- Department of Orthopaedic Surgery, Faculty of Medicine, Saga University, Saga, Japan
Lumbar spinal canal stenosis (LSS) is characterized by gait abnormalities, and objective quantitative gait analysis is useful for diagnosis and treatment. This review aimed to provide a review of objective quantitative gait analysis in LSS and note the current status and potential of smart shoes in diagnosing and treating LSS. The characteristics of gait deterioration in LSS include decreased gait velocity and asymmetry due to neuropathy (muscle weakness and pain) in the lower extremities. Previous laboratory objective and quantitative gait analyses mainly comprised marker-based three-dimensional motion analysis and ground reaction force. However, workforce, time, and costs pose some challenges. Recent developments in wearable sensor technology and markerless motion analysis systems have made gait analysis faster, easier, and less expensive outside the laboratory. Smart shoes can provide more accurate gait information than other wearable sensors. As only a few reports exist on gait disorders in patients with LSS, future studies should focus on the accuracy and cost-effectiveness of gait analysis using smart shoes.
1 Introduction
With the advent of an aging society, lumbar spinal canal stenosis (LSS) is a growing and common problem, causing a major health burden worldwide, clinically and socioeconomically (1–8). Although the natural history of LSS is diverse, a progressive loss of function often occurs over time (3, 4). Therefore, early diagnosis and treatment may improve the prognosis of this disease (3, 4).
For early diagnosis of LSS, it is necessary to combine data from various objective biomarkers with self-reported symptoms, standard neurological findings (sensory, motor and reflexes) and imaging studies to improve the accuracy of the diagnostic algorithm. In the further development of digitization throughout healthcare, the more objective term “digital biomarker” has been used to describe this approach in medicine (9–11). Digital biomarkers are classified as physiological indicators (heart rate, pulse, and blood pressure) and behavioral indicators (gait and posture). They are used in fields ranging from sports support to medicine (9–11). Gait is an important biomarker for diagnosing and assessing disease status, as gait patterns are altered in patients with LSS. Objective gait analysis has traditionally been performed in a laboratory, and the recent development and availability of wearable sensor technology have provided a faster, easier, and less expensive method for analysis (3–5). An increasing number of reports have shown that gait analysis using digital biomarkers with wearable sensors can aid in LSS diagnosis, severity, and prognosis (3–5). Wearable sensors, including smartphones, smartwatches, and smart shoes, also known as the Internet of Medical Things (IoMT), are used in medicine and sports owing to their high adherence to daily portable products. Because smart shoes enable a more accurate biomechanical analysis of the ankle joint than smartphones or smart watches owing to the predefined rigid sensor positions in the shoes, studies on gait analysis using smart shoes have increased dramatically in recent years [(12); Figure 1].
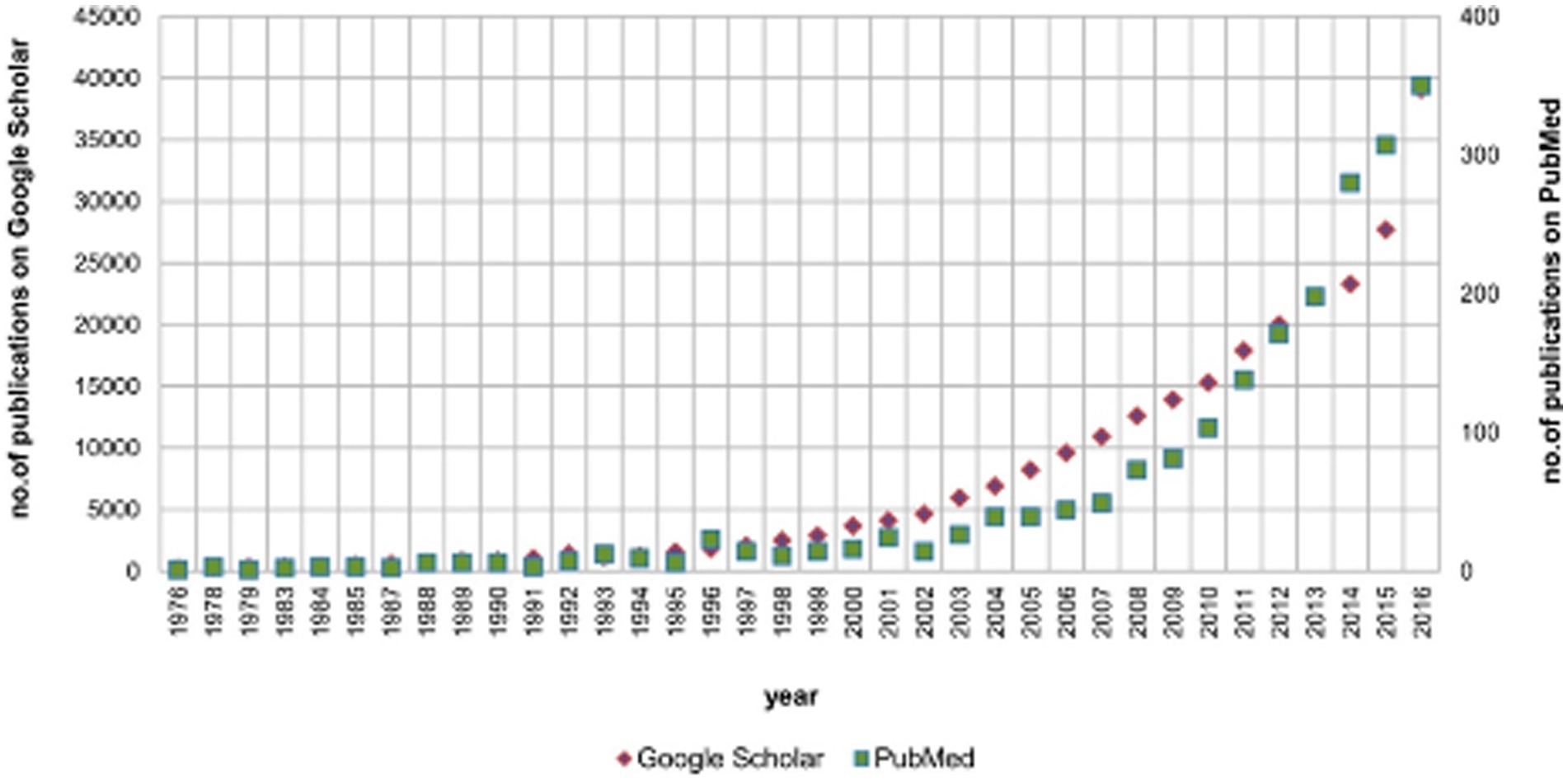
Figure 1. The annual number of publications on gait monitoring with smart shoes using PubMed and Google Scholar. The search criteria included “(gait OR shoe OR walking) AND (inertial OR IMU OR sensor OR wearable).” IMU, inertial measurement unit. Adapted from reference (12) with permission from MDPI.
However, studies using smart shoes have focused on cardiovascular diseases, sports medicine, and neurological diseases (stroke and Parkinson’s disease), with only a few reports on LSS, although gait abnormalities is a major symptom (4, 5, 13).
