- 1The Second Clinical College of Guangzhou University of Chinese Medicine, Guangzhou, Guangdong, China
- 2Guangdong Provincial Hospital of Chinese Medicine, Guangzhou, Guangdong, China
- 3Science and Technology Innovation Center, Guangzhou University of Chinese Medicine, Guangzhou, Guangdong, China
- 4College of Pharmacy, Jinan University, Guangzhou, Guangdong, China
- 5Guangdong Provincial Key Laboratory of Research on Emergency in Traditional Chinese Medicine, Guangzhou, Guangdong, China
Background: Evidence from observational studies and clinical trials suggests that the allergic diseases (ADs) are associated with kidney diseases (KDs). However, the causal association between them remains to be determined. We used bidirectional two-sample Mendelian randomization (MR) analysis to evaluate the potential causality between them.
Methods: Mendelian randomization (MR) was performed using publicly available genome-wide association study (GWAS) summary datasets. Inverse variance weighted (IVW), weighted median, MR-Egger regression, simple mode, and weighted mode methods are used to evaluate the causality between ADs and KDs. Sensitivity and heterogeneity analyses were used to ensure the stability of the results.
Results: The MR results indicated that genetic susceptibility to ADs was associated with a higher risk of CKD [odds ratio (OR) = 1.124, 95% CI = 1.020–1.239, p = 0.019] and unspecified kidney failure (OR = 1.170, 95% CI = 1.004–1.363, p = 0.045) but not with kidney stone, ureter stone or bladder stone (OR = 1.001, 95% CI = 1.000–1.002, p = 0.216), other renal or kidney problem (OR = 1.000, 95% CI = 1.000–1.001, p = 0.339), urinary tract or kidney infection (OR = 1.000, 95% CI = 0.999–1.001, p = 0.604), kidney volume (OR = 0.996, 95% CI = 0.960–1.033, p = 0.812) and cyst of kidney (OR = 0.914, 95% CI = 0.756–1.105, p = 0.354). No causal evidence of KDs on ADs was found in present study.
Conclusion: Results from MR analysis indicate a causal association between ADs and CKD and unspecified kidney failure. These findings partly suggest that early monitoring of CKD risk in patients with ADs is intentional.
Introduction
Allergic diseases (AD) are a category of diseases caused by abnormal immune responses to external environmental stimuli (1) by the body. In recent years, there has been a consistent upward trend (2, 3) in the incidence of these diseases. Common clinical manifestations include allergic rhinitis (4), asthma (5), eczema (6), and atopic dermatitis. The pathogenesis involves genetic factors (7, 8) and immune system abnormalities.
Kidney diseases (KD) constitute a collective term encompassing various types such as chronic kidney disease (CKD), diabetic nephropathy and more (9–14). CKD is a condition characterized by gradual kidney damage (15), often accompanied by a progressive loss of kidney function (16). Epidemiological data indicates that CKD causes 5–10 million deaths annually (17) and the prevalence of kidney stone is as high as 11% in the United States, 9% in Europe (18). CKD is divided into five stages, ranging from mild to end-stage renal disease (ESRD) (19). ESRD presents more severe symptoms (20) and typically necessitates dialysis or kidney transplantation.
Some studies have found a correlation between ADs and KDs. For instance, systemic lupus erythematosus (SLE), as an autoimmune disease, can lead to renal impairment, which is defined as “SLE nephritis (21).” But whether there is a causal relationship between ADs and KDs is still unknown. It is thus important to explore the causal relationship between them.
With the rapid advancement of various omics, including genomics and high-throughput sequencing technologies, biomedical data has shown exponential growth. This has led to the establishment of vast, high-quality databases, ushering in a new era where data drives medical progress. The fusion of big data and artificial intelligence algorithms provides a novel perspective on understanding the causative relationships between diseases and various risk factors. It offers new methods for delving into disease mechanisms. MR analysis is a method based on whole-genome sequencing to assess causality between exposure factors and outcomes (22). It can achieve the same level of evidence as randomized controlled trials (RCT), providing an approach that circumvents potential confounding factors or reverse causation bias (23). It also overcomes the time-consuming, resource-intensive, and costly nature of RCT (24), making it one of the most promising methods for evaluating causality (25).
This study collected publicly available published data and utilized bidirectional two-sample MR analysis to investigate the causality between ADs and KDs.
Materials and methods
Study design
We performed a total of 21 MR analyses to investigate the bidirectional association between ADs and KDs. The analysis primarily satisfies the following three major assumptions based on the characteristics of MR: (1) strong correlation between instrumental variables (IVs) and exposure factors; (2) independence of IVs from confounding factors related to the outcome; (3) IVs are associated with the outcome only through exposure factors (Figure 1). Our analyses are restricted to most of the participants of European descent to minimize racial mismatches.
