- 1Department of Ontology, Affiliated Hospital of Guangdong Medical University, Zhanjiang, China
- 2Department of Geriatrics, Affiliated Hospital of Guangdong Medical University, Zhanjiang, China
- 3Department of Cardiology, Affiliated Hospital of Guangdong Medical University, Zhanjiang, China
Objectives: Previous observational studies have reported a close association between socioeconomic status and pulmonary disease-related morbidity. However, the inherent causal effects remain unclear. Therefore, this bidirectional Mendelian randomization (MR) study aimed to identify the causal relationship between household income and genetic susceptibility to pulmonary diseases.
Methods: An MR study was conducted on a large cohort of European individuals, using publicly available genome-wide association study datasets using a random-effects inverse-variance weighting model as the main standard. Simultaneously, MR-Egger regression, weighted median, and maximum likelihood estimation were applied as supplements. Sensitivity analysis, comprising a heterogeneity test and horizontal pleiotropy test, was performed using the Cochran’s Q, MR-Egger intercept, and MR-PRESSO tests to ensure the reliability of the conclusion.
Results: A higher household income tended to lower the risk of genetic susceptibility to chronic obstructive pulmonary disease (COPD, OR: 0.497, 95% CI = 0.337–0.733, p < 0.001), asthma (OR: 0.687, 95% CI = 0.540–0.876, p = 0.002), and lung cancer (OR: 0.569, 95% CI = 0.433–0.748, p < 0.001), and further indicated potential causality with pneumonia (OR: 0.817; 95% CI = 0.686–0.972, p = 0.022). No association was evident with COVID-19 (OR: 0.934, 95% CI = 0.764–1.142, p = 0.507), tuberculosis (OR: 0.597, 95% CI = 0.512–1.189, p = 0.120), or bronchiectasis (OR: 0.680, 95% CI = 0.311–1.489, p = 0.400). Reverse MR analysis suggested no reverse causal relationship between pulmonary disease and household income status, while sensitivity analysis verified the reliability of the results.
Conclusion: The results revealed that the population with a higher household income tended to have a lower risk of genetic susceptibility to COPD, asthma, and lung cancer.
1 Introduction
The 2017 Global Burden of Disease Study reported that chronic pulmonary diseases are among the primary causes of mortality, impacting approximately 600 million individuals globally, with four million dying prematurely from these diseases. Compared to the results obtained in 1990, the morbidity and mortality rates have increased by 39.8 and 18.0%, respectively (1). Chronic obstructive pulmonary disorder (COPD), asthma, acute respiratory infections (especially the coronavirus disease 2019 [COVID-19] pandemic), and lung cancer are the most common pulmonary diseases which together place a significant financial burden on global public health. Numerous observational studies and meta-analyses have shown that socioeconomic status is closely linked to pulmonary disease-related morbidity (2). For example, one repeated cross-sectional analysis of 160,495 participants spanning half a century in America reported that socioeconomic disparities in pulmonary health have persisted, and potentially worsened over the past six decades. Socioeconomic status can serve as an independent determinant of pulmonary health (3), a perspective which was also supported by independent studies conducted in China and Europe (4, 5). Nevertheless, observational studies have limitations, such as the absence of randomization, potential confounding factors, challenges in controlling variables, and an inability to establish causality due to unaccounted factors that may bias the outcomes. Thus, additional research is necessary to gain a deeper understanding of this relationship.
Mendelian randomization (MR) utilizes valuable genetic variants, such as single nucleotide polymorphisms (SNPs), as instrumental variables (IVs) to evaluate the causal effects between modifiable, non-genetic exposure factors and genetic susceptibility to diseases. This type of analysis is grounded in Mendel’s law of inheritance, which explains the random allocation of genetic variants during meiosis (6). In the absence of randomized controlled trials (RCTs), MR studies offer an alternative approach for causal inference, as genetic variants are randomly assigned during meiosis, thus mimicking the randomization in the RCT process. Compared to traditional observational studies, the main advantage of MR studies is that they are less likely to be influenced by unmeasured confounding factors as genetic variants are identified at the time of conception (7, 8). MR studies have successfully elucidated causal relationships between behavioral exposure, education, socioeconomic conditions, and diseases (9–11). Therefore, the aim of this study was to identify a bidirectional causal relationship between household income and genetic susceptibility to common pulmonary diseases using MR.