This review aimed to provide a scoping review of objective quantitative gait analysis using digital biomarkers in LSS and to note the current status and potential of smart shoes in diagnosing and treating LSS. The scarcity of reports on smart shoes for gait analysis in spinal disease and the heterogeneity of study designs, outcome measures, and variability prevents meta-analyses and adequate systematic reviews. A scoping review cannot locate all relevant literature and cover the scientific literature without bias. Instead, it will discuss the important papers that the authors know about. Thus, this study employed the scoping review method, which allows for a broader, more flexible, and more comprehensive organization and analysis of the existing literature compared to a systematic review.
For this purpose, we also selected many important papers published in peer-reviewed scientific journals and cited extensively the major papers in LSS gait analysis without any deadline restrictions.
2 Digital biomarkers in gait analysis
Digital biomarkers that objectively and temporally measure the physiological data of daily life, which were previously difficult to obtain using wearable sensors such as smartphones, smartwatches, and smart shoes, have been attracting attention (9–11). The emergence of digital biomarkers has revolutionized the measurement of physiological data in daily life. Typical digital biomarkers obtained from wearable sensors include vital signs, electrocardiogram, sleep, activity (daily steps, running distance, and calories burned), and gait analysis (9–11). Digital biomarkers obtained from wearable sensors are characterized by their noninvasiveness, long duration (outside the hospital), variety, and large volume of data. Biomarkers are classified according to the timing of the medical intervention: susceptibility/risk biomarkers and diagnostic biomarkers before diagnosis, prognostic/predictive biomarkers and pharmacodynamic/response biomarkers during diagnosis, safety biomarkers during treatment, and endpoint (surrogate) biomarkers and monitoring biomarkers from diagnosis to treatment efficacy (9–11). Therefore, various digital biomarkers derived from gait analysis have the potential to create new clinical value for the diagnosis, treatment, monitoring, and prognostic inference of LSS.
3 Trends in gait analysis in the laboratory and beyond
Gait analysis has evolved with technological advances, from purely observational to instrumental methods. Characteristic gait abnormalities observed in LSS include painful claudication and a steppage gait. Observational gait analysis is simple and equipment-free; however, it is inherently subjective, and its validity and reliability depend on the examiner’s skill and experience (14). Objective and quantitative gait analysis helps in understanding the pathophysiology of bipedal walking, identifying treatment focus areas, and optimally monitoring changes in the patient’s condition (15). In the clinical and research fields, the most commonly used simple quantitative assessments are the 10-meter walk test for the most comprehensive index of walking speed, the 6-min walk test for assessing walking endurance, and the Timed Up and Go test for applied walking ability (16, 17). Walking speed affects daily mobility functions directly. Furthermore, walking speed and range of motion of the lower limbs were positively correlated, with 1.0 m/s being the speed at which a person can cross a pedestrian crossing and 0.7 m/s indicating a high risk of falling (15–17). The 6-min walk test and the Time Up and Go test can now be easily measured using free smartphone apps. However, these simple assessments do not specifically identify the aspects of gait that differ from those of a healthy gait.
In contrast to performance measures such as gait speed, instrumental quantitative gait analysis contributes to identifying causes that impair bipedal stability and efficiency and events and conditions that should be focused on during treatment. Instrumental quantitative gait analysis is commonly performed according to standard methods based on kinematic analysis of the displacement of body parts during walking (three-dimensional (3D) motion analysis), kinematic analysis of the external forces acting on the body (ground reaction forces), and electromyographic analysis of the muscle activity involved in the walking movement to examine gait parameters, such as spatial (length), temporal (duration), or derived indices (asymmetry, variability) (3, 15). Because these measures can be obtained using multiple inputs from different gait sites, they show high recognition rates and are crucial for classifying and quantifying gait disorders (3, 15, 16). Kinematic measurements can be obtained from any recording device linked to a computer (e.g., motion capture systems or inertial measurement units). The 3D analysis focuses on body movements, and the mainstream approach is optical. Markers attached to various body parts are photographed using a semiconductor camera, and the displacement, angular velocity, angular acceleration, stride length, and stride width of joint movements are calculated (18). Commonly used spatiotemporal gait metrics for quantitative evaluation include spatial (step and stride length) and temporal (step and stride time) parameters, spatiotemporal (walking speed and cadence: composite parameters derived from spatial and temporal variables) parameters, gait asymmetry, gait variability (Table 1), and joint angles (3).

Table 1. Spatiotemporal gait metrics: spatial, temporal, spatiotemporal, gait asymmetry, gait variability.
For kinetic analysis, ground (foot) force reaction (GRF) analysis, including foot pressure analysis, was used to measure the magnitude, direction, and location of the application (19, 20). Adding 3D analysis data to GRF or electromyography data can provide a more comprehensive depiction of the gait. The marker-based system device is the traditionally used and highly accurate method, which combines 3D motion analysis (video analysis, optical motion tracking and analysis, multi-sensor, or gyroscope), electromyography, and GRF analysis in the laboratory for gait analysis (i.e., VICON) [Figure 2; (19, 20)].
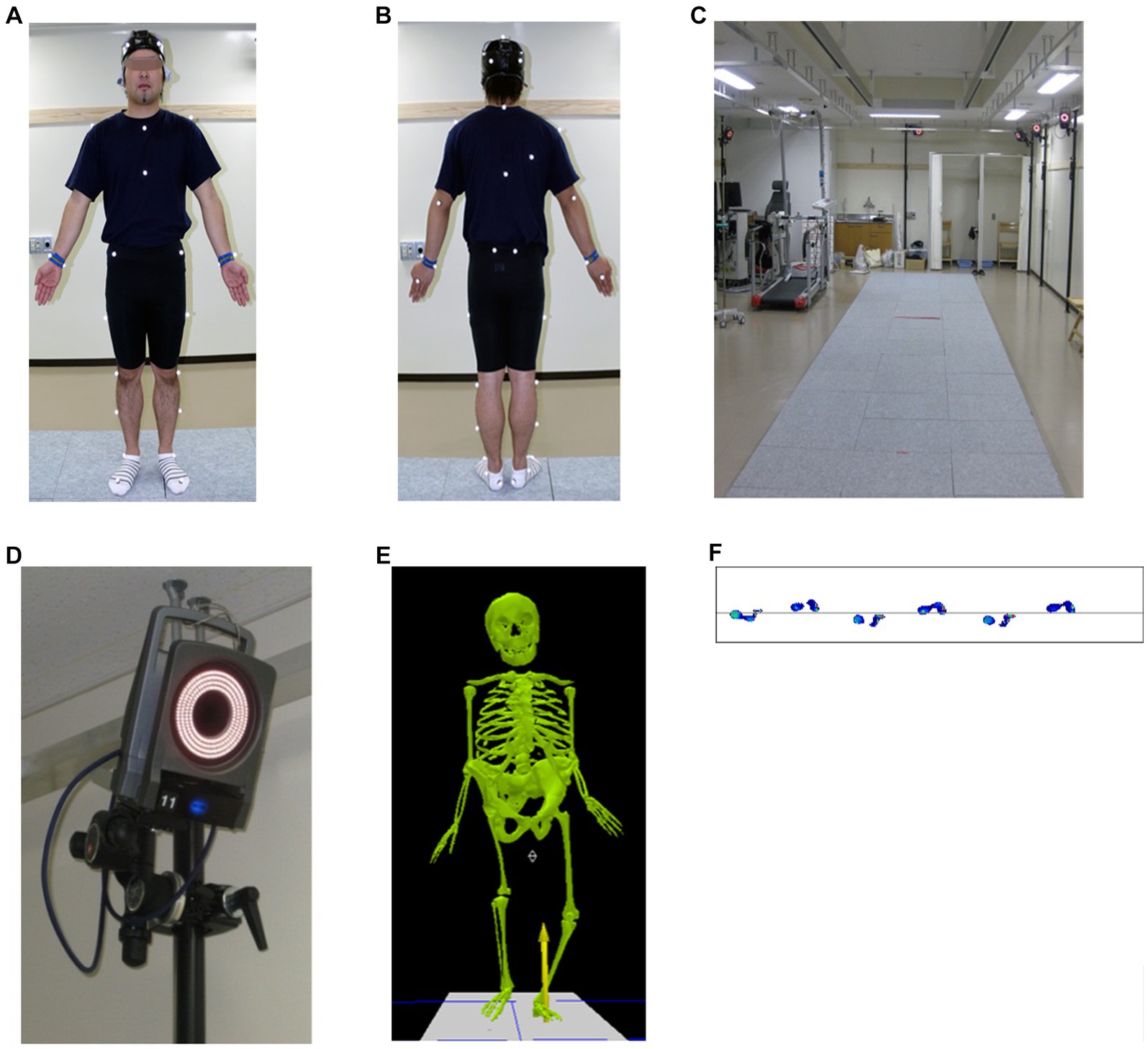
Figure 2. Vicon Motion System™, Oxford, UK. (A,B) Thirty-five infrared reflective markers are attached to the body surface. (C) Patients were asked to walk freely on an approximately 8 m walking path with a ground reaction force meter installed in the center of the path (D) and photographed by 14 infrared cameras. The infrared reflective markers were positioned using the plug-in-gait model at Saga University. The video motion and ground (foot) force reaction data were seamlessly merged to enable spatiotemporal and dynamic evaluation of gait abnormalities (E,F).