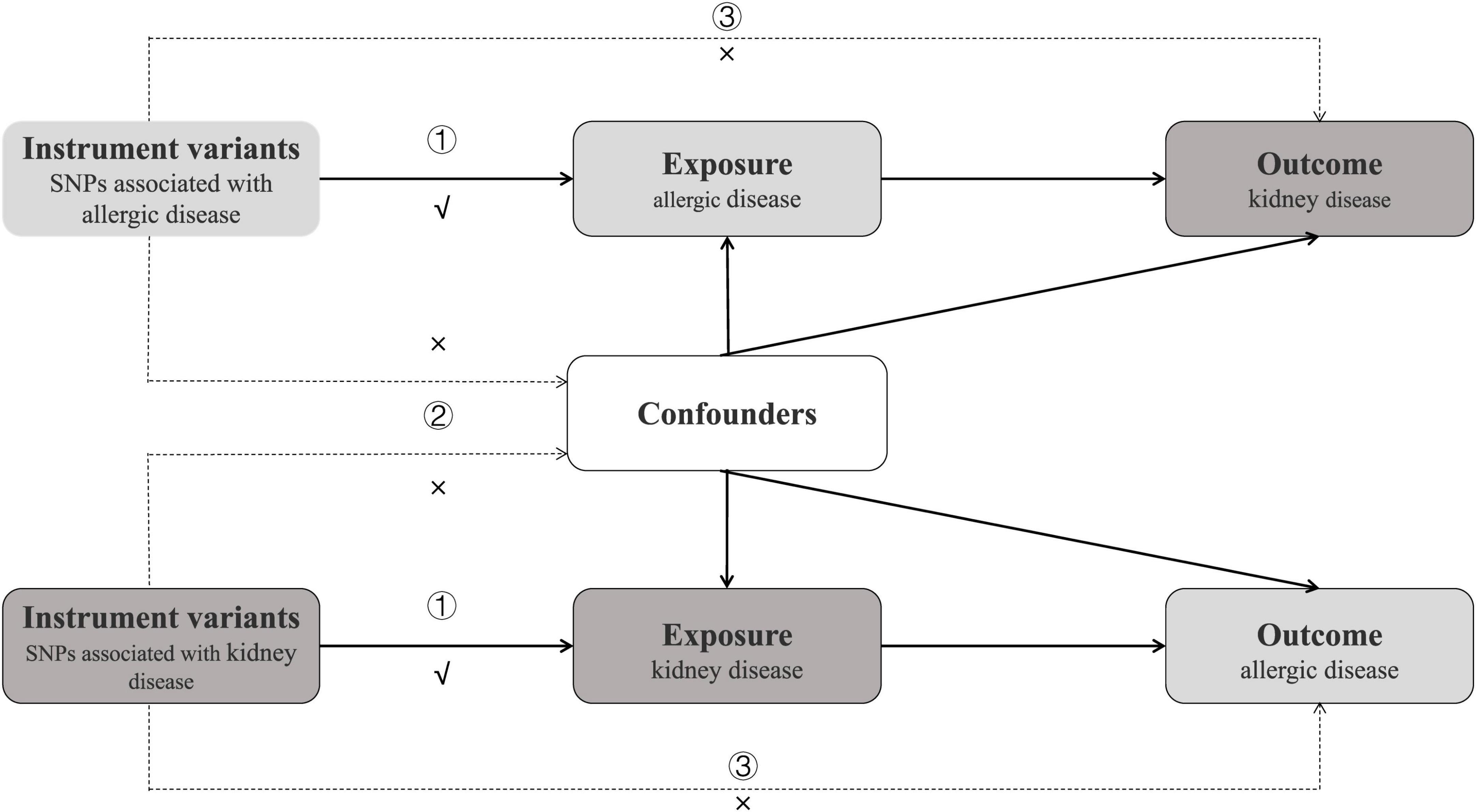
Figure 1. Study design of bidirectional Mendelian randomization study between ADs and 7 kinds of KDs. The instrumental variables meet the three conditions: ① the variables should be highly associated with exposure; ① the variables should be independent of confounding factors of the exposure-outcome association; ② the variables affect the outcome only via the exposure pathway and not through other biological pathways.
Data sources
Summary statistics data for ADs were derived from UK Biobank,1 including 180129 cases and 180709 controls (26). GWAS data for kidney stone, ureter stone or bladder stone (27) were derived from UK Biobank (ebi-a-GCST90038631), including 3,725 cases of European origin and 480,873 controls. Single-nucleotide polymorphisms (SNPs) for other renal or kidney problem (27) were derived from UK Biobank (ebi-a-GCST90038666), including 2,609 cases and 481,989 controls. Summary-level data for urinary tract or kidney infection (27) were derived from UK Biobank that included 2,691 cases and 481,907 controls. Summary statistics for kidney volume (28) were derived from UK Biobank, including 32,860 samples. The summary dataset for cyst of kidney was from FinnGen,2 including 739 cases and 217,185 controls. SNPs for CKD were derived from FinnGen (finn-b-N14_CHRONKIDNEYDIS), including 3,902 cases and 212,841 controls. GWAS data for unspecified kidney failure were derived from FinnGen (finn-b-N14_RENFAILNAS), including 963 cases and 212,841 controls. An overview of the demographics involved in this study is shown in Table 1. The reverse MR analyses used the summary statistics data with ADs as outcome, and KDs as exposure.
Ethics approval
Our analysis used publicly available GWAS summary statistics. No new data were collected, and no new ethical approval was required.
Selection of IVs for MR analyses
Only SNPs associated at genome-wide significance P-value (P < 5 × 10–8) with a minor allele frequency (MAF) greater than 0.01 were considered as potential IVs (29). Independent SNPs were selected according to linkage disequilibrium (LD) (r2 < 0.001, kb = 10,000). At the same time, the strength of IVs is tested by F statistics (F > 10) to ensure that the obtained IVs are strongly related to exposure factors (30). Then, we deleted palindromic SNPs and harmonized exposure-outcome datasets to avoid distortion of strand orientation or allele coding (Figure 2). Finally, we recorded the information of the selected IVs, such as effect allele (EA), beta, standard error (SE), effect allele frequency (eaf) and p-value (Supplementary Table 1).
Two-sample MR analysis
For binary exposures, the casual estimate was presented as an odds ratio (OR) and 95% confidence interval (CI) per log-odds increment in genetically determined risk of the exposures. MR analyses utilized the random-effects inverse-variance weighted (IVW) method as the primary method to estimate the potential bidirectional causal associations between ADs and KDs as it provides a robust causal estimate in the absence of directional pleiotropy (31). Furthermore, we used weighted median, simple mode, weight mode, and MR-Egger (32) methods for alternative analyses. We used MR Egger intercept, MR-PRESSO global test, and Cochran Q statistic to test the presence of heterogeneity or directional pleiotropy. Moreover, visual inspection of the forest plot, funnel plot, and scatter plot were also used to assess the MR “no horizontal pleiotropy” assumption. In addition, sensitivity analysis by applying leave-one-out analysis was conducted. All statistical analyses were performed using the two-sample MR packages in R (version 4.3.1).3 We eliminated the influence of confounding factors by using the Phenoscanner database.4
Reverse MR analyses
To explore whether KDs have any causal impact on ADs, we also performed a reverse MR analysis (i.e., KDs as the exposure and ADs as the outcome) using SNPs that are associated with KDs as IVs.
The threshold selected SNPs less than the genome-wide statistical significance threshold (5 × 10–8) to serve as IVs. Unfortunately, only a few SNPs were selected when we set this condition. To explore more relations between KDs and ADs, we used the second threshold that identified SNPs that were smaller than the genome-wide significance threshold (5 × 10–6) and selected them as the second IVs set to find more potential causality.
GO and KEGG pathway analyses
To collect the potential targets of ADs and KDs, we searched the results from the GeneCards database5 using the keywords “allergic disease,” “kidney disease,” and applied “relevance score > 10” as a screening criterion. The intersection of their targets was obtained using Venny2.1.0.6 To further analyze the potential targets, we imported them into the DAVID database7 for Gene Ontology (GO) and Kyoto Encyclopedia of Genes and Genomes (KEGG) pathway enrichment. The results were visualized by pictures.
