- 1Department of Clinical Chemistry and Informatics, Graduate School of Pharmaceutical Sciences, Kumamoto University, Kumamoto, Japan
- 2Diabetes Care Center, Jinnouchi Hospital, Kumamoto, Japan
- 3Department of Pharmacy, Kumamoto University Hospital, Kumamoto, Japan
- 4Department of Clinical Pharmaceutical Sciences, Graduate School of Pharmaceutical Sciences, Kumamoto University, Kumamoto, Japan
- 5Department of Pharmaceutical Packaging Technology, Faculty of Life Sciences, Kumamoto University, Kumamoto, Japan
- 6Department of Academic Education, I & H Co., Ltd., Ashiya, Japan
Background: The estimation of creatinine clearance (CCr) in older adult patients with diabetes is subject to deviations from the results of actual measurements because of changes in body composition. In the present study, we aimed to create a correction for the equation used for the estimation of CCr in older adult Asian patients with diabetes using body composition parameters.
Methods: We enrolled 50 older Japanese patients with diabetes in whom the measured values of CCr were compared with values estimated using the Cockcroft-Gault equation. The relationships between the error in the estimated CCr and body composition parameters were investigated, and the Cockcroft-Gault equation was corrected using the appropriate parameters. To evaluate the generalizability of the corrected equation, the utility of the Cockcroft-Gault equation, which was corrected on the basis of body composition measured using a household body composition meter, was also investigated.
Results: Body fat mass (BFM) was closely correlated with the error in the estimated CCr. The BFM-corrected Cockcroft-Gault equation was more accurate than the original equation. Similarly, the error became smaller using BFM measured with a household body composition meter.
Conclusion: The BFM-corrected Cockcroft-Gault equation may provide an accurate method of estimating CCr that can be used in general practice.
1 Introduction
Chronic kidney disease is a global public health problem, the global prevalence of which was estimated to be 9.1% in 2017 (1). Aging and diabetes are associated with a deterioration in renal function (2). In developed countries, including Japan, diabetic nephropathy is considered to frequently lead to end-stage renal disease, necessitating dialysis (3). Therefore, accurately estimating renal function is necessary to facilitate the early detection of renal dysfunction and the design of therapeutic approaches that consider the mode of excretion of drugs for patients with diabetes.
In the majority of clinical settings, the Cockcroft-Gault (CG) equation (4), which is designed to estimate creatinine clearance (CCr) using the serum creatinine (SCr) concentration, is often used to evaluate renal function. However, values generated using the CG equation can be affected by patient-specific factors, such as age, body mass, muscle mass, disease, and certain medications (5, 6). Aging and the accompanying changes in body composition affect the SCr concentration because this depends on muscle mass and reduces the accuracy of this method (7, 8). This is a major problem, especially for Asian individuals, who have a lower muscle mass than Westerners (9, 10). In addition, several previous studies have shown that diabetes affects SCr and the accuracy of estimations made using SCr-based equations for Asian people (11, 12). Furthermore, a recent study showed that the relationships between diabetes, muscle loss, and subsequent changes in SCr were affected by aging (13). Therefore, a more accurate method of estimating renal function in older Asian patients with diabetes (e.g., as a simple method of correcting the existing equation) that could be used in clinical practice is required.
In the present study, we compared the accuracies of the original CG equation and a simple corrected version of this equation, based on body composition parameters, in older Japanese adult patients with diabetes. In addition, we investigated the usefulness of the CG equation, which was corrected for body composition parameters measured using a household body composition meter, rather than a precise body composition meter.
2 Materials and methods
2.1 Study design and participants
We conducted a single-center observational study at Jinnouchi Hospital. The participants were older patients with type 2 diabetes who were admitted to Jinnouchi Hospital between January 2019 and April 2020. Using the definition of the Japanese guidelines for medical treatment and its safety in the elderly (14), older patients in this study were defined as those aged ≥ 65 years. All 50 of the participants provided their written informed consent. The exclusion criteria were as follows: ineligibility for bioelectrical impedance analysis [i.e., patients with a pacemaker, defibrillator, or an artificial joint; acute kidney injury; progressive or terminal cancer; myopathy (e.g., muscular dystrophy); or marked dysuria]; and patients who were undergoing dialysis or taking drugs known to inhibit creatinine secretion in the proximal tubule [e.g., cimetidine (15) and trimethoprim (16)]. The characteristics of the participants are shown in Table 1.
2.2 Measurement of actual CCr
The actual creatinine clearance (aCCr) was calculated using the equation shown below.
After emptying the bladder, 24-h urine collection commenced. To measure the duration of urine collection accurately, the times of the commencement and termination of urine collection were recorded for each participant, and the duration of urine collection was calculated in minutes. Therefore, in some of the participants, urine was collected for a slightly longer or shorter time than 1,440 min (24 h), but the aCCr calculated in this way was considered to be more accurate. Consequently, the mean ± SD (minimum-maximum) urine collection time (min) and urine volume (mL) for participants were 1442.8 ± 74.2 (1260–1645) and 1826 ± 707.1 (800–5200), respectively. Furthermore, urinary creatinine excretion (mg/BW/day) was 14.8 ± 4.9 (5.8–34.9). These results indicate that urine collection was nearly complete.
SCr concentrations were measured at the beginning or after the end of urine collection. SCr and urine creatinine concentrations were measured by an enzymatic method using the UniCel DxC600 (Beckman Coulter, Tokyo, Japan).
2.3 Estimated CCr
Estimated CCr (eCCr) was calculated using the Cockcroft-Gault equation shown below.
2.4 Measurement of body composition parameters
Body composition parameters were measured using the InBody® 770 (Inbody Japan Corporation, Tokyo, Japan), which is a high-performance medical body composition meter. We also used the eight-electrode Direct Segmental Multi-frequency bioelectrical impedance analysis method and the RD906 (Tanita Corporation, Tokyo, Japan), which is a household body composition meter. The measurements were made between 16:00 and 17:00 h to avoid the confounding effects of eating and bathing (17). The InBody 770 was used to measure body fat mass (BFM), fat-free mass (FFM), skeletal muscle mass (SM), and appendicular skeletal muscle mass (ASM). FM and SM were also measured using the Tanita RD906.
