- 1Department of Emergency Medicine, Wuhan Puren Hospital, Wuhan University of Science and Technology, Wuhan, China
- 2School of Medicine, Wuhan University of Science and Technology, Wuhan, China
- 3College of Life Science and Technology, Huazhong University of Science and Technology, Wuhan, China
- 4Maintainbiotech. Ltd. (Wuhan), Wuhan, Hubei, China
Background: Acute abdominal pain (AAP) is a common symptom presented in the emergency department (ED), and it is crucial to have objective and accurate triage. This study aims to develop a machine learning-based prediction model for AAP triage. The goal is to identify triage indicators for critically ill patients and ensure the prompt availability of diagnostic and treatment resources.
Methods: In this study, we conducted a retrospective analysis of the medical records of patients admitted to the ED of Wuhan Puren Hospital with acute abdominal pain in 2019. To identify high-risk factors, univariate and multivariate logistic regression analyses were used with thirty-one predictor variables. Evaluation of eight machine learning triage prediction models was conducted using both test and validation cohorts to optimize the AAP triage prediction model.
Results: Eleven clinical indicators with statistical significance (p < 0.05) were identified, and they were found to be associated with the severity of acute abdominal pain. Among the eight machine learning models constructed from the training and test cohorts, the model based on the artificial neural network (ANN) demonstrated the best performance, achieving an accuracy of 0.9792 and an area under the curve (AUC) of 0.9972. Further optimization results indicate that the AUC value of the ANN model could reach 0.9832 by incorporating only seven variables: history of diabetes, history of stroke, pulse, blood pressure, pale appearance, bowel sounds, and location of the pain.
Conclusion: The ANN model is the most effective in predicting the triage of AAP. Furthermore, when only seven variables are considered, including history of diabetes, etc., the model still shows good predictive performance. This is helpful for the rapid clinical triage of AAP patients and the allocation of medical resources.
1 Introduction
AAP is a condition that occurs within the abdomen and has a sudden onset, typically lasting less than a week (1). Patients with AAP are one of the major groups in the ED, accounting for 5–10% of all visits (2–4). AAP can have multiple causes, including gastrointestinal disorders, thoracic cardiovascular disease, and neurological disorders. They also vary in complexity and risk and often involve the clinical care needs of various specialties (including gynecology, pediatrics, internal medicine, surgery, etc.), which may require interdisciplinary and multidisciplinary collaboration, particularly in emergencies such as acute appendicitis, ruptured abdominal aortic aneurysm, and ectopic pregnancy (2, 5, 6). However, in the complex environment of the ED, where most patients with AAP claim to be in urgent need of pain management measures (7, 8), misdiagnosis or other inappropriate management measures can have catastrophic consequences, as well as lead to a range of legal disputes (9–11). This undoubtedly poses a greater challenge to the triage work of healthcare professionals in the ED. Therefore, there is a need to further clarify the risk characteristics of patients with AAP in terms of the severity of their condition. Additionally, there is a need to enhance the ability of healthcare professionals to differentiate the causes of AAP in the ED.
With the utilization of various advanced technologies in clinical medicine, such as computed tomography (CT), ultrasound, and magnetic resonance imaging (MRI), medical imaging plays a crucial role in delivering accurate clinical diagnoses and efficient care for patients with AAP (12, 13). However, non-essential diagnostics inevitably increase the cost of care for patients and the burden on hospital systems. Additionally, there are potential risk factors, such as allergies to contrast media and radiation exposure, that cannot be ignored, particularly in areas with limited healthcare resources (14–16). According to Trentzsch et al., it is crucial to quickly determine the underlying cause in AAP and assess whether urgent or immediate surgical intervention is needed (17). Therefore, there is an urgent need for an efficient triage tool that does not rely solely on radiological techniques but can accurately assess the criticality of AAP based on the physician’s initial assessment and laboratory results. The development of machine learning and artificial intelligence has made this possible. Applying machine learning to emergency triage not only helps improve the accuracy of triage but also reduces the workload of medical staff (18–21). Some studies have attempted to apply machine learning or artificial intelligence to emergency triage of patients with AAP (22–24). However, the clinical indicators included in different studies are often limited by clinical practice experience, and there are also differences in disease assessment standards and hospital preferences for patients seeking treatment. Therefore, the generalization ability of these models in various populations and medical institutions is limited (25, 26). In China, due to the relatively recent implementation of the emergency pre-check triage system, there are limited studies on emergency triage related to AAP, and there is currently no standardized approach. Therefore, to accurately triage patients with AAP and select appropriate treatment strategies, it is crucial to establish a triage prediction model for patients with AAP in our hospital.
In this study, we aimed to construct and optimize a prediction model for AAP triage using a machine learning approach, which is not dependent on imaging diagnosis. To clarify the risky clinical features of AAP patients in terms of the degree of criticality of their condition, so as to achieve accurate triage of patients with AAP.
2 Materials and methods
2.1 Study design
This retrospective study was conducted in accordance with the Declaration of Helsinki regarding the Ethical Principles for Medical Research Involving Human Subjects (27). The study was approved by the Ethical Committee of Wuhan Puren Hospital (MR-42-24-001914), but informed consent was waived due to the study’s retrospective nature.
