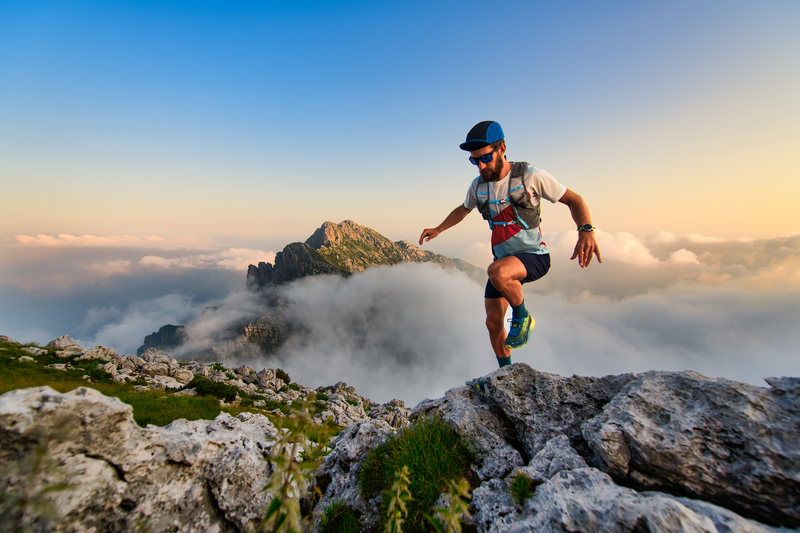
95% of researchers rate our articles as excellent or good
Learn more about the work of our research integrity team to safeguard the quality of each article we publish.
Find out more
BRIEF RESEARCH REPORT article
Front. Med. , 20 March 2024
Sec. Precision Medicine
Volume 11 - 2024 | https://doi.org/10.3389/fmed.2024.1361317
This article is part of the Research Topic Women in Science - Precision Medicine 2023 View all 4 articles
Introduction: Databases used for clinical interpretation in oncology rely on genetic data derived primarily from patients of European ancestry, leading to biases in cancer genetics research and clinical practice. One practical issue that arises in this context is the potential misclassification of multi-ancestral population variants as tumor-associated because they are not represented in reference genomes against which tumor sequencing data is aligned.
Methods: To systematically find misclassified variants, we compared somatic variants in census genes from the Catalogue of Somatic Mutations in Cancer (COSMIC) V99 with multi-ancestral population variants from the Genome Aggregation Databases’ Linkage Disequilibrium (GnomAD). By comparing genomic coordinates, reference, and alternate alleles, we could identify misclassified variants in genes associated with cancer.
Results: We found 192 of 208 genes in COSMIC’s cancer-associated census genes (92.31%) to be associated with variant misclassifications. Among the 1,906,732 variants in COSMIC, 6,957 variants (0.36%) aligned with normal population variants in GnomAD, concerning for misclassification. The African / African American ancestral population included the greatest number of misclassified variants and also had the greatest number of unique misclassified variants.
Conclusion: The direct, systematic comparison of variants from COSMIC for co-occurrence in GnomAD supports a more accurate interpretation of tumor sequencing data and reduces bias related to genomic ancestry.
With the rapid advances of targeted therapies and associated biomarkers, genetic data is increasingly necessary to facilitate clinical management of cancer (1, 2). Collaborative databases are used by clinicians to help classify variants from molecular testing as associated with malignancy, inherited rare cancer syndromes, or normal population variation (3, 4). As with many genetics efforts, underrepresentation of non-European ancestral populations in these clinical databases is a critical bottleneck to their universal applicability.
Numerous clinical challenges currently arise from the overwhelming overrepresentation of patients of European ancestry in cancer genetics data. These include inadequate training of clinical tools (as observed in the first generation of commercially available polygenic risk scores) (5, 6); less accurate prediction of treatment response for specific populations in clinic (7–9); inadvertent biases against offering available interventions or studies to patients (10); and insufficient representation in precision oncology registries to inform future translational research work (11, 12).
In the context of clinical variant interpretation databases, one such potential issue is the misclassification of variants as somatic (associated with the cancer) when they are, in fact, germline (associated with patients’ ancestral populations). This may occur depending on the reference genome used and can be clinically problematic if misclassified variants are directly relevant to diagnosis, treatment, or prognosis (13). In other words, such variants may be used as an indication for potential treatment when they may not be cancer-specific, or may be accidentally used by oncologists to provide inaccurate prognostic information or molecular pathologists in the course of diagnosis. Misclassified variants are also critical to be aware of in the context of cancer research. Human variant origin (whether germline or somatic) is often a necessary specification in translational oncology studies ranging from drug mechanism of action to inclusion criteria for clinical trials (14, 15).