2.5 Statistical analysis
Data are shown as the mean ± standard deviation (SD). To compare the sex differences among the participants, Welch’s t-test was used for continuous variables and Fisher’s exact test was used for categorical variables. To evaluate the performance of each equation, Pearson correlation coefficients were calculated. Biases, fixed errors, and proportional errors were calculated using Brand–Altman analysis (18). Applying regression analysis to the Bland-Altman plot, it was determined that proportional bias was present when a significant correlation was observed. The mean absolute error and the percentage of participants with an error within 30% of the aCCr (p30) were used. To compare the equations, paired t-tests were performed. The level of significance was set at p < 0.05. Statistical analyses were performed using JMP® Pro 16.2 (SAS Institute Inc., Cary, NC, USA).
3 Results
3.1 Participants’ characteristics
Fifty participants were included in the study, the characteristics of whom are shown in Table 1. Twenty-nine (58%) participants were men. Significant sex differences were identified in height, body mass, body composition (percentage BFM, FFM, SM, ASM, and skeletal muscle index), and SCr concentrations of the participants.
3.2 Correlation between aCCr and eCCr
The relationships between aCCr and eCCr are shown in Figure 1. The eCCr was significantly correlated with aCCr (R2 = 0.3537, p < 0.001, Figure 1A). However, a significant fixed error [bias: mean (95% confidence interval [CI]): –8.82 (–14.6 to –2.99), p < 0.004] and a significant proportional error (slope: –0.45, p < 0.002) were observed (Figure 1B and Table 2).
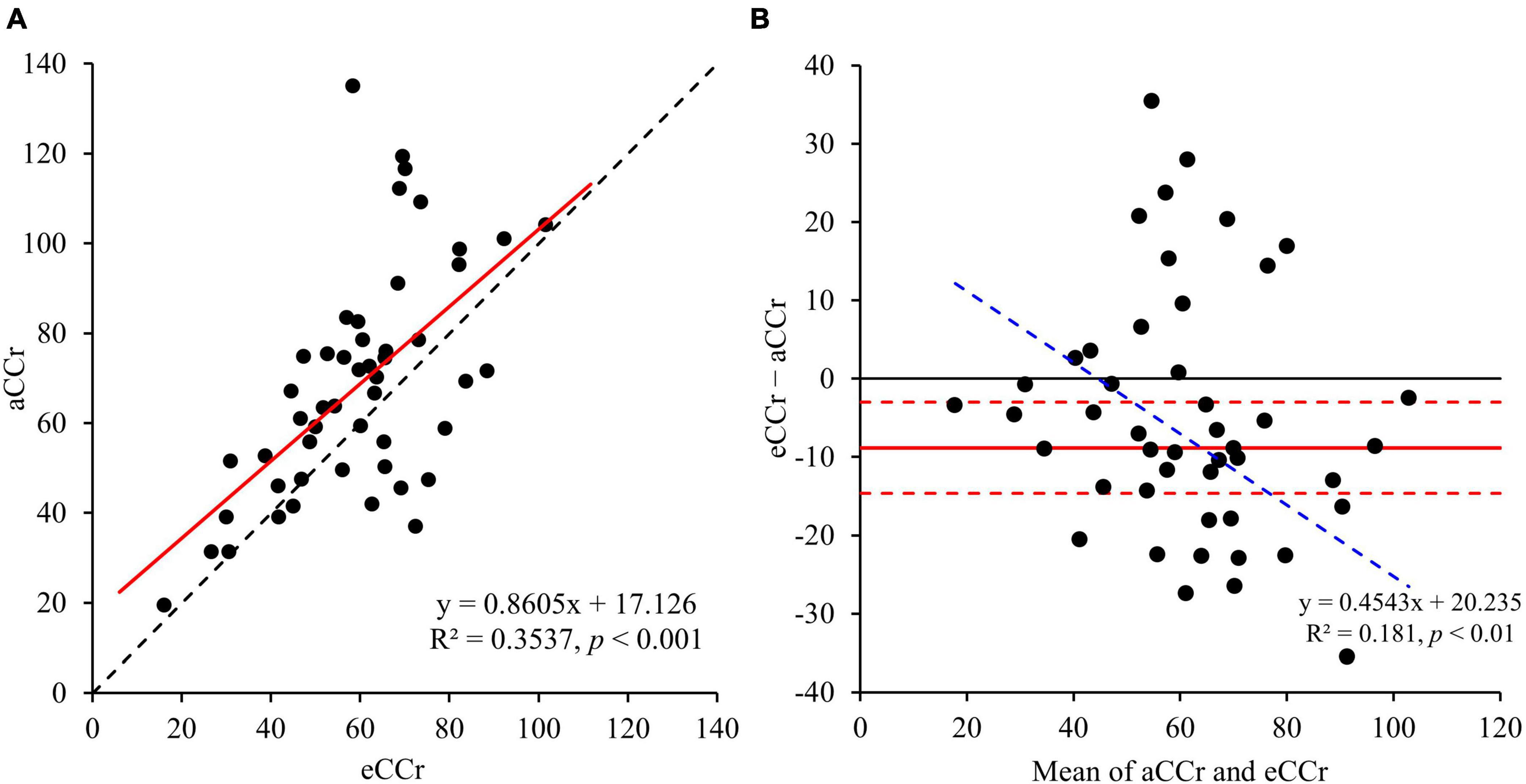
Figure 1. (A) Linear regression. y = x is shown by the dotted line and the regression line is shown as the solid red line. (B) Bland–Altman plots. The solid red line indicates the mean difference, the red dotted lines represent the upper and lower 95% limits of agreement and the blue dotted line is shown as regression line to indicate proportional error. aCCr, actual creatinine clearance; eCCr, estimated creatinine clearance.
