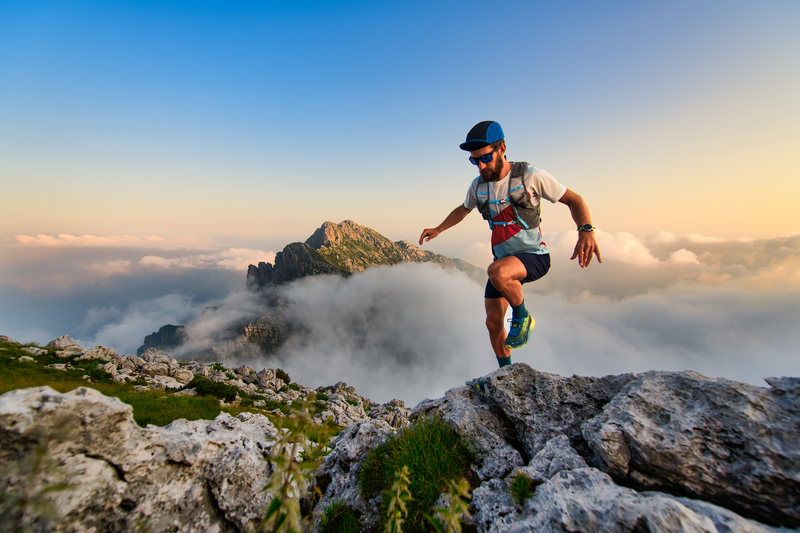
95% of researchers rate our articles as excellent or good
Learn more about the work of our research integrity team to safeguard the quality of each article we publish.
Find out more
EDITORIAL article
Front. Med. , 27 June 2024
Sec. Pathology
Volume 11 - 2024 | https://doi.org/10.3389/fmed.2024.1447294
This article is part of the Research Topic Artificial Intelligence-Assisted Medical Imaging Solutions for Integrating Pathology and Radiology Automated Systems View all 9 articles
Editorial on the Research Topic
Artificial intelligence-assisted medical imaging solutions for integrating pathology and radiology automated systems
Pathology and radiology scientists are showing interest in Artificial Intelligence (AI) in the recent time. The advancement of cancer diagnostic methods often incorporates insights from both the domains of pathology and radiography. An integrated diagnostic reporting system that combines textual analysis, representative visuals, and molecular diagnostic data would be advantageous in facilitating informed judgments on the advancement of diagnostic technologies. Artificial intelligence is currently extensively used in a range of diagnostic applications, such as radiography, cancer detection, and infectious disease identification. It is crucial to acknowledge that clinical diagnosis, translational research, and fundamental research are interrelated and might potentially gain advantages from the expanded capabilities of AI. A total of eight publications have been published on this Research Topic. The objective of this Research Topic is to pinpoint the fundamental research challenges related to pathology and radiology in relation to the growing use of AI technology. Furthermore, it is important to have a more profound understanding of the possible uses of AI technology in practical situations.
A total of eight publications have been published on this Research Topic. Waseem Sabir et al. compare the effectiveness of four vision transformers in terms of their capacity to locate anomalies in images derived from computerized tomography (CT) scans and to correctly identify and categorize patients with pulmonary fibrosis. Yuenyong et al. suggested using AI to create a system that can automatically identify centroblast cells by scanning patches from a whole slide image. The AI system is based on the object detection concept, and the only type of object of interest is the centroblast cell. Using 1,669 sample centroblast cell occurrences from whole slide image of five distinct patients, we trained the AI model. The data was divided into training and validation sets of 80% and 20%, respectively. Rauf et al. suggested an 82-layer deep learning architecture based on the residual bottleneck technique has been suggested. An automated computer-aided diagnostic system is needed to identify common maternal fetuses from ultrasound images. The suggested design has incorporated three residual blocks, each with two highway pathways and one skip link. Albalawi et al. investigated the histological images of Oral Squamous Cell Carcinoma and oral epithelium's capacity for discrimination. With a publicly accessible database of 1,224 images taken at different magnifications from 230 patients, a customized deep learning model built on top of EfficientNetB3 was created to distinguish between normal epithelium and OSCC tissues.