2 Materials and methods
2.1 Study design and information of GWAS datasets
To achieve impartial results, an MR study depends on three fundamental assumptions: (1) the selected genetic IVs must be significantly associated with the exposure factor; (2) the IVs should be independent of potential confounders associated with exposure factors and outcomes; and (3) the IVs should affect the outcomes only through the exposure factor (7).
This study was based on a large sample cohort of European individuals extracted from publicly available genome-wide association study (GWAS) datasets. The variable genetic information used in this study was extracted from the Integrative Epidemiology Unit (IEU) GWAS database1 (12), a publicly available GWAS summary database. The GWAS summary dataset contains data on the “average total household income before tax,” encompassing the household income status of 397,751 samples originally from the UK biobank database. Annual household income was divided into five intervals: >100,000 (in pounds), 52,000–100,000, 31,000–51,999, 18,000–30,999, and <18,000. Pulmonary diseases encompass a range of conditions, including chronic obstructive pulmonary disease (COPD), asthma, tuberculosis, bronchiectasis, COVID-19, pneumonia, and lung cancer. Amongst which, the data of COPD, asthma, tuberculosis, bronchiectasis, and pneumonia were collected from the FinnGen consortium. The cases were identified using the International Classification of Diseases (ICD). For further information, please refer to https://r10.risteys.finngen.fi/. For COVID-19 cases, individuals must have laboratory-confirmed SARS-CoV-2 infection (RNA and/or serology-based), EHR/ICD coding indicating COVID-19, or self-reporting of a positive COVID-19 test (e.g., via questionnaire). We followed sample size and timeliness priorities to make the best possible choice. Detailed information on all the GWAS datasets is listed in Table 1. The GWAS datasets of household income status and pulmonary diseases were originated from different consortiums to decrease potential bias caused by sample overlap. In addition, to minimize racial mismatches, all GWAS datasets involved in this study majorly enrolled populations of European ancestry. This study involved 14 times MR analyses to explore the bidirectional association between annual household income status and seven different pulmonary diseases.
2.2 Selection criteria for IVs
SNPs filtered according to the three pivotal MR assumptions were used as IVs. First, SNPs were matched using a genome-wide statistical significance threshold (p < 5 × 10−8). Second, the corresponding linkage disequilibrium state, as well as the independence of these SNPs in the linkage disequilibrium state and the independence of these SNPs within a 0–10,000 kb window at a threshold of r2 < 0.001. Third, to evaluate the assumption that the IVs affect the outcomes only through the exposure factor, potential phenotypes that may be relevant to the IVs were investigated by searching the human genotype–phenotype association database (PhenoScanner-V2, http://www.phenoscanner.medschl.cam.ac.uk/) (16). Fourth, the SNPs identified as IVs were further matched with those in the outcome GWAS dataset to establish genetic associations. The summary SNP phenotype and outcome statistics were harmonized to ensure effect size alignment, while palindromic SNPs were excluded. Finally, F-statistics (>10) were applied to evaluate the strength of the IVs to avoid the influence of weak instrumental bias (17).
2.3 Mendelian randomization study and sensitivity analysis
The MR study was performed using a random-effects inverse-variance weighting (IVW) model (18) as the primary standard and three other models (MR-Egger regression (19), weighted median (20) and maximum likelihood (21)) as supplements to evaluate the potential causal relationship between household income status and seven pulmonary diseases. A reverse MR study evaluated the potential causal relationship between seven pulmonary diseases and household income status using the same methods. In addition, a sensitivity analysis was performed to measure the reliability and stability of the conclusion. The sensitivity analysis comprised: (1) Cochran’s Q test (according to the IVW or MR-Egger regression model), (2) horizontal pleiotropy test using MR-Egger intercept (22) and MR-PRESSO test (23), and (3) “leave-one-out” test (in which each SNP was abandoned successively to repeat the IVW analysis to identify whether any specific SNP drives the causal relationship estimate). The results are reported as odds ratios (OR) with corresponding 95% confidence intervals (CI) and p-values and are illustrated as scatter plots. The evidential threshold in the MR analysis was defined as p-value <0.004 (0.05/14) according to the Bonferroni correction method. A p-value <0.05, but above the Bonferroni-corrected evidential threshold, was considered to indicate a potential association. For sensitivity analysis, statistical significance was set at p-value <0.05. The “TwoSampleMR” (24) and “MR-PRESSO” (23) packages of R 4.0.3 software were used to process and visualize the data.