Results
SNPs selection
For the first four kinds of KDs (ebi-a-GCST90038631, ebi-a-GCST90038666, ebi-a-GCST90038630, ebi-a-GCST90016670), we found that 1 SNP of the 74 AD-related SNPs was not available in ebi-a-GCST90038631, 2 SNPs were not available in ebi-a-GCST90038630 and 5 SNPs were not available in ebi-a-GCST90016670. For the latter three (finn-b-N14_CYSTKID, finn-b-N14_CHRONKIDNEYDIS, finn-b-N14_RENFAILNAS), we found that 4 SNPs of 72 AD-related SNPs were not available in finn-b-N14_CYSTKID. At the same time, we excluded 6 improper SNPs in finn-b-N14_CHRONKIDNEYDIS and finn-b-N14_RENFAILNAS. In addition, we all excluded 3 palindrome sequences (rs11658582, rs301802, rs760805). Therefore, we finally included 70, 71, 69, 66, 65, 63, and 63 SNPs as IVs to analyze. The F statistics of the IVs were all largely greater than 10 (Supplementary Table 2), indicating no evidence of weak instrument bias was detected. Eventually, after removing pleiotropic SNPs identified by the MR-PRESSO test and the MR-Egger regression, there was no evidence of horizontal pleiotropy of the IVs.
Causality of ADs and 7 KDs
(1) Kidney stone, ureter stone or bladder stone
In the set of IVs (p < 5 × 10–8), we found no genetic liability to ADs that was causally associated with kidney stone, ureter stone or bladder stone (OR = 1.001, 95% CI = 1.000–1.002, p = 0.216, IVW) (Figure 3).
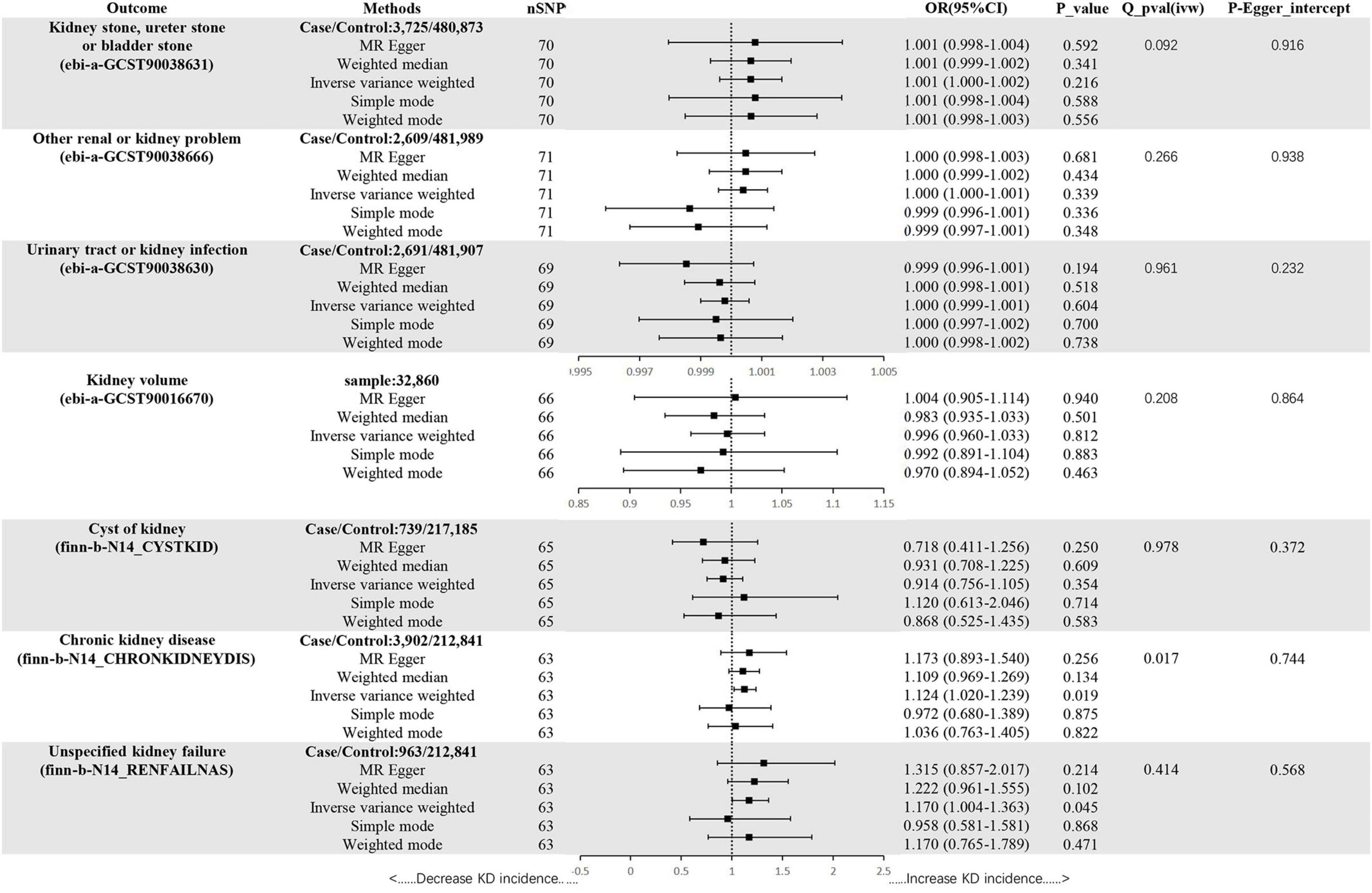
Figure 3. Mendelian randomization estimates of ADs on KDs. SNPs, single-nucleotide polymorphisms; OR, odds ratio; CI, confidence interval; Q, heterogeneity statistic Q.
(2) Other renal or kidney problem
In the set of IVs (p < 5 × 10–8), we found no genetic liability to ADs that was causally associated with other renal or kidney problem (OR = 1.000, 95% CI = 1.000–1.001, p = 0.339, IVW) (Figure 3).
(3) Urinary tract or kidney infection
In the set of IVs (p < 5 × 10–8), we found no genetic liability to ADs that was causally associated with urinary tract or kidney infection (OR = 1.000, 95% CI = 0.999–1.001, p = 0.604, IVW) (Figure 3).
(4) Kidney volume
In the set of IVs (p < 5 × 10–8), we found no genetic liability to ADs that was causally associated with kidney volume (OR = 0.996, 95% CI = 0.960–1.033, p = 0.812, IVW) (Figure 3).
(5) Cyst of kidney
In the set of IVs (p < 5 × 10–8), we found no genetic liability to ADs that was causally associated with cyst of kidney (OR = 0.914, 95% CI = 0.756–1.105, p = 0.354, IVW) (Figure 3).