2.2 Data source
The case information was obtained from the management information system of Wuhan Puren Hospital. The data included the medical records of patients who visited the ED with AAP as their primary complaint between January 1, 2019, and December 31, 2019. A total of 4,323 cases were screened. Epidata version 3.2 was used for data entry. Inclusion criteria: (1) Age ≥ 14 years old; (2) The primary symptom is acute abdominal pain; (3) Complete diagnostic and treatment records with clear diagnosis. Exclusion criteria: (1) Age <14 years old; (2) Patients who did not continue treatment at this hospital and have an unclear diagnosis; (3) Patients who have canceled their appointment; (4) Gynecological acute abdomen, including pelvic inflammatory disease, pelvic mass, torsion of the adnexa, ectopic pregnancy, etc.; (5) Follow-up patients; (6) Patients with more than half of the characteristic variables missing. Based on the nadir criteria, 1911 patient records that did not meet the requirements were removed, and 2,412 patient records that met the criteria were retained for use in this study.
2.3 Key feature variables and outcome indicators
The following alternative characteristic variables were identified according to Hastings and Yew et al. (28, 29)
• Patient demographic characteristics: gender, age, profession;
• Past medical history: hypertension, diabetes, coronary heart disease, stroke, history of abdominal diseases;
• Medical visit details: method of visit, time of visit, body temperature, pulse, respiration, blood pressure, blood oxygen saturation;
• General symptoms and signs of the patient: pale appearance, facial appearance, mental status;
• Abdominal characteristics of the patient: bowel sounds, triggering factors, location of the pain, nausea and vomiting, diarrhea and fever, hematemesis and melena, tenesmus, syncope and consciousness disorders, duration of pain, quality of the pain, pain score, rebound tenderness, and abdominal muscle tension.
The outcome indicator was whether the triage level indicated a critical patient. Critical patients were defined as individuals who arrived at the hospital with a report of critical illness within the past 24 h, had a resuscitation record for the first 24 h after arrival, had a critical value recorded within 24 h, required emergency surgery, and ultimately died. If one of these conditions is met, it is coded as 1; otherwise, it is coded as 0.
2.4 Data analysis and model construction
Univariate analysis was conducted to screen the characteristic variables. The characteristic variables with a significance level of p < 0.05 were selected as independent variables, while the patient’s critical status was used as the dependent variable. Binary logistic regression was used for multifactorial analysis to identify the risk factors associated with the severity of acute abdomen in patients. The characteristic variables identified through logistic regression were then used as input variables for the prediction model. Statistical analyses were performed using SPSS 26.0 software for Windows (SPSS Inc., Chicago, IL, USA). According to the nature of the outcome variable, it can be divided into binary, unordered multicategory, and ordered multicategory data. The chi-square test is used for binary and unordered multicategory data, while Fisher’s exact test is employed when at least one expected frequency is less than five. The Kruskal-Wallis rank sum test is utilized for ordered multicategories. A p-value of less than 0.05 was considered statistically significant.
A sample database was established based on the statistically significant characteristic variables that were screened. The unbiased randomized sample allocation method was used to code the screened sample data based on the attribute division criteria. The training and testing cohorts were randomly and automatically assigned the data in a 4:1 ratio using Python. A total of eight machine learning models, including logistic regression, K-nearest neighbor, support vector machine, kernel function support vector machine, decision tree, random forest, extreme gradient boosting, and artificial neural network, were built. The evaluation metrics for assessing model performance include accuracy, F1-score, recall, and AUC value. The training, validation, and testing of the model are run based on Python 3.8.8, sklearn 1.3.0, tensorflow-gpu 2.4.0, Keras 2.4.0, with an NVIDIA GeForce 1650 GPU. The structure and parameter settings of the ANN model can be found in Figure 1.
3 Results
3.1 Characteristics of study subjects
A total of 2,412 cases were included in this study, of which 568 cases (23.5%) were classified as critically ill, and 1,844 cases (76.5%) were not classified as critically ill. There were 1,210 males (50.2%) and 1,202 females (49.8%). The age range of the patients was 15–95 years old. Among them, there were 850 patients aged 15–35 years old, accounting for 35.2% of the total. There were 1,009 patients aged 35–65 years old, accounting for 41.8%. Lastly, there were 553 patients aged 65–95 years old, accounting for 23.0% of the total. Among the patients with different occupations, students accounted for the smallest proportion of 324 cases (13.4%). Most of these cases were non-critical patients (295 cases). Employees made up the highest percentage of patients at 48.5%, with a total of 1,171 cases. Unemployed and retired individuals fell in the middle of the list, with a total of 917 cases. The results of the univariate analysis revealed significant differences between the two groups of critically ill patients and non-critically ill patients in terms of gender, age, and occupation (p < 0.05). However, no statistically significant differences were observed in the presence of diarrhea and fever (Table 1).