While some variant callers have advanced filtering of germline variants from tumor-only data using multiple population databases, they require a baseline knowledge of bioinformatics and typically remove germline variants without characterizing more information about the potential germline variants (16). Other efforts have interrogated somatic variants that have been included in population databases (17).
To evaluate this concern, we compared ostensibly somatic variants from the Catalogue Of Somatic Mutations In Cancer (COSMIC) database (18), used to categorize cancer-specific variants, to population-specific variants from the Genome Aggregation Database (GnomAD) (19). We observed that over 92% of the 208 cancer-associated genes in COSMIC had at least one misclassified variant, and that 6,957 variants (0.36% of all variants in COSMIC) were concerning for misclassification. Of these, we found that the African / African American genetic ancestry population in GnomAD contained the most variants associated with misclassification in COSMIC and the greatest number of unique misclassified variants. Our findings emphasize the need for accurate variant classification across populations for clinicians and translational researchers.
The Catalogue Of Somatic Mutations In Cancer (COSMIC) contains variants observed in cancers. These variants are aggregated through expert curation via publication review (focused on specific genes / diseases) and tumor genome-wide screening data (18). Variants in COSMIC were obtained using the unified file from v99.
The Genome Aggregation Database (GnomAD) contains variants and allele frequencies collected from over 76,000 individuals. Variants from GnomAD used in this project were obtained from the annotated Linkage Disequilibrium datasets in v2, in which variants were assigned by GnomAD to 8 ancestral populations: African/African American, Latino/Admixed American, Ashkenazi Jewish, East Asian, Finnish European, Estonian, North-Western European and Southern European.
The COSMIC Cancer Gene Census is an ongoing effort by COSMIC to categorize genes that drive cancers (20). The census is updated on an ongoing basis and available with explanations for each gene and its relationship to cancer here: https://cancer.sanger.ac.uk/census.
Data processing and visualization were performed in Python v3.10.7 by leveraging the Pandas library and matplotlib v3.5.3.
The COSMIC dataset was converted from a tab-separated value (.TSV) format to a comma-separated value (.CSV) format using the Pandas library. Reference and alternate allele columns were added by parsing the “CDS” column in Pandas. The. CSV file was partitioned by transcript accessions to generate 34,317 separate files.
For the GnomAD datasets, we generated. CSV files of variants in each ancestral population. We lifted over the coordinates from GRCh37 to GRCh38 and added a “genomic coordinate” column based on the GRCh38 chromosome and position columns.
We iteratively compared each partitioned COSMIC. CSV file and each GnomAD. CSV file based on 3 parameters: genomic coordinate, reference allele, and alternate allele, with misclassified variants defined as matches across both files. These matches were subsequently merged, and duplicate rows deleted, within ancestral populations. Our pipeline systematically quantified the total number of variants and unique genes per ancestral population.
To facilitate a streamlined comparison of all misclassified variants, we merged all 8 ancestral populations into a list of unique variants listed by genomic coordinates, reference (ref) and alternate (alt) allele columns, and allele frequencies per population.
Chi-squared testing was used to identify significant differences by population among misclassified variants and genes.
Figure 1 demonstrates the project concept. Among 208 cancer-associated genes in the COSMIC cancer gene census, 192 (92.3%) were found to have misclassified variants. ABL had the greatest number of unique misclassified variants identified at 274, but 19 genes (ABL1, PTPRT, HLA-A, JAK2, AFF3, PREX2, EGFR, ETV6, MLLT3, ALK, EBF1, MTOR, NOTCH1, AFDN, KMT2C, FA1, FAM135B, FAT3, and MUC4) were associated with over 100 unique misclassified variants. Supplementary Table S1 lists all 192 genes by number of unique positions in the gene and variants observed.
Figure 1. Project overview. Variants that overlapped between COSMIC and GnomAD based on genomic coordinate, reference allele, and alternate allele were incorporated, and variant allele frequencies per population per GnomAD were reported.
