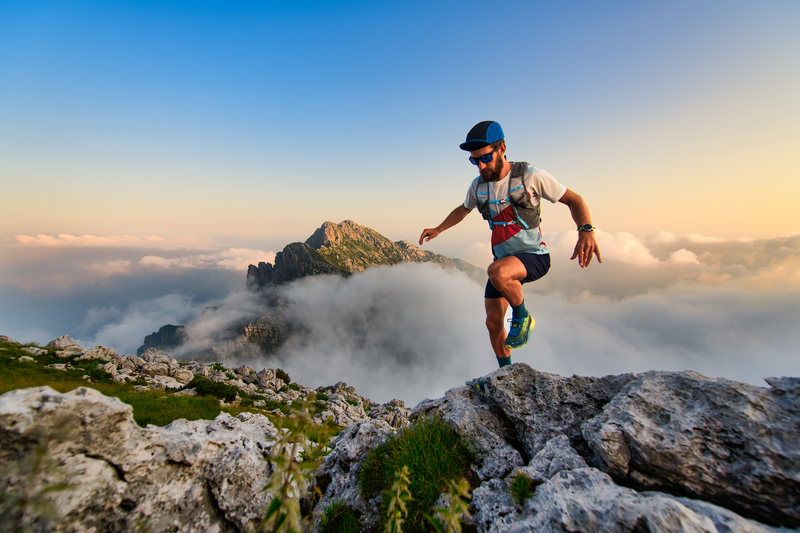
95% of researchers rate our articles as excellent or good
Learn more about the work of our research integrity team to safeguard the quality of each article we publish.
Find out more
ORIGINAL RESEARCH article
Front. Med. , 04 September 2024
Sec. Intensive Care Medicine and Anesthesiology
Volume 11 - 2024 | https://doi.org/10.3389/fmed.2024.1433809
Object: Establish a clinical prognosis model of coronary heart disease (CHD) to predict 28-day mortality in patients with sepsis.
Method: The data were collected retrospectively from septic patients with a previous history of coronary heart disease (CHD) from the Medical Information Mart for Intensive Care (MIMIC)-III database. The included patients were randomly divided into the training cohorts and validation cohorts. The variables were selected using the backward stepwise selection method of Cox regression, and a nomogram was subsequently constructed. The nomogram was compared to the Sequential Organ Failure Assessment (SOFA) model using the C-index, area under the receiver operating characteristic curve (AUC) over time, Net reclassification index (NRI), Integrated discrimination improvement index (IDI), calibration map, and decision curve analysis (DCA).
Result: A total of 800 patients were included in the study. We developed a nomogram based on age, diastolic blood pressure (DBP), pH, lactate, red blood cell distribution width (RDW), anion gap, valvular heart disease, peripheral vascular disease, and acute kidney injury (AKI) stage. The nomogram was evaluated using C-index, AUC, NRI, IDI, calibration plot, and DCA. Our findings revealed that this nomogram outperformed the SOFA score in predicting 28-day mortality in sepsis patients.
Sepsis is caused by an acute infection, which triggers an exaggerated and dysregulated immune response in the host, leading to dysfunction of multiple organs (1). Every year, there are approximately 49 million sepsis patients worldwide (2). Around 30% of patients in the ICU are diagnosed with sepsis (3). The mortality of sepsis can reach up to 40% (4). Patients with sepsis often require different treatment options and may have varying outcomes due to diverse infectious factors, individual variations, and medical history. It is not feasible to evaluate and guide all sepsis patients using a single scoring criterion. The heterogeneity of sepsis patients should receive increasing attention (5, 6).
Coronary heart disease (CHD) is a significant factor that affects the treatment and prognosis of septic patients, and the incidence of fatal CHD in sepsis patients is higher compared to non-septic patients (7). The pathophysiological mechanisms of CHD involve vascular and systemic inflammation, prothrombotic states, vascular stress, altered vascular tone, disrupted hemodynamic homeostasis, and imbalanced metabolism (8). These vascular lesions manifest prominently during the pathological process of sepsis (9, 10). It is often observed in the management of sepsis patients that they have a history of previous CHD. Infection can trigger various cardiovascular events in patients with CHD, including cardiac function deterioration and cardiac arrhythmias (8, 11). Furthermore, a history of CHD can also contribute to increased mortality rates in sepsis patients (12).