3.3 Relationships of the difference between eCCr and aCCr with body composition parameters
The relationships of eCCr/aCCr with the body composition parameters measured using the InBody are shown in Figure 2. BFMmedical was significantly correlated with the difference between the measured and estimated values (R2 = 0.3302, p < 0.001), and the equation generated is shown below. None of the other body composition parameters were correlated with this difference.
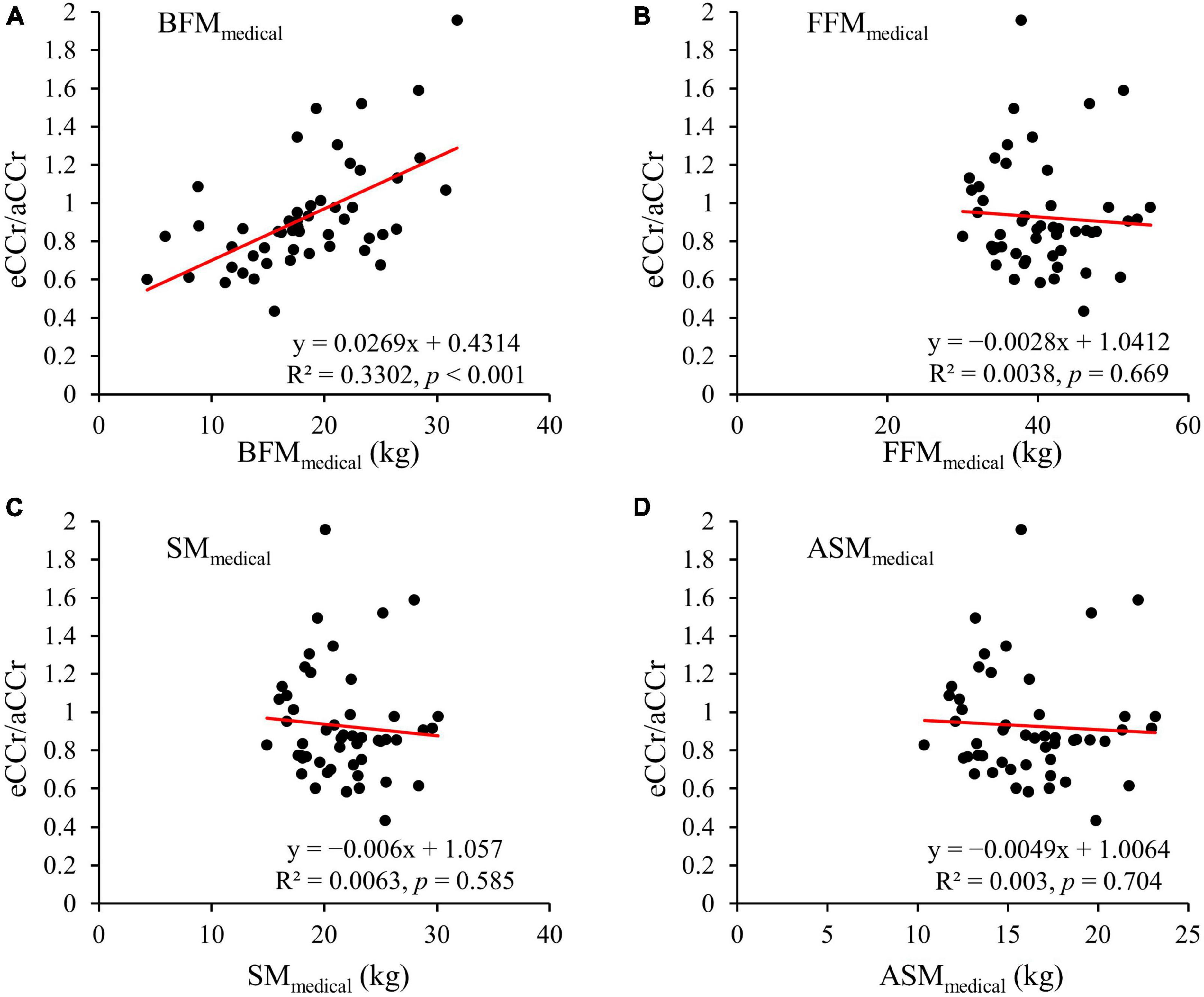
Figure 2. (A) BFMMedical. (B) FFMedical. (C) SMMedical. (D) ASMMedical. y = x = is shown as the dotted line and the regression line is shown as the solid red line. aCCr, actual creatinine clearance; eCCr, estimated creatinine clearance; BFMMedical, fat mass measured using InBody; FFMedical, fat-free mass measured using InBody; SMMedical, skeletal mass measured using InBody; ASMMedical, appendicular skeletal mass measured using InBody.
3.4 Relationships between aCCr and eCCr modified using BFMmedical
To obtain a more accurate eCCr equation, Equation 1 was transformed to obtain the following equation.
The correlation between aCCr and eCCr (modified BFMmedical) was significant (R2 = 0.5271, p < 0.001, Figure 3). In addition, significant fixed errors that were present in the original equation were not present with eCCr (modified BFMmedical) (bias: mean [95% CI]: –3.64 [–8.59 to 1.31], p < 0.004; Figure 3B and Table 2). Furthermore, the mean absolute error of eCCr (modified BFMmedical) was significantly lower than that of eCCr (Table 2). There was also a tendency toward greater accuracy as determined using the values obtained within 30% of the aCCR for eCCr (modified BFMmedical) and eCCr (p30: 78.0%, p = 0.132; Table 2).
