- 1Hospital Santa Rosália, Teófilo Otoni, Minas Gerais, Brazil
- 2Mucuri's Medical School and Telehealth Center, Universidade Federal dos Vales do Jequitinhonha e Mucuri (UFVJM), Teófilo Otoni, Minas Gerais, Brazil
- 3Universidade Federal de Minas Gerais and Institute for Health and Technology Assessment (IATS), Porto Alegre, Rio Grande do Sul, Brazil
- 4Department of Statistics, Universidade Federal de Minas Gerais, Belo Horizonte, Minas Gerais, Brazil
- 5Hospitais da Rede Mater Dei, Belo Horizonte, Minas Gerais, Brazil
- 6Hospital Risoleta Tolentino Neves, Belo Horizonte, Minas Gerais, Brazil
- 7Hospital Universitário de Santa Maria, Santa Maria, Rio Grande do Sul, Brazil
- 8Faculdade de Ciências Médicas de Minas Gerais, Belo Horizonte, Minas Gerais, Brazil
- 9Hospital Júlia Kubitschek, Belo Horizonte, Minas Gerais, Brazil
- 10Hospital João XXIII, Belo Horizonte, Minas Gerais, Brazil
- 11Hospital das Clínicas da Faculdade de Medicina de Botucatu, Av. Prof. Mário Rubens Guimarães Montenegro, UNESP, Botucatu, São Paulo, Brazil
- 12Universidade Federal de São João del-Rei, Divinópolis, Minas Gerais, Brazil
- 13Hospital Nossa Senhora da Conceição and Hospital Cristo Redentor, Porto Alegre, Rio Grande do Sul, Brazil
- 14Hospital Metropolitano Dr. Célio de Castro, Belo Horizonte, Minas Gerais, Brazil
- 15Hospital Santo Antônio, Curvelo, Minas Gerais, Brazil
- 16Hospital Santa Casa de Misericórdia de Belo Horizonte, Belo Horizonte, Minas Gerais, Brazil
- 17Hospital SOS Cárdio, Florianópolis, Santa Catarina, Brazil
- 18Universidade Federal de Santa Maria, Santa Maria, Rio Grande do Sul, Brazil
- 19Hospital Unimed BH, Belo Horizonte, Minas Gerais, Brazil
- 20Hospital Universitário Ciências Médicas, Belo Horizonte, Minas Gerais, Brazil
- 21Department of Internal Medicine, Medical School, Universidade Federal de Minas Gerais, Belo Horizonte, Minas Gerais, Brazil
- 22Telehealth Center, University Hospital, Universidade Federal de Minas Gerais, Belo Horizonte, Minas Gerais, Brazil
- 23Hospital João XXIII, Belo Horizonte, Minas Gerais, Brazil
- 24Hospital São Lucas PUCRS, Porto Alegre, Rio Grande do Sul, Brazil
- 25Pontifica Universidade Católica do Rio Grande do Sul, Porto Alegre, Rio Grande do Sul, Brazil
- 26Institute for Health Technology Assessment (IATS/CNPq), Porto Alegre, Rio Grande do Sul, Brazil
- 27Hospital Mãe de Deus, Porto Alegre, Rio Grande do Sul, Brazil
- 28Hospital Universitário de Canoas, Canoas, Rio Grande do Sul, Brazil
- 29Hospital Bruno Born, Lajeado, Rio Grande do Sul, Brazil
- 30Hospital Metropolitano Odilon Behrens, Belo Horizonte, Minas Gerais, Brazil
- 31Hospital Santa Cruz, Santa Cruz do Sul, Rio Grande do Sul, Brazil
- 32Fundação Hospitalar do Estado de Minas Gerais (FHEMIG), Cidade Administrativa de Minas Gerais, Belo Horizonte, Minas Gerais, Brazil
- 33Centro Universitário de Belo Horizonte (UNIBH), Belo Horizonte, Minas Gerais, Brazil
- 34Hospital Eduardo de Menezes, Belo Horizonte, Minas Gerais, Brazil
- 35Telehealth Center, University Hospital, Universidade Federal de Minas Gerais, Belo Horizonte, Minas Gerais, Brazil
- 36Cardiology Service, University Hospital, Universidade Federal de Minas Gerais, Belo Horizonte, Minas Gerais, Brazil
- 37Department of Internal Medicine, Medical School and University Hospital, Universidade Federal de Minas Gerais, Belo Horizonte, Minas Gerais, Brazil
- 38Institute for Health Technology Assessment (IATS), Porto Alegre, Rio Grande do Sul, Brazil
Background: Predicting the need for invasive mechanical ventilation (IMV) is important for the allocation of human and technological resources, improvement of surveillance, and use of effective therapeutic measures. This study aimed (i) to assess whether the ABC2-SPH score is able to predict the receipt of IMV in COVID-19 patients; (ii) to compare its performance with other existing scores; (iii) to perform score recalibration, and to assess whether recalibration improved prediction.
Methods: Retrospective observational cohort, which included adult laboratory-confirmed COVID-19 patients admitted in 32 hospitals, from 14 Brazilian cities. This study was conducted in two stages: (i) for the assessment of the ABC2-SPH score and comparison with other available scores, patients hospitalized from July 31, 2020, to March 31, 2022, were included; (ii) for ABC2-SPH score recalibration and also comparison with other existing scores, patients admitted from January 1, 2021, to March 31, 2022, were enrolled. For both steps, the area under the receiving operator characteristic score (AUROC) was calculated for all scores, while a calibration plot was assessed only for the ABC2-SPH score. Comparisons between ABC2-SPH and the other scores followed the Delong Test recommendations. Logistic recalibration methods were used to improve results and adapt to the studied sample.