When patients with a history of coronary heart disease develop sepsis, it is crucial to establish an accurate prognosis and appropriate treatment plan based on a specialized scoring system. A nomogram is a graphical tool grounded in a statistical prediction model that calculates the probability of a clinical event in a specific patient through multiple indicators (13). However, nomograms for predicting the prognosis of patients with a history of coronary heart disease who have developed sepsis are scarce. In this study, our objective is to develop a nomogram that predicts the outcome for such patients after the onset of sepsis.
The Medical Information Mart for Intensive Care (MIMIC)-III database is a significant healthcare resource for critically ill patients. It was developed and is managed by the Massachusetts Institute of Technology (MIT), established in 2003 (14). Our study employed version 1.4 of the MIMIC-III database. This comprehensive database encompasses data from over 58,000 inpatients in the intensive care unit at Beth Israel Deaconess Medical Center between 2001 and 2012 (15). It provides a wealth of real-world data for clinical research, including but not limited to vital signs, medications, laboratory measurements, care provider observations and notes, fluid balance, procedure codes, diagnostic codes, imaging reports, length of hospital stay, and more. All data can be extracted using Structured Query Language (SQL) for further analysis. Participants in this study completed a series of NIH-provided courses and passed the required assessment (certificate number: 62299628).
This retrospective study utilized data from a third-party anonymous, publicly accessible database (MIMIC-III), and received approval from an existing institutional review board. As the patient information in the database was anonymized, informed consent was not necessary for this study. The report of this study adheres to the STROBE guidelines (16).
The study population was selected based on the Sepsis-3 criteria for diagnosis. Patients diagnosed with sepsis, severe sepsis, and septic shock were extracted from the MIMIC-III database, utilizing the International Classification of Diseases (ICD)-9 code. The exclusion criteria included: (1) patients under the age of 18; (2) patients with an ICU stay of less than 24 h; (3) patients with a SOFA score of less than 2; and (4) patients lacking a prior diagnosis of CHD. For those with multiple ICU admissions, only data from the first ICU admission was extracted. We randomly allocated 70% of the subjects to the training set for this study, reserving the remaining 30% as test data for the validation set. The data extraction process, based on these inclusion criteria, is represented in Figure 1.
Figure 1. Follow chat of study population selection. MIMIC, Medical Information Mart for Intensive Care; ICD, International Classification of Diseases; SOFA, Sequential Organ Failure Assessment; CHD, Coronary Heart Disease.
We utilized SQL to extract the following information from the MIMIC-III database: age, sex, weight, race, admission type, initial care unit, SOFA score, and Acute Physiology Score III (APSIII). Additionally, we obtained data on interventions such as ventilators, continuous Renal Replacement Therapy (CRRT), and vasoactive drugs; complications including congestive heart failure, arrhythmia, valvular heart disease, peripheral vascular disease, renal failure, liver disease, hypertension, diabetes, obesity, and AKI, among others; laboratory test results such as white blood cell count (WBC), neutrophil percentage, red blood cell distribution width(RDW), hematocrit, sodium, potassium, albumin, lactate, and blood pH; and vital signs, including temperature, heart rate, respiratory rate, blood pressure, and SpO2. All this aforementioned data corresponds to the 24 h prior to ICU admission. The primary outcome measure was the patients’ 28-day mortality, which was obtained from the patient hospitalization data in the MIMIC-III database.
Continuous variables that met the normal distribution were represented by mean ± SD values, while non-normally distributed continuous variables were represented by the median and quartiles [M (Q1, Q3)]. Categorical variables were presented in percentages. Stepwise regression and Cox regression were utilized in the selection of variables for the model (17), choosing the method with higher sensitivity. The predictive model was constructed using logistic regression, to estimate the 28-day mortality among septic patients with prior coronary heart disease.
