2023.05.014
26. Kong, X, Zhao, Y, Wang, X, Yu, Y, Meng, Y, Yan, G, et al. Loganin reduces diabetic kidney injury by inhibiting the activation of NLRP3 inflammasome-mediated pyroptosis. Chem Biol Interact. (2023) 382:110640. doi: 10.1016/j.cbi.2023.110640
27. Banks, WA, Hansen, KM, Erickson, MA, and Crews, FT. High-mobility group box 1 (HMGB1) crosses the BBB bidirectionally. Brain Behav Immun. (2023) 111:386–94. doi: 10.1016/j.bbi.2023.04.018
28. Liu, X, Lu, B, Fu, J, Zhu, X, Song, E, and Song, Y. Amorphous silica nanoparticles induce inflammation via activation of NLRP3 inflammasome and HMGB1/TLR4/MYD88/NF-kb signaling pathway in HUVEC cells. J Hazard Mater. (2021) 404:124050. doi: 10.1016/j.jhazmat.2020.124050
29. Samms, RJ, Cheng, CC, Fourcaudot, M, Heikkinen, S, Khattab, A, Adams, J, et al. FGF21 contributes to metabolic improvements elicited by combination therapy with exenatide and pioglitazone in patients with type 2 diabetes. Am J Physiol Endocrinol Metab. (2022) 323:E123–32. doi: 10.1152/ajpendo.00050.2022
30. Nishikiori, N, Osanai, M, Chiba, H, Kojima, T, Mitamura, Y, Ohguro, H, et al. Glial cell-derived cytokines attenuate the breakdown of vascular integrity in diabetic retinopathy. Diabetes. (2007) 56:1333–40. doi: 10.2337/db06-1431
31. Zhang, F, Yin, J, Liu, L, Liu, S, Zhang, G, Kong, Y, et al. IL-17C neutralization protects the kidney against acute injury and chronic injury. EBioMedicine. (2023) 92:104607. doi: 10.1016/j.ebiom.2023.104607
32. Murakoshi, M, Gohda, T, and Suzuki, Y. Circulating tumor necrosis factor receptors: a potential biomarker for the progression of diabetic kidney disease. Int J Mol Sci. (2020) 21:99–104. doi: 10.3390/ijms21061957
33. Nakamura, A, Shikata, K, Nakatou, T, Kitamura, T, Kajitani, N, Ogawa, D, et al. Combination therapy with an angiotensin-converting-enzyme inhibitor and an angiotensin II receptor antagonist ameliorates microinflammation and oxidative stress in patients with diabetic nephropathy. J Diabetes Investig. (2013) 4:195–201. doi: 10.1111/jdi.12004
34. Kang, J, Postigo-Fernandez, J, Kim, K, Zhu, C, Yu, J, Meroni, M, et al. Notch-mediated hepatocyte MCP-1 secretion causes liver fibrosis. JCI Insight. (2023) 8:17–21. doi: 10.1172/jci.insight.165369
35. Guo, Q, Jin, Y, Chen, X, Ye, X, Shen, X, Lin, M, et al. NF-kappa B in biology and targeted therapy: new insights and translational implications. Signal Transduct Target Ther. (2024) 9:53. doi: 10.1038/s41392-024-01757-9
36. Huang, G, Lv, J, Li, T, Huai, G, Li, X, Xiang, S, et al. Notoginsenoside R1 ameliorates podocyte injury in rats with diabetic nephropathy by activating the PI3K/Akt signaling pathway. Int J Mol Med. (2016) 38:1179–89. doi: 10.3892/ijmm.2016.2713
37. Yang, M, and Zhang, C. The role of innate immunity in diabetic nephropathy and their therapeutic consequences. J Pharm Anal. (2024) 14:39–51. doi: 10.1016/j.jpha.2023.09.003
38. Tu, Y, Zhang, W, Fan, G, Zou, C, Zhang, J, Wu, N, et al. Paclitaxel-loaded ROS-responsive nanoparticles for head and neck cancer therapy. Drug Deliv. (2023) 30:2189106. doi: 10.1080/10717544.2023.2189106
39. Tu, Y, Chen, C, and Fan, G. Association between the expression of secreted phosphoprotein—related genes and prognosis of human cancer. BMC Cancer. (2019) 19:1230. doi: 10.1186/s12885-019-6441-3
40. Lawlor, DA, Harbord, RM, Sterne, JA, Timpson, N, and Davey Smith, G. Mendelian randomization: using genes as instruments for making causal inferences in epidemiology. Stat Med. (2008) 27:1133–63. doi: 10.1002/sim.3034
41. Larsson, SC, Butterworth, AS, and Burgess, S. Mendelian randomization for cardiovascular diseases: principles and applications. Eur Heart J. (2023) 44:4913–24. doi: 10.1093/eurheartj/ehad736
42. Chang, L, Zhou, G, and Xia, J. mGWAS-explorer 2.0: causal analysis and interpretation of metabolite-phenotype associations. Metabolites. (2023) 13:99–108. doi: 10.3390/metabo13070826