Results: Overall, 9,350 patients were included in the study, the median age was 58.5 (IQR 47.0–69.0) years old, and 45.4% were women. Of those, 33.5% were admitted to the ICU, 25.2% received IMV, and 17.8% died. The ABC2-SPH score showed a significantly greater discriminatory capacity, than the CURB-65, STSS, and SUM scores, with potentialized results when we consider only patients younger than 80 years old (AUROC 0.714 [95% CI 0.698–0.731]). Thus, after the ABC2-SPH score recalibration, we observed improvements in calibration (slope = 1.135, intercept = 0.242) and overall performance (Brier score = 0.127).
Conclusion: The ABC2-SPHr risk score demonstrated a good performance to predict the need for mechanical ventilation in COVID-19 hospitalized patients under 80 years of age.
Highlights
• Among 9,3,150, 33.5% were admitted to the ICU, 25.2% received IMV, and 17.8% died.
• Patients who received IMV had higher median age and prevalence of hypertension and diabetes.
• ABC2-SPH score presented poor discrimination and calibration, with better discrimination among patients <80 years.
• In patients <80 years, the score had greater discrimination ability than CURB-65, SOFA, STSS and SUM scores.
• After the recalibration, ABC2-SPHr score obtained better calibration and overall performance.
Background
Since its inception, the COVID-19 pandemic has triggered an unprecedented crisis in health systems worldwide, with increased demand for intensive care unit (ICU) beds and mechanical ventilation (1). Although studies highlight the substantial impact of vaccination on the trajectory of the pandemic, with up to 90% protection against COVID-19-associated invasive mechanical ventilation (IMV) and death among adults (2, 3). It is estimated that the mortality rate associated with IMV continues overcoming 30% (4). A recent systematic review and meta-analysis found a 43% (95% CI 0.29–0.58) pooled IMV mortality rate (1). Knowledge of COVID-19 intensive care unit (ICU) and associated IMV patient characteristics, and outcomes as well as analyzing their regional variability is critically important for patient management and allocation of resources (1). Therefore, it may be helpful to predict which patients are more likely to progress to IMV, to subsidize more assertive health decisions.
Although different prognostic scores have been proposed to predict IMV among COVID-19 patients, the majority of them present methodological limitations, restricting their clinical applicability (for more details, see Supplementary Table S1). Furthermore, most scores were developed in high-income countries, without external validation in low-and middle-income countries.
In this context, the ABC2-SPH risk score for predicting in-hospital mortality was rigorously developed and validated in Brazilian patients with high discrimination (5). This score is the only mortality risk score for COVID-19 tested and validated in the Brazilian population (5). It predicts in-hospital mortality in patients with COVID-19 using easily accessible variables on admission: Age, BUN (blood urea nitrogen), Comorbidities, C-reactive protein, SpO2/FiO2 ratio, Platelet count, and Heart rate. The score ranges from 0 to 20, with the following risk groups: low (0–1), intermediate (2–4), high (5–8), and very high (≥9). It is freely available as an online risk calculator.1 It was developed in a cohort of 3,978 patients admitted to 36 hospitals in five Brazilian states. The validation was carried out on 1,054 patients admitted to the same institutions (temporal validation) and also in a cohort with 474 Spanish patients (external validation). It has shown good overall performance for temporal (AUROC = 0.859 [95% CI 0.833 to 0.885], Brier = 0.108 and calibration [slope = 1.138, intercept = 0.114, value of p = 0.184]) and external validation (AUROC = 0.894 [95% CI 0.870 to 0.919] Brier = 0.093) (5). However, evidence of its accuracy for IMV prediction is still lacking. Therefore, our aims were: (i) to assess whether the ABC2-SPH score is able to predict IMV in COVID-19 patients; (ii) to compare its performance with other existing scores; (iii) to perform score recalibration, and to assess whether recalibration improved prediction.
Methods
Study design
This study is a substudy of the retrospective multicenter cohort Brazilian COVID-19 Registry, conducted in 32 Brazilian hospitals, in 14 cities from five Brazilian states (Minas Gerais, Pernambuco, Rio Grande do Sul, Santa Catarina and São Paulo), described in detail elsewhere (6). The study was approved by the National Commission for Research Ethics (CAAE 30350820.5.1001.0008) and the individual informed consent was waived due to the pandemic circumstances and analysis of unidentified data.
Study population
The cohort study included consecutive adult patients (≥18 years-old) with laboratory-confirmed COVID-19, according to World Health Organization guidance (7), admitted in one of the participating hospitals. For the assessment of the ABC2-SPH score and the comparison with other scores, patients admitted from July 31, 2020, to March 31, 2022, were included. For ABC2-SPH score recalibration and also comparison with other scores, patients admitted from January 1, 2021, to March 31, 2022, were enrolled. However, for recalibration, only patients younger than 80 years were included, since mortality is particularly high for mechanical ventilation at an older age. This supported recommendations for conservative treatment for elderly and/or frail patients (8–10).
Patients with at least one of the following conditions were excluded: (i) pregnant women; (ii) “do not resuscitate” order; (iii) patients who manifested COVID-19 while admitted for other conditions; (iv) those transferred to other hospitals who had no defined outcome (discharged or death); (v) patients who were already on IMV at hospital presentation; and (vi) exclusively for score recalibration, patients ≥80 years old (Figure 1).