A combined analysis of 3D motion and digital biomarker data obtained from ground reaction forces and electromyograms will improve understanding of the indices of spatial and temporal factors in the gait cycle, characteristics of the center of gravity movement that contribute to gait efficiency, and the relationship between joint motion and muscle activity in the lower limbs and trunk. However, laboratory gait analyses, including marker-based 3D motion capture systems, GRF, and electromyography, have disadvantages regarding space, equipment, time, workforce, cost, technical expertise, and exhaustive data analysis, making their clinical application difficult (21). There is also the problem of the “Hawthorne effect” in which people consciously alter their gait because they know that they were monitored (21) and the “white coat effect” (22), in which tension in an unfamiliar environment can alter patient performance. In addition, marker-based gait analysis requires subjects to expose their skin for accurate marker placement to obtain more accurate data, which may cause inconvenience (23). Recently, the accuracy of markerless 3D measurements, such as Media Pipe1 and OpenPose,2 has improved; these require no expertise or special cameras, are free for noncommercial use, and are expected to expand opportunities for clinical applications (23). Notably, lower limb range of motion (ROM) was measured in the sagittal plane using OpenPose from images taken with a single digital camera (23). Although OpenPose cannot substitute a complete 3D motion analysis system, it can be used for gait analysis (23). OpenPose is a markerless system without special cameras, thus reducing analysis costs and time. Thus, the development and increased availability of wearable sensor and video analysis technology, especially markerless systems using human posture tracking algorithms, has provided a faster, easier, less expensive, and more representative way to measure regular walking patterns (or ‘free-living’ gait) outside the laboratory as an alternative to marker-based gait analysis in the laboratory (3–5, 24).
Wearable sensors and markerless 3D measurement can provide a more accurate assessment of a patient’s gait and posture in “everyday life,” which may not be reflected in tests performed by a physician in the hospital or outside the laboratory. Therefore, combining wearable sensors and markerless 3D measurement (OpenPose, Media Pipe) could be a “game changer” in motion and gait analysis.
4 Summary of publications on objective quantitative gait analysis using digital biomarkers in LSS
The most characteristic clinical presentation of LSS is neurogenic intermittent claudication, which causes pain and numbness from the buttocks to the lower extremities on one or both sides during walking, resulting in a slower walking speed and shorter total walking distance (3, 25). A systematic literature review by Wang et al. in 2022 revealed that most conventional quantitative gait analyses of LSS were performance-oriented studies on walking speed and distance, such as motorized treadmill trials (24 publications) and timed up-and-go trials (19 publications) (24).
Patients with LSS often have postures that cause the lumbar spine to flex more to maximize spinal canal volume and minimize pain and symptoms during walking, leading to postural abnormalities (25, 26). In addition to lower-extremity pain, muscle weakness and sensory disturbances can result in balance dysfunction (24, 26, 27). Furthermore, changes in sagittal spinal alignment may affect the hips (28, 29) and knees (30). Kinematic (3D motion analysis), kinetic (GRF), and electromyographic (EMG) analyses of gait can produce abnormalities in spatial, temporal, or derived indices (asymmetry and variability) of gait. These observations were made upon reflecting on these LSS-induced lower-extremity neuropathies and alignment abnormalities in the spine and lower-extremity joints from objective quantitative gait analysis using instruments (3, 16).
Table 2 summarizes the publications on objective quantitative gait analysis using digital biomarkers in LSS. Although most studies have investigated spatiotemporal gait metrics (spatial, temporal, spatiotemporal, gait asymmetry, gait variability), only a few investigated trunk and lower-extremity joint angles, plantar pressure distribution, and EMG (Table 3).