43. Zhao, JH, Stacey, D, Eriksson, N, Macdonald-Dunlop, E, Hedman, AK, Kalnapenkis, A, et al. Genetics of circulating inflammatory proteins identifies drivers of immune-mediated disease risk and therapeutic targets. Nat Immunol. (2023) 24:1540–51. doi: 10.1038/s41590-023-01588-w
44. Sakaue, S, Kanai, M, Tanigawa, Y, Karjalainen, J, Kurki, M, Koshiba, S, et al. A cross-population atlas of genetic associations for 220 human phenotypes. Nat Genet. (2021) 53:1415–24. doi: 10.1038/s41588-021-00931-x
45. Xiang, M, Wang, Y, Gao, Z, Wang, J, Chen, Q, Sun, Z, et al. Exploring causal correlations between inflammatory cytokines and systemic lupus erythematosus: a Mendelian randomization. Front Immunol. (2022) 13:985729. doi: 10.3389/fimmu.2022.985729
46. Liu, B, Lyu, L, Zhou, W, Song, J, Ye, D, Mao, Y, et al. Associations of the circulating levels of cytokines with risk of amyotrophic lateral sclerosis: a Mendelian randomization study. BMC Med. (2023) 21:39. doi: 10.1186/s12916-023-02736-7
47. Zou, M, Zhang, W, Shen, L, Xu, Y, and Zhu, Y. Causal association between inflammatory bowel disease and herpes virus infections: a two-sample bidirectional Mendelian randomization study. Front Immunol. (2023) 14:1203707. doi: 10.3389/fimmu.2023.1203707
48. Burgess, S, and Thompson, SGCRP CHD Genetics Collaboration. Avoiding bias from weak instruments in Mendelian randomization studies. Int J Epidemiol. (2011) 40:755–64. doi: 10.1093/ije/dyr036
49. Burgess, S, and Thompson, SG. Interpreting findings from Mendelian randomization using the MR-Egger method. Eur J Epidemiol. (2017) 32:377–89. doi: 10.1007/s10654-017-0255-x
50. Rogne, T, Gill, D, Liew, Z, Shi, X, Stensrud, VH, Nilsen, TIL, et al. Mediating factors in the association of maternal educational level with pregnancy outcomes: a Mendelian randomization study. JAMA Netw Open. (2024) 7:e2351166. doi: 10.1001/jamanetworkopen.2023.51166
51. Bowden, J, Del Greco, MF, Minelli, C, Davey Smith, G, Sheehan, NA, and Thompson, JR. Assessing the suitability of summary data for two-sample Mendelian randomization analyses using MR-Egger regression: the role of the I2 statistic. Int J Epidemiol. (2016) 45:1961–74. doi: 10.1093/ije/dyw220
52. Storey, JD, and Tibshirani, R. Statistical significance for genomewide studies. Proc Natl Acad Sci USA. (2003) 100:9440–5. doi: 10.1073/pnas.1530509100
53. Bowden, J, Davey Smith, G, and Burgess, S. Mendelian randomization with invalid instruments: effect estimation and bias detection through Egger regression. Int J Epidemiol. (2015) 44:512–25. doi: 10.1093/ije/dyv080
54. Li, W, Xu, JW, Chai, JL, Guo, CC, Li, GZ, Gao, M, et al. Complex causal association between genetically predicted 731 immunocyte phenotype and osteonecrosis: a bidirectional two-sample Mendelian randomization analysis. Int J Surg. (2024) 110:3285–93. doi: 10.1097/JS9.0000000000001327
55. Hayreh, SS. Ocular vascular occlusive disorders: natural history of visual outcome. Prog Retin Eye Res. (2014) 41:1–25. doi: 10.1016/j.preteyeres.2014.04.001
56. Pierce, BL, and Burgess, S. Efficient design for Mendelian randomization studies: subsample and 2-sample instrumental variable estimators. Am J Epidemiol. (2013) 178:1177–84. doi: 10.1093/aje/kwt084
57. Bowden, J, Spiller, W, Del Greco, MF, Sheehan, N, Thompson, J, Minelli, C, et al. Improving the visualization, interpretation and analysis of two-sample summary data Mendelian randomization via the radial plot and radial regression. Int J Epidemiol. (2018) 47:1264–78. doi: 10.1093/ije/dyy101
58. Li, L, Ren, Q, Zheng, Q, Bai, Y, He, S, Zhang, Y, et al. Causal associations between gastroesophageal reflux disease and lung cancer risk: a Mendelian randomization study. Cancer Med. (2023) 12:7552–9. doi: 10.1002/cam4.5498