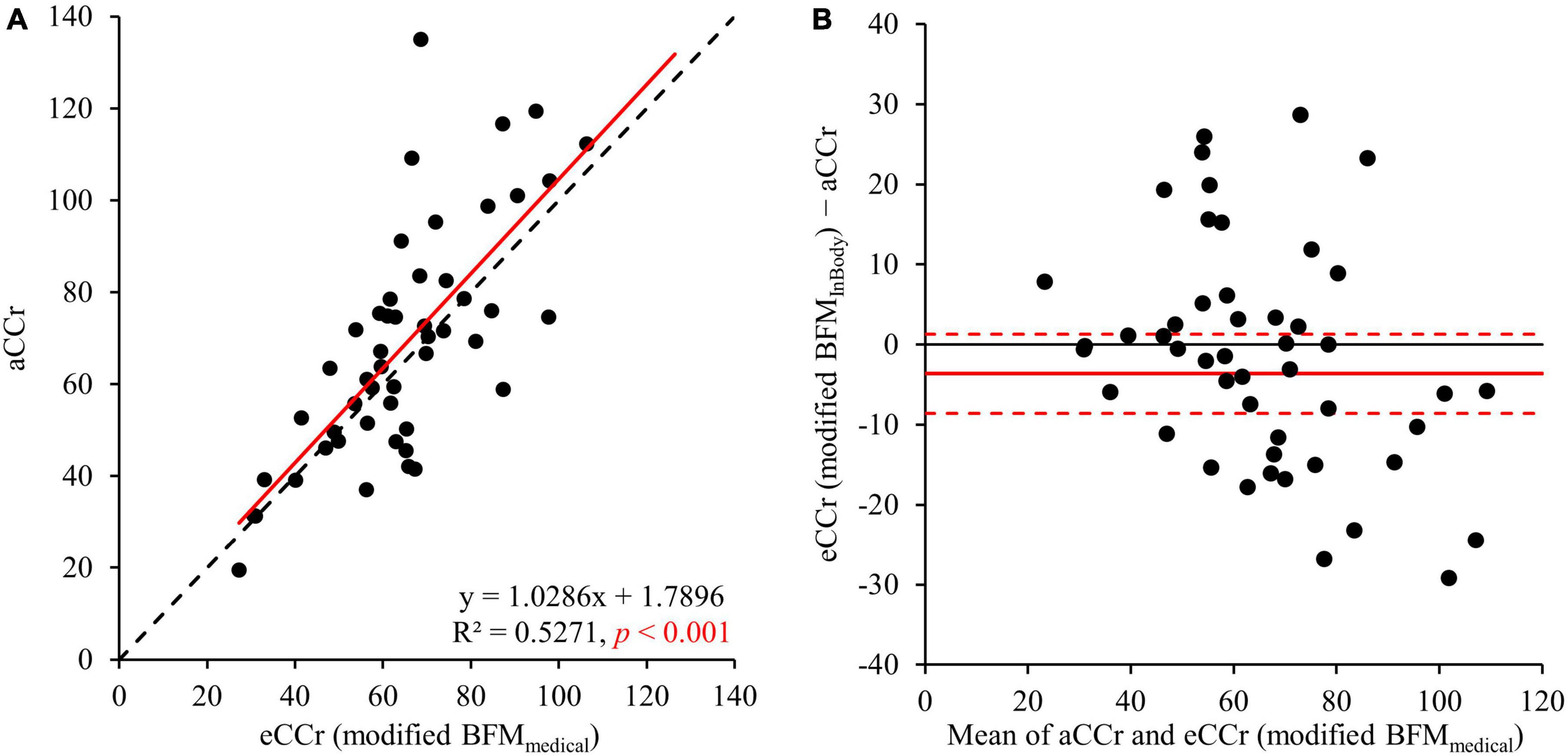
Figure 3. Relationships between aCCr and eCCr modified using BFMMedical. (A) Linear regression. y = x is shown as the dotted line and the regression line is shown as the solid red line. (B) Bland–Altman plots. The solid red line indicates the mean difference and the dotted lines represent the upper and lower 95% limits of agreement. aCCr, actual creatinine clearance; eCCr, estimated creatinine clearance; BFMMedical, fat mass measured using InBody.
3.5 Comparison of eCCr (modified BFMmedical) and eCCr (modified BFMhousehold)
The results of the comparison of eCCr (modified BFMmedical) and eCCr (modified BFMhousehold) are shown in Figure 4. BFMhousehold was significantly correlated with BFMmedical (R = 0.8385, p < 0.001) (Figure 4A). The eCCr (modified BFMhousehold) was calculated by substituting BFMhousehold into Equation 2. Therefore, eCCr (modified BFMhousehold) was also significantly correlated with aCCr (R2 = 0.5327, p < 0.001) (Figure 4B). In addition, eCCr (modified BFMhousehold) performed similarly to eCCr (modified BFMmedical) (Figure 4C and Table 2).
