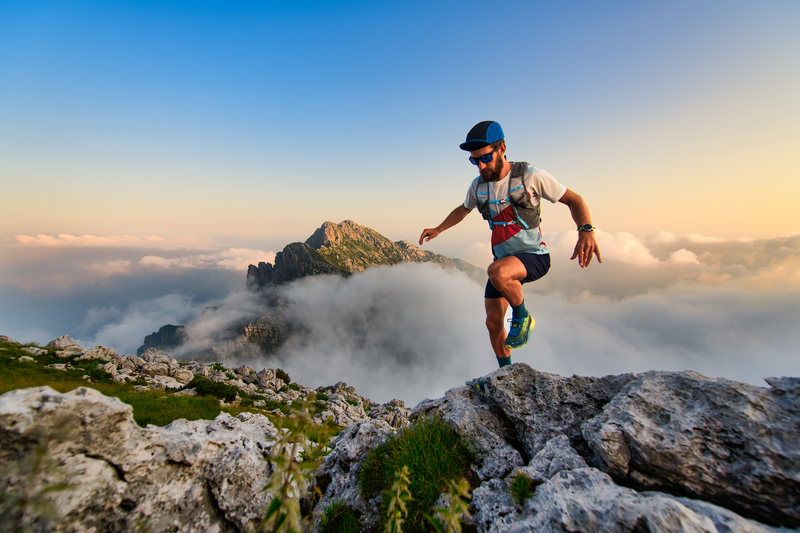
95% of researchers rate our articles as excellent or good
Learn more about the work of our research integrity team to safeguard the quality of each article we publish.
Find out more
ORIGINAL RESEARCH article
Front. Med. , 08 November 2024
Sec. Pulmonary Medicine
Volume 11 - 2024 | https://doi.org/10.3389/fmed.2024.1457048
This article is part of the Research Topic Decoding Immune-Vascular Dynamics for Lung Repair and Therapeutic Innovation View all articles
Background: To identify the risk factors for bronchiectasis patients with active pulmonary tuberculosis (APTB) and to develop a predictive nomogram model for estimating the risk of APTB in bronchiectasis patients.
Methods: A retrospective cohort study was conducted on 16,750 bronchiectasis patients hospitalized at the Affiliated Hospital of Guangdong Medical University and the Second Affiliated Hospital of Guangdong Medical University between January 2019 and December 2023. The 390 patients with APTB were classified as the case group, while 818 patients were randomly sampled by computer at a 1:20 ratio from the 16,360 patients with other infections to serve as the control group. Relevant indicators potentially leading to APTB in bronchiectasis patients were collected. Patients were categorized into APTB and inactive pulmonary tuberculosis (IPTB) groups based on the presence of tuberculosis. The general characteristics of both groups were compared. Variables were screened using the least absolute shrinkage and selection operator (LASSO) analysis, followed by multivariate logistic regression analysis. A nomogram model was established based on the analysis results. The model’s predictive performance was evaluated using calibration curves, C-index, and ROC curves, and internal validation was performed using the bootstrap method.
Results: LASSO analysis identified 28 potential risk factors. Multivariate analysis showed that age, gender, TC, ALB, MCV, FIB, PDW, LYM, hemoptysis, and hypertension are independent risk factors for bronchiectasis patients with APTB (p < 0.05). The nomogram demonstrated strong calibration and discrimination, with a C-index of 0.745 (95% CI: 0.715–0.775) and an AUC of 0.744 for the ROC curve. Internal validation using the bootstrap method produced a C-index of 0.738, further confirming the model’s robustness.
Conclusion: The nomogram model, developed using common clinical serological characteristics, holds significant clinical value for assessing the risk of APTB in bronchiectasis patients.
Bronchiectasis (BE) is a chronic respiratory disease characterized by abnormal and irreversible dilation and distortion of the small airways, which leads to bacterial colonization in the damaged airways and results in chronic cough and sputum production (1). The development of bronchiectasis invariably involves inflammation in the bronchial walls, with most patients exhibiting mixed inflammation predominantly involving neutrophils, though some patients also show increased numbers of eosinophils and monocytes (2, 3). Inflammation has multiple sources, in most cases, it is caused by pathogenic microorganisms (usually bacteria and mycobacteria) (4, 5). Neutrophils play a critical role in the pathogenesis of bronchiectasis by contributing to structural alterations in the bronchial wall, causing damage that leads to bronchial dilation (6).