59. Power, GM, Sanderson, E, Pagoni, P, Fraser, A, Morris, T, Prince, C, et al. Methodological approaches, challenges, and opportunities in the application of Mendelian randomisation to lifecourse epidemiology: a systematic literature review. Eur J Epidemiol. (2023) 39:501–20. doi: 10.1007/s10654-023-01032-1
60. Potthoff, MJ, Boney-Montoya, J, Choi, M, He, T, Sunny, NE, Satapati, S, et al. FGF15/19 regulates hepatic glucose metabolism by inhibiting the CREB-PGC-1alpha pathway. Cell Metab. (2011) 13:729–38. doi: 10.1016/j.cmet.2011.03.019
61. Tang, MJ, Su, JB, Xu, TL, Wang, XQ, Zhang, DM, and Wang, XH. Serum fibroblast growth factor 19 and endogenous islet beta cell function in type 2 diabetic patients. Diabetol Metab Syndr. (2019) 11:79. doi: 10.1186/s13098-019-0475-1
62. Zhang, J, Li, H, Bai, N, Xu, Y, Song, Q, Zhang, L, et al. Decrease of FGF19 contributes to the increase of fasting glucose in human in an insulin-independent manner. J Endocrinol Investig. (2019) 42:1019–27. doi: 10.1007/s40618-019-01018-5
63. Babaknejad, N, Nayeri, H, Hemmati, R, Bahrami, S, and Esmaillzadeh, A. An overview of FGF19 and FGF21: the therapeutic role in the treatment of the metabolic disorders and obesity. Horm Metab Res. (2018) 50:441–52. doi: 10.1055/a-0623-2909
64. Rosales-Soto, G, Diaz-Vegas, A, Casas, M, Contreras-Ferrat, A, and Jaimovich, E. Fibroblast growth factor-21 potentiates glucose transport in skeletal muscle fibers. J Mol Endocrinol. (2020) 65:85–95. doi: 10.1530/JME-19-0210
65. Szczepanska, E, and Gietka-Czernel, M. FGF21: a novel regulator of glucose and lipid metabolism and whole-body energy balance. Horm Metab Res. (2022) 54:203–11. doi: 10.1055/a-1778-4159
66. Motahari Rad, M, Bijeh, N, Attarzadeh Hosseini, SR, and Raouf Saeb, A. The effect of two concurrent exercise modalities on serum concentrations of FGF21, irisin, follistatin, and myostatin in men with type 2 diabetes mellitus. Arch Physiol Biochem. (2023) 129:424–33. doi: 10.1080/13813455.2020.1829649
67. Santopaolo, M, Sullivan, N, Thomas, AC, Alvino, VV, Nicholson, LB, Gu, Y, et al. Activation of bone marrow adaptive immunity in type 2 diabetes: rescue by co-stimulation modulator abatacept. Front Immunol. (2021) 12:609406. doi: 10.3389/fimmu.2021.609406
68. Kochumon, S, Arefanian, H, Sindhu, S, Shenouda, S, Thomas, R, Al-Mulla, F, et al. Adipose tissue steroid receptor RNA activator 1 (SRA1) expression is associated with obesity, insulin resistance, and inflammation. Cells. (2021) 10:99–112. doi: 10.3390/cells10102602
69. Aso, Y, Iijima, T, Jojima, T, Saito, M, Tanuma, D, Kase, M, et al. High serum levels of CCL11/eotaxin-1 are associated with diabetic sensorimotor polyneuropathy and peripheral nerve function but not with cardiac autonomic neuropathy in people with type 2 diabetes. Postgrad Med. (2024) 136:318–24. doi: 10.1080/00325481.2024.2347196
70. Pan, X, Kaminga, AC, Wen, SW, and Liu, A. Chemokines in prediabetes and type 2 diabetes: a meta-analysis. Front Immunol. (2021) 12:622438. doi: 10.3389/fimmu.2021.622438
71. Saxton, RA, Tsutsumi, N, Su, LL, Abhiraman, GC, Mohan, K, Henneberg, LT, et al. Structure-based decoupling of the pro-and anti-inflammatory functions of interleukin-10. Science. (2021) 371:21–38. doi: 10.1126/science.abc8433
72. Jin, Z, Zhang, Q, Liu, K, Wang, S, Yan, Y, Zhang, B, et al. The association between interleukin family and diabetes mellitus and its complications: an overview of systematic reviews and meta-analyses. Diabetes Res Clin Pract. (2024) 210:111615. doi: 10.1016/j.diabres.2024.111615
73. Li, Y, Tang, M, Han, B, Wu, S, Li, SJ, He, QH, et al. Tumor necrosis factor superfamily 14 is critical for the development of renal fibrosis. Aging. (2020) 12:25469–86. doi: 10.18632/aging.104151
