- 1Zhejiang Province People’s Hospital, Affiliated People's Hospital, Hangzhou Medical College, Hangzhou, China
- 2Department of Rheumatology and Immunology of Zhejiang Provincial People's Hospital, Center for General Practice Medicine, Hangzhou, China
- 3Zhejiang Provincial Key Laboratory of Traditional Chinese Medicine for Arthritis Diagnosis and Treatment, Hangzhou, China
Background: The extra-articular lesions of rheumatoid arthritis (RA) are reported to involve multiple organs and systems throughout the body, including the heart, kidneys, liver, and lungs. This study assessed the potential causal relationship between RA and the risk of chronic kidney diseases (CKDs) using the Mendelian randomization (MR) analysis.
Method: Independent genetic instruments related to RA and CKD or CKD subtypes at the genome-wide significant level were chosen from the publicly shared summary-level data of genome-wide association studies (GWAS). Then, we obtained some single-nucleotide polymorphisms (SNPs) as instrumental variables (IVs), which are associated with RA in individuals of European origin, and had genome-wide statistical significance (p5 × 10−8). The inverse-variance weighted (IVW) method was the main analysis method in MR analysis. The other methods, such as weighted median, MR–Egger, simple mode, and weighted mode were used as supplementary sensitivity analyses. Furthermore, the levels of pleiotropy and heterogeneity were assessed using Cochran’s Q test and leave-one-out analysis. Furthermore, the relevant datasets were obtained from the Open GWAS database.
Results: Using the IVW method, the main method in MR analysis, the results showed that genetically determined RA was associated with higher risks of CKD [odds ratio (OR): 1.22, 95% confidence interval (CI) 1.13–1.31; p < 0.001], glomerulonephritis (OR: 1.23, 95% CI 1.15–1.31; p < 0.000), amyloidosis (OR = 1.43, 95% CI 1.10–1.88, p < 0.001), and renal failure (OR = 1.18, 95% CI 1.00–1.38, p < 0.001). Then, using multiple MR methods, it was confirmed that the associations persisted in sensitivity analyses, and no pleiotropy was detected.
Conclusion: The findings revealed a causal relationship between RA and CKD, including glomerulonephritis, amyloidosis, and renal failure. Therefore, RA patients should pay more attention to monitoring their kidney function, thus providing the opportunity for earlier intervention and lower the risk of progression to CKDs.
Introduction
Rheumatoid arthritis (RA) is a common autoimmune disease associated with synovial tissue proliferation and inflammation of the joint tissue. It can also cause damage to other tissues and organs such as the heart, kidneys, lungs, and nervous system, leading to serious systemic disorders (1, 2). Approximately 25% of RA patients develop chronic kidney disease (CKD) (3, 4), which is proportionally higher than that of healthy individuals (5).
CKD is a common chronic disease characterized by long-term loss of kidney function with an extremely high mortality rate (6). It affects millions of patients worldwide (7). The worldwide burden of CKD is increasing yearly, with nearly 10% of the adult population worldwide affected by one of its subtypes (8, 9). There are several causes of CKD; however, diabetes, glomerulonephritis, and cystic kidney disease are among the more common and well-studied causes (10). However, the definitive influential factors of CKD have still not been fully investigated.
Recently, a few articles addressed the relationship between RA and CKD. However, from a previous report, most cases can be divided into two categories: chronic inflammation (such as secondary kidney atherosclerosis and amyloidosis) and drug-induced kidney diseases (11). Furthermore, previous studies have shown that elevated levels of inflammatory markers, including C-reactive protein (CRP), in the early clinical stages are related to future CKD (12, 13). Thus, it can be deemed that there are various causes of RA with kidney disease, but the definitive mechanism still needs to be studied.
Mendelian randomization (MR) analysis is a method used in epidemiology to research the causal relationship between genetic exposure factors and outcomes (14, 15). The MR analysis employs SNPs as IVs for exposure, thus it can effectively avoid the interference factors of relevant trait changes and has high scientific value. To explore the potential causal relationship between RA and CKD, the MR analysis was used to study whether RA affects the risk of CKDs from a genetic perspective.
Materials and methods
Study design
The study analyzed the causal relationship between RA and CKD using the MR analysis. RA as an exposure factor and CKD or other different subtypes of CKD (including membranous nephropathy, diabetic nephropathy, hypertensive renal disease, glomerulonephritis, nephrotic syndrome, amyloidosis, and renal failure) as outcome factors. These summary-level data, from genome-wide association studies (GWASs), can be obtained from the IEU Open database.1 This analysis adhered to the Strengthening the Reporting of Observational Studies in Epidemiology (STROBE)-MR Statement (16, 17). The study design overview is presented in Figure 1.
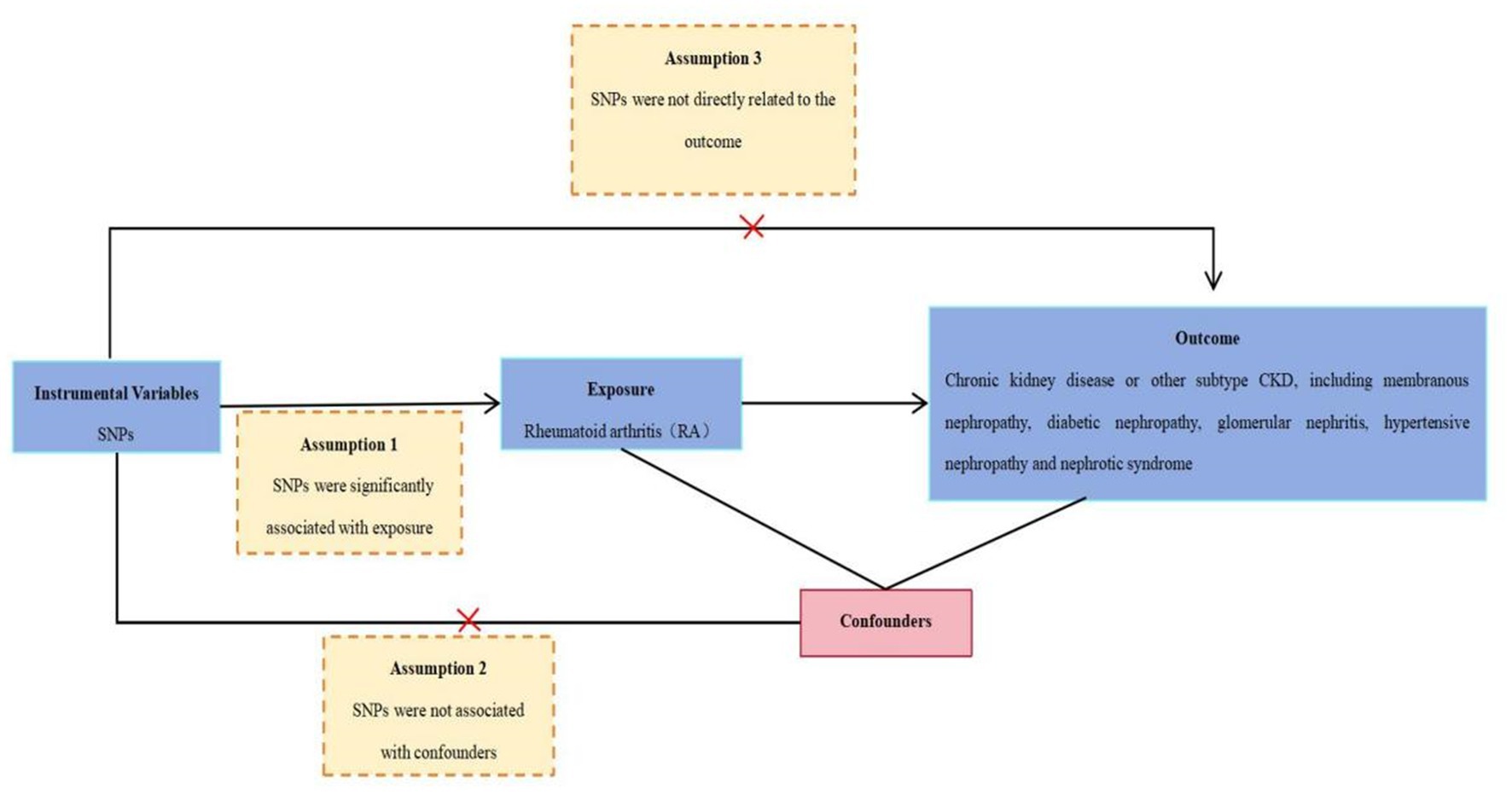
Figure 1. Schematic representation of an MR analysis. Three basic assumptions of MR analysis: Assumption 1: SNPs were significantly associated with RA. Assumption 2: SNPs were not associated with the confounders. Assumption 3: SNPs were not directly related to CKD. MR, Mendelian randomization; SNPs, single-nucleotide polymorphisms. IVs, instrumental variables selection. RA, rheumatoid arthritis. CKD, chronic kidney disease.