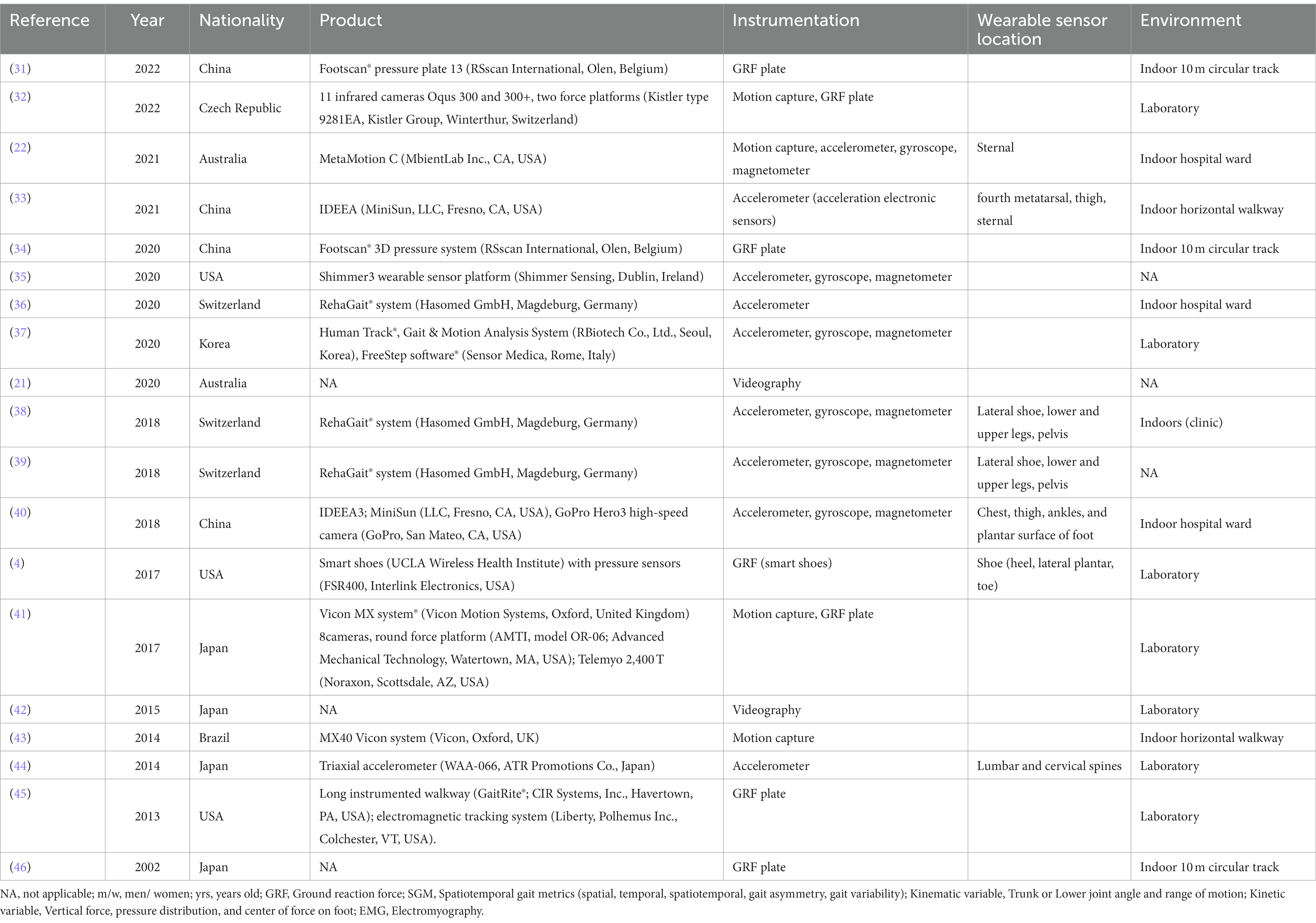
Table 2. Summary of publications on objective quantitative gait analysis using digital biomarkers in LSS.
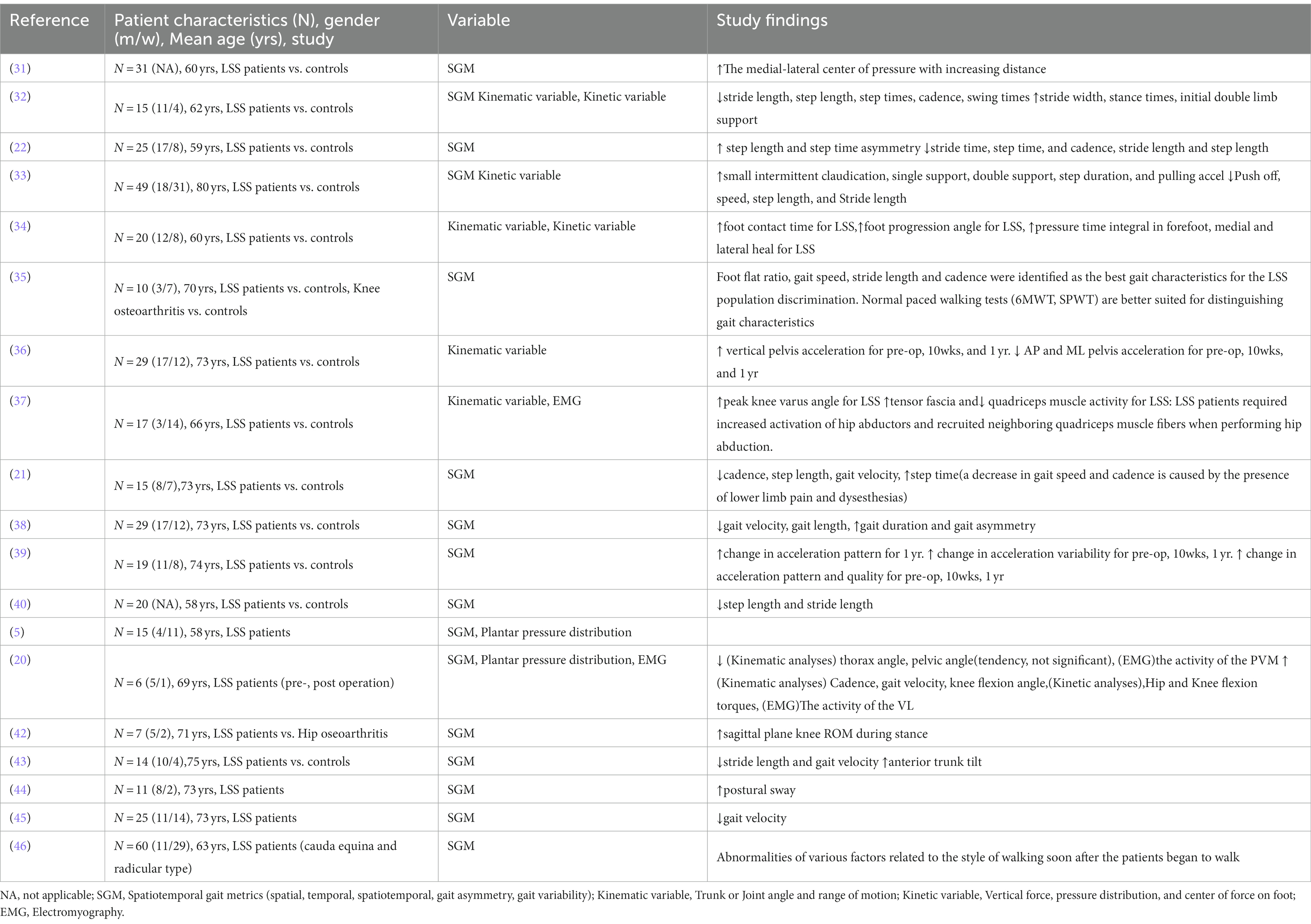
Table 3. Summary of publications on spatiotemporal gait metrics, Kinematic and Kinetic variable and EMG in LSS.
The characteristics of gait deterioration in patients with LSS compared to those in healthy subjects include decreased gait velocity (35, 38, 42, 44, 46), decreased time or length of gait (step or stride) (21, 22, 28, 32, 33, 35, 38, 40, 44), decreased cadence (21, 22, 35, 42), gait asymmetry (38), and prolonged gait duration (21, 22, 32, 35, 38). Kinematic analysis showed that LSS decreased hip ROM (42), increased knee ROM (42) and lumbar flexion (anterior trunk tilt) in the sagittal plane (44), and increased the foot contact time and progression angle (34). This observation may be due to neuropathy (muscle weakness and pain) in the lower extremities caused by LSS. For the EMG variables, muscle activity in the LSS was higher in the tensor fascia, quadriceps (37), and vastus lateralis muscles (20). Additionally, muscle activity was lower in the paravertebral muscles (20) of patients with LSS than in healthy controls (Table 4). Although the number of reports on the gait analysis of LSS using wearable sensors has increased (33, 44), only two studies on smart shoes were written by the same authors (4, 5).
