- 1Shanghai Seventh People’s Hospital Affiliated to Shanghai University of Traditional Chinese Medicine, Shanghai, China
- 2The first affiliated hospital of Anhui University of Traditional Chinese Medicine, Anhui, China
Background: Robot-assisted hand function therapy is pivotal in the rehabilitation of patients with stroke; however, its therapeutic mechanism remains elusive. Currently, research examining the impact of robot-assisted hand function therapy on brain function in patients with stroke is scarce, and there is a lack of studies investigating the correlation between muscle activity and alterations in brain function.
Objective: This study aimed to investigate the correlation between forearm muscle movement and brain functional activation by employing the synchronized use of functional near-infrared spectroscopy and surface electromyography methods. Moreover, it sought to compare neural activity patterns during different rehabilitation tasks and refine the mechanism of robot-assisted hand function therapy for post-stroke hand function impairments.
Methods: Stroke patients with hand dysfunction underwent three sessions of robot-assisted hand function therapy within 2 weeks to 3 months of onset. The fNIRS-sEMG synchronous technique was used to observe brain function and forearm muscle activation. Ten participants were randomly assigned to receive mirror, resistance, or passive rehabilitation training. During the intervention, cortical and muscle activation information was obtained using fNIRS and electromyographic signals. The primary outcomes included changes in oxyhemoglobin concentration and root mean square of surface electromyography.
Results: Compared to the resting state, the Oxy-Hb concentration in the brain regions involved in three rehabilitation tasks with robot-assisted hand function therapy significantly increased (p < 0.05). Mirror therapy significantly enhanced the prefrontal cortex and the superior frontal cortex activation levels. In contrast, resistance therapy significantly promoted the activation of the supplementary motor area and the premotor cortex. Passive rehabilitation tasks showed some activation in the target brain area premotor cortex region. Robot-assisted hand function therapy has shown that forearm muscle movement is closely related to oxygenated hemoglobin concentration activity in specific brain regions during different rehabilitation tasks.
Conclusion: The simultaneous sEMG-fNIRS study found a significant correlation between muscle movement and brain activity after stroke, which provides an important basis for understanding the treatment mechanism of hand function impairment.
Introduction
Stroke, a prevalent neurological disease (1), is witnessing a global increase in incidence and disability rates (2). Among its complications, hand dysfunction stands out, with 38% of patients experiencing significant impairment after 3 months and approximately 30 to 66% failing to recover completely after 6 months (3, 4). This severe hand dysfunction significantly affects patients’ quality of life and their ability to perform daily activities (5).
Robot-assisted hand function therapy is crucial in restoring hand function post-stroke (6, 7). This therapy allows for personalized treatment plans based on individual patient needs, offering enhanced precision and control (8). Robot-assisted hand function therapy has effectively improved hand function in patients with impaired hand function (9).
Although robot-assisted therapy has been widely studied and applied in clinical Settings in the past few decades (10, 11), there are relatively few studies on the mechanism of robot-assisted hand function therapy on brain function and arm muscle activity (12). In this study, near infrared spectroscopy (fNIRS) and surface electromyography (sEMG) were used to investigate the potential mechanism of robot-assisted hand function therapy on brain function changes. The main objective was to study the correlation between brain function and muscle activity in patients with hand dysfunction. Secondly, the effect of robot-assisted hand function therapy on the activation level of cerebral cortex and the activation degree of arm muscle was studied. This study provides real-time and sensitive neural evaluation indicators for robot-assisted hand function treatment, which helps to better understand the differences in cortical activation under different rehabilitation modes, and formulate more reasonable and effective strategies for personalized rehabilitation of hand dysfunction.
Methods
Design overview
Study design
This study was a single-blinded, randomized, controlled clinical trial with a 3-day intervention and a 1-day washout period. Ten patients diagnosed with post-stroke hand dysfunction, recruited from the Rehabilitation Center of Shanghai Seventh People’s Hospital, were randomly allocated into Group A (mirror image rehabilitation), Group B (resistance rehabilitation), and Group C (passive rehabilitation). A schematic representation of the whole study is depicted in Figure 1. The study protocol was approved by the Ethics Committee of Shanghai Seventh People’s Hospital (2023-7th-HIRB-028) and registered by the Chinese Clinical Trial Center ChiCTR 2200063150.
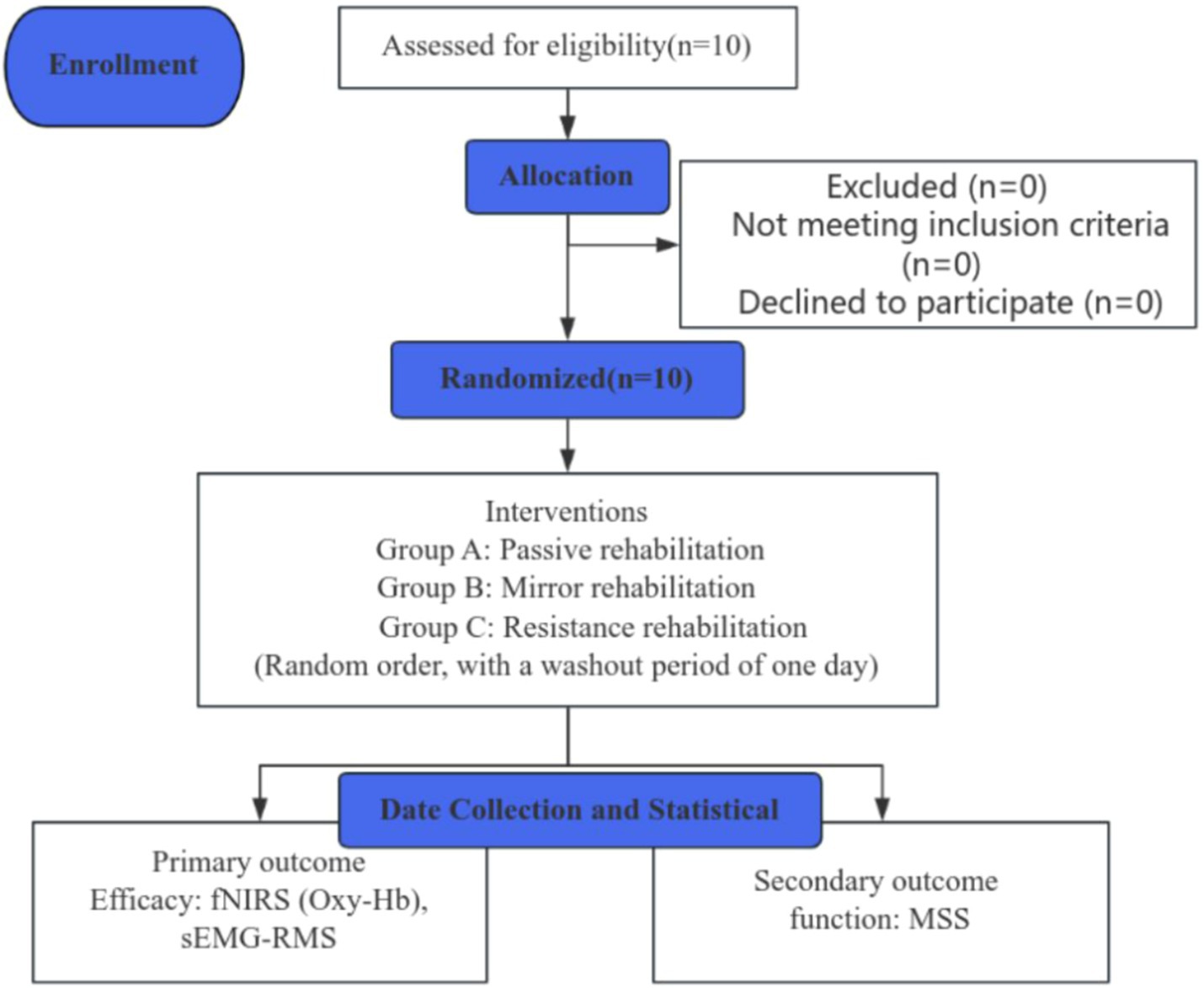
Figure 1. A concise flowchart depicting the entire study process. fNIRS, near-infrared spectroscopy; sEMG, surface electromyography; MSS, Motor Status Scale; RMS, root mean square; Oxy-Hb, oxyhemoglobin.
Participants
Participants who met the diagnostic criteria for stroke-related hand dysfunction outlined by the American Heart Association/American Stroke Association (13) were chosen. Further screening was performed against the inclusion and exclusion criteria.
Inclusion criteria
The inclusion criteria were as follows: (1) confirmed first onset of cerebrovascular disease through brain computed tomographic (CT) or magnetic resonance imaging (MRI) examination; (2) within 2 weeks to 3 months of onset; (3) age between 18 and 75 years; (4) education level of junior high school or higher; (5) Brunnstrom stage hand function above grade 2, indicating unilateral hand motor dysfunction; (6) absence of cognitive impairment, with a mini-mental state examination (MMSE) score of 25 or above; (7) willingness to participate and cooperate with treatment and examination; (8) signed informed consent provided by patients and their families.