Data source of disease
The summary-level GWAS data of RA, CKD, and other subtypes of CKD were obtained from the Open GWAS database. To minimize the bias from population stratification, we decided to incorporate the summary data from the population of European ancestry in this study. A description of the details of the data source is summarized in Table 1.
Instrumental variable selection
This study extracted SNPs, as IVs related to RA, with genome-wide significance levels (p < 5 × 10−8). Thereafter, the threshold was set for linkage disequilibrium at R2 < 0.001 to exclude the effect of linkage disequilibrium. Furthermore, GWAS Catalog2 was used to verify whether the included SNPs were related to confounders. SNPs that were not related to confounders were selected for further analysis. Finally, the F value was calculated to assess the strength of the IVs, and an F-value < 10 indicated a significant bias in causality estimates (18). Thus, an F-value > 10 was selected in this study.
Ethical approval
The GWAS summary data were obtained from published studies in the GWAS open database approved by the institutional review boards. Therefore, this study did not need to acquire ethical approval.
Mendelian randomization analysis
The genetic correlations of RA with CKD and CKD subtypes were based on computation using data from the IEU Open GWAS database. Based on these data, this study utilized SNPs as IVs to evaluate the etiological relationship between RA and CKD and other CKD subsets. The random-effects IVW model was the main statistical approach, complemented by other sensitivity analyses, including MR Egger, weighted median, simple mode, weighted mode, Cochran’s Q, and leave-one-out analysis.
The IVW method is a predominantly dependent method that amounts to a correlation-weighted linear regression between variables by calculating the ORs and the 95% CIs for each additional unit in logarithmic probability (19). The relationship between the outcome and exposure variables was subjected to a weighted linear regression, combining the Wald ratio estimates for each of the meta-analytic IVs in a meta-analytic manner (20). The MR–Egger method can be used to detect and correct for possible unbalanced pleiotropy, although its estimates are usually not significant (21). Finally, the study used Cochrane’s Q and leave-one-out analysis to evaluate the polysemy and heterogeneity levels. A p-value of >0.05 indicates no heterogeneity. The p-value of the MR-Egger intercept was used as an indicator of pleiotropy in one analysis of SNP estimation (21). A p-value of <0.05 suggests horizontal pleiotropy between the exposure and outcome factors, indicating that the outcome remains even in the absence of the exposure factor.
Statistical analysis
In this study, all the statistical examinations were two-sided tests, and R Studio was used to calculate the genetic relevance. The MR analysis was conducted using the “TwoSampleMR” (22) packages in R Studio (R version 4.3.1). A p-value of <0.05 was considered significant.
Results
The association between RA and CKD
In this MR analysis study, five methods were applied to confirm the robustness of the results, including the IVW method (the main method), MR Egger, weighted median, simple mode, and weighted mode (Figure 2). Based on the data from these methods, it can be inferred that a positive association existed between RA and CKD. By using the IVW method, a positive association was seen between RA and CKD (OR = 1.22, 95% CI 1.13–1.31, p < 0.001; Figure 2). The positive relevance was uniform when determined using the MR–Egger method (OR = 1.18, 95% CI 1.05–1.32, p = 0.013), weighted median (OR = 1.26, 95% CI 1.14–1.41, p < 0.001), simple mode (OR = 1.33, 95% CI 1.06–1.68, p = 0.023), and weighted mode (OR = 1.26, 95% CI 1.30–1.40, p < 0.001; Figures 2, 3). Furthermore, the results of sensitivity analyses, including forest plot and leave-one-out analysis showed that no SNP had a significant effect (Figure 4). In addition, MR–Egger did not detect potential horizontal pleiotropy for RA (p = 0.492), and neither the IVW nor MR-Egger methods revealed significant heterogeneity by Cochran’s Q test (p = 0.449, p = 0.418, respectively; Figures 2–6; Table 2).