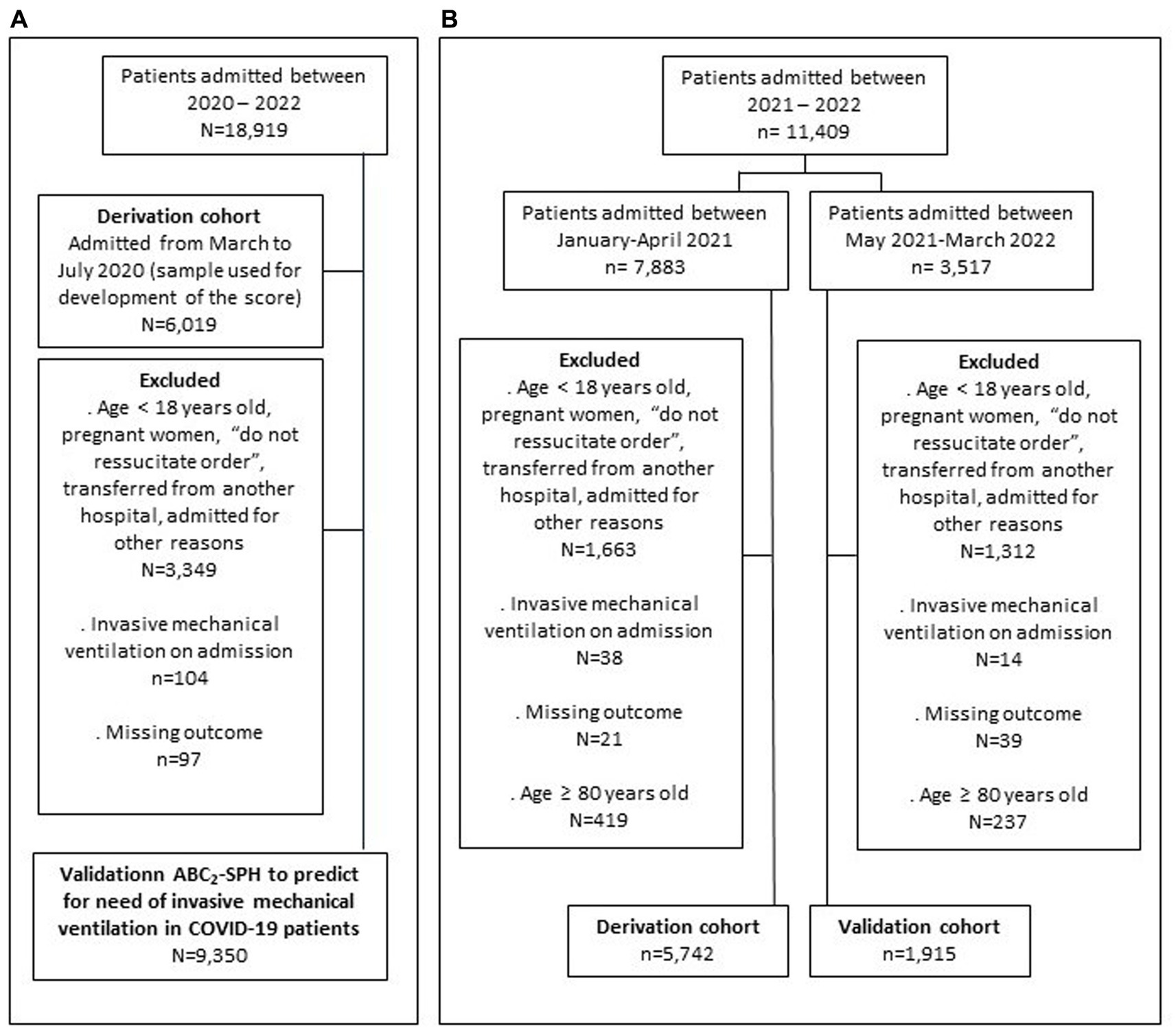
Figure 1. Flowchart of the study conducted in two stages: (A) The first stage, aimed to assess the ABC2-SPH risk score to predict invasive mechanical ventilation in COVID-19 patients and compare with other available scores; and (B) the second stage, aimed to perform ABC2-SPH score recalibration, as well as to compare with other scores.
Data collection
Medical records were reviewed to collect data concerning the patients’ characteristics, including age, sex, pre-existing comorbid medical conditions and medications taken at home; COVID-19-associated symptoms at hospital presentation; clinical assessment upon hospital presentation; laboratory results; inpatient medication, treatment, and outcomes. The data collection instrument was designed with reference to COVID-19 guidelines from the World Health Organization and the Brazilian Ministry of Health, as previously described (6).
A detailed guidance manual for data collection was developed, containing the definitions used in the study (Supplementary material). It was provided to all participating centers, and online training was mandatory before local research personnel were allowed to start collecting study data.
Data was collected by trained researchers from the medical records, using Research Electronic Data Capture (REDCap®) (version 7.3.1) (11, 12), hosted at the Telehealth Center of the University Hospital, of the Universidade Federal de Minas Gerais (13). To ensure reliability and monitor data, a code was developed in the R software that periodically verified possible data entry errors. When detected, the analysts notified the participating center for correction.
Outcomes
The primary outcome was IMV during hospitalization.