Exclusion criteria
The exclusion criteria were as follows: (1) presence of infected or broken skin on the head; (2) poorly controlled epilepsy; (3) severe speech, attention, hearing, visual, intellectual, or mental disorders; cognitive impairment (as assessed by a simple cognitive status checklist: <25 points); (4) participation in other concurrent clinical trials; (5) inability to fully engage in training and evaluation for any reason.
Randomization and masking
Three random numbers were generated using the SPSS 26.0 software and placed into sealed, opaque envelopes. Participants were informed in advance that the training order would be randomized, and they would undergo three kinds of training sequentially, with no option to change the order. Participants meeting the inclusion criteria were randomly selected in the inclusion order and allocated to a group following the label instructions in the envelope.
Due to the particularity of robot-assisted hand function treatment, it is difficult to implement the double-blind method in this study (14), so we adopted the single-blind method. The evaluators were unaware of the grouping assignment of patients and the type of intervention they received.
Interventions
Health education
All patients received comprehensive health education according to the guidelines provided by the American Heart Association/American Stroke Association (15). This accomplishment was facilitated by distributing informative brochures on stroke-related topics to enhance patients’ understanding. The brochures covered various issues, including stroke awareness, risk factors, rehabilitation options, psychological support, and dietary recommendations.
Robot-assisted hand function therapy
The bionic soft hand rehabilitation robot glove (Hunan Sirrem Medical Technology Co., Ltd.) was utilized for robot-assisted hand function therapy. Each rehabilitation session lasted 10 min, with consistent training intensity (duration and strength) for all patients. Ten patients, wearing gloves, sat in a quiet environment. Gloves were worn for all three treatments. The therapy comprises three distinct modalities (Figure 2):
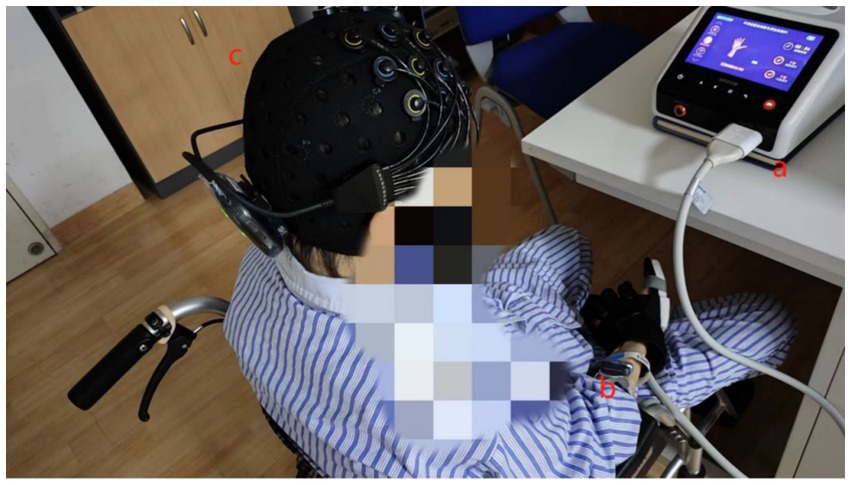
Figure 2. Training task for robot-assisted hand function therapy and data acquisition using fNIRS and sEMG. (a) Patients with robot-assisted hand function therapy gloves. (b) Electrodes placed on the forearm muscles. (c) Participants wearing a near-infrared test cap.
Passive rehabilitation training group (Group A)
This mode involves the soft hand rehabilitation robot guiding the affected hand through grasping and finger movements. The finger movements are driven entirely by the robot, defining this modality as passive rehabilitation training.
Mirror rehabilitation training group (Group B)
Patients in this group observe their unaffected hand performing mirror-image grasping and finger movements displayed on the screen. Subsequently, they imagine their affected hand executing the same movements, aiming to synchronize both sides as closely as possible.
Resistance rehabilitation training group (Group C)
Patients observe finger movements on a screen and replicate them with their affected hand. In contrast, the hand rehabilitation robot applies resistance to finger extension and grasping movements. This mode is characterized as resistance rehabilitation training.
Outcomes
Primary outcome
fNIRS data acquisition
The study utilized a portable near-infrared brain imaging system (Brite MKIII, Artinis, Netherlands) to monitor participants’ hemodynamic changes during rest and rehabilitation tasks (16). Data were synchronously collected and analyzed using the OxySoft software. The device setup included 10 transmitting and eight receiving optodes, arranged in a 2 × 12 channel layout to mirror the distribution characteristics of the brain’s hand motion area, accounting for the arrangement of regions of interest (ROI) from previous studies. This configuration resulted in 24 collection channels symmetrically distributed across the participants’ left and right hemispheres. The distance between the detectors and the light sources was set to 30 mm, emitting wavelengths of 763 nm and 842 nm at a sampling rate of 25 Hz. Participants rested and relaxed for 2 min, followed by a minute of rest with their eyes closed (resting state). Subsequently, they engaged in a 10-min rehabilitation exercise while near-infrared and surface electromyography signals were collected. The task and data collection concluded simultaneously, after which participants were asked to remain still for an additional minute. Event labels such as “A” and “B” denoted the data captured between the start and end of a task. Events A and B signified the commencement and conclusion of synchronized tasks for fNIRS-sEMG, respectively, including the rehabilitation tasks involving the use of a robotic assistive hand glove, which were manually synchronized by the researcher for activation and deactivation.
sEMG data acquisition
The BTS FREEEMG 300 wireless surface electromyography system (BTS Bioengineering, Milan, Italy) was utilized following the Surface Electromyography for the Non-Invasive Assessment of Muscles guidelines (17). This system, renowned for its wireless transmission technology, portability, and features such as high accuracy, long-distance telemetry, and durability is a reliable tool for surface electromyography acquisition and analysis in clinical diagnosis, rehabilitation assessment, and various research applications. The participants’ forearm hair was shaved, and the skin was cleansed with an alcohol swab to minimize skin resistance to the electromyography signal. Wireless surface electrodes were placed on the muscle bellies of the flexor carpi radialis (FCR), flexor digitorum superficialis (FDS), flexor carpi ulnaris (FCU), and abductor pollicis longus (APL) muscles. These electrodes were secured with medical tape and connected wirelessly to the computer, operating at a sampling frequency of 1,000 Hz. The test process is shown in Figure 3.
Secondary outcome
Function
The Motor Status Scale (MSS) test is a crucial assessment tool for evaluating hand function impairment in patients with stroke (18), focusing on hand function and motor control. The MSS scale was divided into shoulder, elbow, wrist, hand and other parts, in which the shoulder and elbow and forearm were graded at 6 levels (0, 1−, 1, 1+, 2−, 2), ranging from involuntary movement to normal movement, and the wrist and hand were graded at 3 levels (0, 1, 2). The shoulder and elbow forearm movement measurement includes 12 shoulder movements and 5 elbow and forearm movements, and also tests the patient’s ability to hold the last position (for 3–5 s) with 5 shoulder movements and 1 elbow movement, with a total score of 40. The wrist and hand measured 3 wrist movements, 15 hand movements, and 3 functional tasks of the hand, with a total score of 42. Higher scores, approaching the total score of 82, indicate better motor function.
Data management
fNIRS data management
The OxySoft software package is designed to process the activation of oxygen concentration during task processes and generate 3D functional cortical activation maps of the brain. Initially, fNIRS data preprocessing is conducted using MATLAB software. The “oxysoft2matlab” plugin from Artinis company converts raw data files (*.oxy5 and *.oxyproj) into the *.nirs format. Subsequently, the Homer2 plugin transforms intensity data into Oxy-Hb. Motion artifacts across all channels are rectified, and any defective channels are identified. A bandpass filter is employed to eliminate noise generated by heartbeat, respiration, and low-frequency signal drifts, with a low frequency of 0.01 Hz and a high frequency of 0.2 Hz. The phase information of the 0.01–0.08 Hz signal is identified using wavelet transform methods. Changes in cortical frequency specificity are assessed by calculating wavelet amplitude, transverse index, and wavelet phase coherence (19). Due to its higher signal-to-noise ratio, Oxy-Hb (20) is selected as the indicator for analyzing oxygen concentration in subsequent analyses. The concentration of Oxy-Hb in relevant brain regions during task processes is calculated, with regions of interest (ROIs) including the prefrontal cortex (PFC), superior frontal cortex (SFC), supplementary motor area (SMA), and premotor cortex (PMC). The change in oxyhemoglobin for each channel is calculated by averaging the oxyhemoglobin across all tasks between events A and B. The average oxyhemoglobin concentration during three rehabilitation tasks with robotic-assisted hand function therapy is obtained. By dividing the sum of oxyhemoglobin concentrations in all channels within each ROI by the number of channels in the ROI, the average oxyhemoglobin concentration for each ROI during task states is determined.
sEMG data management
The acquired raw data undergoes bandpass filtering with a bandwidth of 20–500 Hz. Subsequently, the collected signals are analyzed using the EMGAnalyzer software to calculate the root mean square (RMS) value within the task interval (21). These assessments were conducted by two trained professional therapists, and the entire process was recorded. Subsequently, the average scores from two additional trained professional therapists were analyzed.