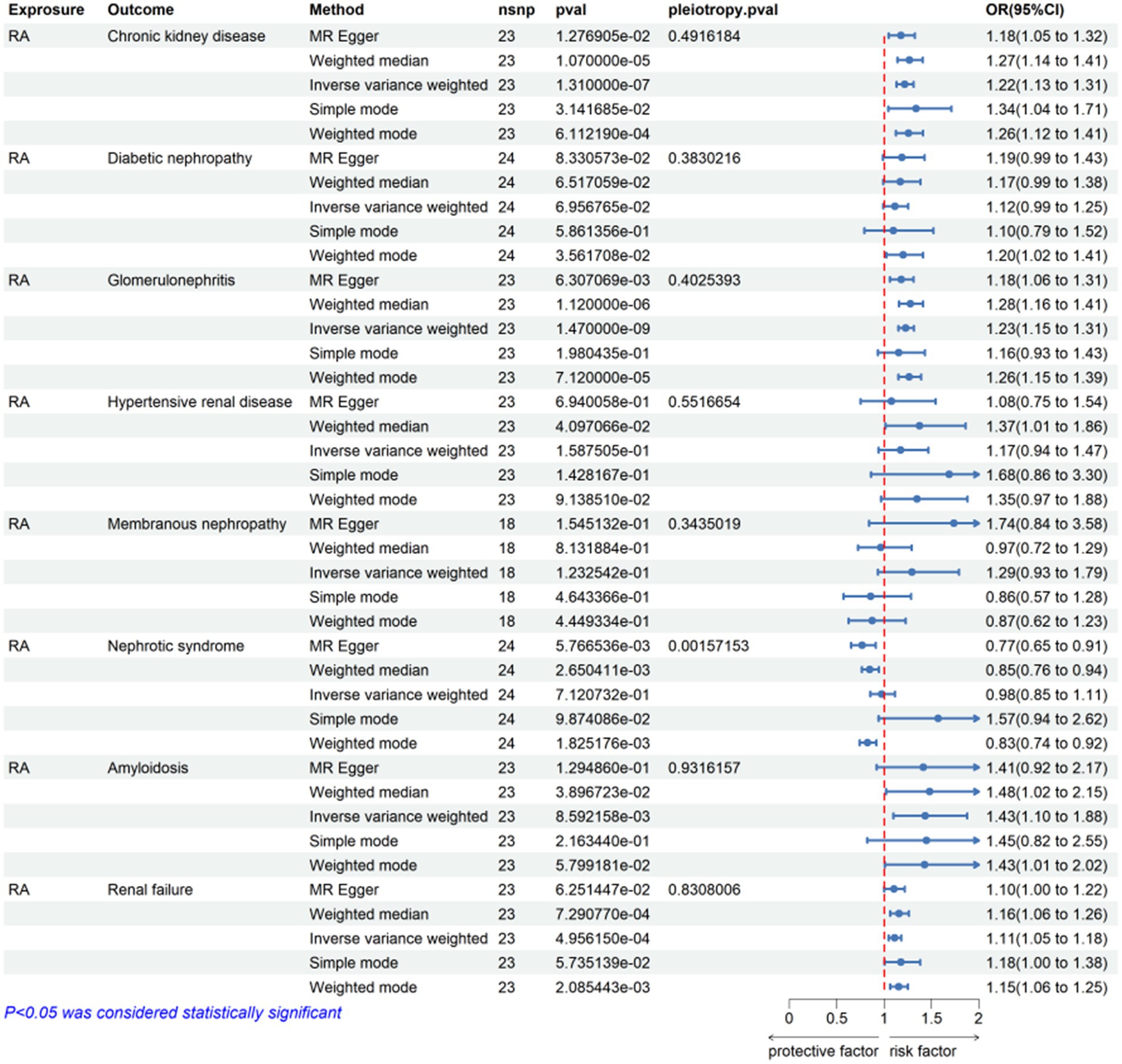
Figure 2. Mendelian randomization for the association of rheumatoid arthritis on CKD and other CKD subsets. RA, rheumatoid arthritis; nsnp, number of SNPs used in MR; OR, odds ratio; CI, confidence interval.
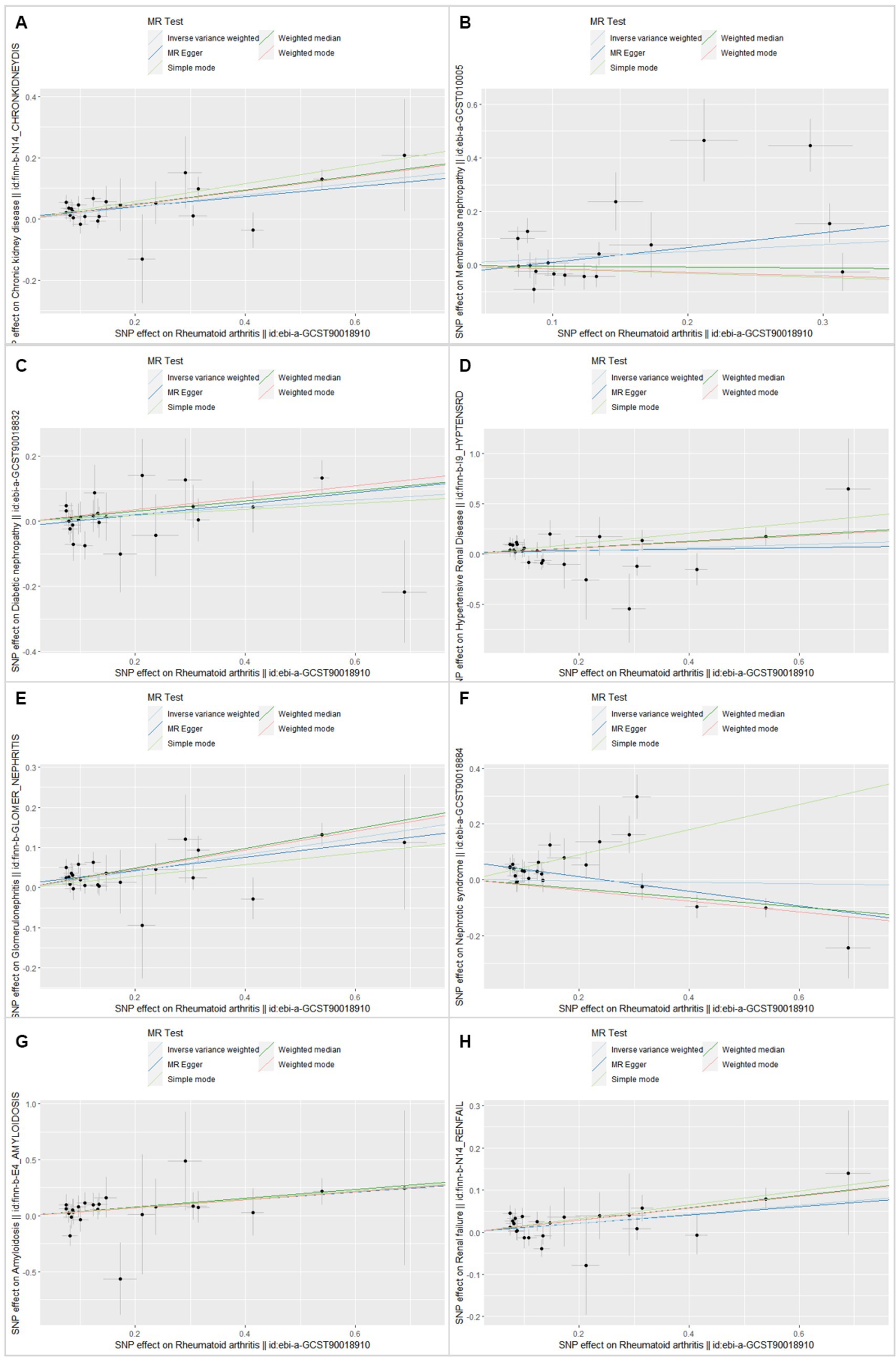
Figure 3. Scatter plot of single-nucleotide polymorphism (SNP) potential effects on rheumatoid arthritis vs. CKD and its subsets. The 95% CI for the effect size on CKD/CKD subsets is shown as vertical lines, and the 95% CI for the effect size on rheumatoid arthritis is shown as horizontal lines. The slope of fitted lines represents the estimated Mendelian randomization effect per method. (A) Chronic kidney disease. (B) Membranous nephropathy. (C) Diabetic nephropathy. (D) Hypertensive renal disease. (E) Glomerulonephritis. (F) Nephrotic syndrome. (G) Amyloidosis. (H) Renal failure.