Sample size
Model validation followed guidance from the Transparent Reporting of a Multivariable Prediction Model for Individual Prediction or Diagnosis (TRIPOD) checklist (14, 15) and the Prediction model Risk Of Bias Assessment Tool (PROBAST) (16). TRIPOD checklist ideally recommends at least 100 events (as deaths) and 100 non-events as samples for score validation. In the present analysis, the sample size was not calculated, since all patients eligible by the inclusion criteria were enrolled.
Statistical analysis
Continuous variables were described by medians and interquartile ranges (IQR) and categorical variables were represented by absolute and relative frequencies. Data were analyzed with R software (version 4.0.2), using mice (function mice), pROC (functions roc, ci.auc, roc.test), glmnet (function cv.glmnet), tidyverse (dplyr functions), gtsummary (function tbl_summary) and ggplot2 packages. value of ps <0.05 were considered statistically significant.
The statistical analysis was divided into two stages: (i) evaluation of the ABC2-SPH risk score for predicting IMV in COVID-19 patients and comparison with other available scores; and (ii) recalibration of the ABC2-SPH score, as well as comparison with other scores.
ABC2-SPH assessment and comparison with other risk scores
Discrimination of the ABC2-SPH score was compared to other existing scores, including CALL (17), COVID-IRS (18), CURB-65 (19), PREDI-CO (20), SOFA (21), STSS (22), SUM (23) and 4C Mortality Score (24). The scores were chosen based on the two conditions: (i) parameters available within the Registry’s database, and (ii) accessible methods for calculation.
The main characteristics of the scores are listed in Supplementary Table S1. The comparison of ABC2-SPH (5) with other scores (17–24) was performed using the number of complete cases for each score (non-imputed database) through a procedure for unpaired receiving operator characteristic (ROC) curves that is an extension of Delong et al. recommendations (25). This procedure was implemented in the pROC package, function “roc.test.” Due to the multiple comparisons, alpha was corrected using the Bonferroni method.
Score recalibration
The score was recalibrated, in an attempt to improve the prediction risk of IMV among patients with COVID-19. The sample of patients included COVID-19 patients under 80 years of age, divided into derivation (from January 1 to April 30, 2021) and validation cohorts (from May 1, 2021, to March 31, 2022), resulting in approximately 75 and 25% of the sample, respectively. This division guarantees the minimum of 100 events in the validation cohort, as recommended by the TRIPOD checklist (14, 15).
The recalibration methods consisted of fitting a logistic regression model [for more details, see Steyerberg et al. (26)] in the derivation sample and the evaluation of the method was done in the validation sample.
Missing data
To handle missing values, multiple imputation by chained equations (MICE) was used, considering missing at random assumption. The imputation technique included all variables with up to 30% missing values. The prediction of missing values was performed using all variables included in the analysis. Invasive mechanical ventilation was not imputed, and was not used as a predictor in the MICE model in the validation dataset. The predictive mean matching (PMM) method was used for continuous predictors and polytomous regression for categorical variables. Ten imputed datasets were obtained with 10 iterations, and their results were combined following Rubin’s rules (27).
Performance measures
Model’s discrimination was assessed by the area under the ROC curve (AUROC), with 95% confidence interval (95% CI) calculated by bootstrap resampling, through 2,000 samples. A value of 0.5 indicates no predictive ability, 0.60 to 0.69 is considered poor, 0.70 to 0.89 good, and 0.90 to 1.0 excellent (28).
The accuracy of the predictive model was assessed using the Brier score, a measure that quantifies how close predictions are to the truth (29). The score ranges between 0 and 1, in which smaller values indicate superior model performance. Results were stratified by age groups (<60, 60–69, 70–79 and ≥ 80 years-old), sex and presence or absence of key comorbidities before recalibration, to assess score performance in different subgroups.
Calibration was assessed graphically by plotting the predicted IMV probabilities against the observed IMV, testing intercept equals zero and slope equals one, simultaneously.
Results
ABC2-SPH assessment and comparison with other risk scores
Overall, 9,350 patients were included in the study, the median age was 58.5 (IQR 47.0–69.0) years old, and 45.4% were women. Of those, 33.5% were admitted to the ICU, 25.2% received IMV, and 17.8% died. Patients who received IMV were older; had a higher frequency of hypertension, diabetes, obesity, chronic kidney disease, rheumatologic disease and previous transplant; a higher number of comorbidities; and a higher frequency of ICU, dialysis, thromboembolism and mortality, when compared to those who did not receive IMV (Table 1). They also had a higher frequency dyspnea, cough, fever, nausea, and arthralgia; clinical findings such as fever, tachycardia and arterial hypotension (Supplementary Table S4) and laboratory findings such as neutrophilia, lymphopenia, thrombocytopenia and increased lactate, D-dimer and C-reactive protein, when compared to those who did not receive IMV (Supplementary Tables S4, S5).