To evaluate the discriminative ability of the model, we employed Harrell’s concordance index (C-index), which measures the model’s prediction accuracy and enables comparison with the existing SOFA indicator (represented by the area under the curve, AUC). The AUC scale extends from 0 to 1, where 1 signifies complete agreement, and 0.5 suggests that the model’s performance is no better than chance. Larger AUC values denote more accurate prognostic stratification. Calibration curves were constructed using bootstrapping with 500 resamplings to assess the agreement between the predicted survival probability by the model and the observed adverse outcomes, thereby validating the clinical applicability of the model based on decision curve analysis (DCA).
All statistical analyses were conducted using the R software version 4.2.2 (R Foundation for Statistical Computing, Vienna, Austria). All tests were two-sided, and a p-value less than 0.05 was considered statistically significant.
A total of 4,777 patients were identified through the screening criteria based on the ICD codes. After applying the exclusion criteria, 800 patients were ultimately included in the final dataset. These patients were randomly assigned to Training cohorts (n = 560) and Validation cohorts (n = 240). Patient baseline characteristics are presented in Table 1. The Training cohort consisted of 349 males (62.3%) and 211 females (37.7%), with a median age of 70 years (IQR = 56–81 years). The Validation cohort included 158 males (65.8%) and 82 females (34.2%), with a median age of 68 years (IQR = 57–80 years). The majority of patients in both cohorts were white(>70%), and most admissions were emergencies (>95%). The most common initial care unit was the MICU (68.4% vs. 67.9%). Over 60% of the patients had health insurance. The median body temperature for both groups was 37.0°C (IQR = 36.0–37.0°C), and the median heart rate was 92 bpm (IQR = 79–104.25 bpm) and 91 bpm (IQR = 80–104 bpm). The median respiratory rate was 21 bpm (IQR = 17.75–24 bpm vs. 18–24 bpm). The median systolic blood pressure was 107 mmHg (IQR = 101–116 vs. 100–116 mmHg). Both groups had median diastolic blood pressures of 56 mmHg (IQR = 50–63 and 50–61.25 mmHg). The median SOFA score was 7.0 in both groups (IQR = 4–10 vs. 4–9). Median APSIII scores were 60 (IQR = 46–75) and 58.5(IQR = 41.75–77). In these patients, the majority had a pH less than 7.35 (45.7% vs. 45.4%). Over 60% of patients had lactate levels ranging between 0.5 and 1.6 mmol/L. Abnormalities in WBC count were observed in 71.3 and 67.1% of patients. RDW was abnormal in 69.3 and 64.6% of the Training and Validation cohorts, respectively. Of these patients, 57 and 51.2% received mechanical ventilation, 21 and 20% used vasopressors, and 12 and 8.6% underwent CRRT. A majority of patients had comorbidities such as congestive heart failure (64 and 59.6%), hypertension (68 and 70.4%), diabetes mellitus (33 and 30.4%), and renal failure (40 and 44.2%). Over 80% of the patients in both groups developed AKI. There were no statistical difference in basic characteristics in two groups except PaCO2 (p = 0.022). Missing data values obtained for all 800 patients were below 20%. If the missing data followed a normal distribution, the mean was used to fill in the missing data. If it did not, the median was used. The all-cause mortality rate in all patients was 66.8% (n = 535). The mortality rates in the Training and Validation cohorts were 65.9% (n = 369) and 69.2% (n = 166), respectively.
Based on the Cox regression and the stepwise regression method, the following variables were included in the preliminary model: age (hazard ratio [HR]: 1.01, 95% confidence interval [CI]: 1–1.02, p = 0.049); diastolic blood pressure (DBP; HR: 0.97, CI: 0.94–0.992, p = 0.034); pH values (pH < 7.35, HR: 1.4, CI 1.05–1.88, p = 0.022, pH > 7.45: HR: 1.55, CI: 1.05–2.29, p = 0.049); lactic acid (HR: 1.45, CI: 1.11–1.91, p = 0.007); RDW (HR: 1.51, CI: 1.13–2.01, p = 0.005); anion gap (HR: 4.15, CI: 1.36–11.09, p < 0.001); valvular heart disease (HR: 1.54, CI: 1.12–2.1, p = 0.007); peripheral vascular disease (HR: 1.65, CI: 1.22–2.23, p = 0.001); and AKI stage (stage I: HR: 1.34, CI: 0.81–2.22, p = 0.256, stage II: HR: 1.49, CI: 0.91–2.43, p = 0.11, stage III: HR: 2.88, CI: 1.78–4.67, p < 0.001). A nomogram was established using these nine selected variables. The nomogram functions by assigning a score to each variable on a corresponding scale. The scores of all variables are then summed to obtain a total score, which is used to estimate the probability of sepsis occurrence by drawing a vertical line on the main axis (Figure 2). HRs for other indicators, 95% CI, and statistical measures are presented in Table 2.