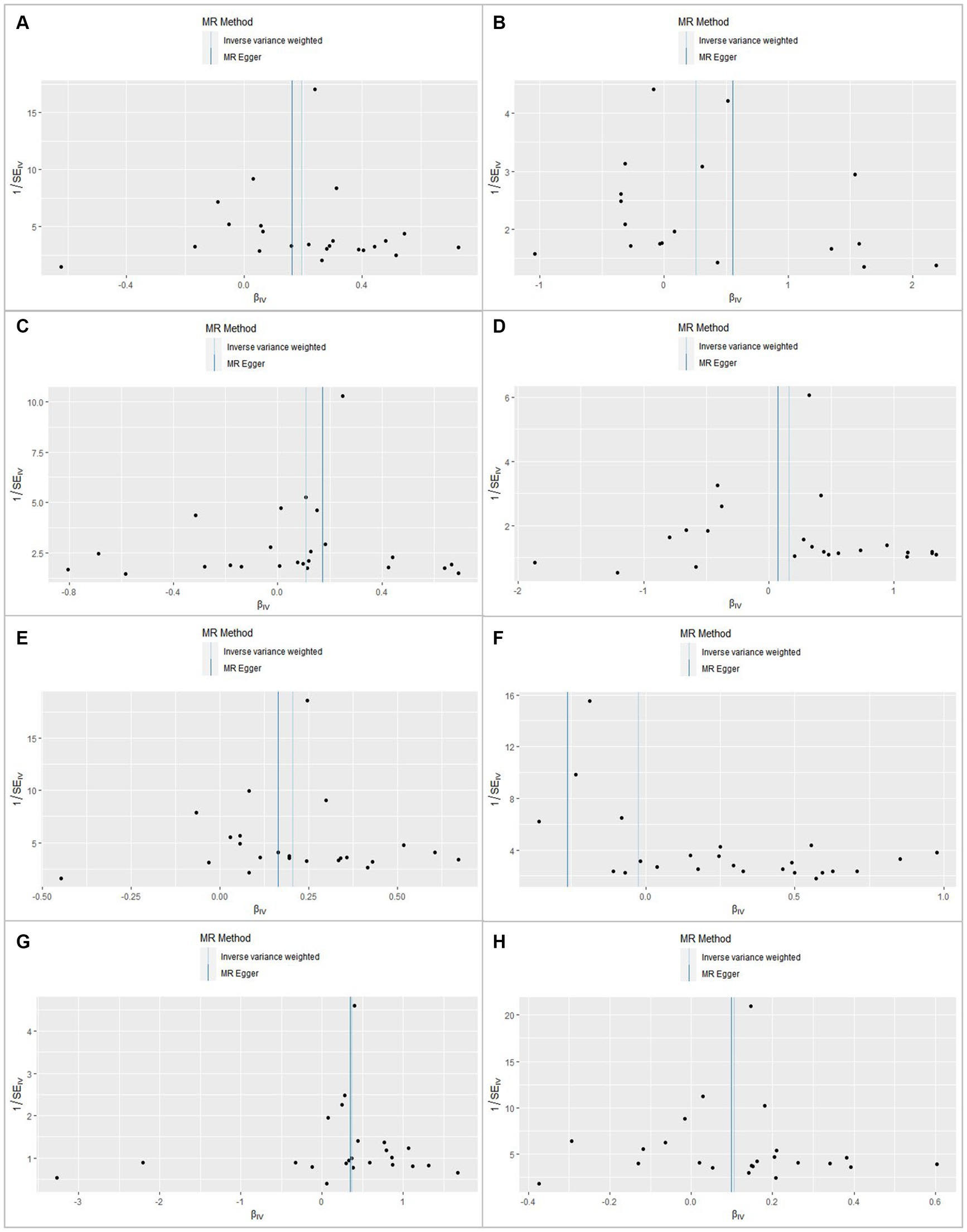
Figure 4. Funnel plot for rheumatoid arthritis shows the estimation using the inverse of the standard error of the causal estimate with each SNP as a tool. The vertical line represents the estimated causal effect obtained using the IVW and MR–Egger methods. (A) Chronic kidney disease. (B) Membranous nephropathy. (C) Diabetic nephropathy. (D) Hypertensive renal disease. (E) Glomerulonephritis. (F) Nephrotic syndrome. (G) Amyloidosis. (H) Renal failure.
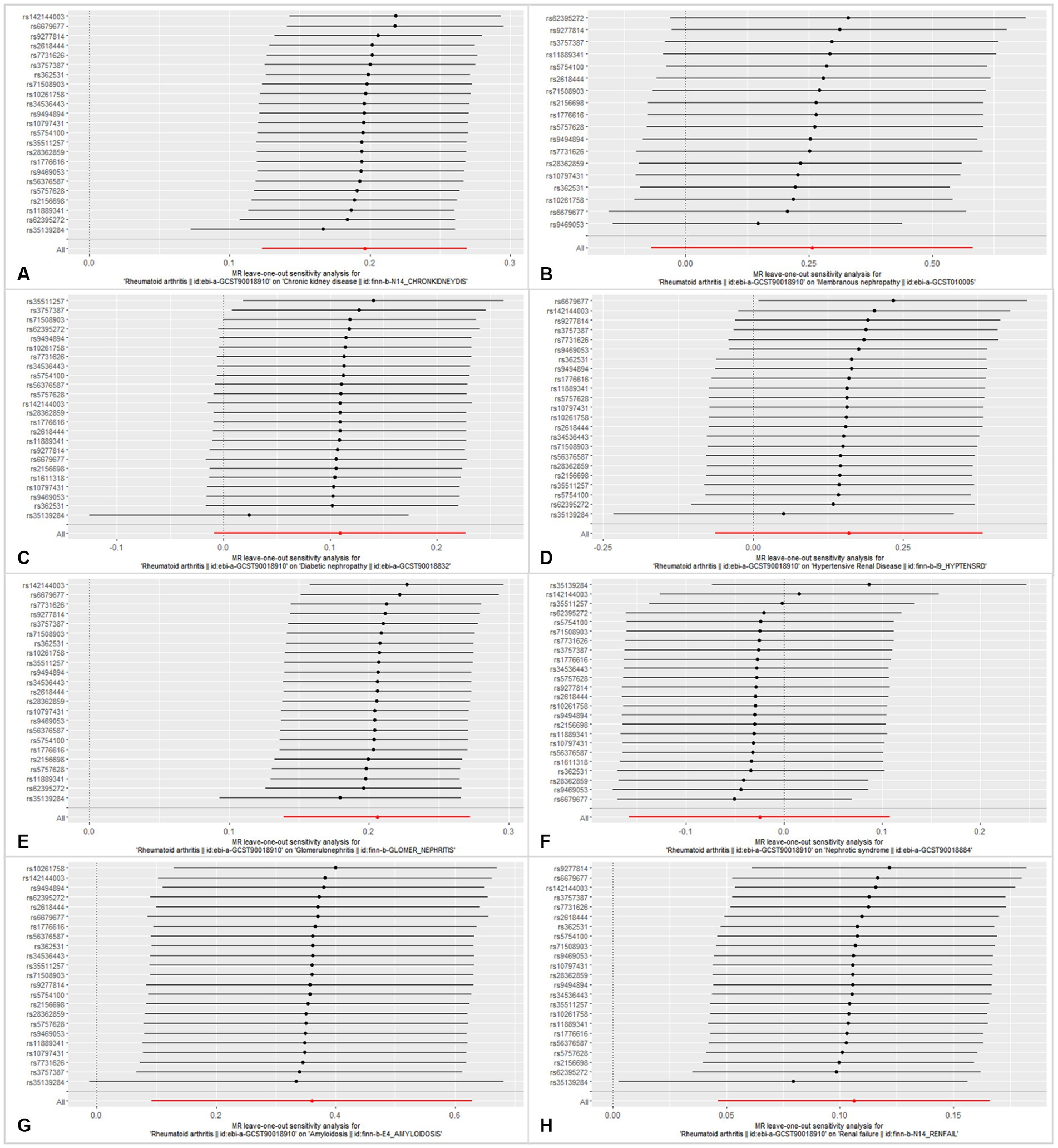
Figure 5. Leave-one-out analysis of each SNP associated with CKD and other CKD subtypes, and the red lines represent estimations from the IVW test. (A) Chronic kidney disease. (B) Membranous nephropathy. (C) Diabetic nephropathy. (D) Hypertensive renal disease. (E) Glomerulonephritis. (F) Nephrotic syndrome. (G) Amyloidosis. (H) Renal failure.