Statistics
Statistical analysis was conducted using the SPSS software (Version 26.0, Chicago, IL, United States). Pearson correlation analysis assessed the relationship between the RMS and Oxy-Hb concentrations, and between different rehabilitation tasks and Oxy-Hb concentrations. Multivariate analysis of variance was used to examine whether there were differences in oxygenated hemoglobin concentration and RMS among the three rehabilitation tasks. Multiple postmortem comparisons were performed using LSD testing to identify differences between interventions and brain regions. Wilcoxon was used for data that did not conform to normal distribution. Descriptive statistics were reported as mean ± standard deviation (SD). The significance level for all statistical tests was set at 0.05.
Results
Accounting for all patients
The study enrolled 10 stroke patients with hand dysfunction. Finally, all 10 patients completed three robot-assisted hand function tests over a 5-day trial period, and the results were analyzed. No adverse events were reported during the study.
Demographic characteristics
Ten participants completed the trial without any dropouts. Their mean age was 52.4 ± 6.3 years. There were no significant differences in age, gender, and baseline hand function among the three groups (see Table 1).
Hand function
Following three sessions of robot-assisted hand function therapy, the patient’s MSS scores showed improvement (p < 0.05). Five days later, the baseline MSS score increased from 41.8 ± 23.4 to 46.7 ± 22.4 (see Table 2).
Brain function
As shown in Table 3, multivariate ANOVA showed differences in Oxy-Hb concentrations in the functional cortex of the brain for the three robot-assisted rehabilitation tasks, and between-group comparisons found significant differences in Hb concentrations between Groups A and B. Figure 4 shows significant changes in oxygenated hemoglobin concentration in brain function, with the cerebral cortex in different robot-assisted rehabilitation tasks where closer to red color indicates higher levels of activation. A showed that the activation of each brain region in the resting state was not significant. B showed that the activation level of PMC region in passive treatment task was higher than that in other brain regions. C showed that in the mirror therapy task, brain activation in the healthy hand was higher than that in the affected hand, but PFC and SFC on the affected side were also higher than that in other tasks. Compared to other brain regions, area D showed high levels of PMC and SMA activation during resistance therapy. Figure 5 illustrates that passive processing tasks have a small level of activation of brain regions, but significantly activate M1 and PMC. The mirror therapy task increased the activation levels of PFC and SFC, while the resistance therapy task enhanced the activation levels of SMA and PMC. Figure 6 demonstrates significant differences in Oxy-Hb concentration in the brain’s functional cortex between resting and task states across the three types of robot-assisted rehabilitation tasks (p < 0.05), brain regions in the task state were more activated than those in the resting state. The activation of brain function was more effective in the group with robot-assisted hand function therapy than in the group without robot-assisted hand function therapy.
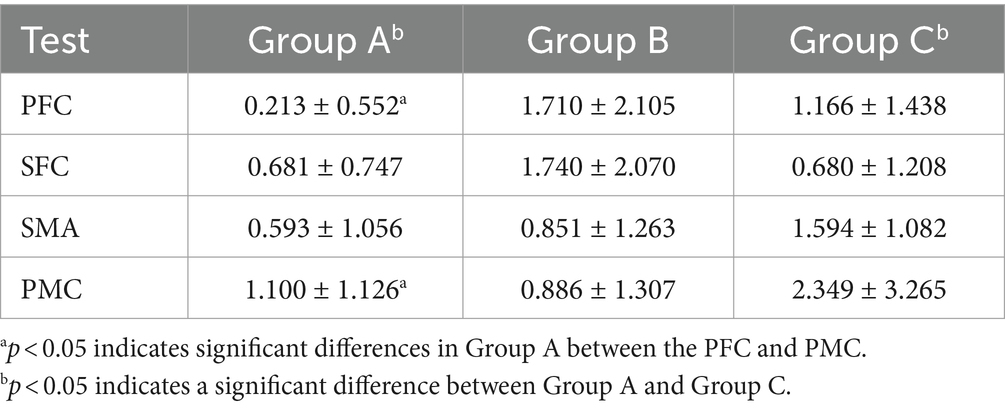
Table 3. Comparative analysis of the impact of various rehabilitation tasks on changes in oxyhemoglobin levels in the functional brain cortex.
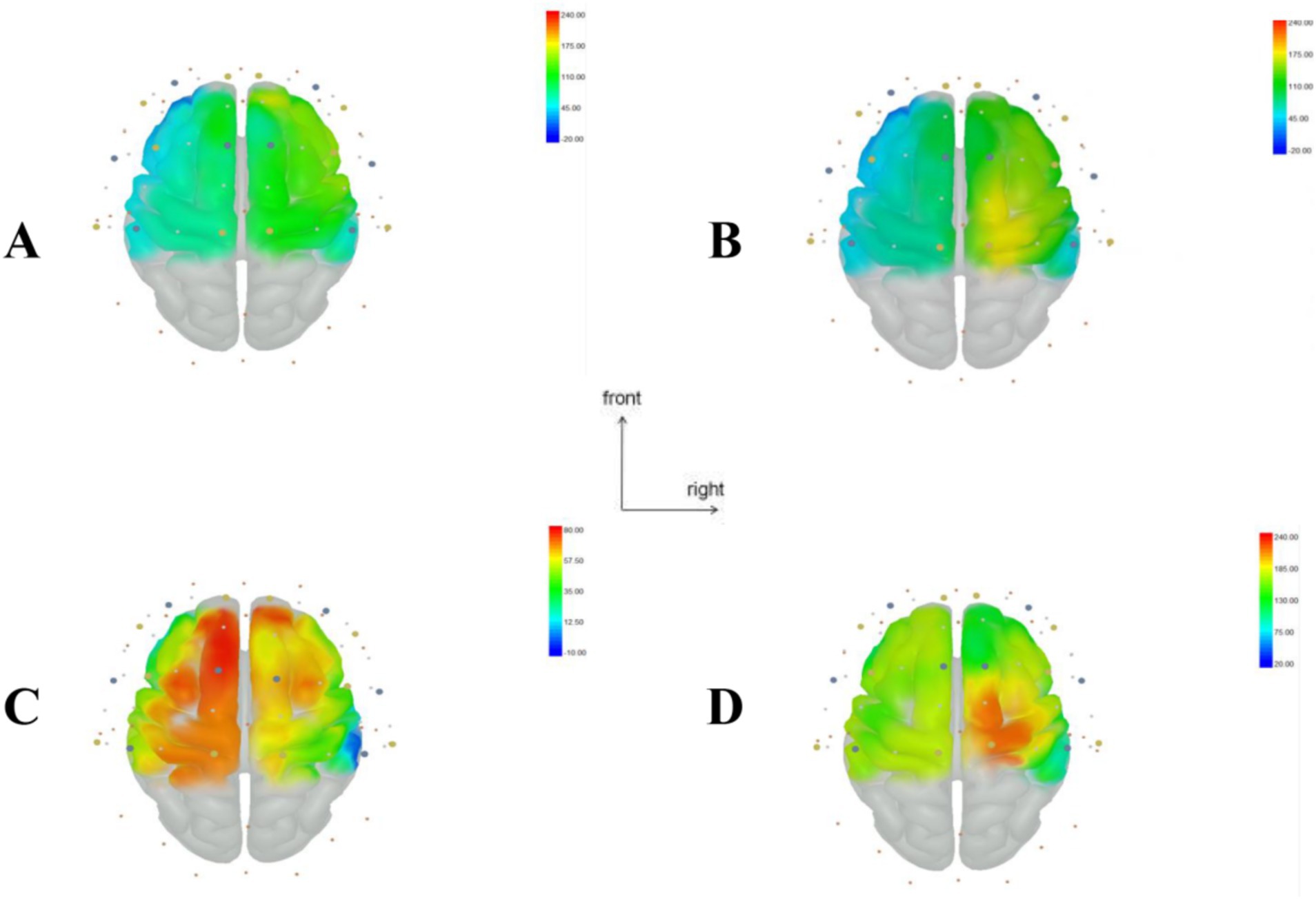
Figure 4. Visualization of regions of interest (ROI) in the brain during resting and task states in Groups A, B, and C. (A) Resting state brain functional area map. (B) Brain functional area activation map during passive rehabilitation task. (C) Brain functional area activation map during mirror task. (D) Brain functional area activation map during resistance task. This figure illustrates the change in oxyhemoglobin values, with larger T values, closer to red, indicating stronger activation in brain regions, while smaller T values closer to blue suggest weaker activation.