74. Lee, SH, Ihm, CG, Sohn, SD, Lee, TW, Kim, MJ, Koh, G, et al. Polymorphisms in interleukin-1 beta and interleukin-1 receptor antagonist genes are associated with kidney failure in Korean patients with type 2 diabetes mellitus. Am J Nephrol. (2004) 24:410–4. doi: 10.1159/000080044
75. Rohm, TV, Meier, DT, Olefsky, JM, and Donath, MY. Inflammation in obesity, diabetes, and related disorders. Immunity. (2022) 55:31–55. doi: 10.1016/j.immuni.2021.12.013
76. Habib, HA, Heeba, GH, and Khalifa, MMA. Comparative effects of incretin-based therapy on early-onset diabetic nephropathy in rats: role of TNF-alpha, TGF-beta and c-caspase-3. Life Sci. (2021) 278:119624. doi: 10.1016/j.lfs.2021.119624
77. Yang, H, Xie, T, Li, D, Du, X, Wang, T, Li, C, et al. Tim-3 aggravates podocyte injury in diabetic nephropathy by promoting macrophage activation via the NF-kappa B/TNF-alpha pathway. Mol Metab. (2019) 23:24–36. doi: 10.1016/j.molmet.2019.02.007
78. Asthana, P, and Wong, HLX. Preventing obesity, insulin resistance and type 2 diabetes by targeting MT1-MMP. Biochim Biophys Acta Mol basis Dis. (2024) 1870:167081. doi: 10.1016/j.bbadis.2024.167081
79. Mullican, SE, Lin-Schmidt, X, Chin, CN, Chavez, JA, Furman, JL, Armstrong, AA, et al. GFRAL is the receptor for GDF15 and the ligand promotes weight loss in mice and nonhuman primates. Nat Med. (2017) 23:1150–7. doi: 10.1038/nm.4392
80. Huang, XW, Pang, SW, Yang, LZ, Han, T, Chen, JM, Huang, CW, et al. TNFSF14 mediates the impact of docosahexaenoic acid on atopic dermatitis: a Mendelian randomization study. Eur Rev Med Pharmacol Sci. (2024) 28:107–17. doi: 10.26355/eurrev_202401_34896
81. Yan, XA, Shen, E, Cui, A, Zhou, F, and Zhuang, Y. Assessing the causal relationship between CRP, IL-1alpha, IL-1beta, and IL-6 levels and intervertebral disc degeneration: a two-sample Mendelian randomization study. Sci Rep. (2024) 14:23716. doi: 10.1038/s41598-024-73205-1
82. Kozlowski, M, Borzyszkowska, D, Mirko, J, Turon-Skrzypinska, A, Piotrowska, K, Toloczko-Grabarek, A, et al. Preoperative serum levels of PDGF-AB, PDGF-BB, TGF-alpha, EGF and ANG-2 in the diagnosis of endometrial Cancer. Cancers. (2023) 15:102–31. doi: 10.3390/cancers15194815
83. Wang, Y, Zhang, Y, Chen, R, and Tian, X. Autocrine EGF and TGF-α promote primary and acquired resistance to ALK/c-Met kinase inhibitors in non-small-cell lung cancer. Pharmacol Res Perspect. (2023) 11:e01047. doi: 10.1002/prp2.1047
84. Posey, JE, Harel, T, Liu, P, Rosenfeld, JA, James, RA, Coban Akdemir, ZH, et al. Resolution of disease phenotypes resulting from multilocus genomic variation. N Engl J Med. (2017) 376:21–31. doi: 10.1056/NEJMoa1516767
Keywords: Mendelian randomization analysis, bidirectional, inflammatory cytokines, type 2 diabetes mellitus, diabetic nephropathy, horizontal pleiotropy
Citation: Song S, Ni J, Sun Y, Pu Q, Zhang L, Yan Q and Yu J (2024) Association of inflammatory cytokines with type 2 diabetes mellitus and diabetic nephropathy: a bidirectional Mendelian randomization study. Front. Med. 11:1459752. doi: 10.3389/fmed.2024.1459752
Edited by:
Ying-Yong Zhao, Northwest University, ChinaReviewed by:
Tiankui Ma, The First Affiliated Hospital of China Medical University, ChinaGeorge Grant, Independent Researcher, Aberdeen, United Kingdom
Copyright © 2024 Song, Ni, Sun, Pu, Zhang, Yan and Yu. This is an open-access article distributed under the terms of the Creative Commons Attribution License (CC BY). The use, distribution or reproduction in other forums is permitted, provided the original author(s) and the copyright owner(s) are credited and that the original publication in this journal is cited, in accordance with accepted academic practice. No use, distribution or reproduction is permitted which does not comply with these terms.
*Correspondence: Qianhua Yan, eW91aGFua3VuMDQyM0AxNjMuY29t; Jiangyi Yu, MTQwMTc0MzExOEBxcS5jb20=