(6) Chronic kidney disease
In the set of IVs (p < 5 × 10–8), we found ADs were causally associated with CKD (OR = 1.124, 95% CI = 1.020–1.239, p = 0.019, IVW) (Figure 3).
(7) Unspecified kidney failure
In the set of IVs (p < 5 × 10–8), we found ADs were causally associated with unspecified kidney failure (OR = 1.170, 95% CI = 1.004–1.363, p = 0.045, IVW) (Figure 3).
Sensitivity analyses
The MR-Egger, weighted mode, simple mode and weighted median methods yielded similar causal estimates for magnitude and direction (Figure 3). We found no evidence of horizontal pleiotropy for ADs in KDs when using the MR-Egger regression intercept approach. Although a Cochrane Q result (ADs-CKD) showed heterogeneity (p < 0.05), the result of MR-PRESSO revealed no outliers, so we chose IVW as the main method. Other results showed no heterogeneity. It can be seen from the leave-one–out figure that when any SNP is excluded for analysis, the results are stable (Supplementary Figures 1–4).
Reverse-direction MR analyses
To evaluate reverse causality, we used KDs as exposure and ADs as outcome. SNPs associated with KDs extracted from previous GWAS were used as IVs (Supplementary Table 3). We found that 7 KDs were not causally associated with ADs. The relevant analysis results are shown in the Supplementary Table 4.
GO and KEGG pathway analyses
Allergic diseases (ADs) and KDs are strongly influenced by genetic factors, it is suggested that the two diseases may have the same genetic mechanism and biological process. The results of GO and KEGG enrichment analyses indicated that they occurred and developed mainly through targets such as IL-6, ACTN4, TNF and pathways such as PI3k-Akt, AGE-RAGE signaling pathways (Figure 4).
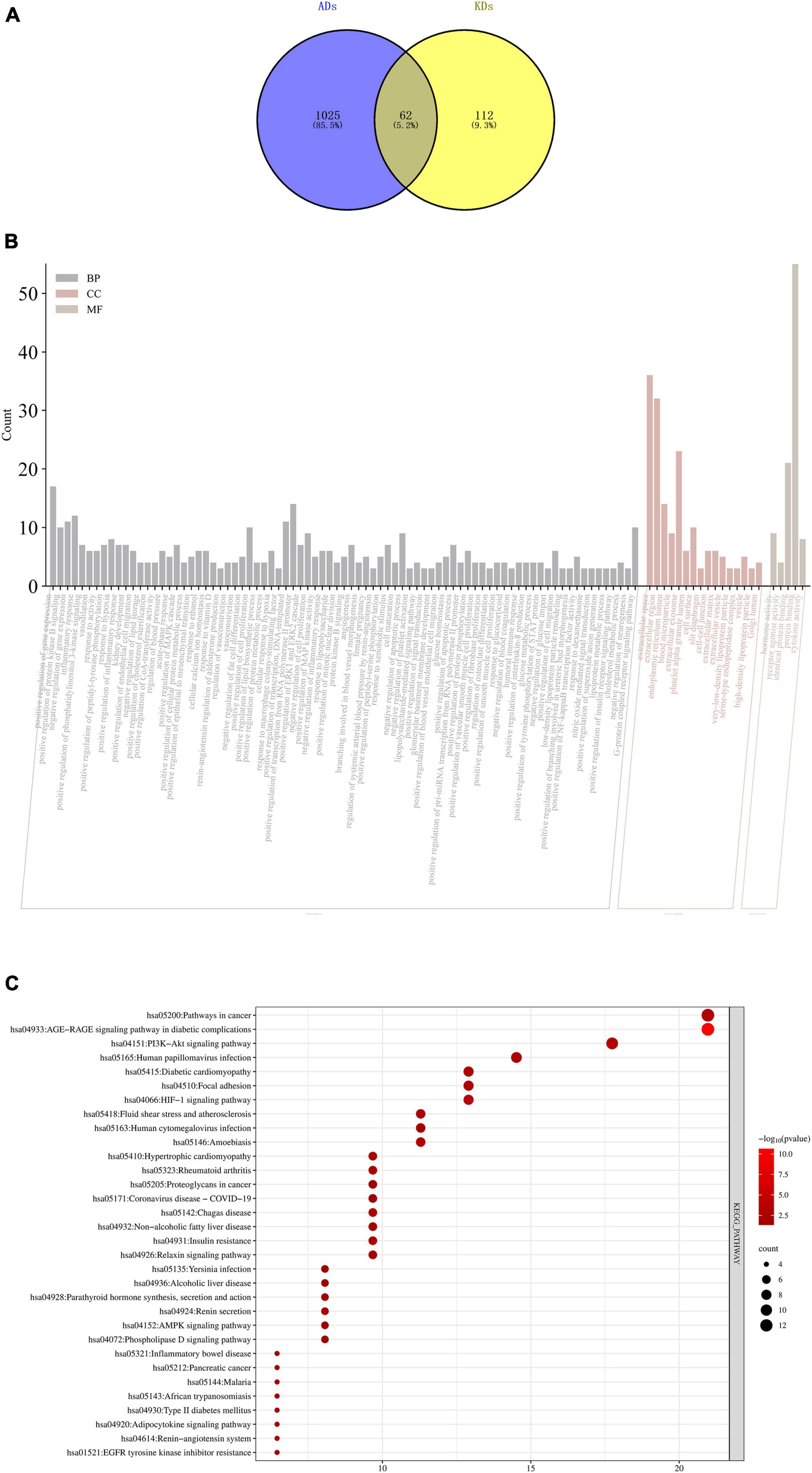
Figure 4. (A) Intersection targets of ADs and KDs; (B) GO enrichment analyses of intersection targets of ADs and KDs. The order from left to right is BP, CC and MF, the X-axis represents the function of GO, and the Y-axis represents the number of genes enriched by GO; (C) KEGG enrichment analyses of intersection targets of ADs and KDs. The X-axis represents the number of genes enriched by KEGG, and the Y-axis represents KEGG signaling pathway. The circle area indicates the number of targets, and the red color from deep to shallow indicates the enrichment degree from low to high. BP, biological process; CC, cell component; MF, molecular function.
Discussion
Allergic diseases (ADs) and KDs are both significant public health problems that have aroused widespread concern in academic community (33, 34). However, the evidence regarding their association remains incomplete. We found that genetically predicted ADs were significantly positively associated with risk of CKD and non-specific renal failure in this study. This relationship demonstrates a unidirectional characteristic, meaning that when KDs are considered as the exposure factor, no association with ADs is found.