5 Smart shoes: status quo and quo vadis
Smart shoes are ordinary shoes with technological innovations, such as biometric data recording and automatic size adjustment according to the individual (13). Shoes with at least one actuator or sensor built in are “smart.” Leading companies have developed smart shoes incorporating various technologies, including pressure sensors, accelerometers, gyro sensors, piezoelectric pedometers, and Bluetooth. These smart shoes can analyze posture, gait patterns, and ankle momentum and measure the number of steps and calories burned via a smart app (13); they include Lechal Shoes that navigate using GPS (13, 47), Google’s talking shoes (48), Adidas’ Micropacer (49), Nike’s Adapt BB, Puma’s Fit Intelligence, Samsung’s IOFIT, and Asics’ EVORIDE ORPHE.
The shoe incorporates pressure, acceleration, and gyroscope sensors to track the user’s activity. Real-time feedback can be provided by connecting it to a personal computer or smartphone. Asics’ EVORIDE ORPHE enables multifaceted gait analysis by linking 3D motion analysis using OpenPose from videos captured by a single digital camera with kinematics and GRF data obtained from smart shoes (Figure 3). However, no comparisons have been made between marker-based 3D movement analysis (numerous video cameras and infrared markers) combined with GRF measurements in the laboratory (Figure 2) and markerless 3D movement analysis outside the laboratory using low-cost and convenient smart shoes and a single digital camera on a smartphone in patients with LSS. This aspect requires further exploration.
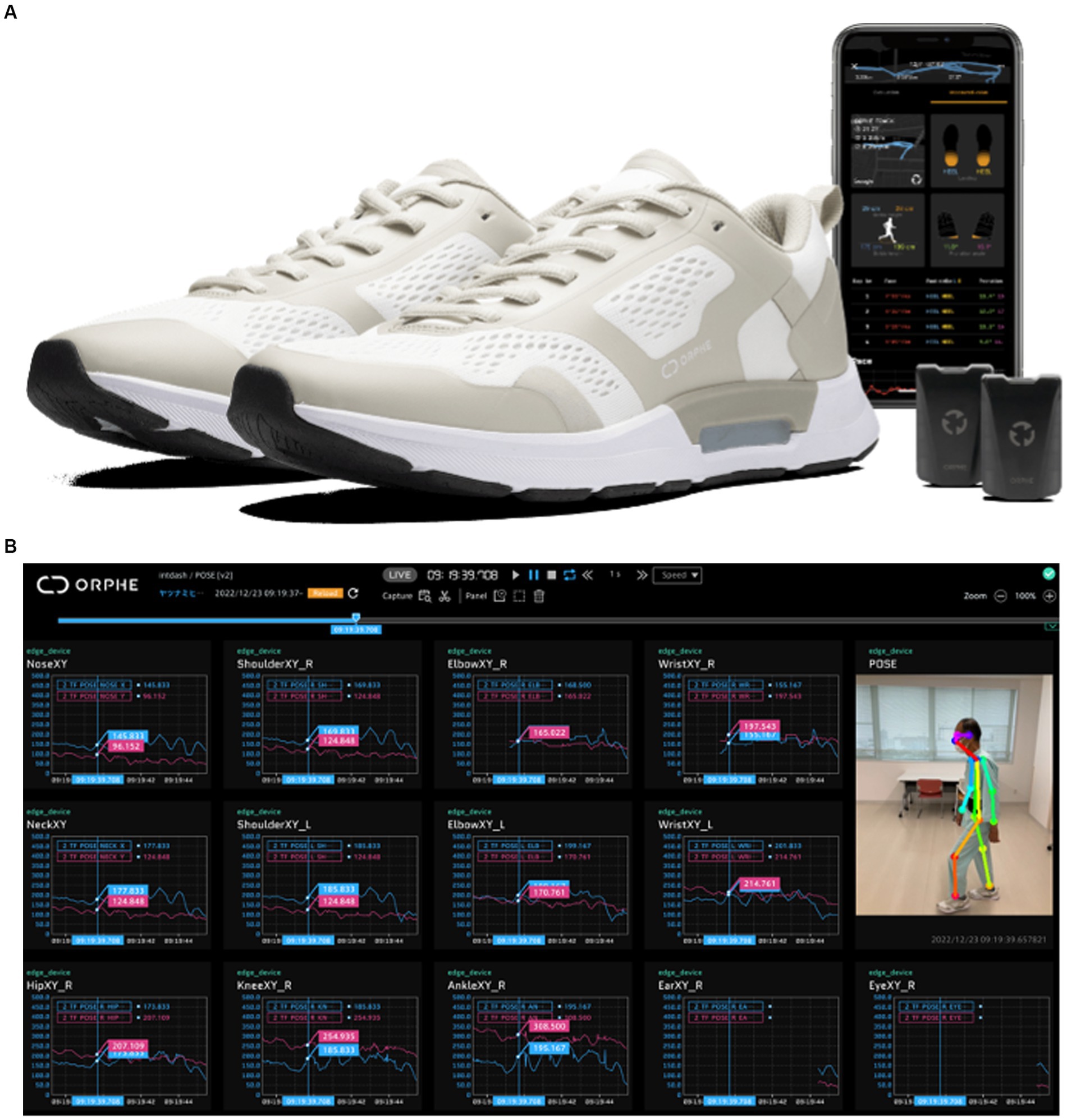
Figure 3. Asics’ EVORIDE ORPHE smart shoes can measure the time of each segment of the gait cycle, landing and departure angles, spatiotemporal evaluation of gait using 6-axis (3-axis acceleration, 3-axis angular velocity) motion sensors built into the plantar surface, and indicators for gait evaluation such as ankle joint angle and plantar pressure [landing impact, ground (foot) force reaction]. (A) Is adapted from https://orphe.io/presswith permission of ORPHE. (B) Linkage with 3D motion analysis was done by linking the videos captured by a single digital camera with multifaceted gait analysis using OpenPose.
Biofeedback systems combined with smart shoes can prevent injuries in runners (50, 51), prevent and detect falls in older patients (50, 52), monitor posture in patients with back pain (52), and detect gait abnormalities in osteoarthritis to prevent joint damage (53). Moreover, Bluetooth-and Wi-Fi-capable smart shoes can help the visually impaired navigate their destinations using Google Maps functionality (13, 54). Smart shoes are a useful tool for evaluating gait analysis because they (1) have predefined rigid sensor positions on the soles for accurate and flexible biomechanical analysis, (2) can monitor the highly fixed movement of gait and automatically assess functional biomechanics, and (3) are discreet and non-stigmatizing to incorporate, improve patient acceptance and long-term adherence, and allow gait to be assessed spatiotemporally and mechanically (12). When comparing the accuracy of the number of steps by wearing the sites at the hip, buttock, thigh, ankle, and wrist, the ankle joint showed the highest accuracy (55). Therefore, smart shoes are more suitable as wearable sensors for gait analysis than smartphones or smartwatches because they provide more gait information (gait asymmetry and GRF) (4, 5, 12, 56).