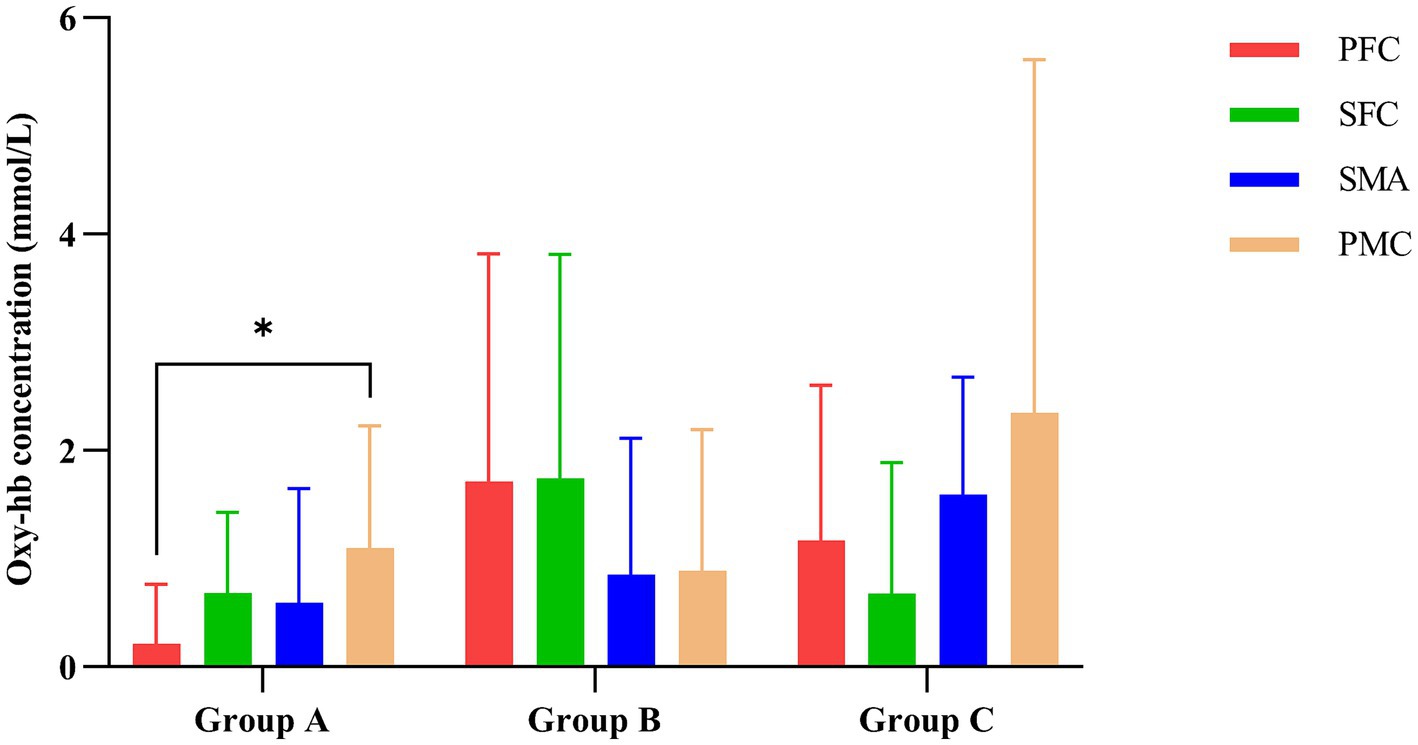
Figure 5. Impact of various rehabilitation tasks on the changes in oxygenated hemoglobin in the brain’s functional cortex. *p < 0.05 indicates significant differences in Group A between the PFC and PMC.
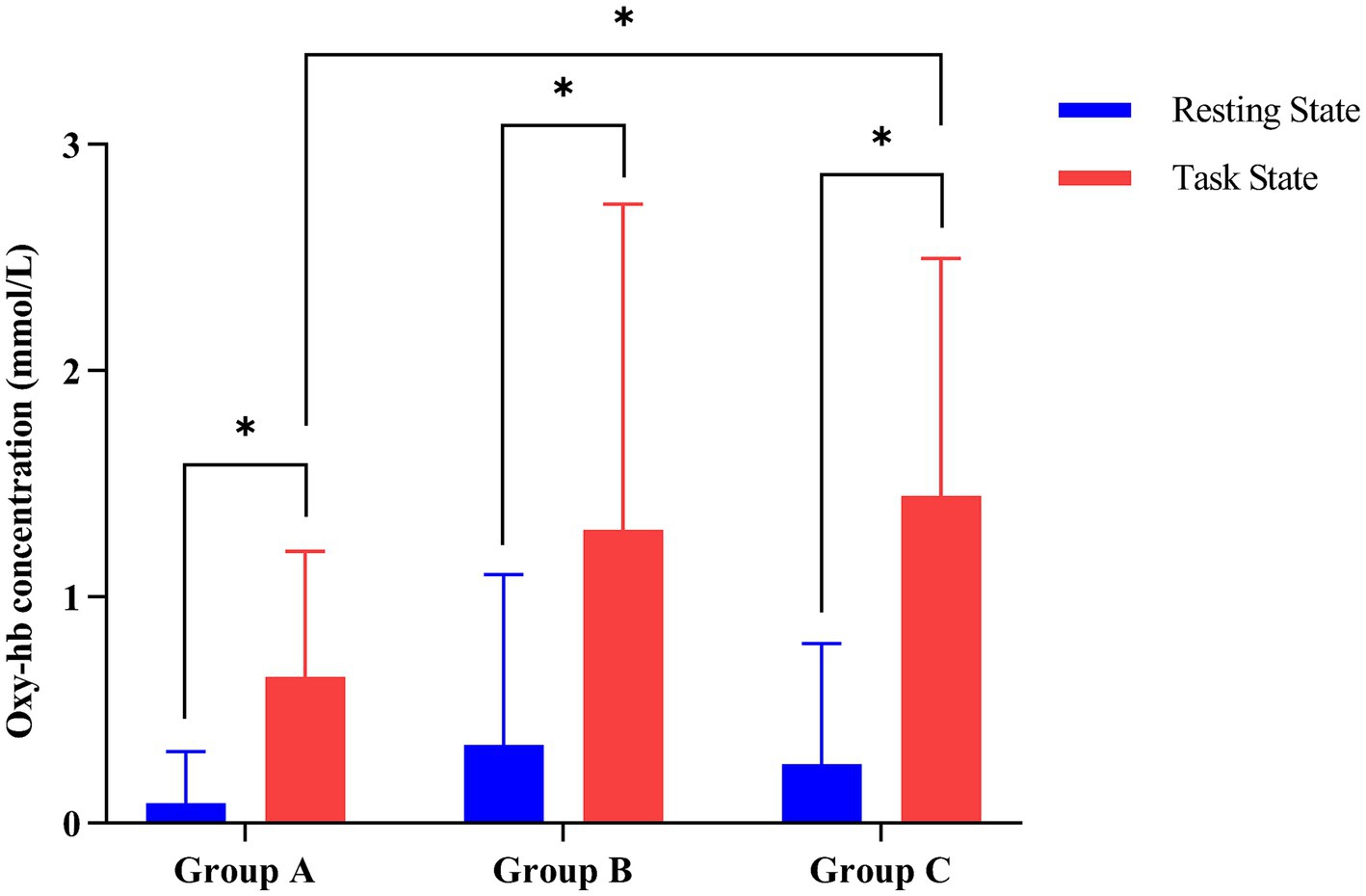
Figure 6. Contrasting changes in oxyhemoglobin levels between task and resting states in the three groups of robot-assisted hand function therapies. (Group A) Passive rehabilitation task. (Group B) Mirror rehabilitation task. (Group C) Resistance rehabilitation task. *p < 0.05 indicated that there was statistically significant difference between task state and resting state oxygenated hemoglobin in the three groups.
Muscle activity
Figure 7 displays the magnitude of change in the RMS of the target arm muscles during rehabilitation tasks assisted by three types of robotic hands. Figure 8 compares the difference in RMS values of arm muscles across the three tasks. Analysis of variance showed that there was no significant difference in RMS of arm muscles among the three rehabilitation tasks (p > 0.05). Multiple comparisons showed that the intensity of target muscle activity in the resistance rehabilitation group was significantly higher than that in the mirror rehabilitation group (p < 0.05). There was no significant difference between the groups.