The connection between ADs and KDs may manifest in several aspects:
Immune system: Both diseases often trigger the production of autoantibodies within the body, which may target self-tissues or organs (35). For example, the production of anti-nuclear antibodies (ANA) and double-stranded DNA antibodies in SLE is related to disease activity and kidney involvement.
Inflammation: Inflammatory markers in the bloodstream, such as c-reactive protein (CRP) (36) and white blood cell count (37), are common immunological indicators. These markers typically rise during disease activity or exacerbation (38, 39).
Immune cells: Various types of immune cells, including T cells, B cells, monocytes and natural killer cells, play vital roles in both diseases (40, 41).
Immune complexes: Research has shown that the deposition of immune complexes can trigger inflammation and damage to glomeruli, leading to glomerulonephritis.
Cytokines: Cytokines serve as signaling molecules that facilitate interactions between cells and play critical roles in immune responses. Studies have found that tumor necrosis factor (TNF) and interleukin-6 (IL-6) are over-activated in autoimmune diseases and CKD (42).
There is no causality between ADs and other KDs, such as kidney infection and kidney stone. The reasons may be: (1) differences in participants’ age and sample size; (2) weak instrumental variables, pleiotropy, heterogeneity, linkage disequilibrium, population stratification and other factors will affect the accuracy of MR analysis. Therefore, the relationship between these diseases remains to be explored.
Traditional correlation studies, including correlation and regression analyses, are based on established parameter models for statistical analysis. These methods can only infer trends of co-variation between variables and cannot elucidate causative associations. While randomized controlled trials (RCT) are considered the gold standard for causation inference, they are often limited by ethical considerations, feasibility issues related to follow-up, and are difficult to conduct on a large scale. MR analysis effectively facilitates the translation of big data into clinical applications and offers a new perspective for gaining a deeper understanding of diseases and their prevention and treatment (25). It holds significant potential in the field of medical research.
To the best of our knowledge, this is the most comprehensive MR analysis conducted to examine the causality between ADs and KDs. The results of this study are less susceptible to confounding and reverse causation biases, providing a robust remedy for the limitations of traditional observational studies. However, there are fewer SNPs available in reverse MR analyses, the results are less reliable, and a larger sample size data set is needed for verification.
By means of GO and KEGG enrichment analyses, this study preliminarily explored that their similar mechanisms may be related to IL-6, ACTN4, TNF and PI3k-Akt, AGE-RAGE signaling pathways, which pointed out the direction for future basic research on their mechanisms.
Interleukin-6 (IL-6) is involved in regulating inflammatory reaction (43), immune reaction and other biological processes. Previous studies have found that the increase of IL-6 level is related to the severity of asthma (44), allergic rhinitis and other diseases. Therefore, IL-6 and its signaling pathway have become potential targets for the treatment of ADs (45).
Interleukin-6 (IL-6) not only participates in the initiation of inflammatory reaction, but also regulates immune response, including affecting the activation of B cells (antibody-producing cells) and antibody production. In some cases, IL-6 can help regulate the balance of immune system and reduce inflammation.
The role of IL-6 in KDs is equally complex and important, and it participates in the development of various renal pathological states (46), including nephritis, renal fibrosis (47), and CKD. IL-6 promotes the attraction and activation of inflammatory cells, the expression of fibrosis-related factors, which aggravates the structural changes and functional loss of kidney.
ACTN4 (α-actin-4) is a kind of protein found in human body, belonging to α -actin protein family, which mainly plays a role in cytoskeleton composition and intracellular signal transmission. It participates in many intracellular processes, including cell migration, cell division and signal transmission, by cross-linking actin filaments (48).
Although ACTN4 may indirectly affect the processes related to immune response, up to now, the research directly related to ACTN4 and the mechanism of ADs is not sufficient. Future research may reveal more information, especially in the cross field of cell biology and immunology.
In the kidney, ACTN4 is mainly expressed in glomerular podocytes (49). Podocyte is a key component of glomerulus, which helps to maintain the selectivity of filtration barrier and prevent macromolecular substances such as protein from leaking from blood to urine. ACTN4 helps podocytes maintain their morphology and structure, thus maintaining the filtration function of glomerulus.
Tumor necrosis factor (TNF) is a cytokine that plays an important role in human immune system, and it is very important for regulating biological processes such as inflammatory reaction (50), cell proliferation, differentiation and death. ADs are usually caused by excessive immune response in the body. This reaction leads to a large number of IgE antibodies, which in turn activates mast cells and eosinophils and releases inflammatory mediators including histamine, thus causing allergic symptoms. TNF can promote the migration of inflammatory cells, such as eosinophils and neutrophils, to the inflammatory site, aggravate allergic reactions (51), promote the production of cytokines, enhance the activity of immune cells, and lead to a stronger immune response (52).
Under the background of KDs, TNF can be over-expressed as a part of inflammation and immune response, activating inflammatory cells in the kidney, such as macrophages and T cells, leading to inflammatory reaction, aggravating the progress of the disease and affecting renal function (53).
PI3k-Akt signaling pathway is an important signal transduction pathway in cells, which is involved in regulating cell growth, division, survival, metabolism and other biological functions (54). PI3k-Akt pathway plays a key role in regulating the activation and survival of immune cells, especially T cells and B cells. During allergic reaction, the activation of these immune cells is the basis of producing specific IgE antibodies and releasing inflammatory mediators, and the activation of PI3k-Akt pathway is an important regulatory factor in this process (55). PI3k-Akt signaling pathway is involved in regulating the survival, proliferation and release of inflammatory mediators of inflammatory cells, affecting the chemotaxis and migration of immune cells to inflammatory sites, thus aggravating allergic reactions.
PI3k-Akt signaling pathway also plays an important role in KDs, involving the growth, metabolism, repair and protection mechanism of kidney (56). It may not only be a protective mechanism during injury, but also promote the pathological process when the disease progresses. Therefore, the study of this pathway is not only helpful to deeply understand the molecular mechanism of KDs, but also provides a possible target for developing new therapeutic strategies.
AGE-RAGE interaction can cause inflammation and oxidative stress, thus playing a role in the development of KDs, ADs and inflammatory diseases. Some studies show that the activation of AGE-RAGE system is related to the occurrence and development of ADs, such as asthma, allergic rhinitis and eczema. This may be because the activation of AGE-RAGE system can lead to the release of inflammatory mediators, affect the activity of immune cells and promote the occurrence of allergic inflammation. Activation of AGE-RAGE signaling pathway can also lead to the increase of intracellular oxidative stress, thus affecting the physiological function and immune regulation of cells, or promoting the destruction of mucosal barrier, thus aggravating the degree of allergic inflammation.