In Asia, the prevalence of bronchiectasis is significantly higher than in Western countries (7) and APTB is one of the main causes of bronchiectasis (8, 9). According to an analysis of data from the Korean Bronchiectasis Audit and Research Collaboration cohort, APTB is the most common non-idiopathic cause of bronchiectasis, followed by pulmonary infections, asthma, non-tuberculous mycobacterial (NTM) infections, and COPD (10). Post-tuberculosis bronchiectasis prevalence ranges from 35 to 86% based on radiological findings (11). APTB and bronchiectasis are interrelated, and bronchiectasis may occur during tuberculosis or as a sequela of the disease (12). A study has shown that differences in severity and mortality between bronchiectasis patients with APTB and those with bronchiectasis due to other causes (13). Currently, literature reports that there are significant differences (p < 0.05) in serological indicators such as adenosine deaminase, lactate dehydrogenase (14), platelet count, thrombin time, D-dimer (15), and fibrinogen (16) between patients with APTB and healthy individuals. Compared to patients without APTB, patients with APTB show significant differences (p < 0.05) in mean corpuscular volume, erythrocyte sedimentation rate, albumin levels, adenosine deaminase levels, monocyte count to high-density lipoprotein ratio, and high-sensitivity C-reactive protein to lymphocyte count ratio (17). Research has demonstrated that coagulation indicators change following antituberculosis treatment in TB patients, with APTT and Fbg-C levels significantly higher in TB patients compared to healthy individuals (18). This suggests a correlation between coagulation indicators and the APTB.
Currently, there are no reports analyzing the hematological parameters influencing the co-occurrence of bronchiectasis with active pulmonary tuberculosis. This study analyzes the factors affecting patients with bronchiectasis combined with active pulmonary tuberculosis and establishes a predictive model using a nomogram. The nomogram prediction model is simple and practical, allowing for the quantification of the incidence and recurrence of clinical events (19). The aforementioned inflammation and coagulation markers may aid in distinguishing PTB activity. Moreover, these biomarkers are easily accessible, making a scoring system based on them highly applicable in clinical practice, particularly in resource-limited areas. It holds great significance for disease screening.
A retrospective cohort study was conducted on 16,750 bronchiectasis patients hospitalized at the Affiliated Hospital and the Second Affiliated Hospital of Guangdong Medical University from January 2019 to December 2023. Of these, 390 patients were diagnosed with APTB, while 16,360 patients suffered from other bacterial infections (other bacterial infections in the lungs are caused by Gram-negative or Gram-positive bacteria, as confirmed by sputum culture results. These infections are often associated with elevated inflammatory markers such as CRP and procalcitonin, increased neutrophil counts, or symptom improvement following empirical antibiotic treatment, even in the absence of definitive pathogen identification). The 390 patients with APTB were classified as the case group, while 818 patients were randomly sampled by computer at a 1/20 ratio from the 16,360 patients with other infections to serve as the control group. Clinical data were collected for these 1,208 patients, including general information (such as age and gender), and serological characteristics [including: lactate dehydrogenase (LDH), triglycerides (TG), high-density lipoprotein (HDL), low-density lipoprotein (LDL), total cholesterol (TC), albumin (ALB), prothrombin time (PT), activated partial thromboplastin time (APTT), fibrinogen (FIB), thrombin time (TT), monocytes (MON), white blood cells (WBC), lymphocytes (LYM), neutrophils (NEU), red blood cells (RBC), hemoglobin (HGB), red cell distribution width (RDW), mean corpuscular volume (MCV), hematocrit (HCT), platelets (PLT), platelet distribution width (PDW), mean platelet volume (MPV), and plateletcrit (PCT), totaling 36 potential risk factors].
This study was approved by the ethics committees of the Affiliated Hospital of Guangdong Medical University (No: PJKT2024-053) and the Second Affiliated Hospital of Guangdong Medical University (No: PJKT2024-022).