We identified 6,957 unique variants out of 1,906,732 (0.36%) total in COSMIC that aligned with normal population variants in GnomAD (Figure 2). We evaluated how many of these variants were reported in each ancestral population. The population with the greatest inclusion of misclassified variants was the African/African American population, with 5,320 misclassified variants (76.47%). The Ashkenazi Jewish population had the second greatest inclusion of misclassified variants, with 4,668 (67.10%). Comparatively, the other populations included between 59 and 64% of the misclassified variants. The difference of included misclassified variants across populations was statistically significant (p < 1×10−5).
Figure 2. Variants concerning for misclassification in COSMIC by ancestral population. The X-axis displays the number of overlapping variants between COSMIC and GnomAD, while the Y-axis specifies the total count. Variants may be in multiple ancestral groups.
To assess the extent to which the total number of variants in GnomAD may influence the number of misclassified variants reported in each population, we compared the number of misclassified variants per population to the overall numbers of variants per population in GnomAD (Figure 3). The African/African American population had the largest number reported variants at 17,478,395, with variants misclassified in COSMIC representing 0.03%. The Southern European population had the smallest number of reported variants at 9,071,699, with variants misclassified in COSMIC representing 0.05%. However, the proportion of misclassified variants across all GnomAD variants per population was not statistically significant.
Figure 3. Misclassified variants (aligned between GnomAD and COSMIC) compared to total variants in GnomAD by population. The X-axis lists the specific population, while the Y-axis reports the number of total variants in GnomAD (blue) and number of overlapping variants between COSMIC and GnomAD concerning for misclassification (purple).
We compared shared variants pairwise by population to determine the extent of population-specific versus shared variants across populations (Figure 4). In each pairwise comparison, we reported number shared variants across those populations. We also report the number of variants unique to that population. The African/African American population had more misclassified variants unique to its population (1,019) relative to any other population, followed by the East Asian (326) and Ashkenazi Jewish (216) populations. In contrast, European populations and the Admixed American/Latino population had <100 unique variants.
Figure 4. Variants compared pairwise across ancestral groups. The base number of variants is the 6,957 total unique variants identified. Diagonal entries show the number of variants unique to that ancestral group.
In this project, we compared variants in the COSMIC cancer gene census to variants in GnomAD across ancestral population to identify potentially misclassified population-level variants. We sought to demonstrate in a straightforward fashion the clinical relevance of our findings by directly comparing across these databases and providing a systematic assessment that reflects the breadth of this issue across an established list of cancer-specific genes.
Although we observed a comparatively low fraction of all variants in COSMIC were affected by potential misclassification, concerningly, we found that over 90 percent of genes in the COSMIC cancer gene census had at least one misclassified variant. Given that COSMIC is routinely used in molecular pathology laboratories to make recommendations for diagnosis, prediction, and prognostication based on cancer-specific variants, it is crucial to identify and address issues that systematically affect accurate variant classification (21).
Among the 19 genes associated with over 100 unique misclassified variants, several are concerning because of variants’ role in prediction of therapy use for patients. ABL1 mutations, although usually specific to known resistance mutations, can be used to select alternative therapies in chronic myeloid leukemia (18). While JAK2 V617F and exon 12 mutations are well known to contribute to development of leukemia, other variants are still considered if identified (22). EGFR and ALK mutations are critically relevant for prediction of treatment response in lung cancer (23). Numerous clinical trials actively seek patients with somatic variants in these genes as well as genes underlying therapeutic targets, such as mTOR, to study therapy response. Misclassification is critically important to correctly identifying somatic origin, and accordingly appropriate prediction of patient treatment response, in this setting.
In their documentation, COSMIC reports that they use the Cancer Mutation Census (CMC) as a tool to help users annotate somatic mutations. This effort actually already includes data from ClinVar (germline pathogenic variants associated with inherited disease) (24) and gnomAD, but is aligned to GRCh37, not automatically integrated into COSMIC, uses its own definition of “mutation significance” rather than drawing on existing equivalent efforts [such as OncoKB (25)] and confusingly combines definitions from ClinVar that were intended for germline variants. We would certainly suggest that COSMIC consider updating, refining, integrating this effort and consider using it as a filtration step for curated variants.