AGE-RAGE signaling pathway accelerates the progress of KDs (57) by regulating inflammatory reaction, fibrosis process and oxidative stress. Activation of AGE-RAGE signaling pathway can induce inflammatory reactions in renal tubules, interstitium and glomerulus (58), and increase the release of inflammatory factors, such as TNF-α and IL-6. The release of these inflammatory factors will lead to inflammatory cell infiltration and pathological changes in renal tissue. The activation of AGE-RAGE signaling pathway can also promote the fibrosis process of kidney tissue, which leads to the increase of collagen deposition and the proliferation of extracellular matrix, which leads to glomerular sclerosis, interstitial fibrosis and tubulointerstitial scar formation, thus affecting the normal structure and function of kidney.
Limitations of the study
(1) This study primarily involves populations of European descent, and there may be racial differences limiting its generalizability.
(2) There may be variations in age and gender distribution between the populations in the two samples used in this study, which could potentially introduce bias (59).
(3) This study only covers a subset (asthma, hay fever, eczema) of allergic reactions, and future research should include large-scale and abundant data to obtain more comprehensive results.
Conclusion
In summary, we found that genetically predicted ADs was significantly positively associated with risk of CKD and non-specific renal failure. Further exploration of this association can provide insights into the potential biological roles of immune factors in the pathogenesis of KDs. This result guides us to strengthen interdisciplinary cooperation and the monitoring of immune markers in patients with CKD in clinical practice, so as to improve the therapeutic effect and provide strong support for the long-term management and prevention of patients with CKD.
Data availability statement
The original contributions presented in this study are included in this article/Supplementary material, further inquiries can be directed to the corresponding authors.
Ethics statement
Ethical approval was not required for this study involving humans in accordance with the local legislation and institutional requirements. Written informed consent to participate in this study was not required from the participants or the participants’ legal guardians/next of kin in accordance with the national legislation and the institutional requirements.
Authors contributions
ZP: Conceptualization, Data curation, Methodology, Writing – original draft. XD: Investigation, Methodology, Software, Writing – original draft. YL: Formal Analysis, Methodology, Writing – original draft. ZL: Writing – original draft. YW: Writing – original draft. WZ: Supervision, Writing – review & editing. BD: Supervision, Writing – review & editing.
Funding
The authors declare that no financial support was received for the research, authorship, and/or publication of this article.
Acknowledgments
We thank the participants of all GWAS cohorts included in the present study and the investigators of the IEU Open GWAS project, UK Biobank, FINNGEN for sharing the GWAS summary statistics.
Conflict of interest
The authors declare that the research was conducted in the absence of any commercial or financial relationships that could be construed as a potential conflict of interest.
Publisher’s note
All claims expressed in this article are solely those of the authors and do not necessarily represent those of their affiliated organizations, or those of the publisher, the editors and the reviewers. Any product that may be evaluated in this article, or claim that may be made by its manufacturer, is not guaranteed or endorsed by the publisher.
Supplementary material
The Supplementary Material for this article can be found online at: https://www.frontiersin.org/articles/10.3389/fmed.2024.1347152/full#supplementary-material
Abbreviations
AD, allergic disease; CI, confidence interval; CKD, chronic kidney disease; EA, effect allele; eaf, effect allele frequency; ESRD, end-stage renal disease; GWAS, genome-wide association study; IV, instrument variable; KD, kidney disease; LD, linkage disequilibrium; MAF, minor allele frequency; MR, Mendelian randomization; OR, odds ratio, RCT, randomized controlled trial; SE, standard error; SNP, single-nucleotide polymorphism.
Footnotes
- ^ http://www.ukbiobank.ac.uk/
- ^ https://r9.finngen.fi/
- ^ www.r-project.org/
- ^ http://www.phenoscanner.medschl.cam.ac.uk/
- ^ http://www.genecards.org
- ^ https://bioinfogp.cnb.csic.es/tools/venny
- ^ david.ncifcrf.gov
References
1. Lemiere C, Lavoie G, Doyen V, Vandenplas O. Irritant-induced asthma. J Allergy Clin Immunol Pract. (2022) 10:2799–806. doi: 10.1016/j.jaip.2022.06.045
2. Eigenmann P. Diagnosis of allergy syndromes: Do symptoms always mean allergy? Allergy. (2005) 60(Suppl. 79):6–09. doi: 10.1111/j.1398-9995.2005.00850.x
3. Zhang Y, Lan F, Zhang L. Advances and highlights in allergic rhinitis. Allergy. (2021) 76:3383–9. doi: 10.1111/all.15044
4. Bousquet J, Anto J, Bachert C, Baiardini I, Bosnic-Anticevich S, Walter C, et al. Allergic rhinitis. Nat Rev Dis Prim. (2020) 6:95. doi: 10.1038/s41572-020-00227-0
5. Papadopoulos N, Miligkos M, Xepapadaki PA. Current perspective of allergic asthma: From mechanisms to management. Handb Exp Pharmacol. (2022) 268:69–93. doi: 10.1007/164_2021_483
6. Wang J, Zhao Z, Zhang Y, Li B, Huang C, Zhang X, et al. Asthma, allergic rhinitis and eczema among parents of preschool children in relation to climate, and dampness and mold in dwellings in China. Environ Int. (2019) 130:104910. doi: 10.1016/j.envint.2019.104910