Bronchiectasis Diagnosis Criteria: Patients must meet the criteria outlined in the “European Respiratory Society guidelines for the management of adult bronchiectasis” (20), specifically abnormal radiographic changes indicative of bronchiectasis on high-resolution computed tomography (HRCT). APTB Diagnosis Criteria: Patients must meet the criteria specified in the “WHO consolidated guidelines on tuberculosis: Module 3: Diagnosis – Tests for tuberculosis infection” (21), which include a positive sputum acid-fast bacilli smear and effective anti-tuberculosis treatment, or a positive nucleic acid detection for Mycobacterium tuberculosis in bronchial alveolar lavage fluid using next-generation sequencing (NGS). Patients with bronchiectasis who develop APTB are defined as those in whom bronchiectasis precedes the onset of pulmonary tuberculosis, meaning that bronchiectasis is not caused by tuberculosis.
Exclusion Criteria: (1) Patients with diseases of vital organs such as the liver or kidneys. (2) Patients with concomitant human immunodeficiency virus (HIV) infection and cellular immunodeficiency. (3) Patients with concomitant malignant tumors, autoimmune diseases, or chronic viral infections (such as hepatitis B or hepatitis C). (4) Patients with severe circulatory dysfunction. (5) Patients who have recently received immunostimulant or immunosuppressant therapy. (6) Extrapulmonary infections.
Statistical analyses were performed using SPSS 20.0 software. Count data were expressed as n (%), and comparisons between groups were made using the χ2 test. Normally distributed continuous data were expressed as mean ± standard deviation ( ±s), and comparisons between two groups were made using the independent sample t-test. Non-normally distributed continuous data were expressed as median [M (Q1, Q3)], and comparisons between groups were made using the rank-sum test.
The Least Absolute Shrinkage and Selection Operator (LASSO) regression analysis was employed to identify potential risk factors. Multivariate logistic regression analysis was then conducted on the identified variables to further screen for independent risk factors for bronchiectasis with APTB. The nomogram model was constructed using the “rms” package in R software. The receiver operating characteristic (ROC) curve was plotted to evaluate the model’s discrimination ability, and a calibration curve was drawn to assess and refine the model. The Bootstrap method was used for internal validation. Finally, decision curve analysis (DCA) was performed to evaluate the clinical utility of the model. All analyses were performed using R software (version 4.3.3). p < 0.05 was considered statistically significant.
The case group consisted of 390 patients with APTB, while the control group included 818 patients with other infections. Compared to the control group, the case group showed significantly higher values of LDH, TG, PT, APTT, FIB, TT, MON, WBC, NEU, RDW, PLT and PDW, whereas HDL, LDL, TC, ALB, LYM, HGB, MCV and MPV were significantly lower (p < 0.05) (Table 1).
Patients with concomitant APTB were taken as the dependent variable, while serological and other characteristics were considered as independent variables. To account for multicollinearity among the variables, the optimal penalty coefficient λ was determined through five-fold cross-validation in the LASSO regression model. This process ultimately identified 28 potential risk factors: age, gender, LDH, TG, HDL, TC, ALB, PT, APTT, FIB, TT, MON, WBC, LYM, NEU, HCT, MCV, RDW, PLT, MPV, PCT, PDW, drinking, cough, vomica, hemoptysis, polypnea, and hypertension (p < 0.05) (Figure 1).
Figure 1. (A) Distribution of LASSO coefficients for the risk characteristics. The coefficient profile is plotted against the log (λ) sequence. The optimal lambda results in 28 features with non-zero coefficients. (B) Selection of the optimal parameters (lambda, λ) for the LASSO model using five-fold cross-validation. The binomial deviance curve is plotted against log (lambda). Dotted vertical lines are drawn at the optimal values using the minimum criterion and the 1-standard error (1-SE) criterion.
The 28 potential risk factors were included in the multivariate logistic regression model, with independent variables transformed into binary variables. The results showed that age, gender, TC, ALB, MCV, FIB, PDW, LYM, hemoptysis, and hypertension are independent risk factors for the occurrence of APTB in bronchiectasis patients (p < 0.05) (Table 2).
Table 2. Predictive factors for bronchiectasis with active pulmonary tuberculosis identified by multivariate logistic regression analysis.