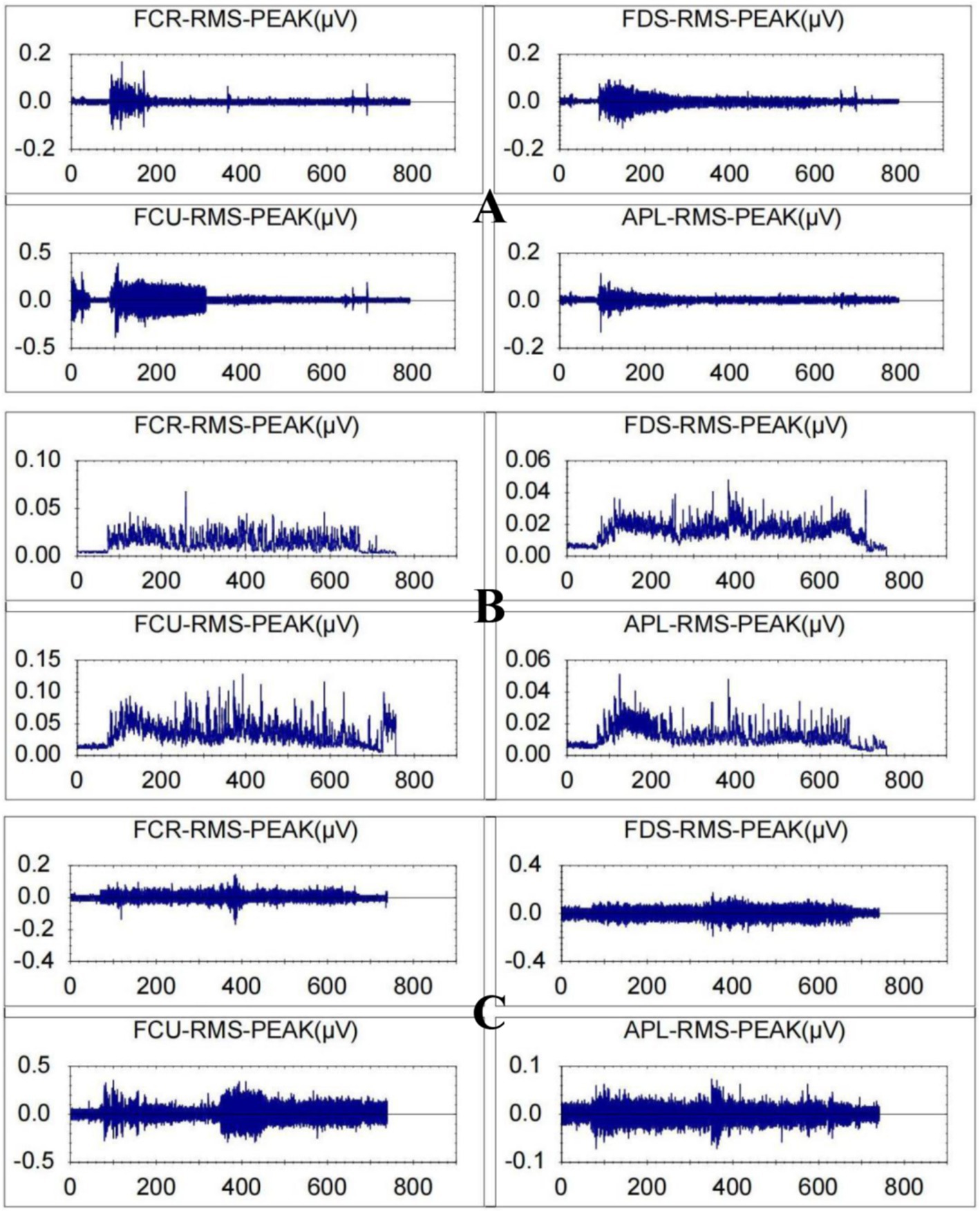
Figure 7. Root mean square values for a patient with stroke-related hand dysfunction during robot-assisted hand function therapy. (A) Passive rehabilitation task. (B) Mirror rehabilitation task. (C) Resistance rehabilitation task; RMS, root mean square; FCR, flexor carpi radialis; FDS, flexor digitorum superficialis, FCU, flexor carpi ulnaris; APL, abductor pollicis longus. The ordinate is in microvolts, and the abscissa is in seconds.
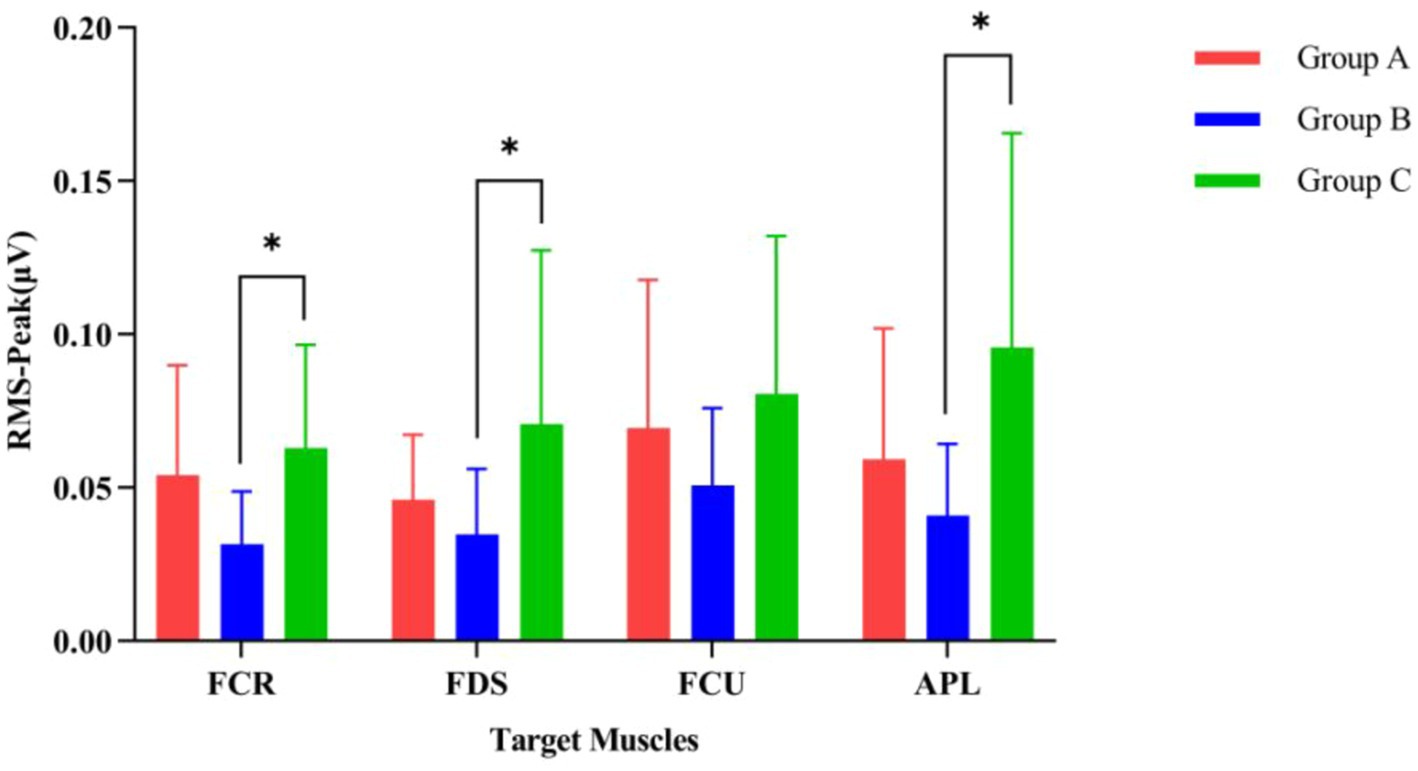
Figure 8. Comparison of the activation levels of arm muscles during three different rehabilitation tasks assisted by robotic gloves. (Group A) Passive rehabilitation task. (Group B) Mirror rehabilitation task. (Group C) Resistance rehabilitation task. RMS, root mean square; FCR, flexor carpi radialis; FDS, flexor digitorum superficialis; FCU, flexor carpi ulnaris; APL, abductor pollicis longus. *p < 0.05 indicates that the arm muscles of the three groups are significantly different under exercise.
Correlation analysis
Pearson correlation analysis revealed the relationship between the activation levels of arm muscles (RMS-peak) and the blood flow activity in brain regions (Oxy-Hb concentration) across three different rehabilitation task conditions involving robot-assisted hand function therapy (Figure 9). In passive rehabilitation tasks, there was a positive correlation between arm muscle activation (RMS) and Oxy-Hb data of cerebral functional cortex ROI, with a correlation coefficient of 0.4659, but the difference was not significant (p > 0.05). In mirror rehabilitation tasks, RMS displayed a significant positive correlation with the Oxy-Hb data of the brain functional cortex ROI, indicating a correlation coefficient of 0.6387 (p < 0.05). Similarly, in resistance rehabilitation tasks, RMS exhibited a significant positive correlation with the Oxy-Hb data of the brain functional cortex ROI, with a correlation coefficient of 0.6982 (p > 0.05).