3 Results
3.1 Results of the MR study
Forty-three SNPs were identified as IVs in each outcome dataset. The F-statistic score of all the selected SNPs was >10 (COPD: 57.64, asthma: 57.87, tuberculosis: 57.77, bronchiectasis: 57.77, COVID-19: 57.77, pneumonia: 57.49, and lung cancer: 57.76), indicating a low risk of weak instrumental bias.
According to the results of random-effects IVW model with Bonferroni correction as the primary standard, a higher household income tended to lower the risk of genetic susceptibility to COPD (OR: 0.497, 95% CI = 0.337–0.733, p < 0.001), asthma (OR: 0.687, 95% CI = 0.540–0.876, p = 0.002), and lung cancer (OR: 0.569, 95% CI = 0.433–0.748, p < 0.001), and indicated a potential causality with pneumonia (OR: 0.817, 95% CI = 0.686–0.972, p = 0.022). However, no evidence was obtained to suggest a potential causal relationship between household income status and COVID-19 (OR: 0.934, 95% CI = 0.764–1.142, p = 0.507), tuberculosis (OR: 0.597, 95% CI = 0.512–1.189, p = 0.120), or bronchiectasis (OR: 0.680, 95% CI = 0.311–1.489, p = 0.400). The results of the weighted median and maximum likelihood estimation models supported these conclusions. Unfortunately, the MR-Egger regression model results did not show any statistically significant differences.
In summary, the MR study revealed that the population with superior household income tended to have a lower genetic susceptibility risk to COPD, asthma, and lung cancer. Detailed information is displayed in the forest map in Figure 1, and as a scatter plot in Supplementary Figure S1.
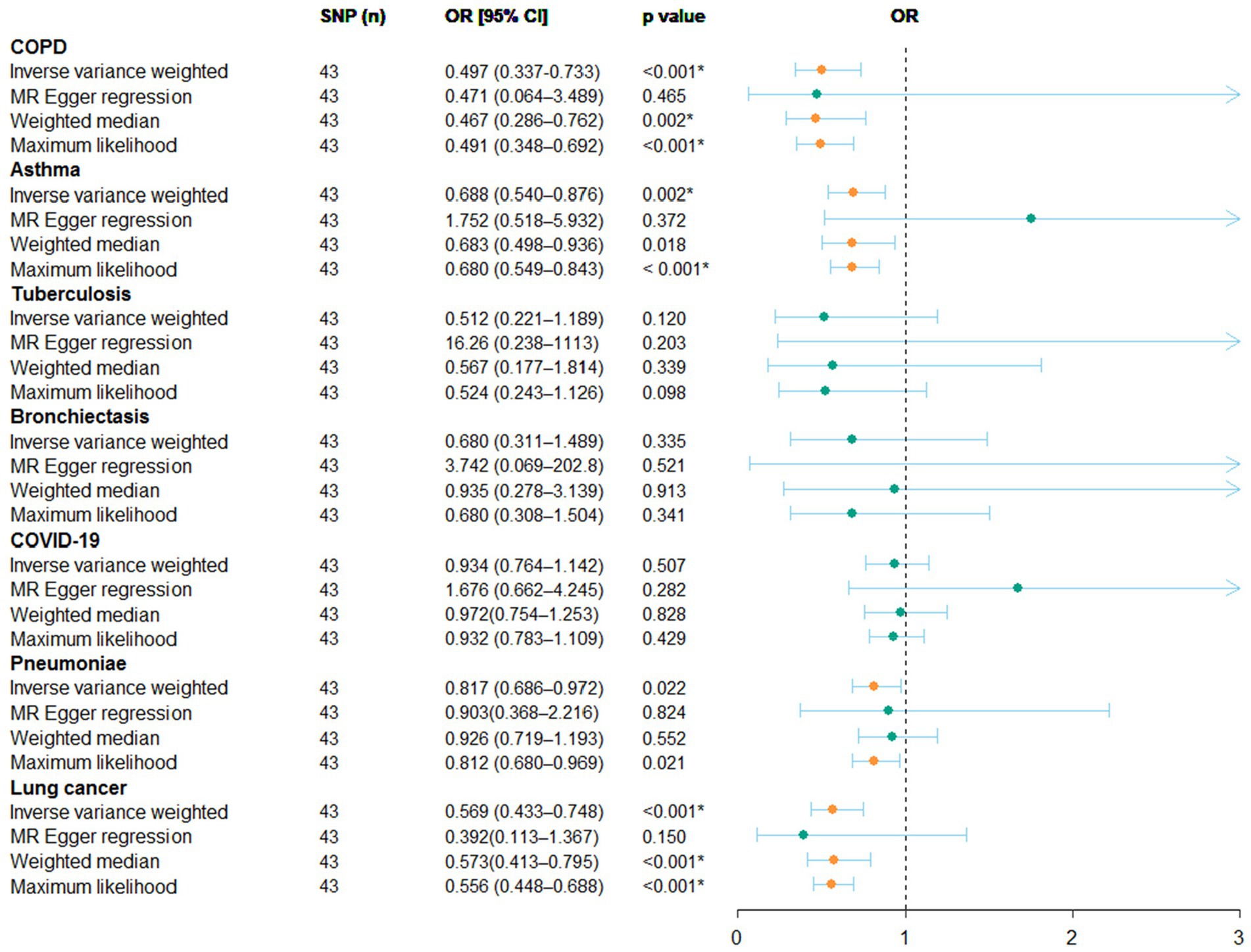
Figure 1. Result of the MR study illustrated as a forest plot. The causal relationship between household income status and pulmonary diseases by MR study is shown. OR, odds ratio; CI, confidence interval.
3.2 Results of sensitivity analyses in the MR study
The results of Cochran’s Q test indicated a certain degree of heterogeneity among the IVs in lung cancer (Table 2), but not in the other six types of pulmonary diseases. Thus, the random-effects IVW model was used to minimize the effect of heterogeneity in the MR study to the greatest possible extent. More importantly, no horizontal pleiotropy was detected using the MR-Egger intercept or MR-PRESSO tests (Table 2). In addition, the “leave-one-out” method indicated that no specific SNP among the IVs significantly affected the overall results (Supplementary Figure S2). In summary, the sensitivity analyses verified the robustness of the conclusions.
3.3 Results of reverse MR study and sensitivity analyses
The number of SNPs that were ultimately identified as IVs in different pulmonary diseases in the reverse MR study were 15 for asthma, 13 for lung cancer, 7 for COVID-19, 3 for COPD, 1 for bronchiectasis and pneumonia, and 0 for tuberculosis. Based on the results of random-effects IVW model with Bonferroni correction as the primary standard, the reverse MR study suggested no reverse causal relationship between pulmonary disease and household income. Detailed information is displayed in Table 3.