Binzagr focuses on the use of SHapley Additive exPlanations (SHAP) technique to understand model predictions. The intermediate layer in this research consisted of a hybrid model of three Convolutional Neural Networks (CNNs)—InceptionV3, InceptionResNetV2, and VGG16—which merged their predictions. This text provides an elucidation of the choice that impacts the model's forecast. Chauhan et al. primarily focuses on the detection of brain tumors to enhance medical study. A Balanced binary Tree CNN (BT-CNN) is presented to enhance the performance of brain tumor classification. The BT-CNN is designed with a binary tree-like structure. The system consists of two separate modules: the convolution module and the depthwise separable convolution group module. Al Moteri et al. presents a sophisticated machine learning model that relies on EfficientNetV2. This model integrates cutting-edge methods in image processing with neural network design, with the goal of enhancing accuracy, speed, and resilience in classification. They used the EfficientNetV2 model, which was specifically adapted for the precise goal of classifying breast cancer images. Imran et al. provide a transformer-based model that can segment histopathological images, distinguishing between inflammation and other types of malignancies. Additionally, the model can detect skin tissues and borders, which are crucial for decision-making purposes. Precise segmentation of these tissue types will ultimately result in precise identification and categorization of non-melanoma skin cancer.
This editorial published eight research papers that specifically investigated the use of AI-Assisted medical imaging solutions aimed at integrating automated systems in pathology and radiology. The objective was to curate relevant publications within the AI-driven medical and healthcare sector. The findings from this topic highlight a growing trend in the development and research of AI-based medical imaging solutions. To further advance this field, future methodologies could focus on leveraging AI techniques to seamlessly integrate automated systems in both pathology and radiology.
PS: Writing – review & editing, Writing – original draft. MD: Writing – review & editing, Writing – original draft. VR: Writing – review & editing, Investigation, Conceptualization. AB: Writing – review & editing, Investigation, Conceptualization. TA: Writing – review & editing, Investigation, Conceptualization.
The author(s) declare that no financial support was received for the research, authorship, and/or publication of this article.
We express our gratitude to the authors for their significant contributions to this Research Topic. We extend our sincere gratitude to the dedicated reviewers for their thorough and timely evaluations, which greatly improved the quality of this publication. Finally, we would like to express our gratitude to the Frontiers in Medicine journal editorial staff for their unwavering support, which has been crucial in realizing this Research Topic.
The authors declare that the research was conducted in the absence of any commercial or financial relationships that could be construed as a potential conflict of interest.
The author(s) declared that they were an editorial board member of Frontiers, at the time of submission. This had no impact on the peer review process and the final decision.
All claims expressed in this article are solely those of the authors and do not necessarily represent those of their affiliated organizations, or those of the publisher, the editors and the reviewers. Any product that may be evaluated in this article, or claim that may be made by its manufacturer, is not guaranteed or endorsed by the publisher.
Keywords: pathology, radiology, computer-aided diagnosis, explainable AI, generalized models
Citation: Singh P, Diwakar M, Ravi V, Bijalwan A and Agrawal T (2024) Editorial: Artificial intelligence-assisted medical imaging solutions for integrating pathology and radiology automated systems. Front. Med. 11:1447294. doi: 10.3389/fmed.2024.1447294
Received: 11 June 2024; Accepted: 17 June 2024;
Published: 27 June 2024.
Edited and reviewed by: Luigi M. Terracciano, University of Basel, Switzerland
Copyright © 2024 Singh, Diwakar, Ravi, Bijalwan and Agrawal. This is an open-access article distributed under the terms of the Creative Commons Attribution License (CC BY). The use, distribution or reproduction in other forums is permitted, provided the original author(s) and the copyright owner(s) are credited and that the original publication in this journal is cited, in accordance with accepted academic practice. No use, distribution or reproduction is permitted which does not comply with these terms.
*Correspondence: Manoj Diwakar, bWFub2ouZGl3YWthckBnbWFpbC5jb20=
Disclaimer: All claims expressed in this article are solely those of the authors and do not necessarily represent those of their affiliated organizations, or those of the publisher, the editors and the reviewers. Any product that may be evaluated in this article or claim that may be made by its manufacturer is not guaranteed or endorsed by the publisher.
Research integrity at Frontiers
Learn more about the work of our research integrity team to safeguard the quality of each article we publish.