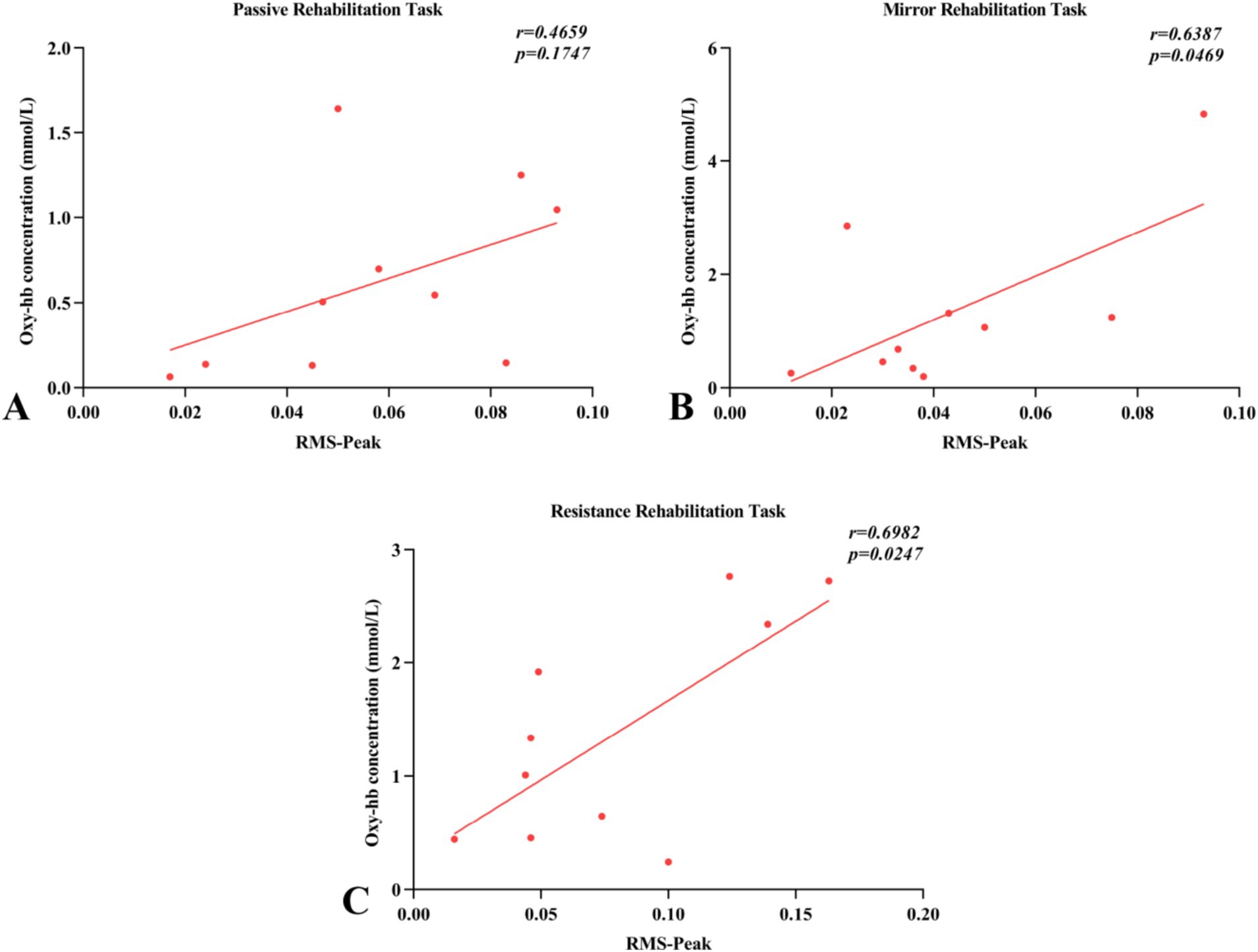
Figure 9. Pearson’s correlations between surface electromyography root mean square (RMS) and regions of interest (ROI) of fNIRS (oxyhemoglobin concentration) for the three rehabilitation tasks. (A–C) Indicated that the changes of blood flow movement in brain functional areas of passive rehabilitation task, mirror rehabilitation task and resistance rehabilitation task were correlated with Pearson of arm EMG-RMS.
Discussion
Innovation
Multimodal data fusion
This study combines fNIRS and sEMG for synchronous monitoring, offering a more comprehensive understanding of motor control and brain function. Unlike traditional research relying on single measurements, this enhances the assessment accuracy through the combination.
Focus on neuroplasticity research
This study provides a new perspective in stroke rehab by investigating neuroplastic changes in patients with robot-assisted therapy. The focus aims to clarify how it facilitates neuronal reorganization for motor function recovery.
Individualized rehabilitation for specific motor tasks
By capturing muscle and brain responses in various robot-assisted tasks, this research supports individualized programs. The tailored strategy meets diverse needs, enhancing rehab efficacy.
Mechanisms of treatment
Motor learning and neuroplasticity
Compared with the resting state, the concentration of Oxy-Hb in the brain region of rehabilitation task is significantly increased, that is, the increase of cerebral blood flow, which is correlated with the increase of brain functional activity. When brain regions are active, their neurons consume more oxygen and require more efficient blood supply to meet oxygen and nutrient requirements. This complex relationship between brain activity and blood flow is called neurovascular coupling (22). Activation of functional brain regions suggests that robot-assisted therapy may promote neuroplasticity (23, 24). The results suggesting that robot-assisted hand therapy significantly increased cerebral blood flow to the region by activating a brain region associated with hand function, thereby promoting brain reorganization and functional recovery. Robot-assisted therapy triggers neural plasticity through standardized and repetitive motor training, stimulating motor neurons. This mechanism of improving neuroplasticity through motor learning and exercises is of great significance for rehabilitation after nerve injury.
Enhanced feedback mechanism
fNIRS and sEMG offer real-time feedback, enabling patients to understand their motor performance and brain activity. Robot-assisted therapy uses precise movement and feedback mechanisms to effectively guide patients to training, activating the motor cortex, sensory cortex (25), and other related neural pathways in the brain. This not only helps to improve the patient’s motor capacity, but may also further accelerate recovery by strengthening neural connections and promoting neuroplasticity. This helps them adjust exercise strategies promptly, improving learning and rehabilitation.
Bidirectional effects on the brain
Robot-assisted hand function boosts hand skills and activates brain regions for movement through specific tasks, promoting the neural basis of motion. Utilizing fNIRS to monitor blood flow changes (oxyhemoglobin concentration) in the brain, our findings indicate that various rehabilitation tasks assisted by robots exert distinct effects on the activation sites and levels of the brain cortex. Specifically, mirror therapy tasks notably enhanced the activation levels in the PFC and SFC, regions closely associated with higher cognitive functions, attention control, and motor planning (26). This association suggests mirror therapy can improve the brain’s attention and movement-planning capacity, stimulating these regions (27, 28). The resistance rehabilitation task significantly promoted the activation of SMA and PMC (29). The SMA is involved in the coordination of complex movements and the integration of sensory feedback (30), while the PMC plays an important role in the preparation and organization of movements (31). In resistance rehabilitation tasks, resistance training directly stimulates brain regions involved in movement execution and control by enhancing the muscle response to resistance, and increased activation of these regions suggests that resistance rehabilitation tasks help improve an individual’s motor skills and overall functional performance in strength training. Patients perform tasks not only physically but also through brain cognition, integrating movement and perception for better recovery.
Improved motor control and coordination
Robot-assisted training enhances motor control and coordination accuracy. Mirror rehabilitation tasks in post-stroke rehab help patients regain forearm and hand control, facilitating overall motor function recovery. While passive rehabilitation tasks had a minimal impact on the activation levels of targeted brain areas, they still exhibited some activation in the PMC region (32). This activation could be attributed to the brain’s processing and response to external movement stimuli during passive movements, particularly in regions implicated in movement planning and anticipation. Therefore, mirror and resistance rehabilitation tasks are more effective at activating brain areas associated with movement, a conclusion consistent with previous research (33).
Relevance
The correlation between the RMS of sEMG, as measured by fNIRS, and the ROI signifies the synchronization or consistency observed in brain activity within a specific ROI with the electrical activity of muscles, as measured by EMG. This correlation reflects the relationship between muscle movement and brain activity.
Mechanism elucidation
Previous studies have demonstrated a significant association between muscle movement and brain activity, which can aid in comprehending the underlying causes of impaired hand function resulting from stroke.
During robotic assistance for hand function rehabilitation post-stroke, a correlation was observed between muscle movement activity in the brain’s cortical areas and Oxy-Hb concentration. Specifically, during muscle movement, the brain regions associated with planning and executing movements become more active (neuromuscular coupling) (34), necessitating increased oxygen and nutrients to support this neural activity. Consequently, blood flow to these areas increases (cerebral blood flow coupling) (35), resulting in Oxy-Hb concentration increase, indicating a close relationship between muscle movement and activity in specific brain regions. Mirror rehabilitation may enhance patients’ perception and imagination of movement (36), further activating brain areas related to movement planning and execution (37), such as the motor cortex, and frontal and parietal lobes (mirror training effect). This involvement in perception and imagination may activate muscle movement, particularly in passive, mirror, and resistance rehabilitation processes tailored to engage the brain’s movement-related areas (38). The sEMG signal, originating from the muscle electrical activity of the α-motor neurons in the spinal cord under brain motor cortex control (39), undergoes characteristic changes (amplitude and frequency) influenced by factors such as varying muscle activity levels and functional states. The cerebral cortex plays a pivotal role in muscle activity control, transmitting motor signals via nerve impulses, thereby eliciting muscle contractions (40, 41).
Clinical application
This comprehension provides a clinical foundation for designing more effective rehabilitation training methods aimed at assisting patients in regaining hand function. The correlation analysis between the fNIRS-sEMG synchronization method in the brain region and the Oxy-Hb concentration revealed that different rehabilitation tasks with robot-assisted hand functional therapy yielded varying effects on forearm muscle movement. Mirror rehabilitation promotes nerve regeneration and muscle coordination (42), resistance rehabilitation enhances muscle strength and endurance (43), and passive rehabilitation aids in muscle relaxation and improves joint mobility (44).