The overrepresentation of variants, and particularly unique population-specific variants, corresponding to the African / African-American ancestral population is also strongly concerning for inadvertent bias. This finding is likely associated with the known phenomenon of decreased linkage disequilibrium and increased occurrence of variants in African ancestral populations (26–28). We observed no significant difference in proportion of misclassified variants among proportion of variants in GnomAD across populations, suggesting that the number of misclassified variants in a population relates to the total number of variants present. Projects such as the Human Pangenome Reference demonstrate the limitations of the current reference genome and opportunities in moving to genomic references with greater diversity, with “3.7 million additional single-nucleotide polymorphisms (SNPs) in regions non-syntenic to GRCh38” among other expansions (29). From a clinical standpoint, moving towards these comprehensive reference efforts at a rate much faster than the transition from GRCh37 to GRCh38 may have the opportunity to adequately serve more patients.
There are limitations to this work we wish to acknowledge. Our overlap search across COSMIC and GnomAD is currently limited to single nucleotide variants. As broad population-level data for structural variants, mutational signatures, and chromosome-scale changes becomes more widely available in future, this could easily be incorporated into the same framework. From the variant to the gene level, it would be fair to draw comparisons across other cancer driver gene datasets. It would also be ideal to expand and streamline this analysis in future across other germline and somatic variant classification and annotation databases (such as ClinVar and OncoKB) (25). One effort examined overlapping variants between GnomAD and The Cancer Genome Atlas, but intentionally focused on rare population variants to study potential biological etiologies including statistical chance, convergent evolution, and correlated mutational rates at specific genetic sites (30). Realistically, the need to apply this search across multiple germline and somatic databases reflects an ongoing limitation of the field regarding data siloing, and the issue of adequate population representation remains active across all of these (31).
The variants that we identified in this study that are misclassified as somatic when actually germline underscores the need for ongoing efforts to improve inclusivity of genetic data across diverse ancestral populations. As we demonstrate, by correctly identifying variants linked to disease as opposed to population, this effort directly offers benefit to all oncology patients.
Publicly available datasets were analyzed in this study. Direct links to data are provided in manuscript.
RP: Conceptualization, Data curation, Formal analysis, Visualization, Writing – original draft, Writing – review & editing. MW: Conceptualization, Data curation, Formal analysis, Supervision, Writing – original draft. PR: Formal analysis, Supervision, Visualization, Writing – review & editing.
The author(s) declare financial support was received for the research, authorship, and/or publication of this article. Funding for this study is provided in part by the Uniformed Services University of the Health Sciences (USUHS) and the Intramural Research Program of the National Cancer Institute, National Institutes of Health (ZIA BC 012112, PR).
We wish to thank Dr. Stanley Lipkowitz for his help facilitating the generation and publication of this manuscript. The contents of this publication are the sole responsibility of the author(s) and do not necessarily reflect the views, opinions, or policies of Uniformed Services University of the Health Sciences (USUHS), the Department of Defense (DoD) or the Departments of the Army, Navy, or Air Force. Mention of trade names, commercial products, or organizations does not imply endorsement by the U.S. government.
The authors declare that the research was conducted in the absence of any commercial or financial relationships that could be construed as a potential conflict of interest.
All claims expressed in this article are solely those of the authors and do not necessarily represent those of their affiliated organizations, or those of the publisher, the editors and the reviewers. Any product that may be evaluated in this article, or claim that may be made by its manufacturer, is not guaranteed or endorsed by the publisher.