7. Gough H, Grabenhenrich L, Reich A, Eckers N, Nitsche O, Schramm D, et al. Allergic multimorbidity of asthma, rhinitis and eczema over 20 years in the German birth cohort MAS. Pediatr Allergy Immunol. (2015) 26:431–7. doi: 10.1111/pai.12410
8. Sroka-Tomaszewska J, Trzeciak M. Molecular mechanisms of atopic dermatitis pathogenesis. Int J Mol Sci. (2021) 22:4130. doi: 10.3390/ijms22084130
9. Perazella M, Markowitz G. Drug-induced acute interstitial nephritis. Nat Rev Nephrol. (2010) 6:461–70. doi: 10.1038/nrneph.2010.71
10. Pietrucha-Dilanchian, P, Hooton T. Diagnosis, treatment, and prevention of urinary tract infection. Microbiol Spectr. (2016) 4:6. doi: 10.1128/microbiolspec.UTI-0021-2015
11. Siener R. Nutrition and kidney stone disease. Nutrients. (2021) 13:1917. doi: 10.3390/nu13061917
12. Evan A, Worcester E, Coe F, Williams J, Lingeman J. Mechanisms of human kidney stone formation. Urolithiasis. (2015) 43(Suppl. 1):19–32. doi: 10.1007/s00240-014-0701-0
13. Sakhaee K, Maalouf N, Sinnott B. Clinical review. Kidney stones 2012: Pathogenesis, diagnosis, and management. J Clin Endocrinol Metab. (2012) 97:1847–60. doi: 10.1210/jc.2011-3492
14. Katabathina V, Kota G, Dasyam A, Shanbhogue A, Prasad S. Adult renal cystic disease: A genetic, biological, and developmental primer. Radiographics. (2010) 30:1509–23. doi: 10.1148/rg.306105513
15. Drawz P, Rahman M. Chronic kidney disease. Ann Intern Med. (2015) 162:ITC1–16. doi: 10.7326/AITC201506020
16. Wei W, Zhao Y, Zhang Y, Jin H, Shou S. The role of IL-10 in kidney disease. Int Immunopharmacol. (2022) 108:108917. doi: 10.1016/j.intimp.2022.108917
17. Rasheed H, Zheng J, Rees J, Sanderson E, Thomas L, Richardson T, et al. The causal effects of serum lipids and apolipoproteins on kidney function: Multivariable and bidirectional Mendelian-randomization analyses. Int J Epidemiol. (2021) 50:1569–79. doi: 10.1093/ije/dyab014
18. Shen X, Chen Y, Chen Y, Liang H, Li G, Hao Z. Is the METS-IR index a potential new biomarker for kidney stone development? Front Endocrinol (Lausanne). (2022) 13:914812. doi: 10.3389/fendo.2022.914812
19. Kennedy O, Pirastu N, Poole R, Fallowfield J, Hayes P, Grzeszkowiak E, et al. Coffee consumption and kidney function: A Mendelian randomization study. Am J Kidney Dis. (2020) 75:753–61. doi: 10.1053/j.ajkd.2019.08.025
20. Kim B, King B, Vrtiska T, Irazabal M, Torres V, Harris P. Inherited renal cystic diseases. Abdom Radiol. (2016) 41:1035–51. doi: 10.1007/s00261-016-0754-3
21. Anders H, Saxena R, Zhao M, Parodis I, Salmon J, Mohan C. Lupus nephritis. Nat Rev Dis Prim. (2020) 6:7. doi: 10.1038/s41572-019-0141-9
22. Bowden J, Holmes M. Meta-analysis and Mendelian randomization: A review. Res Synth Methods. (2019) 10:486–96. doi: 10.1002/jrsm.1346
23. Smith G, Ebrahim S. ‘Mendelian randomization’: Can genetic epidemiology contribute to understanding environmental determinants of disease? Int J Epidemiol. (2003) 32:1–22. doi: 10.1093/ije/dyg070
24. Sekula P, Del G, Pattaro C, Köttgen A. Mendelian randomization as an approach to assess causality using observational data. J Am Soc Nephrol. (2016) 27:3253–65. doi: 10.1681/ASN.2016010098
25. Birney E. Mendelian randomization. Cold Spring Harb Perspect Med. (2022) 12:a041302. doi: 10.1101/cshperspect.a041302
26. Ferreira M, Vonk J, Baurecht H, Marenholz I, Tian C, Hoffman J, et al. Shared genetic origin of asthma, hay fever and eczema elucidates allergic disease biology. Nat Genet. (2017) 49:1752–7. doi: 10.1038/ng.3985
27. Dönertaş H, Fabian D, Valenzuela M, Partridge L, Thornton J. Common genetic associations between age-related diseases. Nat Aging. (2021) 1:400–12. doi: 10.1038/s43587-021-00051-5
28. Liu Y, Basty N, Whitcher B, Bell J, Sorokin E, van Bruggen N, et al. Genetic architecture of 11 organ traits derived from abdominal MRI using deep learning. Elife. (2021) 10:e65554. doi: 10.7554/eLife.65554
29. Huang W, Xiao J, Ji J, Chen L. Association of lipid-lowering drugs with COVID-19 outcomes from a Mendelian randomization study. Elife. (2021) 10:e73873. doi: 10.7554/eLife.73873
30. Hemani G, Zheng J, Elsworth B, Wade K, Haberland V, Baird D, et al. The MR-Base platform supports systematic causal inference across the human phenome. Elife. (2018) 7:e34408. doi: 10.7554/eLife.34408
31. Gao N, Kong M, Li X, Wei D, Zhu X, Hong Z, et al. Systemic lupus erythematosus and cardiovascular disease: A Mendelian randomization study. Front Immunol. (2022) 13:908831. doi: 10.3389/fimmu.2022.908831
32. Burgess S, Thompson S. Interpreting findings from Mendelian randomization using the MR-Egger method. Eur J Epidemiol. (2017) 32:377–89. doi: 10.1007/s10654-017-0255-x
33. Matasar M, Neugut A. Epidemiology of anaphylaxis in the United States. Curr Allergy Asthma Rep. (2003) 3:30–5. doi: 10.1007/s11882-003-0007-8
34. Siddiqui Z, Walker A, Pirwani M, Tahiri M, Syed I. Allergic rhinitis: Diagnosis and management. Br J Hosp Med (Lond). (2022) 83:1–09. doi: 10.12968/hmed.2021.0570
35. Place D, Kanneganti T. The innate immune system and cell death in autoinflammatory and autoimmune disease. Curr Opin Immunol. (2020) 67:95–105. doi: 10.1016/j.coi.2020.10.013
36. Machowska A, Carrero J, Lindholm B, Stenvinkel P. Therapeutics targeting persistent inflammation in chronic kidney disease. Transl Res. (2016) 167:204–13. doi: 10.1016/j.trsl.2015.06.012
37. Messerer D, Halbgebauer R, Nilsson B, Pavenstädt H, Radermacher P, Huber-Lang M. Immunopathophysiology of trauma-related acute kidney injury. Nat Rev Nephrol. (2021) 17:91–111. doi: 10.1038/s41581-020-00344-9
38. Wang Y, Shaw K, Zhang G, Chung E, Hu M, Cao Q, et al. Interleukin-33 exacerbates IgA glomerulonephritis in transgenic mice overexpressing B cell activating factor. J Am Soc Nephrol. (2022) 33:966–84. doi: 10.1681/ASN.2021081145