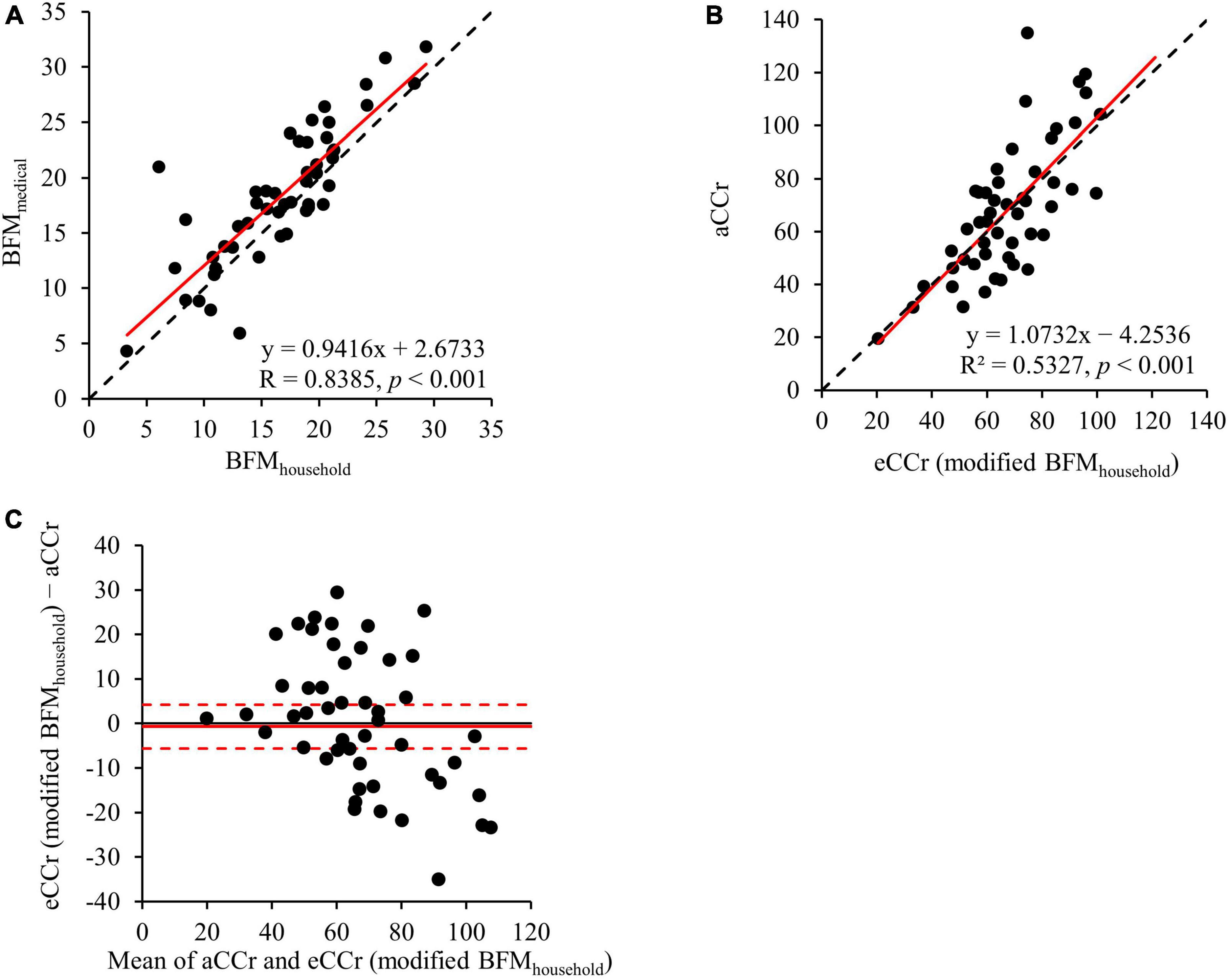
Figure 4. Comparison of eCCr (modified BFMMedical) and eCCr (modified BFMhousehold). (A) Linear regression of the relationship between BFMMedical and BFMhousehold. y = x is shown as the dotted line and the regression line is shown as the solid red line. (B) Linear regression of the relationship between aCCr and eCCr (modified BFMhousehold). y = x is shown as the dotted line and the regression line is shown as the solid red line. (C) Bland–Altman plots for aCCr and eCCr (modified BFMhousehold). The solid red line indicates the mean difference and the dotted lines represent the upper and lower 95% limits of agreement. aCCr, actual creatinine clearance; eCCr, estimated creatinine clearance; BFMMedical, fat mass measured using InBody; BFMhousehold, fat mass measured using a household body composition analyzer.
4 Discussion
This study showed that the original Cockcroft-Gault equation underestimated CCr in older Japanese adult patients with diabetes and that BFM was closely correlated with the difference between aCCr and eCCr. In addition, the use of modified equations using BFM measured using medical devices reduced this error. Finally, we showed that this error was also reduced by the use of a formula adjusted using BFM measured with a household body composition meter.
Obesity is one of the causes of the underestimation of renal dysfunction when using the CG equation (19), and the prevalence of obesity in patients with diabetes is high in Japan (20). Similarly, the prevalence of obesity (44%) in the participants in the present study was high. Nevertheless, the eCCr for older adult patients with diabetes was underestimated using the CG equation (Figure 1 and Table 2). Several previous studies (21–23) have shown that SCr-based formulae underestimate the renal function of Asian people, including Japanese people. In particular, the CG equation has been shown to underestimate CCr in older Japanese adult patients (24). Therefore, eCCr is not always overestimated in older Asian patients with diabetes.
To improve the accuracy of the estimation of CCr using the CG equation, we investigated the relationships between body composition parameters measured using a medical device and the error in the eCCr (eCCr/aCCr). We found that BFM was closely correlated with the error in eCCr and that eCCr was underestimated in participants with a low BFM and overestimated in those with a high BFM (Figure 2A). Interestingly, the indices of muscle mass (FFM, SM, and ASM), which should closely correlate with creatinine production, did not correlate with the error in eCCr (Figure 2D). Otani et al. (8) previously reported the usefulness of correcting eCCr using BFM for bedridden older Japanese people, and suggested that the high relative adiposity of bedridden patients may explain the error in eCCr. In general, Japanese patients with diabetes have a high percentage of body fat (25) and a low percentage of muscle mass (26). Furthermore, aging is associated with an increase in the percentage of body fat (27). This result has also been demonstrated in studies of Japanese (28) and Singaporean (29) adult cohorts. This evidence suggests that older Asians have a high body fat percentage (i.e., a relatively high amount of body fat per unit body weight). Consistent with this evidence, the participants in the present study had a high percentage of body fat (Table 1). These findings may explain why BFM correlates with the error in eCCr in older patients with diabetes. Therefore, we modified the CG equation using BFM, which yielded a superior predictive performance to the original CG equation (Figure 2 and Table 2). In addition, BFM contributed more strongly to the errors of the estimation equation than any other body composition parameter (Supplementary Table 1), and no significant partial correlations were observed with the other parameters (Supplementary Tables 3, 4). These results suggest that the correction of the CG equation with BFM improves the assessment of renal function in older adult Japanese patients with diabetes.