Figure 2. Nomogram for predicting 28-day survival. Left column shows the points bar (top) and nine parameters, each to be scored with a vertical line to the points bar, according to the different parameter values. The sum of the points is calculated (total points range, 0–400), and a vertical line is drawn from the total points bar to the 28-days survival probability below, to obtain survival probability of the patient. DBP, Diastolic blood pressure; Lac, Lactate; RDW, Red blood cell volume distribution width; AG, Anion gap; VHD, Valvular heart disease; PVD, Peripheral vascular disease; AKI, Acute kidney injury.
We utilized C-index, AUC, NRI, and IDI metrics to evaluate the nomogram’s performance. The C-index for the nomogram of the Training cohort was higher than that of the SOFA score (0.667 vs. 0.661). Similarly, the C-index of the Validation cohort’s nomogram also surpassed the SOFA score (0.661 vs. 0.659). These findings were further confirmed by the AUC plots. The AUC for the Training and Validation cohorts were 0.719 (SOFA: 0.679) and 0.724 (SOFA: 0.684; Figure 3), respectively. The median NRI value was 0.159 (95% CI: 0.048–0.364) in the Training cohort and 0.269 (95% CI, 0.048–0.613) in the Validation cohort. The IDI was 0.121 (p < 0.001) for the Training cohort, and 0.103 (p < 0.001) for the Validation cohort (Figure 4).
Figure 3. Receiver operating characteristic (ROC) curves for the nomogram and SOFA model, showing AUCs for: 28-days survival. (A) Training cohort; (B) Validation cohort; SOFA, Sequential Organ Failure Assessment; AUC, area under the curve.
Figure 4. Calibration curves for 28-days survival. The abscissa (x-axis) is the predicted survival rate and the ordinate (y-axis) is the actual survival rate. The red dotted line is the reference line (predicted value equals the actual value), the solid black line is the curve fitting line, and the error bars represent 95% confidence intervals. The calibration curves depict the agreement between predicted probabilities and observed outcomes. (A) Training cohort; (B) Validation cohort.
We incorporated variables such as age, DBP, pH, lactate levels, RDW, anion gap, valvular heart disease, peripheral vascular disease, and AKI stage into a preliminary model, leading to the establishment of a nomogram for predicting the prognosis of sepsis patients. The congruence between the calibration curve and the standard curve in the training and validation cohorts’ calibration plots indicates that the 28-day survival prediction aligns with the observed outcomes (Figure 4).
Figure 5. Decision-curve analysis of the nomogram for 28-days survival. In all figures the black line is above the red line, showing that the area under the curve is larger for the new nomogram model than for the SOFA model. (A) Training cohort; (B) Validation cohort; SOFA, Sequential Organ Failure Assessment; Black dotted line, nomogram model; Red dotted line, SOFA model.
The above results show that our clinical precautionary model is superior to the SOFA score in terms of sensitivity and specificity. In order to verify whether this clinical pre-curative model will be beneficial in clinical practice, we used a DCA curve. In both the Training and Validation cohorts, the nomogram (black line) is above the SOFA score (red line). The net benefit of the nomogram outperformed the SOFA score at any given predicted probability (Figure 5), suggesting that the nomogram plays a significant role in predicting 28-day mortality.
A search of the MIMIC-III database identified 4,777 patients who met the diagnostic criteria for sepsis, of which 800 ultimately met the inclusion criteria. Based on the data from these 800 patients, we found that age, DBP, pH, lactic acid levels, RDW, anion gap, valvular heart disease, peripheral vascular disease, and AKI grade were all correlated with the 28-day mortality of septic patients with a history of coronary heart disease. To optimize the 28-day mortality of such patients, we developed a nomogram, which clinical practitioners can use in the future to better analyze the prognosis of patients with a history of coronary heart disease.