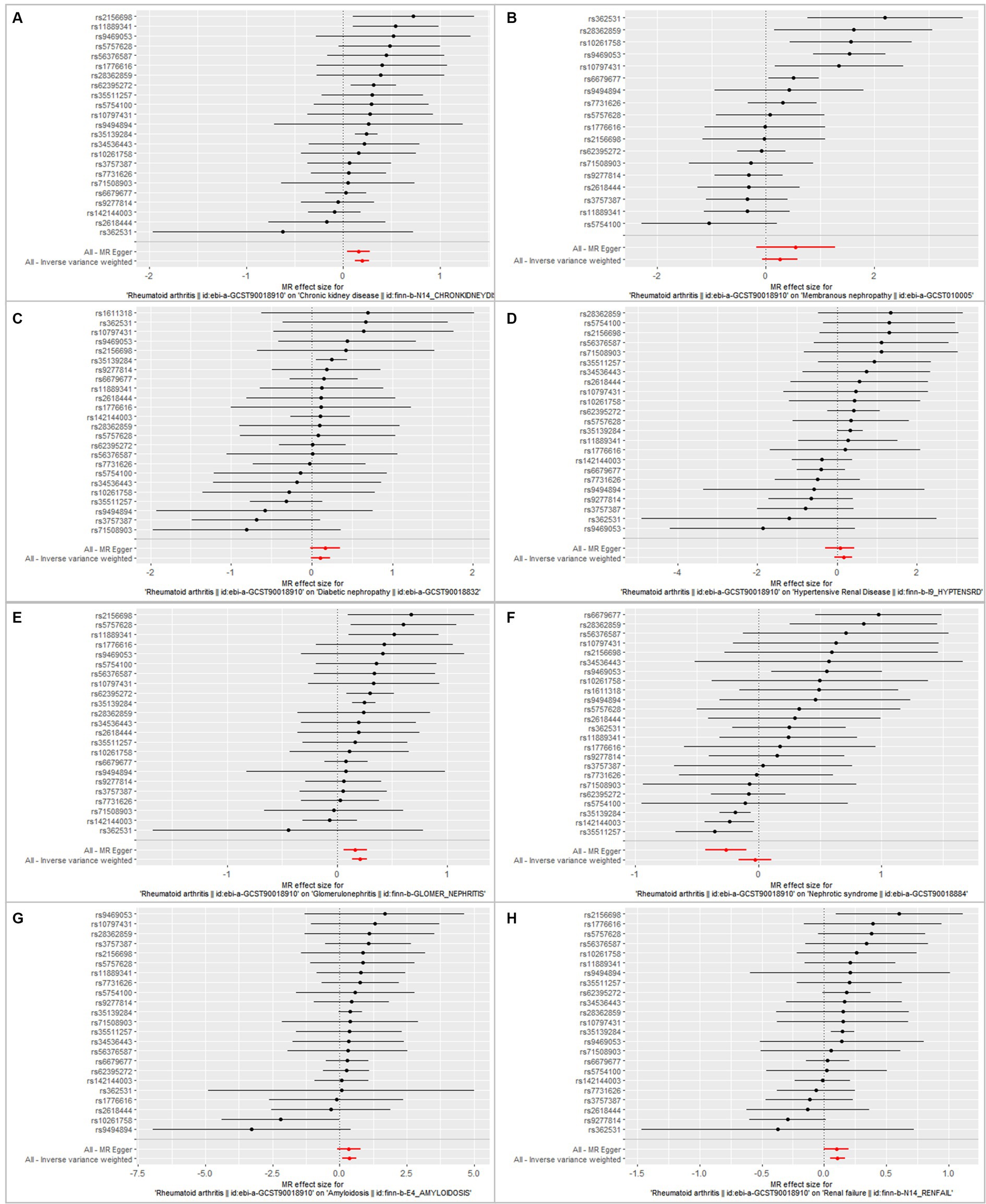
Figure 6. Forest plot showing the association between RA and CKD/CKD subtypes using instrumental variables of each SNP, alone or with all of the SNPs, using the MR–Egger or inverse-variance weighted methods. Bars show effect size and 95% confidence interval. (A) Chronic kidney disease. (B) Membranous nephropathy. (C) Diabetic nephropathy. (D) Hypertensive renal disease. (E) Glomerulonephritis. (F) Nephrotic syndrome. (G) Amyloidosis. (H) Renal failure.