Medical body composition meters, such as the InBody770, can be used to accurately measure body composition parameters with the eight-electrode Direct Segmental Multi-frequency bioelectrical impedance analysis method (30) and dual-energy X-ray absorptiometry (19). Therefore, these meters have been used in many studies, including in the field of nephrology (7, 31–33). However, such meters are expensive and are thus only available in some medical institutions. In contrast, household body composition meters, such as the Tanita RD906, are cheaper than medical body composition analyzers and are easy to use, such that they can be used in a wider range of facilities. Therefore, to render the BFM-corrected CG equation more widely applicable, we next investigated whether BFM measured using a household body composition meter provides a useable alternative to BFM measured using a medical device for the modification of the CG equation. We found that the BFMhousehold values were similar to the BFMmedical values (Figure 4A). In addition, the CG equation corrected using BFMhousehold was similarly accurate to the equation in which BFMmedical was used (Figures 4B, C, and Table 2). Moreover, the improvement in accuracy of the BFM-corrected CG equation was consistent, regardless of SCr concentrations and gender and this trend was more pronounced in men and patients with chronic kidney disease (Supplementary Table 2). This gender difference may be explained by the gender disparity in the prevalence of sarcopenia among older adults. A previous report (34) indicated a higher prevalence of sarcopenia in older Japanese men than in women. Therefore, the larger difference between eCCr and aCCr in men might have led to a more pronounced correction in the estimation equations. These results suggest that the use of the CG equation corrected for BFM is suitable for use not only in specialist medical institutions, but also in wider clinical settings, such as in community pharmacies, for patients with chronic kidney disease.
The present study has some limitations. First, the participants were exclusively Japanese. Therefore, whether the present findings can be generalized to individuals of other ethnicities, including members of other Asian populations, is unclear. Second, the effects of circadian variation and the season on BFM were not investigated. Measurements of body composition were performed between 16:00 and 17:00 h to minimize the effects of bathing and eating, but whether our findings are applicable in other situations is unclear. Third, we aimed to correct estimations of CCr; therefore, this method is unlikely to be applied to the estimation of the glomerular filtration rate. The expression of organic cation transporters, which are one type of creatinine transporter (35), has been reported to be high in the presence of oxidative stress and high concentrations of advanced glycation end-products in rats (36). On the basis of these findings, Tsuda et al. suggested that patients with diabetes have greater tubular secretion of creatinine (11). Therefore, the equation corrected for BFM may only be applicable to eCCr. Fourth, there was a proportional error associated with the corrected equation. The correction for BFM improved the fixed error associated with the CG equation, but did not affect the proportional error (Table 2). Fifth, the presence of albuminuria reduced the improvement of accuracy in the BFMhousehold-corrected CG equation (Supplementary Table 2). The definitive mechanism is unknown, but using the correction equation is an important limitation. Sixth, although we examined confounders, we cannot rule out the existence of unknown confounders owing to the study design in this study. Indeed, the potential association between nutritional parameters and errors in renal function estimation equations cannot be denied. The nutritional parameters measured in this study, such as total cholesterol, serum albumin, serum iron, and various electrolyte concentrations, did not show a significant association with equation errors (Supplementary Tables 3, 4). However, other nutritional parameters, including transthyretin, total lymphocyte count, and dietary intake, have not been investigated. Seventh, although BFMhousehold and BFMmedical were closely correlated, the differences in the values obtained were large in some of the participants. We attempted to determine the causes of these differences, but could not identify a clear explanation. Eighth, the investigation of factors that could influence body composition is insufficient. In this study, there were few participants with a history of heart failure or the use of diuretics, factors that could potentially affect body composition. Therefore, it is unclear whether the results of this study are consistent in patients with these conditions. Finally, this study is a single-center study. The results of this study need to be validated in future additional studies, involving multiple facilities, to avoid excessive generalization of the current findings. To resolve these limitations, further studies are required.
5 Conclusion
This study shows that fat mass can be used to improve the accuracy with which CCr is estimated in older Japanese adult patients with diabetes. This result helps improve the underestimated renal function in individuals with diabetes, which is a prevailing foundational condition of renal impairment. Therefore, the improvement has the potential to enhance the refinement of drug dosages contingent upon CCr. In addition, the accuracy can be improved even when fat mass is assessed using a low-cost household body composition analyzer. The simplification of body composition assessment in this study is promising for clinical implementation and research of estimating body composition-based renal function in the field of nephrology. Although further studies to validate the results of this study are warranted, we consider that this method of modifying the CG equation based on BFM should be clinically useful.
Data availability statement
The raw data supporting the conclusions of this article will be made available by the authors, without undue reservation.
Ethics statement
The studies involving humans were approved by the Independent Ethics Committee of Kumamoto University Faculty of Life Sciences and Jinnouchi Hospital Institutional Review Board. The studies were conducted in accordance with the local legislation and institutional requirements. The participants provided their written informed consent to participate in this study.
Author contributions
SH conceived and designed the study. YK, TI, YI, and SH were responsible for project administration, responsible for supervision, and reviewed and edited the manuscript. SU, YH, AY, HN, and HJ were responsible for collecting data. SU, YH, and YK were responsible for the formal analysis. SU and YK were responsible for writing the original draft of the manuscript and the data curation. All authors were responsible for the conceptualization of the study and the methodology and approved the submitted version
Acknowledgments
We thank Mark Cleasby and Ellen Knapp, from Edanz (https://jp.edanz.com/ac) for editing a draft of this manuscript.
Conflict of interest
SH is employed by I & H Co., Ltd.
The remaining authors declare that the research was conducted in the absence of any commercial or financial relationships that could be construed as a potential conflict of interest.
Publisher’s note
All claims expressed in this article are solely those of the authors and do not necessarily represent those of their affiliated organizations, or those of the publisher, the editors and the reviewers. Any product that may be evaluated in this article, or claim that may be made by its manufacturer, is not guaranteed or endorsed by the publisher.