4 Discussion
Previous epidemiological studies have demonstrated that socioeconomic status has a significant impact on pulmonary diseases (25). Given its integral nature as an aspect of socioeconomic status, household income status has also consistently been linked to pulmonary diseases. Nevertheless, the underlying causal relationship remains unclear. To the best of our knowledge, this study is the first to investigate the causal relationship between household income status and pulmonary diseases using a bidirectional two-sample MR approach. Our findings suggest that individuals with a higher household income tend to have a lower risk of developing COPD, asthma, and lung cancer. Additionally, a reverse MR analysis did not indicate any reverse causal relationship between pulmonary disease and household income status.
Our findings are supported by accumulating evidence from both cohort studies and meta-analyses. Adeloye et al. reported that more than three-quarters of global COPD cases occur in low- and middle-income countries (26). Meanwhile, a multicenter study with 11,042 participants directed by Matthew Grigsby revealed that the prevalence of COPD was negatively associated with monthly household income status (OR = 0.96 per category, 95% CI = 0.93–0.99) (27). Moreover, Pakhale et al. reviewed the association between socioeconomic status and COPD morbidity in Ottawa. They showed that the population with a lower household income had a significantly higher COPD prevalence than the average citizen (28). Similarly, Yan Borné et al. suggested that, in Sweden, low annual income is a risk factor for COPD (hazard ratio [HR] = 2.23; 95% CI = 1.97–2.53, p < 0.001) (29). Asthma is another threatening respiratory disease affecting all age groups, particularly children (30). Multiple observational studies have concluded that populations with inferior family income status are at an increased risk of suffering from asthma (31–33). In addition, lung cancer is an increasing problem in developing countries because of increasing smoking trends, high incidence of air pollution, lack of awareness and screening, delayed presentation, and late diagnosis (34). In this study, we identified low-income status as a risk factor for lung cancer. This viewpoint concurs with the conclusions drawn by Orlewska et al. in Poland (35) and Fabian Tetzlaff et al. in Germany (36). Additionally, a prior study on the geographical distribution of mortality in urban areas in Spain revealed that areas with high mortality from COPD and lung cancer often coincided with low-income neighborhoods (37). Together, the results of these studies show that household income status and the poor living environment which occurs as a consequence are risk factors for respiratory diseases, such as COPD, asthma, and lung cancer.