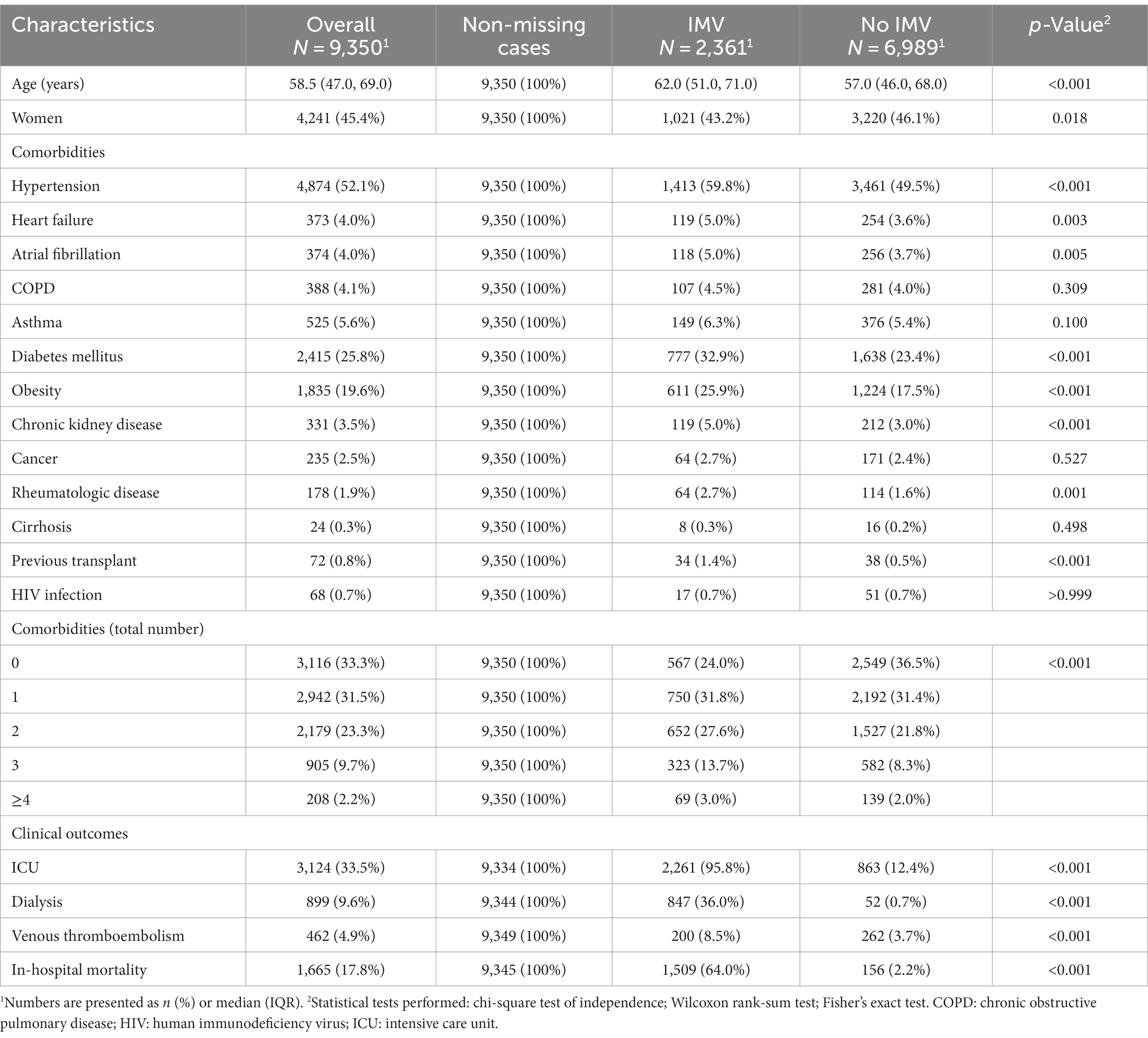
Table 1. Demographic data, clinical characteristics, and outcomes of a cohort of Brazilian patients admitted to hospital with COVID-19, from July 31, 2020, to March 31, 2022.
The AUROC for the ABC2-SPH 0.677 (0.661–0.694), and the Brier score 0.196. Subject-specific risks were calculated, and patients were classified according to ABC2-SPH risk groups (Table 2). Score’s performance was worse among older patients, especially the octogenarians, and patients with chronic pulmonary obstructive disease (Supplementary Table S1).
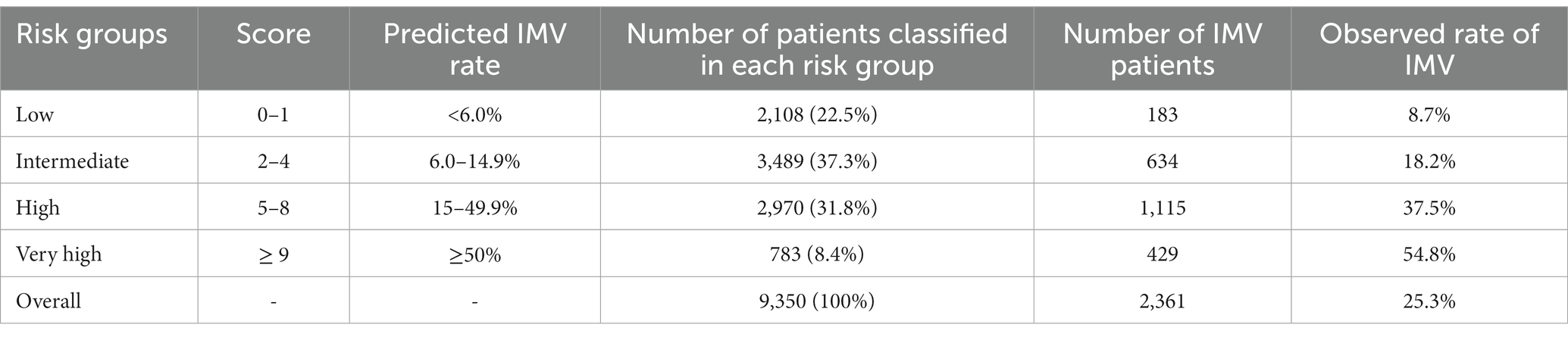
Table 2. Predicted and observed invasive mechanical ventilation (IMV) rates observed with ABC2-SPH score.