Based on the results of the multivariate logistic regression analysis and considering clinical practicality (22), we eventually selected seven independent risk factors for the nomogram analysis: age, gender, MCV, TC, ALB, FIB, and LYM. The nomogram evaluation model was constructed using the “rms” package in R software. Each factor was assigned a corresponding score, and the total score was the sum of the scores for each factor. The higher the total score, the higher the probability of bronchiectasis patients developing APTB (Figure 2).
Figure 2. Nomogram model for predicting the risk of concomitant active pulmonary tuberculosis in bronchiectasis patients. Each variable is listed on the left side of the image. Each category for the variables is associated with a certain number of points. These points are found by drawing a vertical line up to the “Points” scale at the top of the nomogram. After calculating the points for each variable based on the patient’s characteristics, you sum up the total points. The total points can be located along the “Total Points” scale at the bottom of the chart. After identifying the total points, you draw a vertical line from the “Total Points” row down to the “Risk of TB” scale. This will give you the predicted probability of developing TB based on the given characteristics.
The calibration curve indicated strong consistency within this cohort (Figure 3A). The C-index of the nomogram prediction model was 0.745 (95% CI: 0.715–0.775), and internal validation using the Bootstrap method yielded a C-index of 0.738, indicating good discriminative ability. Additionally, the ROC curve generated from the multi-center cohort demonstrated robust predictive value, with an AUC of 0.744 (Figure 3B).
Figure 3. (A) Calibration curve. The solid line represents the performance of the nomogram, with closer proximity to the diagonal dashed line indicating better predictive accuracy. (B) ROC curve representing the discriminatory power of the model. The dashed line indicates the 95% confidence interval.
Clinical decision analysis is presented in Figure 4. When the threshold probabilities for patients and doctors are 8 and 79%, respectively, the nomogram model’s prediction of APTB infection risk provides greater benefits.
Figure 4. Decision curve for the nomogram. The thin solid line represents the assumption that all patients are in the active tuberculosis phase, while the thick solid line represents the assumption that bronchiectasis patients have other concurrent infections. The decision curve indicates that if the threshold probabilities for patients and doctors are 8 and 79%, respectively, using this nomogram prediction model to assess the risk of concomitant active pulmonary tuberculosis provides greater benefit compared to conventional prediction methods in the current study.
The APTB is a significant public health issue worldwide, making the timely and accurate identification of suspected cases crucial. APTB and bronchiectasis are interrelated. Our study highlights the risk of APTB in bronchiectasis patients and identifies a total of 28 risk factors through LASSO regression. Further multivariate logistic regression analysis identified 10 independent risk factors: age, gender, TC, ALB, MCV, FIB, PDW, LYM, hemoptysis, and hypertension. Considering clinical practicality, we selected 7 independent risk factors for inclusion in the nomogram analysis: age, gender, MCV, TC, ALB, FIB, and LYM.
Studies have shown that elderly individuals are more susceptible to infectious diseases, particularly respiratory infections (23). The elderly is considered a major reservoir for Mycobacterium tuberculosis infection due to their increased susceptibility to new infections and the reactivation of latent Mycobacterium tuberculosis infection (24). However, a retrospective cohort study by Abbara et al. (25) indicated that patients aged 65 and above are less likely to exhibit the “classic” clinical or radiological features of APTB, which may lead to delays between symptom onset and diagnosis. Our nomogram prediction model suggests a lower prevalence of APTB in elderly individuals, which may be attributed to decreased immune sensitivity in this population. Van Zyl Smit et al. (26) provided evidence that more men than women are infected with APTB (26). However, some scientists believe that the incidence of APTB infection in women may be underestimated in developing regions (27). In our study, gender was identified as one of the predictive factors, with the incidence of APTB being higher in men than in women. Previous studies have shown that MCV is a marker of pulmonary inflammation (28). In patients with APTB, MCV levels are negatively correlated with pulmonary bacterial load (29), and hypoalbuminemia is positively correlated with the severity of APTB (30). Our case group had lower levels of TC, ALB, and MCV, likely due to the high replication rate and activity of tuberculosis in active pulmonary tuberculosis patients, which leads to greater nutritional consumption. APTB patients are attacked by toxins and bacteria, which can activate immune cells in the lung tissue and release inflammatory mediators (16). This, in turn, activates platelets and promotes their adhesion and aggregation, therefore, patients with APTB have a hypercoagulable state (16). In our study, FIB levels were higher in the case group, consistent with previous research findings. When an inflammatory response occurs, the counts of white blood cells and neutrophils in the blood significantly increase (31). Our control group consisted of bronchiectasis patients with other infections, primarily bacterial pneumonia, at our hospital. Therefore, compared to the case group, the control group had higher LYM levels. Controversially, few studies have demonstrated that ESR and CRP are sensitive markers for tuberculosis (32). However, hematological parameters such as hemoglobin, PCV, RBC count, blood indices, platelet count, WBC count, and ESR can still be utilized for diagnosis, prognosis, and follow-up of patients (33). Inflammatory biomarkers like MLR, CRP, and hs-CRP have been shown to be associated with TB diagnosis (18). CRP, in particular, may be a reliable indicator for diagnosing TB co-infected with other infections (34).