3.2 Multiple logistic regression analysis
The 31 characteristic variables that were found to be statistically significant were included as independent variables in the multifactorial logistic regression analysis. From this analysis, a total of 11 independent risk factors were identified as significantly associated with the dependent variable. These risk factors include the history of diabetes and stroke, pulse, blood pressure, pale appearance, bowel sounds, location of the pain, nausea and vomiting, vomited blood and black stools, quality of the pain, and rebound tenderness (Table 2).
3.3 Performance of prediction models
To predict critical patients with acute abdomen, we constructed eight models, which can be classified into two categories: (1) Traditional machine learning models: Logistic Regression (LR), K-nearest neighbors algorithm (KNN), Support Vector Machine (SVM), Kernel SVM, Decision Tree (DT), Random Forest (RF), and XGBoost; (2) Artificial Neural Network (ANN). We used 80% of the samples (n = 1929) as the training cohort and the remaining 20% (n = 483) as the test set. The remaining 20% of the samples (n = 483) serve as the test set. All eight models were first cross-validated on the training set using a 5-fold cross-validation technique. This process was employed to optimize the hyperparameters of the models. The training set was divided into 5 equal parts. Each time, four parts of the data were used as the training cohort, while the predictive performance of the models was evaluated on the remaining part. In total, five training and testing sessions were conducted to determine the optimal hyperparameters for the model. The accuracy and AUC of the eight models were evaluated using five-fold cross-validation, as shown in Figure 2. Among the eight models, the Artificial Neural Network (ANN) model achieved the highest Area Under the Curve (AUC) value of 0.9877 ± 0.0056 and an average accuracy of 97.67% ± 0.48. Although slightly lower than RF and XGBoost, the ANN model performed exceptionally well.
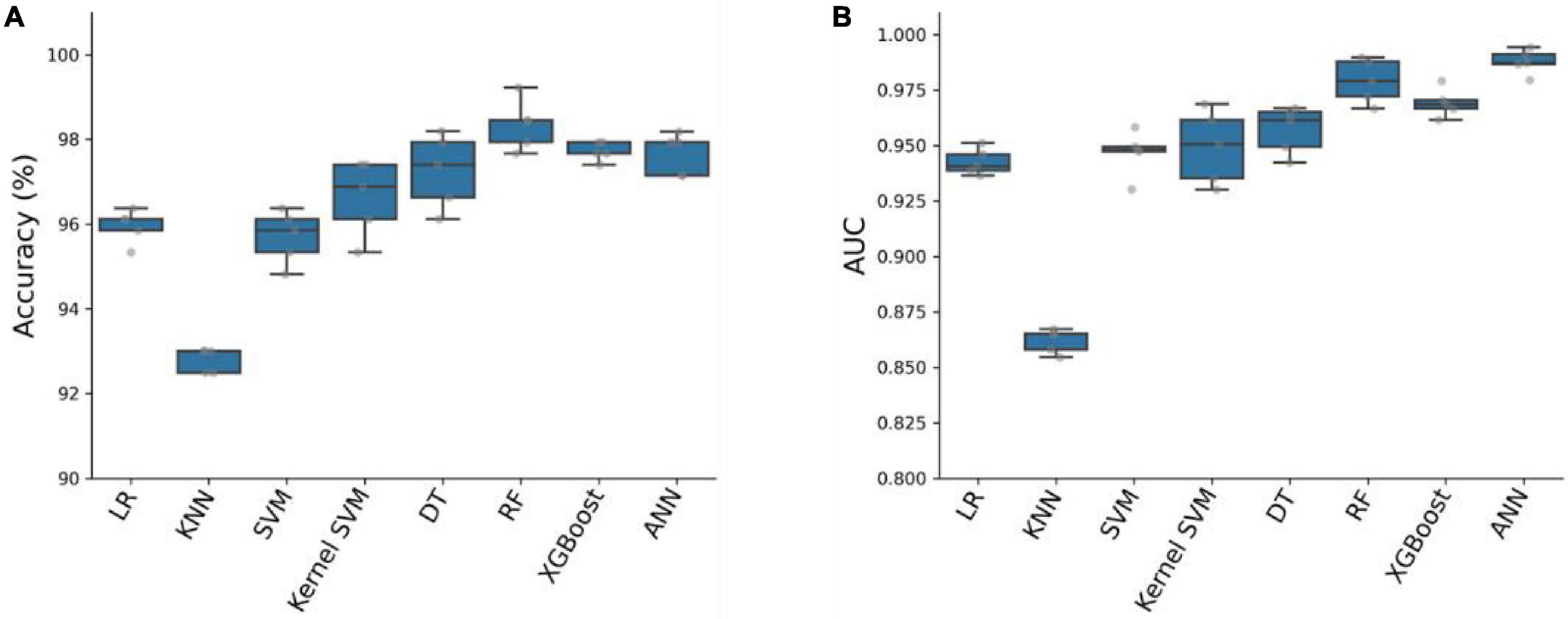
Figure 2. Accuracy (A) and AUC (B) of eight prediction models on the training cohort with five-fold cross-validation.