For the comparison with other scores, the main characteristics of each score are shown in Supplementary Table S2. When compared with other scores in a complete case analysis, the ABC2-SPH score achieved a significantly higher discriminatory capacity than CURB-65, STSS, and SUM scores (Table 3; Figure 2A). When assessing specifically the sample < 80 years, ABC2-SPH score still achieved a significantly higher discriminatory capacity than CURB-65, STSS, SOFA and SUM scores (Supplementary Table S3).
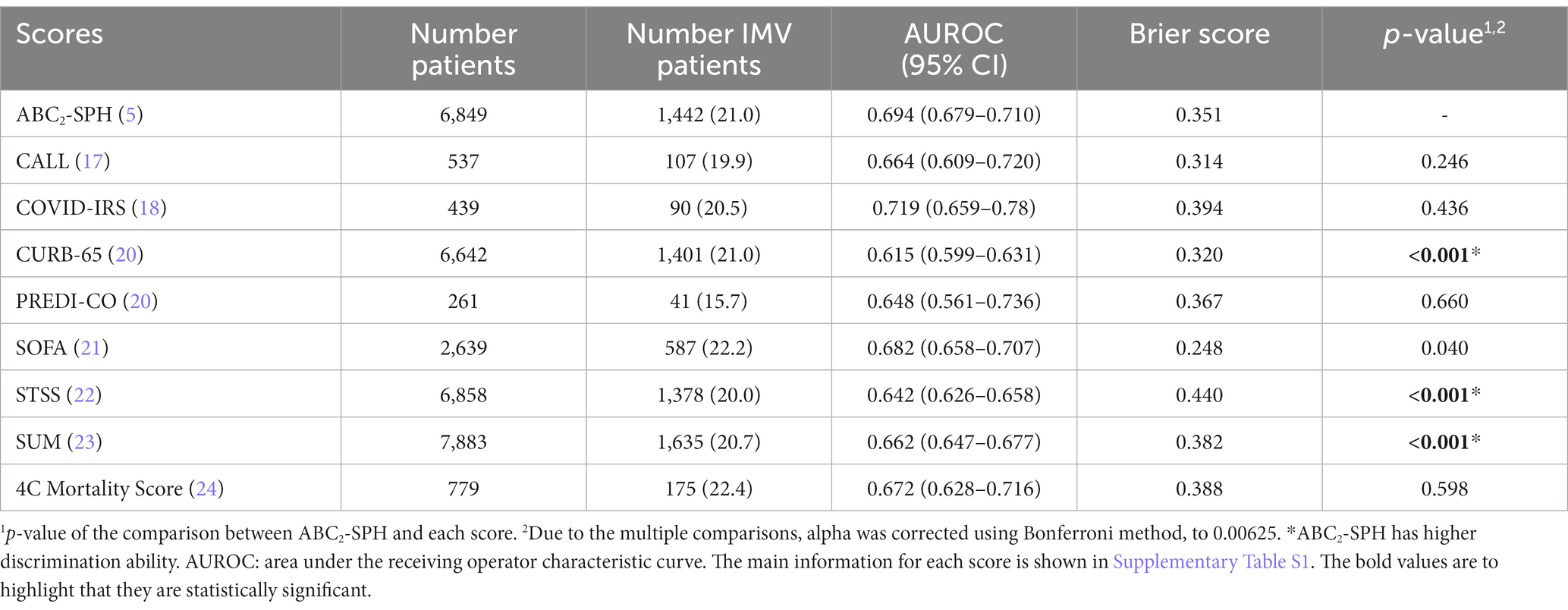
Table 3. Discrimination ability for each score to predict invasive mechanical ventilation applied in the database of COVID-19 patients (complete case analysis) and comparison of the ABC2-SPH and other existing scores.