Supplementary materials
The Supplementary Material for this article can be found online at: https://www.frontiersin.org/articles/10.3389/fmed.2024.1228383/full#supplementary-material
References
1. Bikbov B, Purcell C, Levey A, Smith M, Abdoli A, Abebe M, et al. Global, regional, and national burden of chronic kidney disease, 1990–2017: a systematic analysis for the Global Burden of Disease Study 2017. Lancet. (2020) 395:709–33. doi: 10.1016/S0140-6736(20)30045-3
2. Mallappallil M, Friedman E, Delano B, McFarlane S, Salifu M. Chronic kidney disease in the elderly: evaluation and management. Clin Pract Lond Engl. (2014) 11:525–35. doi: 10.2217/cpr.14.46
3. Narres M, Claessen H, Droste S, Kvitkina T, Koch M, Kuss O, et al. The Incidence of End-Stage Renal Disease in the Diabetic (Compared to the Non-Diabetic) Population: A Systematic Review. PLoS One. (2016) 11:e0147329. doi: 10.1371/journal.pone.0147329
4. Cockcroft D, Gault M. Prediction of creatinine clearance from serum creatinine. Nephron. (1976) 16:31–41. doi: 10.1159/000180580
5. Winter M, Guhr K, Berg G. Impact of various body weights and serum creatinine concentrations on the bias and accuracy of the Cockcroft-Gault equation. Pharmacotherapy. (2012) 32:604–12. doi: 10.1002/j.1875-9114.2012.01098.x
6. Scappaticci G, Regal R. Cockcroft-Gault revisited: New de-liver-ance on recommendations for use in cirrhosis. World J Hepatol. (2017) 9:131–8. doi: 10.4254/wjh.v9.i3.131
7. Nakatani S, Maeda K, Akagi J, Ichigi M, Murakami M, Harada Y, et al. Coefficient of Determination between Estimated and Measured Renal Function in Japanese Patients with Sarcopenia May Be Improved by Adjusting for Muscle Mass and Sex: A Prospective Study. Biol Pharm Bull. (2019) 42:1350–7. doi: 10.1248/bpb.b19-00154
8. Otani T, Kase Y, Kunitomo K, Shimooka K, Naoe M, Yamamoto H, et al. Novel formula using triceps skinfold thickness to revise the Cockcroft-Gault equation for estimating renal function in Japanese bedridden elderly patients. J Med Invest. (2018) 65:195–202. doi: 10.2152/jmi.65.195
9. Lee R, Wang Z, Heo M, Ross R, Janssen I, Heymsfield S. Total-body skeletal muscle mass: development and cross-validation of anthropometric prediction models. Am J Clin Nutr. (2000) 72:796–803. doi: 10.1093/ajcn/72.3.796
10. Choo V. WHO reassesses appropriate body-mass index for Asian populations. Lancet Lond Engl. (2002) 360:235. doi: 10.1016/S0140-6736(02)09512-0
11. Tsuda A, Ishimura E, Ohno Y, Ichii M, Nakatani S, Machida Y, et al. Poor Glycemic Control Is a Major Factor in the Overestimation of Glomerular Filtration Rate in Diabetic Patients. Diabetes Care. (2014) 37:596–603. doi: 10.2337/dc13-1899
12. Lee E, Cho Y, Kim J, Koo B, Cho H, Ku Y, et al. Derivation of a new equation for estimating creatinine clearance by using fat-free mass and serum creatinine concentration in Korean patients with type 2 diabetes mellitus. Diabetes Res Clin Pract. (2009) 83:44–9. doi: 10.1016/j.diabres.2008.09.047
13. Kashima S, Inoue K, Matsumoto M. Low creatinine levels in diabetes mellitus among older individuals: the Yuport Medical Checkup Center Study. Sci Rep. (2021) 11:15167. doi: 10.1038/s41598-021-94441-9
14. Akishita M. Guidelines for medical treatment and its safety in the elderly. Nippon Ronen Igakkai Zasshi. (2007) 44:31–4. doi: 10.3143/geriatrics.44.31
15. Roubenoff R, Drew H, Moyer M, Petri M, Whiting-O’Keefe Q, Hellmann D. Oral Cimetidine Improves the Accuracy and Precision of Creatinine Clearance in Lupus Nephritis. Ann Intern Med. (1990) 113:501–6. doi: 10.7326/0003-4819-113-7-501
16. Berglund F, Killander J, Pompeius R. Effect of trimethoprim-sulfamethoxazole on the renal excretion of creatinine in man. J Urol. (1975) 114:802–8.