We trained eight models using the entire training set and evaluated their predictive performance on the test set. The accuracy, AUC, recall, and F1 scores of the models on the training and test sets are shown in Table 3. Among the eight models, the Artificial Neural Network (ANN) achieved the highest Area Under the Curve (AUC) of 0.9972 on the test set (Figure 3). In addition, the Artificial Neural Network (ANN) achieved an accuracy of 97.92% and an F1 score of 0.9793, which were only slightly lower than the highest performing Decision Tree (DT) model. It is worth noting that the AUC of the ANN on the test set only slightly decreases compared to the AUC on the training set, but it is better than all other models. This indicates that the ANN model has better generalization ability compared to the other models, which is important for clinical applications. Overall, the ANN model has better predictive performance.
3.4 Optimization of ANN algorithm prediction model
To further optimize the ANN prediction model, we randomly included a single or a combination of multiple feature variables in the analysis. The results showed that when only a single feature variable was included, the AUC of the ANN model ranged from 0.540 to 0.873. However, when 2–3 variables were included simultaneously, the AUC ranged from 0.599 to 0.905. This suggests that relying solely on clinical feature data of less than 3 variables has significant limitations in AAP triage. In contrast, when we included 11 feature variables obtained from multifactorial logistic regression analyses simultaneously, the AUC reached 0.993. This value was nearly identical to the AUC when all feature variables were included (Table 4). To identify the main factor variables among this group of characteristic variables, various combinations of tests were conducted. The results showed that when including seven variables, history of diabetes and stroke, pulse, blood pressure, pale appearance, and location of the pain, the AUC could reach 0.983. In this situation, we can consider these seven characteristic variables to be significantly important for assessing the severity of AAP.
4 Discussion
Before the widespread availability of medical imaging, the traditional treatment approach depended on the expertise of doctors. They would form their opinions based on the patient’s medical history and physical examination, as well as their own clinical experience (30, 31). In the event of a missed diagnosis or misdiagnosis, it could directly increase the mortality rate (32). To prevent misdiagnosis of critically ill patients with specific conditions, many of them undergo unnecessary surgery (33). Despite the current agreement on the use of CT and ultrasound in AAP, the complexity of AAP still presents a significant challenge for emergency physicians (29, 34–37). Therefore, it is essential to develop a predictive triage system to stratify the risk of AAP patients as accurately as possible (38–40).
In traditional pretest triage, patients are assessed based on age, gender, vital signs, SPO2, consciousness, Glasgow score, blood glucose, and pain (41). However, they are not assessed based on secondary complaints, concomitant symptoms, and past medical history. The advancement of computer-assisted decision-making technology has enabled the assessment of disease risk based solely on clinical information, facilitating rapid triage in the ED. Prediction models exist for common intra-abdominal diseases like appendicitis, pelvic inflammatory disease, and left-sided diverticulitis (42–45). However, there is currently no standardized model for assessing risk and triaging patients with acute abdominal pain (AAP). In this study, we retrospectively analyzed the potential critical risk profiles of patients taking AAP by screening individuals with a comprehensive medical history and clarifying their diagnoses. Our results showed that 11 clinical features, including history of diabetes and stroke, pulse, blood pressure, pale appearance, nausea and vomiting, vomiting of blood and black stools, bowel sounds, location and quality of the pain, and tenderness/rebound tenderness, were strongly correlated with the severity of acute abdomen. The artificial neural network (ANN) model was also effective in predicting the severity of acute abdomen when assessed by combining only seven variables: history of diabetes mellitus, history of stroke, pulse at the time of consultation, blood pressure at the time of consultation, pale appearance, bowel sounds, and site of pain. The three variables of diabetes history, bowel sounds, and pain site were also utilized as key factors in Wang et al.’s risk stratification method for patients with acute appendicitis (46). It is worth mentioning that this method includes more clinical features and laboratory findings. While the method provides an accurate score assessment, it may be somewhat limited in situations where healthcare professionals are initially faced with an urgent claim from an AAP patient. This includes challenges such as the timeliness of laboratory findings and the difficulty in determining the etiology of the disease in some AAP patients, even with laboratory tests (47, 48). In addition, it is important to consider a history of stroke in patients with acute abdominal pain (AAP). These patients may be overlooked during triage because they do not exhibit typical clinical symptoms, despite presenting with abdominal pain. Gastrointestinal symptoms may also trigger central nervous system disorders. For example, Taichi et al. reported a case of acute cerebral infarction caused by colon cancer (49). The determination of the pain site variable aligns with the quadrant partitioning commonly used in most studies (50, 51). Diagnostic imaging and algorithms based on it can facilitate a prompt diagnosis (52, 53). Nevertheless, we need to clarify that in certain special cases, we must consider the potential side effects of radiation exposure, particularly in pregnant patients with AAP (54). However, it is undeniable that laboratory testing and imaging have made an excellent contribution to the management of AAP (55). Sufficient clinical information not only facilitates the interpretation of laboratory results but also helps radiologists make accurate imaging diagnoses (56).