Among the 800 patients included in the study, the all-cause mortality rate was 66.9% (n = 535), which is higher than that of sepsis alone (40%) (4). This suggests that septic patients with a history of coronary heart disease have a higher risk of mortality. Moreover, for the majority of patients (n > 70%), several indicators including pH, PaO2, WBC, hemoglobin, RDW, HCT, PT, PTT, albumin, BUN, and creatinine were within abnormal ranges. Over 80% of the patients also had concurrent AKI. A higher number of patients required vasopressor support, and a significant number underwent CRRT.
Age is an independent risk factor for most diseases, and our study aligns with this conclusion. For most patients, the objective of blood pressure management is to maintain it within a suitable range; extremes in either direction can be detrimental. Intriguingly, our study revealed that a moderately elevated DBP is advantageous for the prognosis of septic patients with CHD. A pediatric sepsis study showed that survivors had notably higher DBP than non-survivors, and low DBP could serve as an independent risk factor for 28-day survival following a multivariate factor analysis (18). This could be due to the potential for higher DBP levels to mitigate tissue hypoperfusion and decrease the likelihood of septic shock, providing a fresh perspective for sepsis treatment. Our findings also suggest that RDW can be used as a prognostic factor in septic patients with CHD. Other studies have identified RDW as a predictor of all-cause mortality in sepsis patients (19). Furthermore, an abnormal anion gap was associated with a decreased 28-day mortality. When Xu Sun et al. analyzed data from critically ill surgical patients in the MIMIC-IV database, they observed a link between a high anion gap and a higher 90-day all-cause mortality risk in these patients, with the cumulative survival rate being higher in the low anion gap group (20). Concurrently, patients with valvular heart disease, peripheral vascular disease, AKI, and higher AKI stage had a lower predicted 28-day survival rate.
The SOFA score is a valuable tool for predicting short-term mortality in patients with sepsis (1, 21). However, it cannot determine the prognosis of patients with coronary heart disease, as SOFA scores can vary based on the source of infection (22). We evaluated our nomogram by calculating the C-index and AUC, demonstrating that this model performs better than the SOFA score. The clinical benefit of the nomogram, superior to the SOFA score, was further confirmed by calculating the NRI and IDI values. Through DCA, we verified the clinical utility of this nomogram. Our findings indicated that septic patients with hypertension derived more substantial benefits from the nomogram compared to the SOFA score.
Limitations: Our study does have several limitations. Firstly, it was a retrospective analysis using clinical data extracted from the MIMIC-III database, and it has not been validated using other databases or clinical studies. Secondly, the sample size was relatively small, which may have resulted in the exclusion of some potentially significant indicators. Lastly, although the C-index was greater than 0.5, it was less than 0.7 (0.667 and 0.661), indicating room for improvement. These findings guide the direction for future research.
We developed a nomogram that utilizes age, DBP, pH, lactic acid levels, RDW, anion gap, valvular heart disease, peripheral vascular disease, and AKI grade as indicators to predict the 28-day mortality in septic patients with a history of CHD. This clinical prognosis model is more applicable than the SOFA score for the Sepsis population with CHD.
The raw data supporting the conclusions of this article will be made available by the authors, without undue reservation.
Ethical approval was not required for the studies involving humans because considering that this study was based on the analysis of an anonymous third-party public database with prior approval from the Institutional Review Board, no ethical review was required. The studies were conducted in accordance with the local legislation and institutional requirements. Written informed consent for participation was not required from the participants or the participants’ legal guardians/next of kin in accordance with the national legislation and institutional requirements because please insert the reason why written informed consent was not required.
QG: Conceptualization, Data curation, Formal analysis, Investigation, Methodology, Project administration, Software, Writing – original draft, Writing – review & editing. PH: Formal analysis, Methodology, Software, Writing – review & editing. QY: Formal analysis, Software, Writing – review & editing. XM: Supervision, Validation, Writing – review & editing. MZ: Funding acquisition, Project administration, Resources, Supervision, Validation, Writing – review & editing.
The author(s) declare that financial support was received for the research, authorship, and/or publication of this article. This research was supported by Heilongjiang Province Key R&D Program (GA21C011) and the National Natural Scientific Foundation of China (82172164) and Heilongjiang Province Key R&D Program (JD22C005).