17. Kushner R, Gudivaka R, Schoeller D. Clinical characteristics influencing bioelectrical impedance analysis measurements. Am J Clin Nutr. (1996) 64:423S–7S. doi: 10.1093/ajcn/64.3.423S
18. Martin Bland J, Altman Douglas G. Statistical methods for assessing agreement between two methods of clinical measurement. Lancet. (1986) 327:307–10. doi: 10.1016/S0140-6736(86)90837-8
19. Demirovic J, Pai A, Pai M. Estimation of creatinine clearance in morbidly obese patients. Am J Health Syst Pharm. (2009) 66:642–8. doi: 10.2146/ajhp080200
20. Miyazawa I, Kadota A, Miura K, Okamoto M, Nakamura T, Ikai T, et al. Twelve-year trends of increasing overweight and obesity in patients with diabetes: the Shiga Diabetes Clinical Survey. Endocr J. (2018) 65:527–36. doi: 10.1507/endocrj.EJ17-0415
21. Horio M, Imai E, Yasuda Y, Watanabe T, Matsuo S. Modification of the CKD Epidemiology Collaboration (CKD-EPI) Equation for Japanese: Accuracy and Use for Population Estimates. Am J Kidney Dis. (2010) 56:32–8. doi: 10.1053/j.ajkd.2010.02.344
22. Ma Y, Zuo L, Chen J, Luo Q, Yu X, Li Y, et al. Modified glomerular filtration rate estimating equation for Chinese patients with chronic kidney disease. J Am Soc Nephrol JASN. (2006) 17:2937–44. doi: 10.1681/ASN.2006040368
23. Matsuo S, Imai E, Horio M, Yasuda Y, Tomita K, Nitta K, et al. Revised Equations for Estimated GFR From Serum Creatinine in Japan. Am J Kidney Dis. (2009) 53:982–92. doi: 10.1053/j.ajkd.2008.12.034
24. Matsuo M, Yamagishi F. Age-dependent error in creatinine clearance estimated by Cockcroft–Gault equation for the elderly patients in a Japanese hospital: a cross-sectional study. J Anesth. (2019) 33:155–8. doi: 10.1007/s00540-018-2596-3
25. Kera T, Kawai H, Hirano H, Kojima M, Watanabe Y, Fujiwara Y, et al. Comparison of body composition and physical and cognitive function between older Japanese adults with no diabetes, prediabetes and diabetes: A cross-sectional study in community-dwelling Japanese older people. Geriatr Gerontol Int. (2018) 18:1031–7. doi: 10.1111/ggi.13301
26. Tajiri Y, Kato T, Nakayama H, Yamada K. Reduction of skeletal muscle, especially in lower limbs, in Japanese type 2 diabetic patients with insulin resistance and cardiovascular risk factors. Metab Syndr Relat Disord. (2010) 8:137–42. doi: 10.1089/met.2009.0043
27. Macek P, Terek-Derszniak M, Biskup M, Krol H, Smok-Kalwat J, Gozdz S, et al. Assessment of Age-Induced Changes in Body Fat Percentage and BMI Aided by Bayesian Modelling: A Cross-Sectional Cohort Study in Middle-Aged and Older Adults. Clin Interv Aging. (2020) 15:2301–11. doi: 10.2147/CIA.S277171
28. Miyatake N, Takenami S, Kawasaki Y, Kunihashi Y, Nishikawa H, Numata T. Clinical evaluation of body fat percentage in 11,833 Japanese measured by air displacement plethysmograph. Intern Med Tokyo Jpn. (2005) 44:702–5. doi: 10.2169/internalmedicine.44.702
29. Chen K, Wee S, Pang B, Lau L, Jabbar K, Seah W, et al. Relationship between BMI with percentage body fat and obesity in Singaporean adults – The Yishun Study. BMC Public Health. (2021) 21:1030. doi: 10.1186/s12889-021-11070-7
30. McLester C, Nickerson B, Kliszczewicz B, McLester J. Reliability and Agreement of Various InBody Body Composition Analyzers as Compared to Dual-Energy X-Ray Absorptiometry in Healthy Men and Women. J Clin Densitom Off J Int Soc Clin Densitom. (2020) 23:443–50. doi: 10.1016/j.jocd.2018.10.008
31. Kolonko A, Pokora P, Słabiak-Błaż N, Czerwieńska B, Karkoszka H, Kuczera P, et al. The Relationship between Initial Tacrolimus Metabolism Rate and Recipients Body Composition in Kidney Transplantation. J Clin Med. (2021) 10:5793. doi: 10.3390/jcm10245793
32. Yun H, Ryoo S, Kim J, Choi Y, Park I, Shin G, et al. Trabecular bone score may indicate chronic kidney disease-mineral and bone disorder (CKD-MBD) phenotypes in hemodialysis patients: a prospective observational study. BMC Nephrol. (2020) 21:299. doi: 10.1186/s12882-020-01944-0
33. Akiyama Y, Matsuoka R, Masuda T, Iwamoto S, Sugie S, Muto T, et al. Comparative Impact of Isolated Ultrafiltration and Hemodialysis on Fluid Distribution: A Bioimpedance Study. Blood Purif. (2021): doi: 10.1159/000518228
34. Chen L, Liu L, Woo J, Assantachai P, Auyeung T, Bahyah K, et al. Sarcopenia in Asia: Consensus Report of the Asian Working Group for Sarcopenia. J Am Med Dir Assoc. (2014) 15:95–101. doi: 10.1016/j.jamda.2013.11.025
35. Urakami Y, Kimura N, Okuda M, Inui K. Creatinine Transport by Basolateral Organic Cation Transporter hOCT2 in the Human Kidney. Pharm Res. (2004) 21:976–81. doi: 10.1023/B:PHAM.0000029286.45788.ad
Keywords: cockcroft-gault equation, correction equation, diabetes, creatinine clearance, fat mass, body composition analyzer
Citation: Utsumi S, Kondo Y, Harada Y, Yoshida A, Nishimura H, Narita Y, Irie T, Jinnouchi H, Ishitsuka Y and Hirata S (2024) Evaluation of a creatinine clearance correction equation based on body fat mass in older Japanese patients with diabetes. Front. Med. 11:1228383. doi: 10.3389/fmed.2024.1228383
Received: 24 May 2023; Accepted: 22 January 2024;
Published: 08 February 2024.
Edited by:
Chia-Ter Chao, National Taiwan University Hospital, TaiwanReviewed by:
Cai-Mei Zheng, Taipei Medical University, TaiwanChih-Chien Sung, Tri-Service General Hospital, Taiwan
Copyright © 2024 Utsumi, Kondo, Harada, Yoshida, Nishimura, Narita, Irie, Jinnouchi, Ishitsuka and Hirata. This is an open-access article distributed under the terms of the Creative Commons Attribution License (CC BY). The use, distribution or reproduction in other forums is permitted, provided the original author(s) and the copyright owner(s) are credited and that the original publication in this journal is cited, in accordance with accepted academic practice. No use, distribution or reproduction is permitted which does not comply with these terms.
*Correspondence: Yuki Kondo, ykondo@kumamoto-u.ac.jp; Sumio Hirata, hirata@kumamoto-u.ac.jp
†These authors have contributed equally to this work