The use of artificial intelligence (AI) in automated agricultural machinery (AAP) can be traced back to the 1970s when it was introduced by Gunn and applied to AAP diagnosis (57). Since then, AI has begun to play a crucial role in the healthcare system and has been consistently optimized. Brejnebøl et al. (58) demonstrated that AI algorithms based on CT scans have benefited the diagnosis of patients with acute appendicitis, albeit with low sensitivity (58). In a recent review, Lam et al. confirmed the significant role of AI in predicting acute appendicitis and emphasized the need for its development in terms of clinical usability (59). It is important to note that artificial intelligence (AI) relies on machine learning, with different algorithmic models producing varying effects. It requires large amounts of data for validation in multiple simulations, making it an exploratory process. For example, three prediction models were developed in a recent study by Henn et al. (60). The tree-based algorithmic model showed the best performance in AAP-assisted decision making. However, even with the incorporation of laboratory test results, its AUC for predicting surgery was only around 0.8. While our model’s performance appears to be strong, it does not necessarily indicate the same level of applicability across different samples. This could be influenced by factors such as sample size, characteristics of the population included, and so on. In addition, it is worth mentioning that AI is not only widely used in the AAP, but also played a crucial role in the 2019 COVID-19 pandemic, helping governments and healthcare workers make timely and accurate judgments, greatly reducing the loss of life and property for people (61).
Although the artificial neural network (ANN) model in this study performs well in predicting APP triage, it still has the following limitations. Firstly, the conclusions drawn from this retrospective study are limited by the available data. Secondly, the time to danger for critically ill patients in this study was defined as 24 h. This definition may focus more on patients with rapidly deteriorating or extremely severe conditions, thereby ignoring those who are equally dangerous but relatively less urgent. It should be noted that due to the limited number of acute abdominal cases related to obstetrics and gynecology in our hospital, it is not representative and therefore excluded from this study. Finally, this was a single-center study, and no additional external validation trials have been conducted.
5 Conclusion
Pre-screening and triaging patients with acute abdominal pain is a major challenge in healthcare. In this study, we developed a machine learning algorithm to construct an AAP triage prediction model, with the artificial neural network (ANN) model demonstrating the best performance. The model can assist clinical staff in promptly and accurately identifying patients at high risk of acute abdomen. This enables them to take timely interventions to reduce the danger, relying solely on seven crucial risk factors, especially in situations with limited medical resources. Although an increasing number of studies have begun to focus on the application of AI in clinical diagnostic decision-making, rigorous scientific validation is necessary to assess its clinical usability.
The future emphasis should be on developing and validating joint analysis and prediction models based on multi-center big data. This will help advance the development and application of outcome prediction and treatment plan prediction models.
Data availability statement
The original contributions presented in this study are included in this article, further inquiries can be directed to the corresponding authors.
Ethics statement
The studies involving humans were approved by the Ethical Committee of Wuhan Puren Hospital. The studies were conducted in accordance with the local legislation and institutional requirements. The ethics committee/institutional review board waived the requirement of written informed consent for participation from the participants or the participants’ legal guardians/next of kin because This was a large retrospective study.
Author contributions
TG: Conceptualization, Supervision, Formal analysis, Funding acquisition, Resources, Writing – original draft. XL: Conceptualization, Writing – original draft, Data curation, Investigation, Validation. RL: Data curation, Investigation, Writing – original draft, Formal analysis, Methodology. JH: Data curation, Formal analysis, Investigation, Methodology, Writing – original draft. DL: Data curation, Formal analysis, Methodology, Writing – original draft, Software, Visualization. WT: Data curation, Methodology, Software, Visualization, Writing – original draft. JS: Methodology, Writing – original draft, Formal analysis, Validation. PC: Formal analysis, Methodology, Validation, Writing – original draft. HS: Conceptualization, Project administration, Supervision, Writing – review and editing. WW: Conceptualization, Project administration, Resources, Supervision, Writing – review and editing.
Funding
The author(s) declare that financial support was received for the research, authorship, and/or publication of this article. This work was supported by the Youth Project of Wuhan Health Commission (WG21Q07) and Wuhan Medical Research Project (WG19A01).
Conflict of interest
HS was also employed by Maintainbiotech Ltd.
The remaining authors declare that the research was conducted in the absence of any commercial or financial relationships that could be construed as a potential conflict of interest.
Publisher’s note
All claims expressed in this article are solely those of the authors and do not necessarily represent those of their affiliated organizations, or those of the publisher, the editors and the reviewers. Any product that may be evaluated in this article, or claim that may be made by its manufacturer, is not guaranteed or endorsed by the publisher.
References
1. Mayumi T, Yoshida M, Tazuma S, Furukawa A, Nishii O, Shigematsu K, et al. The practice guidelines for primary care of acute abdomen 2015. J Gen Fam Med. (2016) 17:5–52.
2. Laméris W, Van Randen A, Van Es HW, van Heesewijk JP, van Ramshorst B, Bouma WH, et al. Imaging strategies for detection of urgent conditions in patients with acute abdominal pain: Diagnostic accuracy study. BMJ. (2009) 338:b2431. doi: 10.1136/bmj.b2431
3. Macaluso C, McNamara R. Evaluation and management of acute abdominal pain in the emergency department. Int J Gen Med. (2012) 5:789. doi: 10.2147/IJGM.S25936
4. de Burlet K, Lam A, Larsen P, Dennett E. Acute abdominal pain-changes in the way we assess it over a decade. N Z Med J Online. (2017) 130:39–44.