Several pathological mechanisms may explain the association between household income and genetic susceptibility to pulmonary diseases. First, it is well known that air pollution and smoking are essential risk factors for pulmonary diseases. Populations with inferior household income status and living standards in low-income countries may not only be susceptible to poor living conditions, but may also be more likely engage in unhealthy lifestyle behaviors such as smoking. Gaffney et al. reported that, in the United States, current/former smoking rates in the highest income quintile decreased by ~50% from 1971 to 2018, while the rates in the lowest income quintile remained largely unchanged at an alarming level of ~60% (3). Secondly, populations with lower household incomes are more likely to face deficiencies in nutrients, such as high-quality protein, fresh fruits and vegetables, dietary fiber, vitamins, and micronutrients. These nutrients are closely linked to immune system function in vivo, particularly during pregnancy and childhood (38–41). Finally, low-income populations are less likely to have access to healthcare services and more likely to lack health consciousness, which may result in respiratory diseases that persist for a long time. Gaffney et al. concluded that, even though the uninsured rate among adults with asthma in the US decreased from 19.4 to 9.6% (adjusted 9.27 percentage points; 95% CI = 7.1–11.5%) from 1997 to 2018, the proportions delaying or foregoing medical care because of cost or going without medications did not improve. Meanwhile, in the same study, a similar trend was also reported in patients with COPD (42).
Although this study has provided valuable scientific insights, however, there are several limitations that need to be addressed. Firstly, the GWAS datasets utilized in the MR analysis primarily including individuals of European descent, suggesting that the findings may not be fully applicable to other ethnic groups. Secondly, the biological mechanisms of SNPs and their impact on human susceptibility to respiratory diseases require further exploration. Finally, the current MR study did not include a sub-group analysis for populations known to be at increased risk of COPD, asthma, and lung cancer. By incorporating such an analysis, we could enhance the practical utility of the study by obtaining a more comprehensive understanding of how household income affects diverse population subgroups. This, in turn, could facilitate the development of more targeted policies and support measures.
5 Conclusion
Overall, the results of this bidirectional MR study demonstrated that individuals with higher household income exhibit a reduced risk of genetic predisposition to COPD, asthma, and lung cancer. These findings highlight the need to include household income disparities in medical reimbursement policies, and to prioritize efforts to enhance equal access to and availability of medical services for individuals in low-income households.
Data availability statement
The original contributions presented in the study are included in the article/Supplementary material, further inquiries can be directed to the corresponding author.
Ethics statement
The requirement of ethical approval was waived for the studies involving humans because the variable genetic information involved in this study was extracted from the Integrative Epidemiology Unit (IEU) GWAS database (12) (https://gwas.mrcieu.ac.uk/), which is a publicly available GWAS summary database. The studies were conducted in accordance with the local legislation and institutional requirements. The participants provided their written informed consent to participate in these studies.
Author contributions
HX: Conceptualization, Writing – original draft. HD: Writing – original draft, Data curation. YW: Data curation, Writing – original draft. YY: Conceptualization, Supervision, Writing – review & editing. XZ: Methodology, Project administration, Writing – review & editing.
Funding
The author(s) declare financial support was received for the research, authorship, and/or publication of this article. The research was funded by the scientific research program of the Affiliated Hospital of Guangdong Medical University (No. 2021113808 and No. 2021093728).
Acknowledgments
We would like to acknowledge the participants and investigators of the IEU OpenGWAS project, the UK Biological Bank and the FinnGen studies for their contributions.
Conflict of interest
The authors declare that the research was conducted in the absence of any commercial or financial relationships that could be construed as a potential conflict of interest.