The Supplementary material for this article can be found online at: https://www.frontiersin.org/articles/10.3389/fmed.2024.1361317/full#supplementary-material
1. Ghazani, AA, Oliver, NM, St Pierre, JP, Garofalo, A, Rainville, IR, Hiller, E, et al. Assigning clinical meaning to somatic and germ-line whole-exome sequencing data in a prospective cancer precision medicine study. Genet Med. (2017) 19:787–95. doi: 10.1038/gim.2016.191
2. Chakravarty, D, and Solit, DB. Clinical cancer genomic profiling. Nat Rev Genet. (2021) 22:483–501. doi: 10.1038/s41576-021-00338-8
3. Tsang, H, Addepalli, K, and Davis, SR. Resources for interpreting variants in precision genomic oncology applications. Front Oncol. (2017) 7:214. doi: 10.3389/fonc.2017.00214
4. Schwartzberg, L, Kim, ES, Liu, D, and Schrag, D. Precision oncology: who, how, what, when, and when not? Am Soc Clin Oncol Educ Book. (2017) 37:160–9. doi: 10.1200/EDBK_174176
5. Martin, AR, Kanai, M, Kamatani, Y, Okada, Y, Neale, BM, and Daly, MJ. Clinical use of current polygenic risk scores may exacerbate health disparities. Nat Genet. (2019) 51:584–91. doi: 10.1038/s41588-019-0379-x
6. Ruan, Y, Lin, YF, Feng, YCA, Chen, CY, Lam, M, Guo, Z, et al. Improving polygenic prediction in ancestrally diverse populations. Nat Genet. (2022) 54:573–80. doi: 10.1038/s41588-022-01054-7
7. Balogun, OD, and Olopade, OI. Addressing health disparities in cancer with genomics. Nat Rev Genet. (2021) 22:621–2. doi: 10.1038/s41576-021-00390-4
8. Nassar, AH, Adib, E, Alaiwi, SA, Zarif, TE, Groha, S, Akl, EW, et al. Ancestry-driven recalibration of tumor mutational burden and disparate clinical outcomes in response to immune checkpoint inhibitors. Cancer Cell. (2022) 40:1161–1172.e5. doi: 10.1016/j.ccell.2022.08.022
9. Martini, R, Gebregzabher, E, Newman, L, and Davis, MB. Enhancing the trajectories of Cancer health disparities research: improving clinical applications of diversity, equity, inclusion, and accessibility. Cancer Discov. (2022) 12:1428–34. doi: 10.1158/2159-8290.CD-22-0278
10. Pierce, LJ . Building a bridge to equity in health and health care in cancer care. Cancer J. (2023) 29:285–6. doi: 10.1097/PPO.0000000000000690
11. Landry, LG, Ali, N, Williams, DR, Rehm, HL, and Bonham, VL. Lack of diversity in genomic databases is a barrier to translating precision medicine research into practice. Health Aff (Millwood). (2018) 37:780–5. doi: 10.1377/hlthaff.2017.1595
12. Cheung, ATM, Palapattu, EL, Pompa, IR, Aldrighetti, CM, Niemierko, A, Willers, H, et al. Racial and ethnic disparities in a real-world precision oncology data registry. NPJ Precis Oncol. (2023) 7:1–6. doi: 10.1038/s41698-023-00351-6
13. Frequently Asked Questions – Genome Reference Consortium . (Accessed Dec 5, 2023). Available at: https://www.ncbi.nlm.nih.gov/grc/help/faq/#human-reference-genome-individuals.
14. Moody, EW, Vagher, J, Espinel, W, Goldgar, D, Hagerty, KJ, and Gammon, A. Comparison of somatic and germline variant interpretation in hereditary cancer genes. JCO Precis Oncol. (2019) 3:1–8. doi: 10.1200/PO.19.00144
15. Subbiah, V, and Kurzrock, R. Universal germline and tumor genomic testing needed to win the war against cancer: genomics is the diagnosis. J Clin Oncol. (2023) 41:3100–3. doi: 10.1200/JCO.22.02833
16. Sukhai, MA, Misyura, M, Thomas, M, Garg, S, Zhang, T, Stickle, N, et al. Somatic tumor variant filtration strategies to optimize tumor-only molecular profiling using targeted next-generation sequencing panels. J Mol Diagn. (2019) 21:261–73. doi: 10.1016/j.jmoldx.2018.09.008
17. Avramović, V, Frederiksen, SD, Brkić, M, and Tarailo-Graovac, M. Driving mosaicism: somatic variants in reference population databases and effect on variant interpretation in rare genetic disease. Hum Genomics. (2021) 15:71. doi: 10.1186/s40246-021-00371-y
18. Tate, JG, Bamford, S, Jubb, HC, Sondka, Z, Beare, DM, Bindal, N, et al. COSMIC: the catalogue of somatic mutations in cancer. Nucleic Acids Res. (2019) 47:D941–7. doi: 10.1093/nar/gky1015
19. Chen, S, Francioli, LC, Goodrich, JK, Collins, RL, Kanai, M, Wang, Q, et al. A genome-wide mutational constraint map quantified from variation in 76,156 human genomes. bioRxiv. (2022):2022.03.20.485034. Available at: https://www.biorxiv.org/content/10.1101/2022.03.20.485034v2