5. Ahn SH, Mayo-Smith WW, Murphy BL, Reinert SE, Cronan JJ. Acute nontraumatic abdominal pain in adult patients: Abdominal radiography compared with CT evaluation. Radiology. (2002) 225:159–64. doi: 10.1148/radiol.2251011282
6. Pan H, Li H, Shen Z, Guo H, Zhao Q, Li J. Observation of the effectiveness of a diagnostic model for acute abdominal pain based on the etiology checklist and process thinking. Risk Manag Healthc Policy. (2021) 14:835–45. doi: 10.2147/RMHP.S295142
7. Thijssen WA, van Miero E, Willekens M, Rebel J, Sandel MH, Giesen P, et al. Complaints and diagnoses of emergency department patients in the Netherlands: A comparative study of integrated primary and emergency care. PLoS One. (2015) 10:e0129739. doi: 10.1371/journal.pone.0129739
8. Cervellin G, Mora R, Ticinesi A, Meschi T, Comelli I, Catena F, et al. Epidemiology and outcomes of acute abdominal pain in a large urban emergency department: Retrospective analysis of 5,340 cases. Ann Transl Med. (2016) 4:362. doi: 10.21037/atm.2016.09.10
9. Natesan S, Lee J, Volkamer H, Thoureen T. Evidence-based medicine approach to abdominal pain. Emerg Med Clin. (2016) 34:165–90.
10. Kachalia A, Gandhi TK, Puopolo AL, Yoon C, Thomas EJ, Griffey R, et al. Missed and delayed diagnoses in the emergency department: A study of closed malpractice claims from 4 liability insurers. Ann Emerg Med. (2007) 49:196–205. doi: 10.1016/j.annemergmed.2006.06.035
11. Selbst SM, Friedman MJ, Singh SB. Epidemiology and etiology of malpractice lawsuits involving children in US emergency departments and urgent care centers. Pediatr Emerg Care. (2005) 21:165–9.
13. Mervak BM, Wilson SB, Handly BD, Altun E, Burke LM. MRI of acute appendicitis. J Magn Reson Imaging. (2019) 50:1367–76.
14. de Burlet KJ, Ing AJ, Larsen PD, Dennett ER. Systematic review of diagnostic pathways for patients presenting with acute abdominal pain. Int J Qual Health Care. (2018) 30:678–83.
15. Stacul F, van der Molen AJ, Reimer P, Webb JA, Thomsen HS, Morcos SK, et al. Contrast induced nephropathy: Updated ESUR contrast media safety committee guidelines. Eur Radiol. (2011) 21:2527–41.
16. Ahmad N, Ali AA. Acute appendicitis is still a morbid disease in the developing world. (2023). Available online at: https://pjmhsonline.com/2020/july-sep/537.pdf (accessed November 9, 2023).
17. Trentzsch H, Werner J, Jauch KW. [Acute abdominal pain in the emergency department - a clinical algorithm for adult patients]. Zentralbl Chir. (2011) 136:118–28. doi: 10.1055/s-0031-1271415
18. Xiao Y, Zhang J, Chi C, Ma Y, Song A. Criticality and clinical department prediction of ED patients using machine learning based on heterogeneous medical data. Comput Biol Med. (2023) 165:107390. doi: 10.1016/j.compbiomed.2023.107390
19. Dugas AF, Kirsch TD, Toerper M, Korley F, Yenokyan G, France D, et al. An electronic emergency triage system to improve patient distribution by critical outcomes. J Emerg Med. (2016) 50:910–8.
20. Zhang X, Kim J, Patzer RE, Pitts SR, Patzer A, Schrager JD. Prediction of Emergency department hospital admission based on natural language processing and neural networks. Methods Inf Med. (2017) 56:377–89. doi: 10.3414/ME17-01-0024
21. Kim J, Chang H, Kim D, Jang DH, Park I, Kim K. Machine learning for prediction of septic shock at initial triage in emergency department. J Crit Care. (2020) 55:163–70.
22. Zaboli A, Ausserhofer D, Pfeifer N. Acute abdominal pain in triage: A retrospective observational study of the Manchester triage system’s validity. J Clin Nurs. (2021) 30:942–51. doi: 10.1111/jocn.15635
23. Aaronson EL, Chang Y, Borczuk PA. prediction model to identify patients without a concerning intraabdominal diagnosis. Am J Emerg Med. (2016) 34:1354–8.