We would like to thank the participants, developers and investigators associated with the Medical Information Mart for Intensive Care (MIMIC)-III database.
The authors declare that the research was conducted in the absence of any commercial or financial relationships that could be construed as a potential conflict of interest.
All claims expressed in this article are solely those of the authors and do not necessarily represent those of their affiliated organizations, or those of the publisher, the editors and the reviewers. Any product that may be evaluated in this article, or claim that may be made by its manufacturer, is not guaranteed or endorsed by the publisher.
1. Singer, M, Deutschman, CS, Seymour, CW, Shankar-Hari, M, Annane, D, Bauer, M, et al. The third international consensus definitions for Sepsis and septic shock (Sepsis-3). JAMA. (2016) 315:801–10. doi: 10.1001/jama.2016.0287
2. Rudd, KE, Johnson, SC, Agesa, KM, Shackelford, KA, Tsoi, D, Kievlan, DR, et al. Global, regional, and national sepsis incidence and mortality, 1990-2017: analysis for the global burden of disease study. Lancet. (2020) 395:200–11. doi: 10.1016/S0140-6736(19)32989-7
3. Machado, FR, Cavalcanti, AB, Bozza, FA, Ferreira, EM, Angotti Carrara, FS, Sousa, JL, et al. The epidemiology of sepsis in Brazilian intensive care units (the Sepsis PREvalence assessment database, SPREAD): an observational study. Lancet Infect Dis. (2017) 17:1180–9. doi: 10.1016/S1473-3099(17)30322-5
4. Vincent, JL, Sakr, Y, Singer, M, Martin-Loeches, I, Machado, FR, Marshall, JC, et al. Prevalence and outcomes of infection among patients in intensive care units in 2017. JAMA. (2020) 323:1478–87. doi: 10.1001/jama.2020.2717
5. Cohen, J, Vincent, JL, Adhikari, NK, Machado, FR, Angus, DC, Calandra, T, et al. Sepsis: a roadmap for future research. Lancet Infect Dis. (2015) 15:581–614. doi: 10.1016/S1473-3099(15)70112-X
6. Marshall, JC. Why have clinical trials in sepsis failed? Trends Mol Med. (2014) 20:195–203. doi: 10.1016/j.molmed.2014.01.007
7. Wang, HE, Moore, JX, Donnelly, JP, Levitan, EB, and Safford, MM. Risk of acute coronary heart disease after Sepsis hospitalization in the REasons for geographic and racial differences in stroke (REGARDS) cohort. Clin Infect Dis. (2017) 65:29–36. doi: 10.1093/cid/cix248
8. Corrales-Medina, VF, Madjid, M, and Musher, DM. Role of acute infection in triggering acute coronary syndromes. Lancet Infect Dis. (2010) 10:83–92. doi: 10.1016/S1473-3099(09)70331-7
9. Gotts, JE, and Matthay, MA. Sepsis: pathophysiology and clinical management. BMJ. (2016) 353:1585. doi: 10.1136/bmj.i1585
10. Miranda, M, Balarini, M, Caixeta, D, and Bouskela, E. Microcirculatory dysfunction in sepsis: pathophysiology, clinical monitoring, and potential therapies. Am J Physiol Heart Circ Physiol. (2016) 311:H24–35. doi: 10.1152/ajpheart.00034.2016
11. Corrales-Medina, VF, Musher, DM, Shachkina, S, and Chirinos, JA. Acute pneumonia and the cardiovascular system. Lancet. (2013) 381:496–505. doi: 10.1016/S0140-6736(12)61266-5
12. Hotchkiss, RS, and Karl, IE. The pathophysiology and treatment of sepsis. N Engl J Med. (2003) 348:138–50. doi: 10.1056/NEJMra021333
13. Guo, CG, Zhao, DB, Liu, Q, Zhou, ZX, Zhao, P, Wang, GQ, et al. A nomogram to predict lymph node metastasis in patients with early gastric cancer. Oncotarget. (2017) 8:12203–10. doi: 10.18632/oncotarget.14660
14. Yang, J, Li, Y, Liu, Q, Li, L, Feng, A, Wang, T, et al. Brief introduction of medical database and data mining technology in big data era. J Evid Based Med. (2020) 13:57–69. doi: 10.1111/jebm.12373