Nomograms are widely used for predicting tumors and various diseases due to their user-friendly digital interface, high predictive accuracy, and ease of understanding (35, 36). Compared to traditional prediction models, they facilitate more rational clinical decision-making (37, 38). The model calculates the risk of APTB, providing insights and methods for its early prevention. The higher the nomogram score, the greater the risk of developing APTB (C-index of 0.745; 95% CI: 0.715–0.775). The established nomogram model was validated using ROC and calibration curves, demonstrating that the predicted probability of APTB risk closely matches the actual occurrence (AUC = 0.744).
However, this study has some limitations: Firstly, since the participants in this study were aged over 18 years, the performance of the diagnostic model in individuals aged under 18 years remains unclear; the data were sourced from only two hospitals, which may introduce selection bias; the retrospective nature of the study may also introduce potential biases in data collection, such as missing or incomplete clinical information. Secondly, although we carefully screened the study subjects and employed multivariate logistic regression to account for confounding variables, potential confounding effects may still persist. Further studies with more homogenous control groups are needed to validate our findings. Overall, the model demonstrates strong clinical utility and can serve as an effective tool for early screening or auxiliary diagnosis, particularly in economically underdeveloped regions or primary healthcare settings.
This study developed a nomogram predictive model with good accuracy and clinical applicability that consists of seven indicators: age, gender, ALB, MCV, FIB, hemoptysis, and hypertension. This model could be valuable in assessing the risk of APTB in patients with bronchiectasis.
The original contributions presented in the study are included in the article/supplementary material, further inquiries can be directed to the corresponding authors.
The studies involving humans were approved by Clinical Research Ethics Committee, Affiliated Hospital of Guangdong Medical University and Medical Ethics Committee, Second Affiliated Hospital of Guangdong Medical University. The studies were conducted in accordance with the local legislation and institutional requirements. The participants provided their written informed consent to participate in this study. Written informed consent was obtained from the individual(s) for the publication of any potentially identifiable images or data included in this article.
YY: Formal analysis, Methodology, Software, Supervision, Writing – original draft. LD: Investigation, Methodology, Writing – original draft. WLY: Methodology, Writing – original draft. WL: Conceptualization, Methodology, Writing – original draft. ZZ: Conceptualization, Investigation, Writing – original draft. XL: Data curation, Investigation, Writing – review & editing. FC: Data curation, Methodology, Writing – review & editing. JP: Data curation, Formal analysis, Writing – review & editing. BC: Formal analysis, Supervision, Writing – review & editing. RC: Funding acquisition, Resources, Writing – review & editing. WMY: Funding acquisition, Resources, Validation, Writing – review & editing.
The author(s) declare that financial support was received for the research, authorship, and/or publication of this article. This study was funded by the Natural Science Foundation of Basic and Applied Basic Research Fund of Guangdong Province (No. 2022A1515012375), the Guangdong Medical Research Fund Project (Nos. A2024728 and A2024723), the Science and Technology Development Special Project of Zhanjiang City (Nos. 2022A01142 and 2022A01110), and the Science and Technology Development Special Fund Competitive Allocation Project of Zhanjiang City (2021A05086).
We would also like to thank everyone who has helped with our research.
The authors declare that the research was conducted in the absence of any commercial or financial relationships that could be construed as a potential conflict of interest.