24. Farahmand S, Shabestari O, Pakrah M, Hossein-Nejad H, Arbab M, Bagheri-Hariri S. Artificial intelligence-based triage for patients with acute abdominal pain in emergency department; a diagnostic accuracy study. Adv J Emerg Med. (2017) 1:e5. doi: 10.22114/AJEM.v1i1.11
25. Zech JR, Badgeley MA, Liu M, Costa AB, Titano JJ, Oermann EK. Variable generalization performance of a deep learning model to detect pneumonia in chest radiographs: A cross-sectional study. PLoS Med. (2018) 15:e1002683. doi: 10.1371/journal.pmed.1002683
26. Zhang C, Bengio S, Hardt M, Recht B, Vinyals O. Understanding deep learning (still) requires rethinking generalization. Commun ACM. (2021) 64:107–15. doi: 10.1145/3446776
27. Shrestha B, Dunn L. The declaration of Helsinki on medical research involving human subjects: A review of seventh revision. J Nepal Health Res Counc. (2019) 17:548–52. doi: 10.33314/jnhrc.v17i4.1042
28. Yew KS, George MK, Allred HB. Acute abdominal pain in adults: Evaluation and diagnosis. Am Fam Phys. (2023) 107:585–96.
29. Hastings RS, Powers RD. Abdominal pain in the ED: A 35 year retrospective. Am J Emerg Med. (2011) 29:711–6. doi: 10.1016/j.ajem.2010.01.045
30. Manterola C, Vial M, Moraga J, Astudillo P. Analgesia in patients with acute abdominal pain. Cochrane Database Syst Rev. (2011) 1:CD005660.
31. Gans SL, Pols MA, Stoker J, Boermeester MA, Group ES. Guideline for the diagnostic pathway in patients with acute abdominal pain. Dig Surg. (2015) 32:23–31.
32. Chanana L, Jegaraj MA, Kalyaniwala K, Yadav B, Abilash K. Clinical profile of non-traumatic acute abdominal pain presenting to an adult emergency department. J Fam Med Prim Care. (2015) 4:422. doi: 10.4103/2249-4863.161344
33. Liu JL, Wyatt JC, Deeks JJ, Clamp S, Keen J, Verde P, et al. Systematic reviews of clinical decision tools for acute abdominal pain. Clin Gov Int J. (2007) 12:2. doi: 10.1108/cgij.2007.24812cae.002
34. Pandharipande PV, Reisner AT, Binder WD, Zaheer A, Gunn ML, Linnau KF, et al. CT in the emergency department: A real-time study of changes in physician decision making. Radiology. (2016) 278:812–21. doi: 10.1148/radiol.2015150473
35. Barat M, Paisant A, Calame P, Purcell Y, Lagadec M, Curac S, et al. Unenhanced CT for clinical triage of elderly patients presenting to the emergency department with acute abdominal pain. Diagn Interv Imaging. (2019) 100:709–19. doi: 10.1016/j.diii.2019.05.004
36. Lindelius A, Törngren S, Sondén A, Pettersson H, Adami J. Impact of surgeon-performed ultrasound on diagnosis of abdominal pain. Emerg Med J. (2023) 25:52142.
37. Laurell H, Hansson LE, Gunnarsson U. Diagnostic pitfalls and accuracy of diagnosis in acute abdominal pain. Scand J Gastroenterol. (2006) 41:1126–31. doi: 10.1080/00365520600587485
38. Finn JC, Fatovich DM, Arendts G, Mountain D, Tohira H, Williams TA, et al. Evidence-based paramedic models of care to reduce unnecessary emergency department attendance – feasibility and safety. BMC Emerg Med. (2013) 13:13. doi: 10.1186/1471-227X-13-13
39. Vieth TL, Rhodes KV. The effect of crowding on access and quality in an academic ED. Am J Emerg Med. (2006) 24:787–94. doi: 10.1016/j.ajem.2006.03.026
40. Gardner RL, Sarkar U, Maselli JH, Gonzales R. Factors associated with longer ED lengths of stay. Am J Emerg Med. (2007) 25:643–50. doi: 10.1016/j.ajem.2006.11.037
41. Mao S. Guidelines for grading the condition of emergency patients (draft for comments). Chin J Crit Care Med. (2011) 4:2. doi: 10.3877/cma.j.issn.1674-6880.2011.04.006
42. Morishita K, Gushimiyagi M, Hashiguchi M, Stein GH, Tokuda Y. Clinical prediction rule to distinguish pelvic inflammatory disease from acute appendicitis in women of childbearing age. Am J Emerg Med. (2007) 25:152–7. doi: 10.1016/j.ajem.2006.06.013
43. Kharbanda AB, Taylor GA, Fishman SJ, Bachur RG. A clinical decision rule to identify children at low risk for appendicitis. Pediatrics. (2005) 116:709–16.
44. Kulik DM, Uleryk EM, Maguire JL. Does this child have appendicitis? A systematic review of clinical prediction rules for children with acute abdominal pain. J Clin Epidemiol. (2013) 66:95–104. doi: 10.1016/j.jclinepi.2012.09.004
45. Laméris W, van Randen A, van Gulik TM, Busch OR, Winkelhagen J, Bossuyt PM, et al. A clinical decision rule to establish the diagnosis of acute diverticulitis at the emergency department. Dis Colon Rectum. (2010) 53:896–904. doi: 10.1007/DCR.0b013e3181d98d86
46. Wang Y, Zhao H, Zhou Z, Tian C, Xiao HL, Wang BE. Establishment and application of early risk stratification method for acute abdominal pain in adults. Chin Med J (Engl). (2017) 130:530–5. doi: 10.4103/0366-6999.200553
47. Ulenaers M, Buchel OC, Van Olmen A, Moons V, D’Haens G, Christiaens P. An uncommon cause of visceral arterial embolism in patients presenting with acute abdominal pain: A report of 2 cases. Acta Gastro Enterol Belg. (2010) 73:55–60.