Enhancing effectiveness and speed
Training approaches that combine muscle movement and brain activity may lead to improved treatment outcomes and faster recovery by promoting neuroplasticity –the reestablishment and strengthening of neural connections in the brain. The resistance and passive rehabilitation groups demonstrated improved arm muscle strength in this study, while the mirror rehabilitation group exhibited comparatively modest gains. This observation is attributed to mirror rehabilitation’s incorporation of the healthy side hand to guide grasping movements, resulting in reduced muscle contraction compared to passive and resistance rehabilitation. Utilizing robotic assistance in hand function therapy prolongs arm muscle contractions, thereby improving muscle strength and functional recovery of the affected upper limb. This evidence collectively underscores the efficacy of robotic-assisted hand function therapy in treating post-stroke hand dysfunction (45).
Limitations
This study has some limitations, such as small sample size and short study time. Future research directions include increasing the sample size, extending the study time, and exploring other modes of rehabilitation robot training.
Conclusion
The present study revealed a significant correlation between muscle movement and brain activity after stroke, a finding that provides an important scientific basis for understanding the therapeutic mechanism of hand functional impairment. By combining fNIRS and surface electromyography (EMG), we were able to clarify the interaction between different movements and corresponding brain regions, which plays a key role in the recovery process of patients.
Data availability statement
The raw data supporting the conclusions of this article will be made available by the authors, without undue reservation.
Ethics statement
The studies involving humans were approved by the Ethics Committee of the Shanghai Seventh People’s Hospital (2023-7th-HIRB-028). The studies were conducted in accordance with the local legislation and institutional requirements. The participants provided their written informed consent to participate in this study. Written informed consent was obtained from the individual(s) for the publication of any potentially identifiable images or data included in this article.
Author contributions
CC: Software, Visualization, Writing – original draft. TL: Methodology, Software, Writing – review & editing. BZ: Validation, Writing – review & editing. XW: Project administration, Resources, Writing – review & editing. ZS: Software, Writing – review & editing. ZZ: Investigation, Writing – review & editing. XR: Formal analysis, Writing – review & editing. MZ: Formal analysis, Writing – review & editing. YS: Data curation, Writing – review & editing. JW: Funding acquisition, Project administration, Writing – review & editing.
Funding
The author(s) declare that financial support was received for the research, authorship, and/or publication of this article. This study is supported by grants through Discipline Leader Training Program of Pudong New Area Health Committee (PWRd2020-07), the Pudong New Area’s “National Traditional Chinese Medicine Development Comprehensive Reform Pilot Zone” Construction project (PDZY-2023-0701) and “2023 Excellent Doctoral Student Training Program in Key Fields” of Shanghai University of Traditional Chinese Medicine (GJ2023020).
Acknowledgments
The authors thank Bullet Edits Limited for the linguistic editing and proofreading of the manuscript.
Conflict of interest
The authors declare that the research was conducted in the absence of any commercial or financial relationships that could be construed as a potential conflict of interest.
Publisher’s note
All claims expressed in this article are solely those of the authors and do not necessarily represent those of their affiliated organizations, or those of the publisher, the editors and the reviewers. Any product that may be evaluated in this article, or claim that may be made by its manufacturer, is not guaranteed or endorsed by the publisher.
References
1. Ferrara, G, Benzi, A, Sturla, L, Marubbi, D, Frumento, D, Spinelli, S, et al. Sirt6 inhibition delays the onset of experimental autoimmune encephalomyelitis by reducing dendritic cell migration. J Neuroinflammation. (2020) 17:228. doi: 10.1186/s12974-020-01906-1
2. GBD 2021 Nervous System Disorders Collaborators. Global, regional, and national burden of disorders affecting the nervous system, 1990–2021: a systematic analysis for the Global Burden of Disease Study 2021. Lancet Neurol. (2024) 23:344–81. doi: 10.1016/S1474-4422(24)00038-3
3. Tu, WJ, and Wang, LD. China stroke surveillance report 2021. Mil Med Res. (2023) 10:33. doi: 10.1186/s40779-023-00463-x
4. Martin, SS, Aday, AW, Almarzooq, ZI, Anderson, CAM, Arora, P, Avery, CL, et al. 2024 heart disease and stroke statistics: a report of US and global data from the American Heart Association. Circulation. (2024) 149:e347–913. doi: 10.1161/CIR.0000000000001209
5. GBD 2019 Stroke Collaborators. Global, regional, and national burden of stroke and its risk factors, 1990–2019: a systematic analysis for the Global Burden of Disease Study 2019. Lancet Neurol. (2021) 20:795–820. doi: 10.1016/S1474-4422(21)00252-0
6. Zheng, QX, Ge, L, Wang, CC, Ma, QS, Liao, YT, Huang, PP, et al. Robot-assisted therapy for balance function rehabilitation after stroke: a systematic review and meta-analysis. Int J Nurs Stud. (2019) 95:7–18. doi: 10.1016/j.ijnurstu.2019.03.015
7. Morone, G, Cocchi, I, Paolucci, S, and Iosa, M. Robot-assisted therapy for arm recovery for stroke patients: state of the art and clinical implication. Expert Rev Med Devices. (2020) 17:223–33. doi: 10.1080/17434440.2020.1733408
8. Devittori, G, Dinacci, D, Romiti, D, Califfi, A, Petrillo, C, Rossi, P, et al. Unsupervised robot-assisted rehabilitation after stroke: feasibility, effect on therapy dose, and user experience. J Neuroeng Rehabil. (2024) 21:52. doi: 10.1186/s12984-024-01347-4
9. Zhang, L, Jia, G, Ma, J, Wang, S, and Cheng, L. Short and long-term effects of robot-assisted therapy on upper limb motor function and activity of daily living in patients post-stroke: a meta-analysis of randomized controlled trials. J Neuroeng Rehabil. (2022) 19:76. doi: 10.1186/s12984-022-01058-8
10. Rodgers, H, Bosomworth, H, Krebs, HI, van Wijck, F, Howel, D, Wilson, N, et al. Robot-assisted training compared with an enhanced upper limb therapy programme and with usual care for upper limb functional limitation after stroke: the RATULS three-group RCT. Health Technol Assess. (2020) 24:1–232. doi: 10.3310/hta24540
11. Sehle, A, Stuerner, J, Hassa, T, Spiteri, S, Schoenfeld, MA, and Liepert, J. Behavioral and neurophysiological effects of an intensified robot-assisted therapy in subacute stroke: a case control study. J Neuroeng Rehabil. (2021) 18:6. doi: 10.1186/s12984-020-00792-1
12. Balch, MHH, Harris, H, Chugh, D, Gnyawali, S, Rink, C, Nimjee, SM, et al. Ischemic stroke-induced polyaxonal innervation at the neuromuscular junction is attenuated by robot-assisted mechanical therapy. Exp Neurol. (2021) 343:113767. doi: 10.1016/j.expneurol.2021.113767
13. Tsao, CW, Aday, AW, Almarzooq, ZI, Anderson, CAM, Arora, P, Avery, CL, et al. Heart disease and stroke Statistics-2023 update: a report from the American Heart Association. Circulation. (2023) 147:e93–e621. doi: 10.1161/CIR.0000000000001137
14. Bhattacharjee, S, Barman, A, Patel, S, and Sahoo, J. The combined effect of robot-assisted therapy and activities of daily living training on upper limb recovery in persons with subacute stroke: a randomized controlled trial. Arch Phys Med Rehabil. (2024) 105:1041–9. doi: 10.1016/j.apmr.2024.01.027
15. Hoh, BL, Ko, NU, Amin-Hanjani, S, Chou, SHY, Cruz-Flores, S, Dangayach, NS, et al. 2023 guideline for the management of patients with aneurysmal subarachnoid hemorrhage: a guideline from the American Heart Association/American Stroke Association. Stroke. (2023) 54:e314–70. doi: 10.1161/STR.0000000000000436
16. Costa, FG, Hakimi, N, and Van Bel, F. Neuroprotection of the perinatal brain by early information of cerebral oxygenation and perfusion patterns. Int J Mol Sci. (2021) 22:5389. doi: 10.3390/ijms22105389