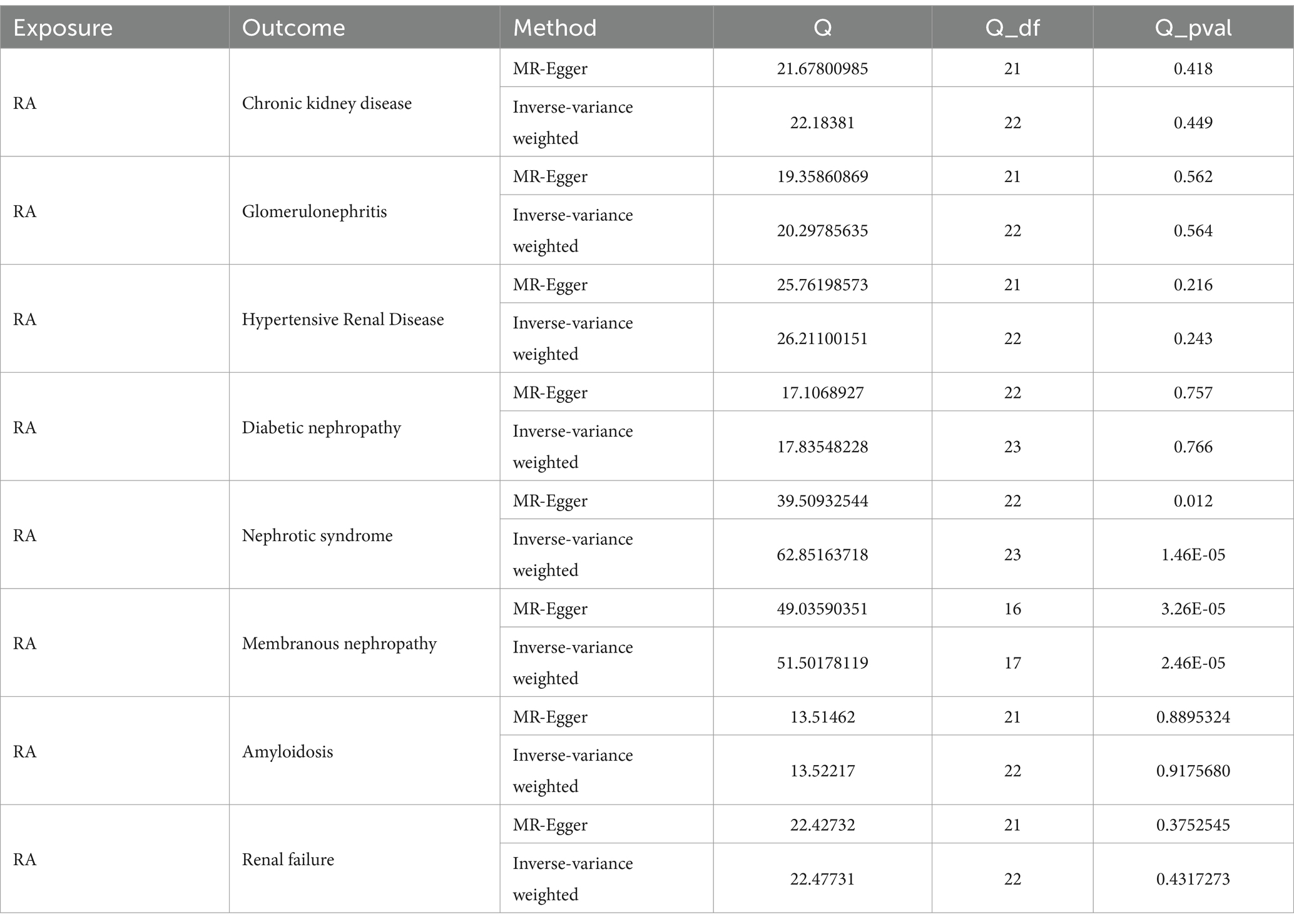
Table 2. The sensitivity analysis results for the association between RA and relevant kidney diseases.
The association between RA and CKD subtypes
By using the IVW method of MR analysis, a positive association existed between RA and glomerulonephritis (OR = 1.23, 95% CI 1.15–1.31, p < 0.000; Figure 2). Furthermore, the positive relevance was uniform when determined using the MR–Egger method (OR = 1.18, 95% CI 1.06–1.31, p = 0.006), weighted median (OR = 1.28, 95% CI 1.16–1.40, p < 0.001), and weighted mode (OR = 1.26, 95% CI 1.14–1.40, p < 0.001; Figures 2, 3). Potential horizontal pleiotropy was not detected for RA (p = 0.344), and there was no significant heterogeneity detected using Cochran’s Q test with either the IVW or MR–Egger methods (p = 0.564, p = 0.562, respectively; Table 2; Figure 4).
Furthermore, by using the IVW method of MR analysis, a positive association was seen between RA and amyloidosis (OR = 1.43, 95% CI 1.10–1.88, p < 0.001). Potential horizontal pleiotropy was not detected for RA (p = 0.932; Figures 2, 3), and there was no significant heterogeneity detected using Cochran’s Q test with either the IVW or MR–Egger methods (p = 0.918, p = 0.890, respectively; Table 2; Figure 4). Furthermore, a positive association existed between RA and renal failure (OR = 1.18, 95% CI 1.00–1.38, p < 0.001), the potential horizontal pleiotropy was not detected for RA (p = 0.831; Figures 2, 3), and there was no significant heterogeneity detected using Cochran’s Q test with either the IVW or MR–Egger methods (p = 0.432, p = 0.375, respectively; Table 2; Figures 4–6).
However, the other CKD subtypes were not significantly associated with RA, membranous nephropathy (OR = 1.29, 95% CI 0.93–1.79, p > 0.05), diabetic nephropathy (OR = 1.11, 95% CI 0.99–1.25, p > 0.05), hypertensive renal disease (OR = 1.17, 95% CI 0.94–1.47, p > 0.05), and nephrotic syndrome (OR = 0.97, 95% CI 0.85–1.11, p > 0.05; Figures 2–6; Table 2).
The description of SNPs selected from these diseases
Comprehensively, the chronic kidney diseases significantly associated with RA, as analyzed using the MR analysis in this study included chronic kidney disease, glomerulonephritis, amyloidosis, and renal failure. The SNPs associated with these four diseases in the dataset were tested for single-nucleotide results, and a p-value of <0.05 indicated significant differences. The results are shown in Table 3, laying the foundation for subsequent correlation studies and analyses.
Discussion
RA is a chronic inflammatory autoimmune disorder that is characterized by joint and extra-articular manifestations (EAMs) and can cause systemic effects (23). The EAMs of RA were more common in patients with severe active disease, for example, the eyes, lungs, skin, heart, and kidney (24). The mortality of RA patients with EAM is 2.5-fold that of RA patients without EAM. In addition, previous studies have found that the prevalence of comorbidities in patients with arthritis is high, including CKD (25) and glomerulonephritis (26).
CKD is a chronic, immune-mediated, and systemic inflammatory disease, which is one of the common complications in RA patients (4, 27–30). Several conditions can be used to explain the relationship between CKD and RA, such as secondary amyloidosis, glomerulonephritis, or a drug-related cause (31, 32). However, the specific causes of CKD in a majority of RA patients are usually not identified. Hence, elucidating the risk factors for developing chronic kidney disease among them is critical. In addition, the associations of RA with various kidney diseases are attributed to chronic inflammation, drug exposure, and toxicity (32). Furthermore, only a few studies have described the prevalence of chronic kidney disease in patients with RA (3, 4, 33). Thus, there are limited data on CKD in RA (34). The association between chronic inflammation and the incidence of CKD in RA patients remains unclear (13). Thus, MR was used to explore the potential causal relationship between RA and CKD in this study.