15. Johnson, AE, Pollard, TJ, Shen, L, Lehman, LW, Feng, M, Ghassemi, M, et al. MIMIC-III, a freely accessible critical care database. Sci Data. (2016) 3:160035. doi: 10.1038/sdata.2016.35
16. von Elm, E, Altman, DG, Egger, M, Pocock, SJ, Gotzsche, PC, Vandenbroucke, JP, et al. The strengthening the reporting of observational studies in epidemiology (STROBE) statement: guidelines for reporting observational studies. Ann Intern Med. (2007) 147:573–7. doi: 10.7326/0003-4819-147-8-200710160-00010
17. Koletsi, D, and Pandis, N. Survival analysis, part 3: cox regression. Am J Orthod Dentofacial Orthop. (2017) 152:722–3. doi: 10.1016/j.ajodo.2017.07.009
18. Lee, EP, Yen, CW, Hsieh, MS, Lin, JJ, Chan, OW, Su, YT, et al. Diastolic blood pressure impact on pediatric refractory septic shock outcomes. Pediatr Neonatol. (2023) 65:222–8. doi: 10.1016/j.pedneo.2023.02.010
19. Wu, H, Liao, B, Cao, T, Ji, T, Huang, J, and Ma, K. Diagnostic value of RDW for the prediction of mortality in adult sepsis patients: a systematic review and meta-analysis. Front Immunol. (2022) 13:997853. doi: 10.3389/fimmu.2022.997853
20. Sun, X, Lu, J, Weng, W, and Yan, Q. Association between anion gap and all-cause mortality of critically ill surgical patients: a retrospective cohort study. BMC Surg. (2023) 23:226. doi: 10.1186/s12893-023-02137-w
21. Seymour, CW, Liu, VX, Iwashyna, TJ, Brunkhorst, FM, Rea, TD, Scherag, A, et al. Assessment of clinical criteria for Sepsis: for the third international consensus definitions for Sepsis and septic shock (Sepsis-3). JAMA. (2016) 315:762–74. doi: 10.1001/jama.2016.0288
22. Pawar, RD, Shih, JA, Balaji, L, Grossestreuer, AV, Patel, PV, Hansen, CK, et al. Variation in SOFA (sequential organ failure assessment) score performance in different infectious states. J Intensive Care Med. (2021) 36:1217–22. doi: 10.1177/0885066620944879
Keywords: 28-day mortality, coronary heart disease, MIMIC-III, nomogram, sepsis
Citation: Gu Q, Huang P, Yang Q, Meng X and Zhao M (2024) A nomogram to predict 28-day mortality in patients with sepsis combined coronary artery disease: retrospective study based on the MIMIC-III database. Front. Med. 11:1433809. doi: 10.3389/fmed.2024.1433809
Received: 16 May 2024; Accepted: 26 August 2024;
Published: 04 September 2024.
Edited by:
Marcos Ferreira Minicucci, São Paulo State University, BrazilReviewed by:
Yanfei Shen, Zhejiang Hospital, ChinaCopyright © 2024 Gu, Huang, Yang, Meng and Zhao. This is an open-access article distributed under the terms of the Creative Commons Attribution License (CC BY). The use, distribution or reproduction in other forums is permitted, provided the original author(s) and the copyright owner(s) are credited and that the original publication in this journal is cited, in accordance with accepted academic practice. No use, distribution or reproduction is permitted which does not comply with these terms.
*Correspondence: Xianglin Meng, bWVuZ3ppOThAMTYzLmNvbQ==; Mingyan Zhao, bWluZ3lhbjE5N0BnbWFpbC5jb20=
Disclaimer: All claims expressed in this article are solely those of the authors and do not necessarily represent those of their affiliated organizations, or those of the publisher, the editors and the reviewers. Any product that may be evaluated in this article or claim that may be made by its manufacturer is not guaranteed or endorsed by the publisher.
Research integrity at Frontiers
Learn more about the work of our research integrity team to safeguard the quality of each article we publish.