17. Farago, E, Macisaac, D, Suk, M, and ADC, C. A review of techniques for surface electromyography signal quality analysis. IEEE Rev Biomed Eng. (2023) 16:472–86. doi: 10.1109/RBME.2022.3164797
18. Ferraro, M, Demaio, JH, Krol, J, Trudell, C, Rannekleiv, K, Edelstein, L, et al. Assessing the motor status score: a scale for the evaluation of upper limb motor outcomes in patients after stroke. Neurorehabil Neural Repair. (2002) 16:283–9. doi: 10.1177/154596830201600306
19. Motiwala, Z, Sandholu, AS, Sengupta, D, and Kulkarni, K. Wavelet coherence phase analysis decodes the universal switching mechanism of Ras GTPase superfamily. iScience. (2023) 26:107031. doi: 10.1016/j.isci.2023.107031
20. Struckmann, W, Bodén, R, Gingnell, M, Fällmar, D, and Persson, J. Modulation of dorsolateral prefrontal cortex functional connectivity after intermittent theta-burst stimulation in depression: combining findings from fNIRS and fMRI. Neuroimage Clin. (2022) 34:103028. doi: 10.1016/j.nicl.2022.103028
21. Marchetti, PH, Gomes, WA, Da Silva, JJ, Magalhaes, RA, LFM, T, and Whiting, WC. Backseat inclination affects the myoelectric activation during the inclined leg press exercise in recreationally trained men. J Strength Cond Res. (2023) 37:e541–5. doi: 10.1519/JSC.0000000000004504
22. Drew, PJ. Neurovascular coupling: motive unknown. Trends Neurosci. (2022) 45:809–19. doi: 10.1016/j.tins.2022.08.004
23. Dimyan, MA, and Cohen, LG. Neuroplasticity in the context of motor rehabilitation after stroke. Nat Rev Neurol. (2011) 7:76–85. doi: 10.1038/nrneurol.2010.200
24. Xing, Y, and Bai, Y. A review of exercise-induced neuroplasticity in ischemic stroke: pathology and mechanisms. Mol Neurobiol. (2020) 57:4218–31. doi: 10.1007/s12035-020-02021-1
25. Ranzani, R, Lambercy, O, Metzger, JC, Califfi, A, Regazzi, S, Dinacci, D, et al. Neurocognitive robot-assisted rehabilitation of hand function: a randomized control trial on motor recovery in subacute stroke. J Neuroeng Rehabil. (2020) 17:115. doi: 10.1186/s12984-020-00746-7
26. Borges, LR, Fernandes, AB, Oliveira Dos Passos, J, Guerra, RO, and Campos, TF. Action observation for upper limb rehabilitation after stroke. Cochrane Database Syst Rev. (2022) 8:CD011887. doi: 10.1002/14651858.CD011887.pub3
27. Zhang, Y, Xing, Y, Li, C, Hua, Y, Hu, J, Wang, Y, et al. Mirror therapy for unilateral neglect after stroke: a systematic review. Eur J Neurol. (2022) 29:358–71. doi: 10.1111/ene.15122
28. Chen, YW, Li, KY, Lin, CH, Hung, PH, Lai, HT, and Wu, CY. The effect of sequential combination of mirror therapy and robot-assisted therapy on motor function, daily function, and self-efficacy after stroke. Sci Rep. (2023) 13:16841. doi: 10.1038/s41598-023-43981-3
29. Shao, C, Wang, Y, Gou, H, Xiao, H, and Chen, T. Strength training of the nonhemiplegic side promotes motor function recovery in patients with stroke: a randomized controlled trial. Arch Phys Med Rehabil. (2023) 104:188–94. doi: 10.1016/j.apmr.2022.09.012
30. Li, X, Fang, F, Li, R, and Zhang, Y. Functional brain controllability alterations in stroke. Front Bioeng Biotechnol. (2022) 10:925970. doi: 10.3389/fbioe.2022.925970
31. Feng, H, Jiang, Y, Lin, J, Qin, WT, Jin, LJ, and Shen, X. Cortical activation and functional connectivity during locomotion tasks in Parkinson's disease with freezing of gait. Front Aging Neurosci. (2023) 15:1068943. doi: 10.3389/fnagi.2023.1068943
32. Zhang, Y, Zhao, W, Wan, C, Wu, X, Huang, J, Wang, X, et al. Exoskeleton rehabilitation robot training for balance and lower limb function in sub-acute stroke patients: a pilot, randomized controlled trial. J Neuroeng Rehabil. (2024) 21:98. doi: 10.1186/s12984-024-01391-0
33. Li, H, Fu, X, Lu, L, Guo, H, Yang, W, Guo, K, et al. Upper limb intelligent feedback robot training significantly activates the cerebral cortex and promotes the functional connectivity of the cerebral cortex in patients with stroke: a functional near-infrared spectroscopy study. Front Neurol. (2023) 14:1042254. doi: 10.3389/fneur.2023.1042254
34. Guo, C, Sui, Y, Xu, S, Zhuang, R, Zhang, M, Zhu, S, et al. Contralaterally controlled neuromuscular electrical stimulation-induced changes in functional connectivity in patients with stroke assessed using functional near-infrared spectroscopy. Front Neural Circuits. (2022) 16:955728. doi: 10.3389/fncir.2022.955728
35. Nakamura, S, Yomota, S, Ito, H, Akinaga, N, Hori, A, Chinomi, K, et al. A novel cognitive function scale using functional near-infrared spectroscopy for evaluating cognitive dysfunction. J Alzheimers Dis. (2021) 81:1579–88. doi: 10.3233/JAD-210072
36. Mekbib, DB, Debeli, DK, Zhang, L, Fang, S, Shao, Y, Yang, W, et al. A novel fully immersive virtual reality environment for upper extremity rehabilitation in patients with stroke. Ann N Y Acad Sci. (2021) 1493:75–89. doi: 10.1111/nyas.14554
37. Xiong, F, Liao, X, Xiao, J, Bai, X, Huang, J, Zhang, B, et al. Emerging limb rehabilitation therapy after post-stroke motor recovery. Front Aging Neurosci. (2022) 14:863379. doi: 10.3389/fnagi.2022.863379
38. Cui, W, Huang, L, Tian, Y, Luo, H, Chen, S, Yang, Y, et al. Effect and mechanism of mirror therapy on lower limb rehabilitation after ischemic stroke: a fMRI study. NeuroRehabilitation. (2022) 51:65–77. doi: 10.3233/NRE-210307
39. Xia, X, Dong, X, Huo, H, Zhang, Y, Song, J, and Wang, D. Clinical study of low-frequency acupoint electrical stimulation to improve thumb-to-finger movements after stroke: a randomized controlled trial. Medicine. (2023) 102:e35755. doi: 10.1097/MD.0000000000035755
40. Fitzpatrick, RC. The cortex, interneurones and motoneurones in the control of movement. J Physiol. (2008) 586:1215–6. doi: 10.1113/jphysiol.2007.149518
41. Reschechtko, S, and Pruszynski, JA. Stretch reflexes. Curr Biol. (2020) 30:R1025–30. doi: 10.1016/j.cub.2020.07.092
42. Hao, J, Xie, H, Harp, K, Chen, Z, and Siu, KC. Effects of virtual reality intervention on neural plasticity in stroke rehabilitation: a systematic review. Arch Phys Med Rehabil. (2022) 103:523–41. doi: 10.1016/j.apmr.2021.06.024
43. Heelas, T, Theis, N, and Hughes, JD. Muscle activation patterns during variable resistance deadlift training with and without elastic bands. J Strength Cond Res. (2021) 35:3006–11. doi: 10.1519/JSC.0000000000003272
44. Wong, JJ, Shearer, HM, Mior, S, Jacobs, C, Côté, P, Randhawa, K, et al. Are manual therapies, passive physical modalities, or acupuncture effective for the management of patients with whiplash-associated disorders or neck pain and associated disorders? An update of the bone and joint decade task force on neck pain and its associated disorders by the OPTIMa collaboration. Spine J. (2016) 16:1598–630. doi: 10.1016/j.spinee.2015.08.024
45. Mehrholz, J, Pollock, A, Pohl, M, Kugler, J, and Elsner, B. Systematic review with network meta-analysis of randomized controlled trials of robotic-assisted arm training for improving activities of daily living and upper limb function after stroke. J Neuroeng Rehabil. (2020) 17:83. doi: 10.1186/s12984-020-00715-0
Keywords: robot-assisted hand function therapy, functional near-infrared spectroscopy, surface electromyography, brain function, stroke
Citation: Cheng C, Liu T, Zhang B, Wu X, Song Z, Zhao Z, Ren X, Zhao M, Su Y and Wang J (2024) Effects of robot-assisted hand function therapy on brain functional mechanisms: a synchronized study using fNIRS and sEMG. Front. Med. 11:1411616. doi: 10.3389/fmed.2024.1411616
Edited by:
Qi Guo, Shanghai University of Medicine and Health Sciences, ChinaReviewed by:
Ying Shen, The First Affiliated Hospital of Nanjing Medical University, ChinaDingjie Suo, Beijing Institute of Technology, China
Davide Frumento, University of Genoa, Italy
Copyright © 2024 Cheng, Liu, Zhang, Wu, Song, Zhao, Ren, Zhao, Su and Wang. This is an open-access article distributed under the terms of the Creative Commons Attribution License (CC BY). The use, distribution or reproduction in other forums is permitted, provided the original author(s) and the copyright owner(s) are credited and that the original publication in this journal is cited, in accordance with accepted academic practice. No use, distribution or reproduction is permitted which does not comply with these terms.
*Correspondence: Jiening Wang, drwjn0606@sina.com
†These authors share first authorship