Publisher’s note
All claims expressed in this article are solely those of the authors and do not necessarily represent those of their affiliated organizations, or those of the publisher, the editors and the reviewers. Any product that may be evaluated in this article, or claim that may be made by its manufacturer, is not guaranteed or endorsed by the publisher.
Supplementary material
The Supplementary material for this article can be found online at: https://www.frontiersin.org/articles/10.3389/fmed.2024.1279697/full#supplementary-material
Abbreviations
MR, Mendelian randomization; SNP, single nucleotide polymorphism; GWAS, genome-wide association study; IV, instrumental variable; IVW, inverse variance weighting; OR, odds ratio; CI, confidence interval; COPD, chronic obstructive pulmonary disease
Footnotes
References
1. Collaborator GBDCRD . Prevalence and attributable health burden of chronic respiratory diseases, 1990-2017: a systematic analysis for the global burden of disease study 2017. Lancet Respir Med. (2020) 8:585–96. doi: 10.1016/S2213-2600(20)30105-3
2. Rocha, V, Soares, S, Stringhini, S, and Fraga, S. Socioeconomic circumstances and respiratory function from childhood to early adulthood: a systematic review and meta-analysis. BMJ Open. (2019) 9:e027528. doi: 10.1136/bmjopen-2018-027528
3. Gaffney, AW, Himmelstein, DU, Christiani, DC, and Woolhandler, S. Socioeconomic inequality in respiratory health in the US from 1959 to 2018. JAMA Intern Med. (2021) 181:968–76. doi: 10.1001/jamainternmed.2021.2441
4. Gaffney, AW, Hang, JQ, Lee, MS, Su, L, Zhang, FY, and Christiani, DC. Socioeconomic status is associated with reduced lung function in China: an analysis from a large cross-sectional study in Shanghai. BMC Public Health. (2016) 16:96. doi: 10.1186/s12889-016-2752-3
5. Rocha, V, Fraga, S, Moreira, C, Carmeli, C, Lenoir, A, Steptoe, A, et al. Life-course socioeconomic disadvantage and lung function: a multicohort study of 70496 individuals. Eur Respir J. (2021) 57:2001600. doi: 10.1183/13993003.01600-2020
6. Emdin, CA, Khera, AV, and Kathiresan, S. Mendelian randomization. JAMA. (2017) 318:1925–6. doi: 10.1001/jama.2017.17219
7. Davies, NM, Holmes, MV, and Davey, SG. Reading Mendelian randomisation studies: a guide, glossary, and checklist for clinicians. BMJ. (2018) 362:k601. doi: 10.1136/bmj.k601
8. Richmond, RC, and Davey Smith, G. Mendelian randomization: concepts and scope. Cold Spring Harb Perspect Med. (2022) 12:a040501. doi: 10.1101/cshperspect.a040501
9. Zheng, X, Yang, Y, Chen, J, and Lu, B. Dissecting the causal relationship between household income status and genetic susceptibility to cardiovascular-related diseases: insights from bidirectional mendelian randomization study. BMC Public Health. (2023) 23:749. doi: 10.1186/s12889-023-15561-7
10. Gao, Y, Mi, J, Liu, Z, and Song, Q. Leisure sedentary behavior and risk of lung Cancer: a two-sample Mendelian randomization study and mediation analysis. Front Genet. (2021) 12:763626. doi: 10.3389/fgene.2021.763626
11. Zhou, H, Zhang, Y, Liu, J, Yang, Y, Fang, W, Hong, S, et al. Education and lung cancer: a Mendelian randomization study. Int J Epidemiol. (2019) 48:743–50. doi: 10.1093/ije/dyz121
12. Elsworth, B, Lyon, M, Alexander, T, et al. The MRC IEU OpenGWAS data inrastructure. bioRxiv. (2020). doi: 10.1101/2020.08.10.244293
13. Bycroft, C, Freeman, C, Petkova, D, Band, G, Elliott, LT, Sharp, K, et al. The UK biobank resource with deep phenotyping and genomic data. Nature. (2018) 562:203–9. doi: 10.1038/s41586-018-0579-z
14. Kurki, MI, Karjalainen, J, Palta, P, et al. FinnGen: Unique genetic insights from combining isolated population and national health register data. medRxiv. (2022).