39. Fu Y, Xiang Y, Li H, Chen A, Dong Z. Inflammation in kidney repair: Mechanism and therapeutic potential. Pharmacol Ther. (2022) 237:108240. doi: 10.1016/j.pharmthera.2022.108240
40. Sato Y, Yanagita M. Immune cells and inflammation in AKI to CKD progression. Am J Physiol Renal Physiol. (2018) 315:F1501–12. doi: 10.1152/ajprenal.00195.2018
41. Saez A, Herrero-Fernandez B, Gomez-Bris R, Sánchez-Martinez H, Gonzalez-Granado J. Pathophysiology of inflammatory bowel disease: Innate immune system. Int J Mol Sci. (2023) 24:1526. doi: 10.3390/ijms24021526
42. Speer T, Dimmeler S, Schunk S, Fliser D, Ridker P. Targeting innate immunity-driven inflammation in CKD and cardiovascular disease. Nat Rev Nephrol. (2022) 18:762–78. doi: 10.1038/s41581-022-00621-9
43. Ishihara K, Hirano T. IL-6 in autoimmune disease and chronic inflammatory proliferative disease. Cytokine Growth Factor Rev. (2002) 13:357–68. doi: 10.1016/s1359-6101(02)00027-8
44. Schmit T, Ghosh S, Mathur R, Barnhardt T, Ambigapathy G, Wu M, et al. IL-6 deficiency exacerbates allergic asthma and abrogates the protective effect of allergic inflammation against streptococcus pneumoniae pathogenesis. J Immunol. (2020) 205:469–79. doi: 10.4049/jimmunol.1900755
45. Namakanova O, Gorshkova E, Zvartsev R, Nedospasov S, Drutskaya M, Gubernatorova E. Therapeutic potential of combining IL-6 and TNF blockade in a mouse model of allergic asthma. Int J Mol Sci. (2022) 23:3521. doi: 10.3390/ijms23073521
46. Koshino A, Schechter M, Sen T, Vart P, Neuen B, Neal B, et al. Interleukin-6 and cardiovascular and kidney outcomes in patients with type 2 diabetes: New insights from CANVAS. Diabetes Care. (2022) 45:2644–52. doi: 10.2337/dc22-0866
47. Guo X, Zhu Y, Sun Y, Li X. IL-6 accelerates renal fibrosis after acute kidney injury via DNMT1-dependent FOXO3a methylation and activation of Wnt/beta-catenin pathway. Int Immunopharmacol. (2022) 109:108746. doi: 10.1016/j.intimp.2022.108746
48. Feng D, Kumar M, Muntel J, Gurley S, Birrane G, Stillman I, et al. Phosphorylation of ACTN4 leads to podocyte vulnerability and proteinuric glomerulosclerosis. J Am Soc Nephrol. (2020) 31:1479–95. doi: 10.1681/ASN.2019101032
49. Dai S, Wang Z, Pan X, Wang W, Chen X, Ren H, et al. Functional analysis of promoter mutations in the ACTN4 and SYNPO genes in focal segmental glomerulosclerosis. Nephrol Dial Transplant. (2010) 25:824–35. doi: 10.1093/ndt/gfp394
50. Lechner A, Henkel F, Hartung F, Bohnacker S, Alessandrini F, Gubernatorova E, et al. Macrophages acquire a TNF-dependent inflammatory memory in allergic asthma. J Allergy Clin Immunol. (2022) 149:2078–90. doi: 10.1016/j.jaci.2021.11.026
51. Li X, Chen X, Gu W, Guo Y, Cheng Y, Peng J, et al. Impaired TNF/TNFR2 signaling enhances Th2 and Th17 polarization and aggravates allergic airway inflammation. Am J Physiol Lung Cell Mol Physiol. (2017) 313:L592–601. doi: 10.1152/ajplung.00409.2016
52. Xue K, Ruan L, Hu J, Fu Z, Tian D, Zou W. Panax notoginseng saponin R1 modulates TNF-alpha/NF-kappaB signaling and attenuates allergic airway inflammation in asthma. Int Immunopharmacol. (2020) 88:106860. doi: 10.1016/j.intimp.2020.106860
53. Mehaffey E, Majid D. Tumor necrosis factor-alpha, kidney function, and hypertension. Am J Physiol Renal Physiol. (2017) 313:F1005–8. doi: 10.1152/ajprenal.00535.2016
54. Wang S, Wuniqiemu T, Tang W, Teng F, Bian Q, Yi L, et al. Luteolin inhibits autophagy in allergic asthma by activating PI3K/Akt/mTOR signaling and inhibiting beclin-1-PI3KC3 complex. Int Immunopharmacol. (2021) 94:107460. doi: 10.1016/j.intimp.2021.107460
55. Ma B, Athari S, Mehrabi N, Zhao L. PI3K/AKT/mTOR and TLR4/MyD88/NF-kappaB signaling inhibitors attenuate pathological mechanisms of allergic asthma. Inflammation. (2021) 44:1895–907. doi: 10.1007/s10753-021-01466-3
56. Xu Z, Jia K, Wang H, Gao F, Zhao S, Li F, et al. METTL14-regulated PI3K/Akt signaling pathway via PTEN affects HDAC5-mediated epithelial-mesenchymal transition of renal tubular cells in diabetic kidney disease. Cell Death Dis. (2021) 12:32. doi: 10.1038/s41419-020-03312-0
57. Qiu Y, Tang L, Wei W. Berberine exerts renoprotective effects by regulating the AGEs-RAGE signaling pathway in mesangial cells during diabetic nephropathy. Mol Cell Endocrinol. (2017) 443:89–105. doi: 10.1016/j.mce.2017.01.009
58. Hong J, Li G, Zhang Q, Ritter J, Li W, Li PLD-. Ribose induces podocyte NLRP3 inflammasome activation and glomerular injury via AGEs/RAGE pathway. Front Cell Dev Biol. (2019) 7:259. doi: 10.3389/fcell.2019.00259
Keywords: allergic diseases, kidney diseases, Mendelian randomization, causality, reverse-direction, GWAS
Citation: Peng Z, Dong X, Long Y, Li Z, Wang Y, Zhu W and Ding B (2024) Causality between allergic diseases and kidney diseases: a two-sample Mendelian randomization study. Front. Med. 11:1347152. doi: 10.3389/fmed.2024.1347152
Received: 07 December 2023; Accepted: 29 February 2024;
Published: 12 March 2024.
Edited by:
Gian Marco Ghiggeri, Giannina Gaslini Institute (IRCCS), ItalyReviewed by:
Lu Liu, Temple University, United StatesMaría Isabel Delgado Dolset, Universidad CEU San Pablo, Spain
Copyright © 2024 Peng, Dong, Long, Li, Wang, Zhu and Ding. This is an open-access article distributed under the terms of the Creative Commons Attribution License (CC BY). The use, distribution or reproduction in other forums is permitted, provided the original author(s) and the copyright owner(s) are credited and that the original publication in this journal is cited, in accordance with accepted academic practice. No use, distribution or reproduction is permitted which does not comply with these terms.
*Correspondence: Wei Zhu, zhuwei9201@163.com; Banghan Ding, banghanding@139.com