Studies on smart shoe gait analyses have increased dramatically in recent years (12). However, they have focused on cardiovascular diseases, sports medicine, and neurological diseases (stroke and Parkinson’s disease), with only a few on degenerative spinal diseases, although gait abnormalities is a major symptom (4, 5, 12). This may be because wearables have only recently emerged as practical tools to assist health management. Smart shoes enable the long-term recording and analysis of superficial information, including walking distance, walking time, and calories burned, which can be obtained from smartphones and smartwatches, and stride length, landing angle and impact, the area where the foot touches the ground, and changes in walking style (4, 5, 12, 56). Smartphones may motivate runners and patients to exercise by encouraging behavioral changes through daily step challenges and goal setting. Furthermore, insole-based systems can easily measure several parameters related to lower-extremity health, such as plantar pressure, body temperature, pulse rate, and gait dynamics (4, 5, 12). Thus, these data-collecting smart shoes are similar to the IoMT.
Accumulating gait data and machine learning algorithms may help establish a warning system for faster and better fall response. Therefore, accurate gait analysis data from smart shoes can help in the early detection, assessment of fall risk, treatment decisions, monitoring of treatment, and outcome evaluation of diseases that cause gait disorders, including LSS. Outcome measurements will shift from being subjective to combining subjective and objective measurement tools derived from digital biomarkers. Information from wearable sensors other than smart shoes will be integrated with artificial intelligence to provide useful information for healthcare providers regarding treatment. With the entry of major shoe companies, market penetration of smart shoes with high comfort and convenience is expected to increase rapidly. However, reports on the efficacy of smart shoes for gait analysis in LSS, usability, data security, and cost-effectiveness are lacking (57). The legal system may be unable to keep pace with advances in connected medical product technology, and data security must be a top priority, particularly concerning patient information.
6 Conclusion
Proper diagnosis and treatment of LSS require objective and subjective methods of assessment. Objective quantitative gait analysis and subjective patient assessment are useful for diagnosis, prevention, therapeutic intervention, treatment management, and outcome assessment. Although objective quantitative methods of gait analysis have been performed using laboratory-based 3D motion analysis, ground reaction force, and electromyography, challenges may occur regarding workforce, time, expertise, and cost. Wearable sensor technology (especially smart shoes) and markerless motion analysis systems have made it possible to replace conventional gait analysis with markers in the laboratory, which is faster, simpler, cheaper, and more reflective of everyday life. Using smart shoes for gait analysis shows great potential; however, evaluating their accuracy and cost-effectiveness is crucial. Future studies should aim to address these concerns and provide more insight into the use of smart shoes for gait analysis in the diagnosis, treatment management, and outcome assessment of LSS. These advances in technology and methods will help healthcare professionals provide better care for patients with LSS.
Author contributions
TM: Conceptualization, Data curation, Investigation, Writing – original draft. HH: Conceptualization, Methodology, Supervision, Visualization, Writing – original draft. TK: Data curation, Formal analysis, Writing – original draft. MT: Supervision, Validation, Visualization, Writing – original draft. TY: Supervision, Validation, Writing – original draft. YT: Investigation, Methodology, Writing – original draft. MM: Supervision, Validation, Writing – review & editing.
Funding
This study was supported by grants from JOASubsidized Science Project Research 2020-2.
Conflict of interest
The authors declare that the research was conducted in the absence of any commercial or financial relationships that could be construed as a potential conflict of interest.
Publisher’s note
All claims expressed in this article are solely those of the authors and do not necessarily represent those of their affiliated organizations, or those of the publisher, the editors and the reviewers. Any product that may be evaluated in this article, or claim that may be made by its manufacturer, is not guaranteed or endorsed by the publisher.
Footnotes
1. ^https://google.github.io/mediapipe/
2. ^https://cmu-perceptual-computing-lab.github.io/openpose/web/html/doc/index.html
References
1. GBD 2015 Disease and Injury Incidence and Prevalence Collaborators. Global, regional, and national incidence, prevalence, and years lived with disability for 310 diseases and injuries, 1990-2015: a systematic analysis for the global burden of disease study 2015. Lancet. (2016) 388:1545–602. doi: 10.1016/s0140-6736(16)31678-6
2. Mallow, GM, Siyaji, ZK, Galbusera, F, Espinoza-Orías, AA, Giers, M, Lundberg, H, et al. Intelligence-based spine care model: a new era of research and clinical decision-making. Global Spine J. (2021) 11:135–45. doi: 10.1177/2192568220973984
3. Natarajan, P, Fonseka, RD, Kim, S, Betteridge, C, Maharaj, M, and Mobbs, RJ. Analysing gait patterns in degenerative lumbar spine diseases: a literature review. J Spine Surg. (2022) 8:139–48. doi: 10.21037/jss-21-91
4. Lee, SI, Campion, A, Huang, A, Park, E, Garst, JH, Jahanforouz, N, et al. Identifying predictors for postoperative clinical outcome in lumbar spinal stenosis patients using smart-shoe technology. J Neuroeng Rehabil. (2017) 14:77. doi: 10.1186/s12984-017-0288-0
5. Lee, SI, Park, E, Huang, A, Mortazavi, B, Garst, JH, Jahanforouz, N, et al. Objectively quantifying walking ability in degenerative spinal disorder patients using sensor equipped smart shoes. Med Eng Phys. (2016) 38:442–9. doi: 10.1016/j.medengphy.2016.02.004
6. Ishimoto, Y, Yoshimura, N, Muraki, S, Yamada, H, Nagata, K, Hashizume, H, et al. Associations between radiographic lumbar spinal stenosis and clinical symptoms in the general population: the Wakayama Spine Study. Osteoarthr Cartil. (2013) 21:783–8. doi: 10.1016/j.joca.2013.02.656
7. Weber, C, Giannadakis, C, Rao, V, Jakola, AS, Nerland, U, Nygaard, ØP, et al. Is there an association between radiological severity of lumbar spinal stenosis and disability, pain, or surgical outcome? a multicenter observational study. Spine (Phila Pa 1976). (2016) 41:E78–83. doi: 10.1097/brs.0000000000001166