MR analysis is a powerful instrument for examining the associations between exposures and outcome factors and helps to prioritize potential causal associations. In comparison to randomized controlled trials, MR analysis has the advantage of controlling confounding factors and overcoming the waste of human and material resources. In addition, compared to observational studies, MR studies are generally considered to provide higher-quality evidence (16). The main virtue is the MR analysis study design, which confirmed the causal inference in the relationship between RA and CKD. This study used data from the IEU Open GWAS database with large sample sizes. In addition, this study included seven other CKD subtypes to confirm the relationship of RA. Finally, the results of the sensitivity analyses ensured the robustness of the associations. The SNPs with statistical significance shown in Table 3, established a foundation for further research and exploration.
Nonetheless, there were certain limitations in this study. The sample size is a common and important limiting factor in such studies, and there is more reliability in the conclusions when the sample size is larger. Furthermore, the data included mixed gender loci and since it is difficult to isolate gender data, gender may cause a bias in the current study, and may have influenced the results to some extent. For further study, a long-term cohort study is needed to validate the conclusions of this study, involving age and gender data. Additionally, the study samples were from individuals of European descent, so the generalizability of the MR analysis results to other races/ethnicities is uncertain. In addition, although confounders were removed through the PhenoScanner V2, the elaboration of specific drug effects was not clear, and therefore, the effects of drugs will be a key direction for subsequent exploration and research.
Conclusion
In conclusion, the findings revealed that there is a causal association between RA and CKD, and in this MR analysis, glomerular nephritis, amyloidosis, and renal failure were found to be related to RA. Therefore, in RA patients, it is necessary to pay attention to the development of these types of chronic kidney diseases. However, it is unclear whether this causality is direct or indirect, and drug effects have not been completely ruled out, hence, further research is required. It is necessary to conduct large-scale prospective cohort studies to validate the preliminary findings of our study, and even more basic experimental studies with human tissues are needed to elucidate the deep molecular mechanisms.
To conclude, long-term follow-up of kidney function should be emphasized in RA patients to have the opportunity for earlier interventions to slow down the progression of CKD.
Data availability statement
The original contributions presented in the study are included in the article/Supplementary material, further inquiries can be directed to the corresponding author.
Author contributions
ZJ: Conceptualization, Methodology, Visualization, Writing – original draft. LC: Data curation, Investigation, Methodology, Writing – original draft. AL: Data curation, Methodology, Writing – original draft. JQ: Data curation, Methodology, Writing – original draft. JW: Investigation, Validation, Writing – original draft. YL: Data curation, Writing – original draft. HJ: Methodology, Writing – original draft. JZ: Formal analysis, Writing – original draft. SH: Project administration, Writing – original draft. CM: Project administration, Writing – original draft. ZY: Supervision, Writing – review & editing, Project administration.
Funding
The author(s) declare that no financial support was received for the research, authorship, and/or publication of this article.
Acknowledgments
The authors express our gratitude to the IEU Open datasets for providing publicly available summarized data from genome-wide association studies on RA, chronic kidney disease, membranous nephropathy, diabetic nephropathy, hypertensive renal disease, glomerulonephritis, nephrotic syndrome, amyloidosis, and renal failure.
Conflict of interest
The authors declare that the research was conducted in the absence of any commercial or financial relationships that could be construed as a potential conflict of interest.
Publisher's note
All claims expressed in this article are solely those of the authors and do not necessarily represent those of their affiliated organizations, or those of the publisher, the editors and the reviewers. Any product that may be evaluated in this article, or claim that may be made by its manufacturer, is not guaranteed or endorsed by the publisher.
Supplementary material
The Supplementary material for this article can be found online at: https://www.frontiersin.org/articles/10.3389/fmed.2024.1360026/full#supplementary-material
Footnotes
References
1. Derksen, VFAM, Huizinga, TWJ, and van der Woude, D. The role of autoantibodies in the pathophysiology of rheumatoid arthritis. Semin Immunopathol. (2017) 39:437–46. doi: 10.1007/s00281-017-0627-z
2. Giles, JT . Extra-articular manifestations and comorbidity in rheumatoid arthritis: potential impact of pre-rheumatoid arthritis prevention. Clin Ther. (2019) 41:1246–55. doi: 10.1016/j.clinthera.2019.04.018
3. Sihvonen, S, Korpela, M, Mustonen, J, Laippala, P, and Pasternack, A. Renal disease as a predictor of increased mortality among patients with rheumatoid arthritis. Nephron Clin Pract. (2004) 96:c107–14. doi: 10.1159/000077372
4. Karstila, K, Korpela, M, Sihvonen, S, and Mustonen, J. Prognosis of clinical renal disease and incidence of new renal findings in patients with rheumatoid arthritis: follow-up of a population-based study. Clin Rheumatol. (2007) 26:2089–95. doi: 10.1007/s10067-007-0625-y
5. Tokoroyama, T, Ando, M, Setoguchi, K, Tsuchiya, K, and Nitta, K. Prevalence, incidence and prognosis of chronic kidney disease classified according to current guidelines: a large retrospective cohort study of rheumatoid arthritis patients. Nephrol Dial Transplant. (2017) 32:2035–42. doi: 10.1093/ndt/gfw315
6. Fraser, SD, Roderick, PJ, May, CR, McIntyre, N, McIntyre, C, Fluck, RJ, et al. The burden of comorbidity in people with chronic kidney disease stage 3: a cohort study. BMC Nephrol. (2015) 16:193. doi: 10.1186/s12882-015-0189-z
7. Thomas, B, Matsushita, K, Abate, KH, Al-Aly, Z, Ärnlöv, J, Asayama, K, et al. Global cardiovascular and renal outcomes of reduced gfr. J Am Soc Nephrol. (2017) 28:2167–79. doi: 10.1681/asn.2016050562
8. Xie, Y, Bowe, B, Mokdad, AH, Xian, H, Yan, Y, Li, T, et al. Analysis of the global burden of disease study highlights the global, regional, and national trends of chronic kidney disease epidemiology from 1990 to 2016. Kidney Int. (2018) 94:567–81. doi: 10.1016/j.kint.2018.04.011