15. Initiative TC-HG . The COVID-19 host genetics initiative, a global initiative to elucidate the role of host genetic factors in susceptibility and severity of the SARS-CoV-2 virus pandemic. Eur J Human Genet. (2020) 28:715–8. doi: 10.1038/s41431-020-0636-6
16. Yuan, S, Carter, P, Bruzelius, M, Vithayathil, M, Kar, S, Mason, AM, et al. Effects of tumour necrosis factor on cardiovascular disease and cancer: a two-sample Mendelian randomization study. EBioMedicine. (2020) 59:102956. doi: 10.1016/j.ebiom.2020.102956
17. Wu, F, Huang, Y, Hu, J, and Shao, Z. Mendelian randomization study of inflammatory bowel disease and bone mineral density. BMC Med. (2020) 18:312. doi: 10.1186/s12916-020-01778-5
18. Burgess, S, Dudbridge, F, and Thompson, SG. Combining information on multiple instrumental variables in Mendelian randomization: comparison of allele score and summarized data methods. Stat Med. (2016) 35:1880–906. doi: 10.1002/sim.6835
19. Bowden, J, Davey Smith, G, and Burgess, S. Mendelian randomization with invalid instruments: effect estimation and bias detection through egger regression. Int J Epidemiol. (2015) 44:512–25. doi: 10.1093/ije/dyv080
20. Bowden, J, Davey Smith, G, Haycock, PC, and Burgess, S. Consistent estimation in Mendelian randomization with some invalid instruments using a weighted median estimator. Genet Epidemiol. (2016) 40:304–14. doi: 10.1002/gepi.21965
21. Nguyen, LT, Schmidt, HA, von Haeseler, A, and Minh, BQ. IQ-TREE: a fast and effective stochastic algorithm for estimating maximum-likelihood phylogenies. Mol Biol Evol. (2015) 32:268–74. doi: 10.1093/molbev/msu300
22. Burgess, S, and Thompson, SG. Interpreting findings from Mendelian randomization using the MR-egger method. Eur J Epidemiol. (2017) 32:377–89. doi: 10.1007/s10654-017-0255-x
23. Verbanck, M, Chen, CY, Neale, B, and Do, R. Detection of widespread horizontal pleiotropy in causal relationships inferred from Mendelian randomization between complex traits and diseases. Nat Genet. (2018) 50:693–8. doi: 10.1038/s41588-018-0099-7
24. Hemani, G, Zheng, J, Elsworth, B, Wade, KH, Haberland, V, Baird, D, et al. The MR-base platform supports systematic causal inference across the human phenome. eLife. (2018) 7:34408. doi: 10.7554/eLife.34408
25. Sahni, S, Talwar, A, Khanijo, S, and Talwar, A. Socioeconomic status and its relationship to chronic respiratory disease. Adv Respirat Med. (2017) 85:97–108. doi: 10.5603/ARM.2017.0016
26. Adeloye, D, Song, P, Zhu, Y, Campbell, H, Sheikh, A, and Rudan, I. Global, regional, and national prevalence of, and risk factors for, chronic obstructive pulmonary disease (COPD) in 2019: a systematic review and modelling analysis. Lancet Respir Med. (2022) 10:447–58. doi: 10.1016/S2213-2600(21)00511-7
27. Grigsby, M, Siddharthan, T, Chowdhury, MA, Siddiquee, A, Rubinstein, A, Sobrino, E, et al. Socioeconomic status and COPD among low- and middle-income countries. Int J Chron Obstruct Pulmon Dis. (2016) 11:2497–507. doi: 10.2147/COPD.S111145
28. Pakhale, S, Tariq, S, Huynh, N, Jama, S, Kaur, T, Charron, C, et al. Prevalence and burden of obstructive lung disease in the urban poor population of Ottawa, Canada: a community-based mixed-method, observational study. BMC Public Health. (2021) 21:183. doi: 10.1186/s12889-021-10209-w
29. Borné, Y, Ashraf, W, Zaigham, S, and Frantz, S. Socioeconomic circumstances and incidence of chronic obstructive pulmonary disease (COPD) in an urban population in Sweden. COPD. (2019) 16:51–7. doi: 10.1080/15412555.2019.1582618
30. Reddel, HK, Bacharier, LB, Bateman, ED, Brightling, CE, Brusselle, GG, Buhl, R, et al. Global initiative for asthma strategy 2021: executive summary and rationale for key changes. Am J Respir Crit Care Med. (2022) 205:17–35. doi: 10.1164/rccm.202109-2205PP