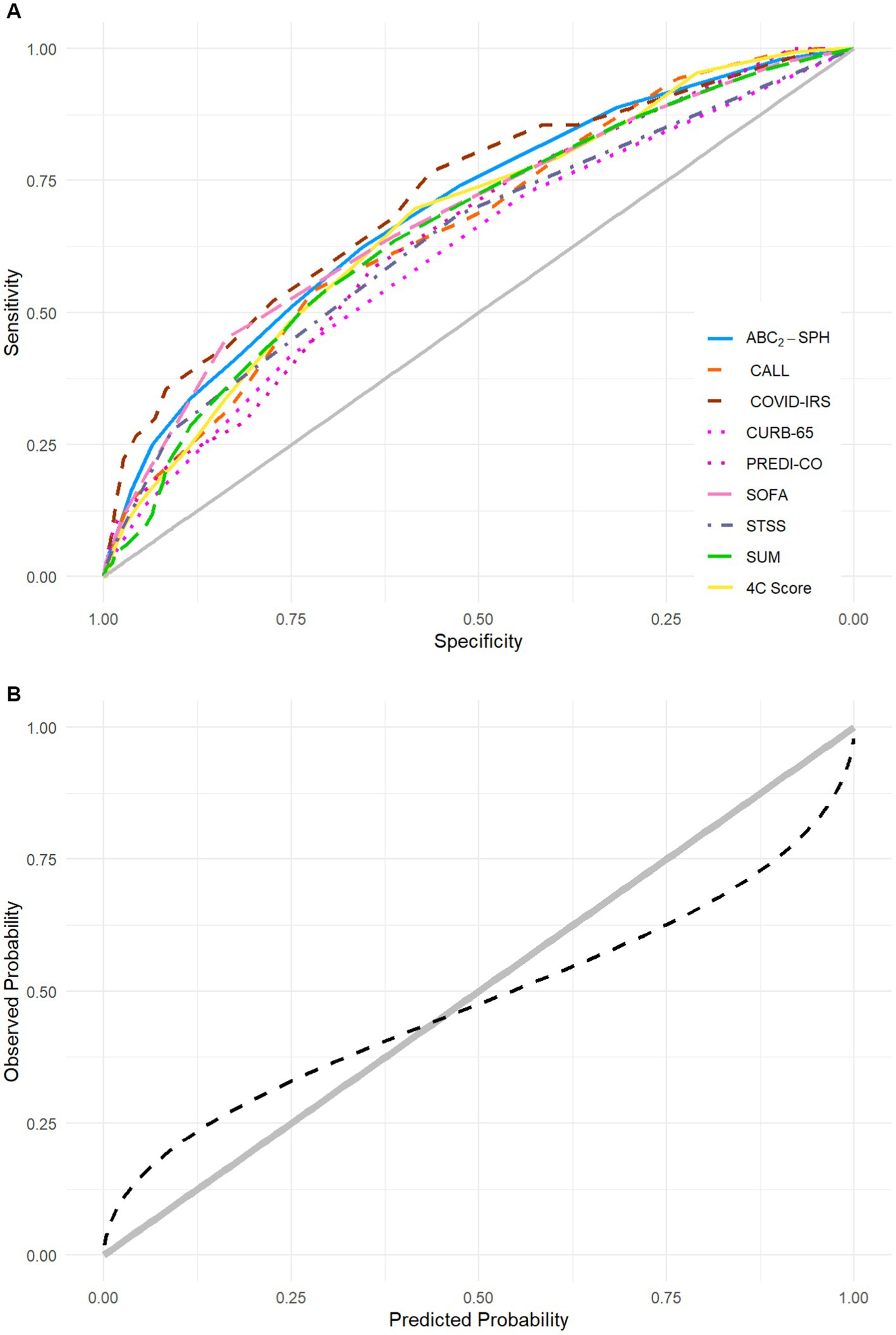
Figure 2. (A) Area under the receiving operator characteristic curves (AUROC) of ABC2-SPH and other scores in this cohort. The main information for each score is shown in Supplementary Table S1. (B) Calibration of ABC2-SPH score.
The calibration curve indicates that the ABC2-SPH underestimated IMV at lower ranges of the score and overestimated it at the higher ones, as observed in Figure 2B (slope = 0.557, intercept = −0.097, value of p < 0.001).
ABC2-SPH score recalibration
When assessing specifically the sample of patients used for score recalibration (<80 years-old admitted to hospital with COVID-19, from January 1, 2021, to March 31, 2022), patients from the validation cohort had a slightly lower age, frequency of hypertension and inotropic requirement; a slightly higher frequency of atrial fibrillation and COPD; a higher frequency of smoking and a lower frequency of outcomes than the derivation cohort (Table 4; Supplementary Table S6). As for laboratory findings, there were no clinically relevant differences (Supplementary Table S7).
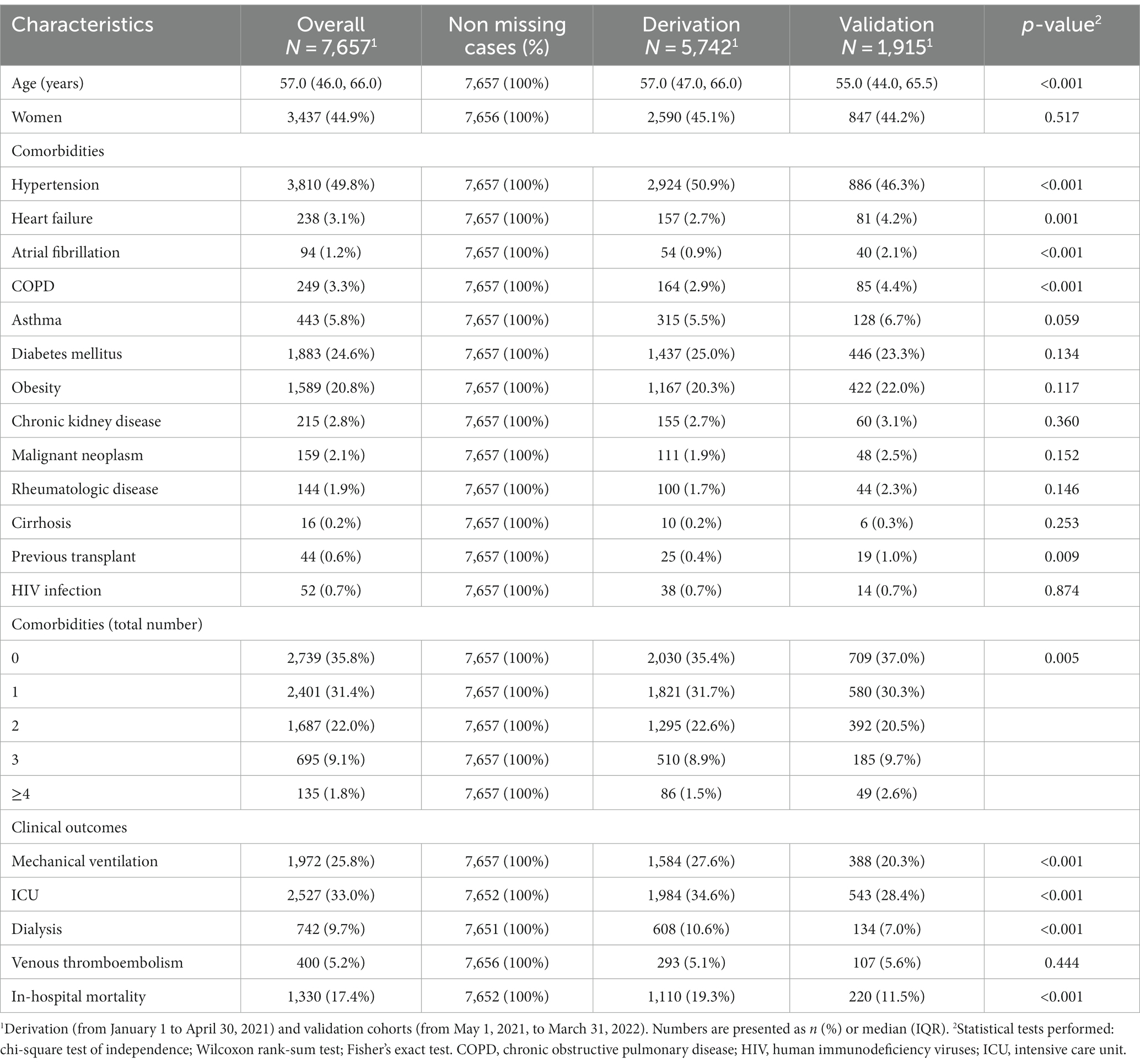
Table 4. Demographic data, clinical characteristics and outcomes of derivation and validation cohorts of patients <80 years-old admitted to hospital with COVID-19, from January 1, 2021, to March 31, 2022, used for score recalibration.