9. GBD Chronic Kidney Disease Collaboration . Global, regional, and national burden of chronic kidney disease, 1990-2017: a systematic analysis for the global burden of disease study 2017. Lancet. (2020) 395:709–33. doi: 10.1016/s0140-6736(20)30045-3
10. Dincer, N, Dagel, T, Afsar, B, Covic, A, Ortiz, A, and Kanbay, M. The effect of chronic kidney disease on lipid metabolism. Int Urol Nephrol. (2019) 51:265–77. doi: 10.1007/s11255-018-2047-y
11. Hanaoka, H, Kikuchi, J, Hiramoto, K, Saito, S, Kondo, Y, and Kaneko, Y. Decreased chronic kidney disease in rheumatoid arthritis in the era of biologic disease-modifying anti-rheumatic drugs. Clin Kidney J. (2022) 15:1373–8. doi: 10.1093/ckj/sfac036
12. Hickson, LJ, Crowson, CS, Gabriel, SE, McCarthy, JT, and Matteson, EL. Development of reduced kidney function in rheumatoid arthritis. Am J Kidney Dis. (2014) 63:206–13. doi: 10.1053/j.ajkd.2013.08.010
13. Kochi, M, Kohagura, K, Shiohira, Y, Iseki, K, and Ohya, Y. Inflammation as a risk of developing chronic kidney disease in rheumatoid arthritis. PLoS One. (2016) 11:e0160225. doi: 10.1371/journal.pone.0160225
14. Lawlor, DA, Harbord, RM, Sterne, JA, Timpson, N, and Davey, SG. Mendelian randomization: using genes as instruments for making causal inferences in epidemiology. Stat Med. (2008) 27:1133–63. doi: 10.1002/sim.3034
15. Li, C, Yang, W, Wei, Q, and Shang, H. Causal Association of Leukocytes Count and Amyotrophic Lateral Sclerosis: a Mendelian randomization study. Mol Neurobiol. (2020) 57:4622–7. doi: 10.1007/s12035-020-02053-7
16. Du, Y, Li, F, Li, S, Ding, L, and Liu, M. Causal relationship between polycysticovary syndrome and chronic kidney disease: a Mendelian randomization study. Front Endocrinol. (2023) 14:1120119. doi: 10.3389/fendo.2023.1120119
17. Skrivankova, VW, Richmond, RC, Woolf, BAR, Yarmolinsky, J, Davies, NM, Swanson, SA, et al. Strengthening the reporting of observational studies in epidemiology using mendelian randomization: the STROBE-MR statement. JAMA. (2021) 326:1614–21. doi: 10.1001/jama.2021.18236
18. Nie, Q, Luo, Q, Yan, W, Zhang, T, Wang, H, and Wu, J. Rheumatoid arthritis and coronary atherosclerosis: a two-sample Mendelian randomization study. Front Cardiovasc Med. (2023) 10:1033644. doi: 10.3389/fcvm.2023.1033644
19. Burgess, S, Butterworth, A, and Thompson, SG. Mendelian randomization analysis with multiple genetic variants using summarized data. Genet Epidemiol. (2013) 37:658–65. doi: 10.1002/gepi.21758
20. Zheng, J, Baird, D, Borges, MC, Bowden, J, Hemani, G, Haycock, P, et al. Recent developments in mendelian randomization studies. Curr Epidemiol Rep. (2017) 4:330–45. doi: 10.1007/s40471-017-0128-6
21. Bowden, J, Davey Smith, G, and Burgess, S. Mendelian randomization with invalid instruments: effect estimation and bias detection through egger regression. Int J Epidemiol. (2015) 44:512–25. doi: 10.1093/ije/dyv080
22. Hemani, G, Zheng, J, Elsworth, B, Wade, KH, Haberland, V, Baird, D, et al. The MR-base platform supports systematic causal inference across the human phenome. eLife. (2018) 7:4408. doi: 10.7554/eLife.34408
23. Cimmino, MA, Parisi, M, Moggiana, G, Mela, GS, and Accardo, S. Prevalence of rheumatoid arthritis in Italy: the Chiavari study. Ann Rheum Dis. (1998) 57:315–8. doi: 10.1136/ard.57.5.315
24. Das, S, and Padhan, P. An overview of the extraarticular involvement in rheumatoid arthritis and its management. J Pharmacol Pharmacother. (2017) 8:81–6. doi: 10.4103/jpp.JPP_194_16
25. Ziade, N, el Khoury, B, Zoghbi, M, Merheb, G, Abi Karam, G, Mroue’, K, et al. Prevalence and pattern of comorbidities in chronic rheumatic and musculoskeletal diseases: the COMORD study. Sci Rep. (2020) 10:7683. doi: 10.1038/s41598-020-64732-8
26. Fayed, A, Shaker, A, Hamza, WM, and Wadie, M. Spectrum of glomerulonephritis in Egyptian patients with rheumatoid arthritis: a university hospital experience. Saudi J Kidney Dis Transpl. (2019) 30:803–11. doi: 10.4103/1319-2442.265455
27. Mustonen, J, Helin, H, Korpela, M, and Pasternack, A. Rheumatoid arthritis and the kidneys. Duodecim. (1985) 101:1549–56.
28. Boers, M, Dijkmans, BA, Breedveld, FC, Camps, JA, Chang, PC, van Brummelen, P, et al. Subclinical renal dysfunction in rheumatoid arthritis. Arthritis Rheum. (1990) 33:95–101. doi: 10.1002/art.1780330113
29. Koseki, Y, Terai, C, Moriguchi, M, Uesato, M, and Kamatani, N. A prospective study of renal disease in patients with early rheumatoid arthritis. Ann Rheum Dis. (2001) 60:327–31. doi: 10.1136/ard.60.4.327
30. Karie, S, Gandjbakhch, F, Janus, N, Launay-Vacher, V, Rozenberg, S, Mai Ba, CU, et al. Kidney disease in RA patients: prevalence and implication on RA-related drugs management: the MATRIX study. Rheumatology. (2008) 47:350–4. doi: 10.1093/rheumatology/kem370
31. Helin, HJ, Korpela, MM, Mustonen, JT, and Pasternack, AI. Renal biopsy findings and clinicopathologic correlations in rheumatoid arthritis. Arthritis Rheum. (1995) 38:242–7. doi: 10.1002/art.1780380213
32. Nakano, M, Ueno, M, Nishi, S, Shimada, H, Hasegawa, H, Watanabe, T, et al. Analysis of renal pathology and drug history in 158 Japanese patients with rheumatoid arthritis. Clin Nephrol. (1998) 50:154–60.
33. Mayer, U, Brsen, JH, and Pape, L. Rapid progressive glomerulonephritis in children. Klin Padiatr. (2019) 231:4–13. doi: 10.1055/a-0669-9271
Keywords: rheumatoid arthritis, chronic kidney diseases, Mendelian randomization analysis, genome-wide association studies, inverse-variance weighted
Citation: Jiang Z, Chen L, Liu A, Qi J, Wang J, Li Y, Jiang H, Zhang J, Huang S, Mao C and Ying Z (2024) Rheumatoid arthritis and the risk of chronic kidney diseases: a Mendelian randomization study. Front. Med. 11:1360026. doi: 10.3389/fmed.2024.1360026
Edited by:
Cong-Qiu Chu, Oregon Health and Science University, United StatesReviewed by:
Qingran Yan, Shanghai Jiao Tong University, ChinaMiguel Angel González-Gay, University of Cantabria, Spain
Tomoaki Higuchi, Tokyo Women's Medical University, Japan
Copyright © 2024 Jiang, Chen, Liu, Qi, Wang, Li, Jiang, Zhang, Huang, Mao and Ying. This is an open-access article distributed under the terms of the Creative Commons Attribution License (CC BY). The use, distribution or reproduction in other forums is permitted, provided the original author(s) and the copyright owner(s) are credited and that the original publication in this journal is cited, in accordance with accepted academic practice. No use, distribution or reproduction is permitted which does not comply with these terms.
*Correspondence: Zhenhua Ying, eWluZ3poMjAyMUAxNjMuY29t