31. Cardet, JC, Louisias, M, King, TS, Castro, M, Codispoti, CD, Dunn, R, et al. Income is an independent risk factor for worse asthma outcomes. J Allergy Clin Immunol. (2018) 141:754–60.e3. doi: 10.1016/j.jaci.2017.04.036
32. Simoneau, T, and Gaffin, JM. Socioeconomic determinants of asthma health. Curr Opin Pediatr. (2023) 35:337–43. doi: 10.1097/MOP.0000000000001235
33. Loftus, PA, and Wise, SK. Epidemiology and economic burden of asthma. Int Forum Allergy Rhinol. (2015) 5:S7–S10. doi: 10.1002/alr.21547
34. Jiwnani, S, Penumadu, P, Ashok, A, and Pramesh, CS. Lung Cancer Management in low and Middle-Income Countries. Thorac Surg Clin. (2022) 32:383–95. doi: 10.1016/j.thorsurg.2022.04.005
35. Orlewska, K, Sliwczynski, A, and Orlewska, E. An ecological study of the link between the risk of most frequent types of cancer in Poland and socioeconomic variables. Int J Public Health. (2018) 63:777–86. doi: 10.1007/s00038-018-1082-x
36. Tetzlaff, F, Hoebel, J, Epping, J, Geyer, S, Golpon, H, and Tetzlaff, J. Time trends and income inequalities in Cancer incidence and Cancer-free life expectancy—a Cancer site-specific analysis of German health insurance data. Front Oncol. (2022) 12:827028. doi: 10.3389/fonc.2022.827028
37. Martinez-Beneito, MA, Vergara-Hernández, C, Botella-Rocamora, P, et al. Geographical variability in mortality in urban areas: a joint analysis of 16 causes of death. Int J Environ Res Public Health. (2021) 18:5664. doi: 10.3390/ijerph18115664
38. Kaluza, J, Harris, HR, Linden, A, and Wolk, A. Long-term consumption of fruits and vegetables and risk of chronic obstructive pulmonary disease: a prospective cohort study of women. Int J Epidemiol. (2018) 47:1897–909. doi: 10.1093/ije/dyy178
39. Kaluza, J, Larsson, SC, Orsini, N, Linden, A, and Wolk, A. Fruit and vegetable consumption and risk of COPD: a prospective cohort study of men. Thorax. (2017) 72:500–9. doi: 10.1136/thoraxjnl-2015-207851
40. Kaluza, J, Harris, H, Wallin, A, Linden, A, and Wolk, A. Dietary Fiber intake and risk of chronic obstructive pulmonary disease: a prospective cohort study of men. Epidemiology. (2018) 29:254–60. doi: 10.1097/EDE.0000000000000750
41. Seyedrezazadeh, E, Moghaddam, MP, Ansarin, K, Asghari Jafarabadi, M, Sharifi, A, Sharma, S, et al. Dietary factors and risk of chronic obstructive pulmonary disease: a systemic review and Meta-analysis. Tanaffos. (2019) 18:294–309.
Keywords: household income status, pulmonary diseases, causal relationship, instrumental variable, Mendelian randomization
Citation: Xu H, Deng H, Wu Y, Yang Y and Zheng X (2024) Household income unequally affects genetic susceptibility to pulmonary diseases: evidence from bidirectional Mendelian randomization study. Front. Med. 11:1279697. doi: 10.3389/fmed.2024.1279697
Edited by:
María Del Carmen Valls Martínez, University of Almeria, SpainReviewed by:
Basim Dubaybo, Wayne State University, United StatesXinpei Wang, Peking University, China
Copyright © 2024 Xu, Deng, Wu, Yang and Zheng. This is an open-access article distributed under the terms of the Creative Commons Attribution License (CC BY). The use, distribution or reproduction in other forums is permitted, provided the original author(s) and the copyright owner(s) are credited and that the original publication in this journal is cited, in accordance with accepted academic practice. No use, distribution or reproduction is permitted which does not comply with these terms.
*Correspondence: Xifeng Zheng, zhengxf@gdmu.edu.cn
†These authors share first authorship