All claims expressed in this article are solely those of the authors and do not necessarily represent those of their affiliated organizations, or those of the publisher, the editors and the reviewers. Any product that may be evaluated in this article, or claim that may be made by its manufacturer, is not guaranteed or endorsed by the publisher.
1. Kelly, C, Chalmers, JD, Crossingham, I, Relph, N, Felix, LM, Evans, DJ, et al. Macrolide antibiotics for bronchiectasis. Cochrane Database Syst Rev. (2018) 3:CD012406. doi: 10.1002/14651858.CD012406.pub2
2. Chalmers, JD, Chang, AB, Chotirmall, SH, Dhar, R, and McShane, PJ. Bronchiectasis. Nat Rev Dis Primers. (2018) 4:45. doi: 10.1038/s41572-018-0042-3
3. Long, MB, and Chalmers, JD. Treating neutrophilic inflammation in airways diseases. Arch Bronconeumol. (2022) 58:463–5. doi: 10.1016/j.arbres.2021.11.003
4. Aogain, MM, Jaggi, TK, and Chotirmall, SH. The airway microbiome: present and future applications. Arch Bronconeumol. (2022) 58:8–10. doi: 10.1016/j.arbres.2021.08.003
5. Solarat, B, Perea, L, Faner, R, de La Rosa, D, Martinez-Garcia, MA, and Sibila, O. Pathophysiology of chronic bronchial infection in bronchiectasis. Arch Bronconeumol. (2023) 59:101–8. doi: 10.1016/j.arbres.2022.09.004
6. Martínez-García, M, Sánchez, CP, and Moreno, RM. The double-edged sword of neutrophilic inflammation in bronchiectasis. Eur Respir J. (2015) 46:898–900. doi: 10.1183/13993003.00961-2015
7. Choi, H, Yang, B, Nam, H, Kyoung, DS, Sim, YS, Park, HY, et al. Population-based prevalence of bronchiectasis and associated comorbidities in South Korea. Eur Respir J. (2019) 54:1900194. doi: 10.1183/13993003.00194-2019
8. Dhar, R, Singh, S, Talwar, D, Mohan, M, Tripathi, SK, Swarnakar, R, et al. Bronchiectasis in India: results from the European multicentre bronchiectasis audit and research collaboration (EMBARC) and respiratory research network of India registry. Lancet Glob Health. (2019) 7:e1269–79. doi: 10.1016/S2214-109X(19)30327-4
9. Qi, Q, Wang, W, Li, T, Zhang, Y, and Li, Y. Aetiology and clinical characteristics of patients with bronchiectasis in a Chinese Han population: a prospective study. Respirology. (2015) 20:917–24. doi: 10.1111/resp.12574
10. Choi, H, Ryu, J, Kim, Y, Yang, B, Hwangbo, B, Kong, SY, et al. Incidence of bronchiectasis concerning tuberculosis epidemiology and other ecological factors: a Korean National Cohort Study. ERJ Open Res. (2020) 6:00097–2020. doi: 10.1183/23120541.00097-2020
11. Meghji, J, Simpson, H, Squire, SB, and Mortimer, K. A systematic review of the prevalence and pattern of imaging defined post-TB lung disease. PLoS One. (2016) 11:e0161176. doi: 10.1371/journal.pone.0161176
12. Meghji, J, Nadeau, G, Davis, KJ, Wang, D, Nyirenda, MJ, Gordon, SB, et al. Non-communicable lung disease in sub-Saharan Africa. A community-based cross-sectional study of adults in urban Malawi. Am J Respir Crit Care Med. (2016) 194:67–76. doi: 10.1164/rccm.201509-1807OC
13. Al-Harbi, A, Al-Ghamdi, M, Khan, M, Al-Rajhi, S, and Al-Jahdali, H. Performance of multidimensional severity scoring Systems in Patients with post-tuberculosis bronchiectasis. Int J Chron Obstruct Pulmon Dis. (2020) 15:2157–65. doi: 10.2147/COPD.S261797