48. Yang L, Jia M, Han P. Primary epiploic appendagitis as an unusual cause of acute abdominal pain in a middle-aged male: A case report. Medicine (Baltimore). (2019) 98:e16846. doi: 10.1097/MD.0000000000016846
49. Fujimori T, Kijima T, Honda S, Yamagata S, Makiishi T. A case of acute cerebral infarction with chief complaints of abdominal pain and bloody diarrhoea: The power of a patient-centered inclusive diagnostic team. Cureus. (2022) 14:e27386. doi: 10.7759/cureus.27386
50. Garcia EM, Camacho MA, Karolyi DR, Kim DH, Cash BD, Chang KJ, et al. ACR Appropriateness Criteria® right lower quadrant pain-suspected appendicitis. J Am Coll Radiol. (2018) 15:S373–87.
51. Amari K, Fukumori N, Anzai K, Yamashita S. The diagnostic process for the evaluation of acute abdominal pain by resident trainees in Japan: A cross-sectional study. Intern Med. (2020) 59:1257–65. doi: 10.2169/internalmedicine.3526-19
52. Mills AM, Huckins DS, Kwok H. Diagnostic characteristics of S100A8/A9 in a multicenter study of patients with acute right lower quadrant abdominal pain. Acad Emerg Med. (2012) 19:48–55. doi: 10.1111/j.1553-2712.2011.01259.x
53. Poletti PA, Platon A, De Perrot T, Sarasin F, Andereggen E, Rutschmann O, et al. Acute appendicitis: Prospective evaluation of a diagnostic algorithm integrating ultrasound and low-dose CT to reduce the need of standard CT. Eur Radiol. (2011) 21:2558–66. doi: 10.1007/s00330-011-2212-5
54. Patel SJ, Reede DL, Katz DS, Subramaniam R, Amorosa JK. Imaging the pregnant patient for nonobstetric conditions: Algorithms and radiation dose considerations. RadioGraphics. (2007) 27:1705–22. doi: 10.1148/rg.276075002
55. Panebianco NL, Jahnes K, Mills AM. Imaging and laboratory testing in acute abdominal pain. Emerg Med Clin. (2011) 29:175–93.
56. Hattori S, Yokota H, Takada T. Impact of clinical information on CT diagnosis by radiologist and subsequent clinical management by physician in acute abdominal pain. Eur Radiol. (2021) 31:5454–63. doi: 10.1007/s00330-021-07700-8
57. Gunn AA. The diagnosis of acute abdominal pain with computer analysis. J R Coll Surg. (1976) 21:170–2.
58. Brejnebøl MW, Nielsen YW, Taubmann O, Eibenberger E, Müller FC. Artificial Intelligence based detection of pneumoperitoneum on CT scans in patients presenting with acute abdominal pain: A clinical diagnostic test accuracy study. Eur J Radiol. (2022) 150:110216. doi: 10.1016/j.ejrad.2022.110216
59. Lam A, Squires E, Tan S, Swen NJ, Barilla A, Kovoor J, et al. Artificial intelligence for predicting acute appendicitis: A systematic review. ANZ J Surg. (2023) 93:2070–8. doi: 10.1111/ans.18610
60. Henn J, Hatterscheidt S, Sahu A, Buness A, Dohmen J, Arensmeyer J, et al. Machine learning for decision-support in acute abdominal pain – proof of concept and central considerations. Zentralbl Chir. (2023) 148:376–83. doi: 10.1055/a-2125-1559
Keywords: acute abdomen, clinical features, machine learning, artificial neural networks, logistic regression, prediction models
Citation: Gan T, Liu X, Liu R, Huang J, Liu D, Tu W, Song J, Cai P, Shen H and Wang W (2024) Machine learning based prediction models for analyzing risk factors in patients with acute abdominal pain: a retrospective study. Front. Med. 11:1354925. doi: 10.3389/fmed.2024.1354925
Received: 13 December 2023; Accepted: 15 May 2024;
Published: 05 June 2024.
Edited by:
Arianna Dagliati, University of Pavia, ItalyReviewed by:
Hasan Zulfiqar, University of Electronic Science and Technology of China, ChinaDinh Tuan Phan Le, New York City Health and Hospitals Corporation, United States
Copyright © 2024 Gan, Liu, Liu, Huang, Liu, Tu, Song, Cai, Shen and Wang. This is an open-access article distributed under the terms of the Creative Commons Attribution License (CC BY). The use, distribution or reproduction in other forums is permitted, provided the original author(s) and the copyright owner(s) are credited and that the original publication in this journal is cited, in accordance with accepted academic practice. No use, distribution or reproduction is permitted which does not comply with these terms.
*Correspondence: Hexiao Shen, shenhxmyt@163.com; Wei Wang, zhang19931117w@163.com