20. Sondka, Z, Bamford, S, Cole, CG, Ward, SA, Dunham, I, and Forbes, SA. The COSMIC cancer gene census: describing genetic dysfunction across all human cancers. Nat Rev Cancer. (2018) 18:696–705. doi: 10.1038/s41568-018-0060-1
21. Li, MM, Datto, M, Duncavage, EJ, Kulkarni, S, Lindeman, NI, Roy, S, et al. Standards and guidelines for the interpretation and reporting of sequence variants in Cancer: a joint consensus recommendation of the Association for Molecular Pathology, American Society of Clinical Oncology, and College of American Pathologists. J Mol Diagn. (2017) 19:4–23. doi: 10.1016/j.jmoldx.2016.10.002
22. Benton, CB, Boddu, PC, DiNardo, CD, Bose, P, Wang, F, Assi, R, et al. Janus kinase 2 variants associated with the transformation of myeloproliferative neoplasms into acute myeloid leukemia. Cancer. (2019) 125:1855–66. doi: 10.1002/cncr.31986
23. Lovly, CM, Iyengar, P, and Gainor, JF. Managing resistance to EFGR- and ALK-targeted therapies. Am Soc Clin Oncol Educ Book. (2017) 37:607–18. doi: 10.1200/EDBK_176251
24. Landrum, MJ, Lee, JM, Riley, GR, Jang, W, Rubinstein, WS, Church, DM, et al. ClinVar: public archive of relationships among sequence variation and human phenotype. Nucleic Acids Res. (2014) 42:D980–5. doi: 10.1093/nar/gkt1113
25. Chakravarty, D, Gao, J, Phillips, SM, Kundra, R, Zhang, H, Wang, J, et al. OncoKB: a precision oncology knowledge base. JCO Precis Oncol. (2017) 2017:PO.17.00011. doi: 10.1200/PO.17.00011
26. Tishkoff, SA, and Williams, SM. Genetic analysis of African populations: human evolution and complex disease. Nat Rev Genet. (2002) 3:611–21. doi: 10.1038/nrg865
27. Shifman, S, Kuypers, J, Kokoris, M, Yakir, B, and Darvasi, A. Linkage disequilibrium patterns of the human genome across populations. Hum Mol Genet. (2003) 12:771–6. doi: 10.1093/hmg/ddg088
28. Charles, BA, Shriner, D, and Rotimi, CN. Accounting for linkage disequilibrium in association analysis of diverse populations. Genet Epidemiol. (2014) 38:265–73. doi: 10.1002/gepi.21788
29. Liao, WW, Asri, M, Ebler, J, Doerr, D, Haukness, M, Hickey, G, et al. A draft human pangenome reference. Nature. (2023) 617:312–24. doi: 10.1038/s41586-023-05896-x
30. Meyerson, W, Leisman, J, Navarro, FCP, and Gerstein, M. Origins and characterization of variants shared between databases of somatic and germline human mutations. BMC Bioinformatics. (2020) 21:227. doi: 10.1186/s12859-020-3508-8
Keywords: germline, somatic, variant classification, misclassification, health disparities
Citation: Pollard RD, Wilkerson MD and Rajagopal PS (2024) Identification of germline population variants misclassified as cancer-associated somatic variants. Front. Med. 11:1361317. doi: 10.3389/fmed.2024.1361317
Received: 25 December 2023; Accepted: 28 February 2024;
Published: 20 March 2024.
Edited by:
Alice Chen, Consultant, Potomac, MD, United StatesReviewed by:
Gagandeep Dhillon, University of Maryland, Baltimore, United StatesCopyright © 2024 Pollard, Wilkerson and Rajagopal. This is an open-access article distributed under the terms of the Creative Commons Attribution License (CC BY). The use, distribution or reproduction in other forums is permitted, provided the original author(s) and the copyright owner(s) are credited and that the original publication in this journal is cited, in accordance with accepted academic practice. No use, distribution or reproduction is permitted which does not comply with these terms.
*Correspondence: Padma Sheila Rajagopal, c2hlaWxhLnJhamFnb3BhbEBuaWguZ292
Disclaimer: All claims expressed in this article are solely those of the authors and do not necessarily represent those of their affiliated organizations, or those of the publisher, the editors and the reviewers. Any product that may be evaluated in this article or claim that may be made by its manufacturer is not guaranteed or endorsed by the publisher.
Research integrity at Frontiers
Learn more about the work of our research integrity team to safeguard the quality of each article we publish.