8. Ramirez-Bautista, JA, Hernández-Zavala, A, Chaparro-Cárdenas, SL, and Huerta-Ruelas, JA. Review on plantar data analysis for disease diagnosis. Biocybern Biomed Eng. (2018) 38:342–61. doi: 10.1016/j.bbe.2018.02.004
9. Vasudevan, S, Saha, A, Tarver, ME, and Patel, B. Digital biomarkers: convergence of digital health technologies and biomarkers. NPJ Digit Med. (2022) 5:36. doi: 10.1038/s41746-022-00583-z
10. Coravos, A, Goldsack, JC, Karlin, DR, Nebeker, C, Perakslis, E, Zimmerman, N, et al. Digital medicine: a primer on measurement. Digit Biomark. (2019) 3:31–71. doi: 10.1159/000500413
11. Babrak, LM, Menetski, J, Rebhan, M, Nisato, G, Zinggeler, M, Brasier, N, et al. Traditional and digital biomarkers: two worlds apart? Digit Biomark. (2019) 3:92–102. doi: 10.1159/000502000
12. Eskofier, B, Lee, S, Baron, M, Simon, A, Martindale, C, Gaßner, H, et al. An overview of smart shoes in the internet of health things: gait and mobility assessment in health promotion and disease monitoring. Appl Sci. (2017) 7:986. doi: 10.3390/app7100986
13. Gokalgandhi, D, Kamdar, L, Shah, N, and Mehendale, N. A review of smart technologies embedded in shoes. J Med Syst. (2020) 44:150. doi: 10.1007/s10916-020-01613-7
14. Wren, TA, Rethlefsen, S, and Kay, RM. Prevalence of specific gait abnormalities in children with cerebral palsy: influence of cerebral palsy subtype, age, and previous surgery. J Pediatr Orthop. (2005) 25:79–83. doi: 10.1097/00004694-200501000-00018
15. Gor-García-Fogeda, MD, Cano de la Cuerda, R, Carratalá Tejada, M, Alguacil-Diego, IM, and Molina-Rueda, F. Observational gait assessments in people with neurological disorders: a systematic review. Arch Phys Med Rehabil. (2016) 97:131–40. doi: 10.1016/j.apmr.2015.07.018
16. Stienen, MN, Ho, AL, Staartjes, VE, Maldaner, N, Veeravagu, A, Desai, A, et al. Objective measures of functional impairment for degenerative diseases of the lumbar spine: a systematic review of the literature. Spine J. (2019) 19:1276–93. doi: 10.1016/j.spinee.2019.02.014
17. Shimada, H, Suzukawa, M, Tiedemann, A, Kobayashi, K, Yoshida, H, and Suzuki, T. Which neuromuscular or cognitive test is the optimal screening tool to predict falls in frail community-dwelling older people? Gerontology. (2009) 55:532–8. doi: 10.1159/000236364
18. Schniepp, R, Möhwald, K, and Wuehr, M. Clinical and automated gait analysis in patients with vestibular, cerebellar, and functional gait disorders: perspectives and limitations. J Neurol. (2019) 266:118–22. doi: 10.1007/s00415-019-09378-x
19. Gonçalves, SB, Lama, SBC, and Silva, MTD. Three decades of gait index development: a comparative review of clinical and research gait indices. Clin Biomech (Bristol, Avon). (2022) 96:105682. doi: 10.1016/j.clinbiomech.2022.105682
20. Khodadadeh, S, and Eisenstein, SM. Gait analysis of patients with low back pain before and after surgery. Spine (Phila Pa 1976). (1993) 18:1451–5.
21. Perring, J, Mobbs, R, and Betteridge, C. Analysis of patterns of gait deterioration in patients with lumbar spinal stenosis. World Neurosurg. (2020) 141:e55–9. doi: 10.1016/j.wneu.2020.04.146
22. Betteridge, C, Mobbs, RJ, Fonseka, RD, Natarajan, P, Ho, D, Choy, WJ, et al. Objectifying clinical gait assessment: using a single-point wearable sensor to quantify the spatiotemporal gait metrics of people with lumbar spinal stenosis. J Spine Surg. (2021) 7:254–68. doi: 10.21037/jss-21-16
23. Ota, M, Tateuchi, H, Hashiguchi, T, and Ichihashi, N. Verification of validity of gait analysis systems during treadmill walking and running using human pose tracking algorithm. Gait Posture. (2021) 85:290–7. doi: 10.1016/j.gaitpost.2021.02.006
24. Wang, J, Ullah, S, Solano, MA, Overley, SC, Bumpass, DB, and Mannen, EM. Changes in kinematics, kinetics, and muscle activity in patients with lumbar spinal stenosis during gait: systematic review. Spine J. (2022) 22:157–67. doi: 10.1016/j.spinee.2021.06.003
25. Takahashi, K, Kagechika, K, Takino, T, Matsui, T, Miyazaki, T, and Shima, I. Changes in epidural pressure during walking in patients with lumbar spinal stenosis. Spine (Phila Pa 1976). (1995) 20:2746–9. doi: 10.1097/00007632-199512150-00017
26. Chakravorty, A, Mobbs, RJ, Anderson, DB, Rooke, K, Phan, K, Yoong, N, et al. The role of wearable devices and objective gait analysis for the assessment and monitoring of patients with lumbar spinal stenosis: systematic review. BMC Musculoskelet Disord. (2019) 20:288. doi: 10.1186/s12891-019-2663-4
27. Thornes, E, Robinson, HS, and Vøllestad, NK. Dynamic balance in patients with degenerative lumbar spinal stenosis; a cross-sectional study. BMC Musculoskelet Disord. (2018) 19:192. doi: 10.1186/s12891-018-2111-x
28. Offierski, CM, and Mac, NI. Hip-spine syndrome. Spine (Phila Pa 1976). (1983) 8:316–21. doi: 10.1097/00007632-198304000-00014
29. Morimoto, T, Kitajima, M, Tsukamoto, M, Yoshihara, T, Sonohata, M, and Mawatari, M. Sagittal spino-pelvic alignment in rapidly destructive coxarthrosis. Eur Spine J. (2018) 27:475–81. doi: 10.1007/s00586-017-5282-5
30. Goodman, SB, Lachiewicz, PF, Liu, N, and Wood, KB. Knee or spine surgery first? A survey of treatment order for patients with concurrent degenerative knee and lumbar spinal disorders. J Arthroplast. (2020) 35:2039–43. doi: 10.1016/j.arth.2020.03.018
31. Wei, W, Jin, Y, Jiang, M, Li, L, Yan, W, Wang, H, et al. Objective evaluation of neurogenic intermittent claudication for patients with lumbar spinal stenosis based on plantar pressure analysis. Spine (Phila Pa 1976). (2022) 47:1746–52. doi: 10.1097/brs.0000000000004444
32. Lodin, J, Jelínek, M, Sameš, M, and Vachata, P. Quantitative gait analysis of patients with severe symptomatic spinal stenosis utilizing the gait profile score: an observational clinical study. Sensors (Basel). (2022) 22. doi: 10.3390/s22041633
33. Li, YG, Li, LP, Li, ZJ, Li, H, Li, Y, Li, Q, et al. Gait analysis in the elderly patients with lumbar spinal stenosis. Int Orthop. (2021) 45:673–9. doi: 10.1007/s00264-020-04935-w
34. Wei, W, Xu, C, Yang, XJ, Lu, CB, Lei, W, and Zhang, Y. Analysis of dynamic plantar pressure before and after the occurrence of neurogenic intermittent claudication in patients with lumbar spinal stenosis: an observational study. Biomed Res Int. (2020) 2020:5043583–9. doi: 10.1155/2020/5043583
35. Odonkor, C, Kuwabara, A, Tomkins-Lane, C, Zhang, W, Muaremi, A, Leutheuser, H, et al. Gait features for discriminating between mobility-limiting musculoskeletal disorders: lumbar spinal stenosis and knee osteoarthritis. Gait Posture. (2020) 80:96–100. doi: 10.1016/j.gaitpost.2020.05.019