14. Emad, A, and Rezaian, GR. Lactate dehydrogenase in bronchoalveolar lavage fluid of patients with active pulmonary tuberculosis. Respiration. (1999) 66:41–5. doi: 10.1159/000029335
15. Min, W, Zi-Feng, J, Jian-Lin, X, and Hao-Hui, F. Role of the fibrinogen degradation products and D-dimer in the differential diagnosis of pulmonary tuberculosis and community-acquired pneumonia. Clin Lab. (2018) 64:135–140. doi: 10.7754/Clin.Lab.2017.170720
16. Yu, Z, Shang, Z, Huang, Q, Wen, F, and Patil, S. Integrating systemic immune-inflammation index, fibrinogen, and T-SPOT.TB for precision distinction of active pulmonary tuberculosis in the era of mycobacterial disease research. Front Microbiol. (2024) 15:1382665. doi: 10.3389/fmicb.2024.1382665
17. Yu, Q, Yan, J, Tian, S, Weng, W, Luo, H, Wei, G, et al. A scoring system developed from a nomogram to differentiate active pulmonary tuberculosis from inactive pulmonary tuberculosis. Front Cell Infect Microbiol. (2022) 12:947954. doi: 10.3389/fcimb.2022.947954
18. Yu, Q, Guo, J, and Gong, F. Construction and validation of a diagnostic scoring system for predicting active pulmonary tuberculosis in patients with positive T-SPOT based on indicators associated with coagulation and inflammation: a retrospective cross-sectional study. Infect Drug Resist. (2023) 16:5755–64. doi: 10.2147/IDR.S410923
19. Park, SY. Nomogram: an analogue tool to deliver digital knowledge. J Thorac Cardiovasc Surg. (2018) 155:1793. doi: 10.1016/j.jtcvs.2017.12.107
20. Polverino, E, Goeminne, PC, McDonnell, MJ, Aliberti, S, Marshall, SE, Loebinger, MR, et al. European Respiratory Society guidelines for the management of adult bronchiectasis. Eur Respir J. (2017) 50:1700629. doi: 10.1183/13993003.00629-2017
21. WHO Consolidated Guidelines on Tuberculosis. Module 3: Diagnosis-tests for tuberculosis infection. Geneva: WHO Guidelines Approved by the Guidelines Review Committee (2022).
22. Sun, X, Zheng, Z, Liang, J, Chen, R, Huang, H, Yao, X, et al. Development and validation of a simple clinical nomogram for predicting obstructive sleep apnea. J Sleep Res. (2022) 31:e13546. doi: 10.1111/jsr.13546
23. Olmo-Fontanez, AM, and Turner, J. Tuberculosis in an aging world. Pathogens. (2022) 11:10. doi: 10.3390/pathogens11101101
24. Di Gennaro, F, Vittozzi, P, Gualano, G, Musso, M, Mosti, S, Mencarini, P, et al. Active pulmonary tuberculosis in elderly patients: a 2016-2019 retrospective analysis from an Italian referral hospital. Antibiotics. (2020) 9:489. doi: 10.3390/antibiotics9080489
25. Abbara, A, Collin, SM, Kon, OM, Buell, K, Sullivan, A, Barrett, J, et al. Time to diagnosis of tuberculosis is greater in older patients: a retrospective cohort review. ERJ Open Res. (2019) 5:00228–2018. doi: 10.1183/23120541.00228-2018
26. van Zyl Smit, RN, Pai, M, Yew, WW, Leung, CC, Zumla, A, Bateman, ED, et al. Global lung health: the colliding epidemics of tuberculosis, tobacco smoking, HIV and COPD. Eur Respir J. (2010) 35:27–33. doi: 10.1183/09031936.00072909
27. Borgdorff, MW, Nagelkerke, NJ, Dye, C, and Nunn, P. Gender and tuberculosis: a comparison of prevalence surveys with notification data to explore sex differences in case detection. Int J Tuberc Lung Dis. (2000) 4:123–32.
28. Abba, AA. Exhaled nitric oxide in diagnosis and management of respiratory diseases. Ann Thorac Med. (2009) 4:173–81. doi: 10.4103/1817-1737.56009
29. Baruch Baluku, J, Musaazi, J, Mulwana, R, Bengo, D, Sekaggya Wiltshire, C, and Andia-Biraro, I. Sensitivity and specificity of the mean corpuscular volume and CD4/CD8 ratio in discriminating between rifampicin resistant and rifampicin sensitive tuberculosis. J Clin Tuberc Other Mycobact Dis. (2020) 21:100205. doi: 10.1016/j.jctube.2020.100205
30. Xu, F, Qu, S, Wang, L, and Qin, Y. Mean platelet volume (MPV): new diagnostic indices for co-morbidity of tuberculosis and diabetes mellitus. BMC Infect Dis. (2021) 21:461. doi: 10.1186/s12879-021-06152-1
31. Wyllie, DH, Bowler, IC, and Peto, TE. Relation between lymphopenia and bacteraemia in UK adults with medical emergencies. J Clin Pathol. (2004) 57:950–5. doi: 10.1136/jcp.2004.017335
32. Shah, AR, Desai, KN, and Maru, AM. Evaluation of hematological parameters in pulmonary tuberculosis patients. J Family Med Prim Care. (2022) 11:4424–8. doi: 10.4103/jfmpc.jfmpc_2451_21
33. Peresi, E, Silva, SM, Calvi, SA, and Marcondes-Machado, J. Cytokines and acute phase serum proteins as markers of inflammatory regression during the treatment of pulmonary tuberculosis. J Bras Pneumol. (2008) 34:942–9. doi: 10.1590/S1806-37132008001100009
34. Ciccacci, F, Floridia, M, Bernardini, R, Sidumo, Z, Mugunhe, RJ, Andreotti, M, et al. Plasma levels of CRP, neopterin and IP-10 in HIV-infected individuals with and without pulmonary tuberculosis. J Clin Tuberc Other Mycobact Dis. (2019) 16:100107. doi: 10.1016/j.jctube.2019.100107
35. Huang, X, Luo, Z, Liang, W, Xie, G, Lang, X, Gou, J, et al. Survival nomogram for young breast Cancer patients based on the SEER database and an external validation cohort. Ann Surg Oncol. (2022) 29:5772–81. doi: 10.1245/s10434-022-11911-8
36. Liu, H, Li, Z, Zhang, Q, Li, Q, Zhong, H, Wang, Y, et al. Multi-institutional development and validation of a nomogram to predict prognosis of early-onset gastric cancer patients. Front Immunol. (2022) 13:1007176. doi: 10.3389/fimmu.2022.1007176
37. Balachandran, VP, Gonen, M, Smith, JJ, and DeMatteo, RP. Nomograms in oncology: more than meets the eye. Lancet Oncol. (2015) 16:e173–80. doi: 10.1016/S1470-2045(14)71116-7
Keywords: active pulmonary tuberculosis, bronchiectasis, nomogram, prediction model, infection
Citation: Yang Y, Du L, Ye W, Liao W, Zheng Z, Lin X, Chen F, Pan J, Chen B, Chen R and Yao W (2024) Analysis of factors influencing bronchiectasis patients with active pulmonary tuberculosis and development of a nomogram prediction model. Front. Med. 11:1457048. doi: 10.3389/fmed.2024.1457048
Received: 30 June 2024; Accepted: 28 October 2024;
Published: 08 November 2024.
Edited by:
Bisheng Zhou, University of Illinois Chicago, United StatesCopyright © 2024 Yang, Du, Ye, Liao, Zheng, Lin, Chen, Pan, Chen, Chen and Yao. This is an open-access article distributed under the terms of the Creative Commons Attribution License (CC BY). The use, distribution or reproduction in other forums is permitted, provided the original author(s) and the copyright owner(s) are credited and that the original publication in this journal is cited, in accordance with accepted academic practice. No use, distribution or reproduction is permitted which does not comply with these terms.
*Correspondence: Weimin Yao, NDkwMjk2NDQzQHFxLmNvbQ==; Riken Chen, Y2hlbnJpa2VuQDEyNi5jb20=
†These authors have contributed equally to this work
Disclaimer: All claims expressed in this article are solely those of the authors and do not necessarily represent those of their affiliated organizations, or those of the publisher, the editors and the reviewers. Any product that may be evaluated in this article or claim that may be made by its manufacturer is not guaranteed or endorsed by the publisher.
Research integrity at Frontiers
Learn more about the work of our research integrity team to safeguard the quality of each article we publish.