36. Bumann, H, Nüesch, C, Loske, S, Byrnes, SK, Kovacs, B, Janssen, R, et al. Severity of degenerative lumbar spinal stenosis affects pelvic rigidity during walking. Spine J. (2020) 20:112–20. doi: 10.1016/j.spinee.2019.08.016
37. Kim, JJ, Cho, H, Park, Y, Jang, J, Kim, JW, and Ryu, JS. Biomechanical influences of gait patterns on knee joint: kinematic & EMG analysis. PLoS One. (2020) 15:e0233593. doi: 10.1371/journal.pone.0233593
38. Loske, S, Nüesch, C, Byrnes, KS, Fiebig, O, Schären, S, Mündermann, A, et al. Decompression surgery improves gait quality in patients with symptomatic lumbar spinal stenosis. Spine J. (2018) 18:2195–204. doi: 10.1016/j.spinee.2018.04.016
39. Byrnes, SK, Nüesch, C, Loske, S, Leuenberger, A, Schären, S, Netzer, C, et al. Inertial sensor-based gait and attractor analysis as clinical measurement tool: functionality and sensitivity in healthy subjects and patients with symptomatic lumbar spinal stenosis. Front Physiol. (2018) 9:1095. doi: 10.3389/fphys.2018.01095
40. Sun, J, Liu, YC, Yan, SH, Wang, SS, Lester, DK, Zeng, JZ, et al. Clinical gait evaluation of patients with lumbar spine stenosis. Orthop Surg. (2018) 10:32–9. doi: 10.1111/os.12367
41. Goto, T, Sakai, T, Enishi, T, Sato, N, Komatsu, K, Sairyo, K, et al. Changes of posture and muscle activities in the trunk and legs during walking in patients with lumbar spinal stenosis after decompression surgery. A preliminary report Gait Posture. Gait Posture. (2017) 51:149–52. doi: 10.1016/j.gaitpost.2016.10.006
42. Yokogawa, N, Toribatake, Y, Murakami, H, Hayashi, H, Yoneyama, T, Watanabe, T, et al. Differences in gait characteristics of patients with lumbar Spinal Canal stenosis (L4 radiculopathy) and those with osteoarthritis of the hip. PLoS One. (2015) 10:e0124745. doi: 10.1371/journal.pone.0124745
43. Garbelotti, SA Jr, Lucareli, PR, Ramalho, A Jr, de Godoy, W, Bernal, M, and D'Andréa Greve, JM. An investigation of the value of tridimensional kinematic analysis in functional diagnosis of lumbar spinal stenosis. Gait Posture. (2014) 40:150–3. doi: 10.1016/j.gaitpost.2014.03.013
44. Nagai, K, Aoyama, T, Yamada, M, Izeki, M, Fujibayashi, S, Takemoto, M, et al. Quantification of changes in gait characteristics associated with intermittent claudication in patients with lumbar spinal stenosis. J Spinal Disord Tech. (2014) 27:E136–42. doi: 10.1097/BSD.0b013e3182a2656b
45. Conrad, BP, Shokat, MS, Abbasi, AZ, Vincent, HK, Seay, A, and Kennedy, DJ. Associations of self-report measures with gait, range of motion and proprioception in patients with lumbar spinal stenosis. Gait Posture. (2013) 38:987–92. doi: 10.1016/j.gaitpost.2013.05.010
46. Suda, Y, Saitou, M, Shibasaki, K, Yamazaki, N, Chiba, K, and Toyama, Y. Gait analysis of patients with neurogenic intermittent claudication. Spine (Phila Pa 1976). (2002) 27:2509–13. doi: 10.1097/00007632-200211150-00016
47. Wasekar, DM, Thakare, PD, and Bijwe, R. Lechal footwear technology. Int Res J Eng Technol. (2016) 3:1457–61.
48. Larkin, R. Meet the new “talking shoes” by google. Available at: https://wwwesoftloadinfo/meet-the-new-talking-shoes-by-google (2016)
49. Zhang, W, Tomizuka, M, and Byl, N. A wireless human motion monitoring system based on joint angle sensors and smart shoes. ASME 2014 dynamic systems and control conference 2014; volume 3: industrial applications; modeling for oil and gas, control and validation, estimation, and control of automotive systems; multi-agent and networked systems; control system design; physical human-robot interaction; rehabilitation robotics; sensing and actuation for control; biomedical systems; time delay systems and stability; unmanned ground and surface robotics; vehicle motion controls; vibration analysis and isolation; vibration and control for energy harvesting; wind energy.
51. Matassa, A, and Manuguerra, V. Designing a smart plantar to support people in sport activities. Adjunct proceedings of the 2015 acm international joint conference on pervasive and ubiquitous computing and proceedings of the 2015 ACM International Symposium on Wearable Computers. Osaka, Japan (2015): 663–668
52. Sazonov, E, Hegde, N, Browning, RC, Melanson, EL, and Sazonova, NA. Posture and activity recognition and energy expenditure estimation in a wearable platform. IEEE J Biomed Health Inform. (2015) 19:1339–46. doi: 10.1109/jbhi.2015.2432454
53. Delgado-Gonzalo, R, Hubbard, J, Renevey, P, Lemkaddem, A, Vellinga, Q, Ashby, D, et al. Real-time gait analysis with accelerometer-based smart shoes. Annu Int Conf IEEE Eng Med Biol Soc. (2017) 2017:148-c. doi: 10.1109/embc.2017.8036783
54. Chen, LA, and Kao, CL. Parametric and nonparametric improvements in bland and Altman's assessment of agreement method. Stat Med. (2021) 40:2155–76. doi: 10.1002/sim.8895
55. Gustafsson, M, Schiøttz-Christensen, B, Wedderkopp, N, and Brønd, JC. Step count in patients with lumbar spinal stenosis: accuracy during walking and nonwalking activities. Spine (Phila Pa 1976). (2022) 47:1203–11. doi: 10.1097/brs.0000000000004385
56. Greenberg, JK, Javeed, S, Zhang, JK, Benedict, B, Frumkin, MR, Xu, Z, et al. Current and future applications of mobile health technology for evaluating spine surgery patients: a review. J Neurosurg Spine. (2023) 38:1–10. doi: 10.3171/2022.12.Spine221302
Keywords: gait analysis, smart shoes, lumbar spinal canal stenosis, digital biomarker, wearable sensor
Citation: Morimoto T, Hirata H, Kobayashi T, Tsukamoto M, Yoshihara T, Toda Y and Mawatari M (2023) Gait analysis using digital biomarkers including smart shoes in lumbar spinal canal stenosis: a scoping review. Front. Med. 10:1302136. doi: 10.3389/fmed.2023.1302136
Edited by:
Eron Grant Manusov, The University of Texas Rio Grande Valley, United StatesReviewed by:
Jimmy Gonzales, The University of Texas Rio Grande Valley, United StatesNaohiro Shibuya, The University of Texas Rio Grande Valley, United States
Copyright © 2023 Morimoto, Hirata, Kobayashi, Tsukamoto, Yoshihara, Toda and Mawatari. This is an open-access article distributed under the terms of the Creative Commons Attribution License (CC BY). The use, distribution or reproduction in other forums is permitted, provided the original author(s) and the copyright owner(s) are credited and that the original publication in this journal is cited, in accordance with accepted academic practice. No use, distribution or reproduction is permitted which does not comply with these terms.
*Correspondence: Tadatsugu Morimoto, bW9yaW1vdG8zQGNjLnNhZ2EtdS5hYy5qcA==