When assessing score performance in this sample before calibration (Table 5), the AUROC for ABC2-SPH was superior to the assessed scores. The recalibrated ABC2-SPH score, named as ABC2-SPHr score, obtained good overall performance (Brier score = 0.132) and calibration (slope = 1.048, intercept = 0.378, value of p < 0.001) (Figure 3) in the validation subsample.
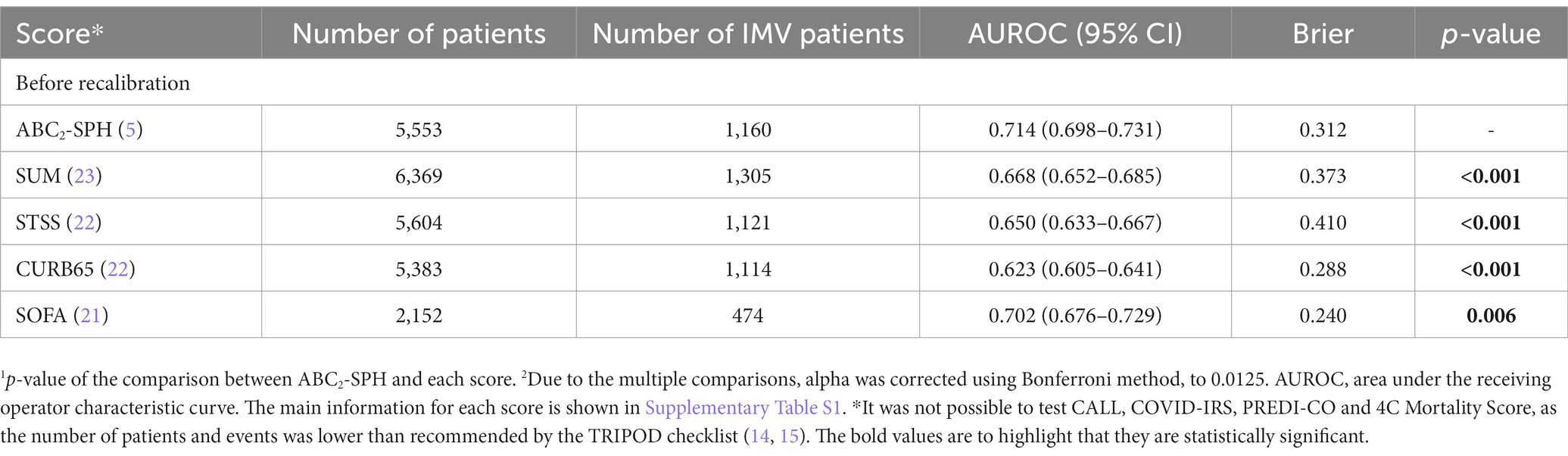
Table 5. Discrimination ability for each score to predict invasive mechanical ventilation applied in the database of COVID-19 patients <80 years-old admitted to hospital with COVID-19, from January 1, 2021, to March 31, 2022 (complete case analysis).
Discussion
The original ABC2-SPH score presented poor discrimination to predict IMV in COVID-19 patients, with an AUROC lower than 0.70, and poor calibration (slope = 0.550, intercept = −0.031, value of p = <0.00). When compared with other scores, it showed a significantly greater discriminatory capacity than the CURB-65 (19), STSS (22), and SUM (23) scores. When assessing data from patients <80 years-old hospitalized in 2021/2022, discriminatory capacity was higher, with an AUROC 0.714 (0.698–0.731). It was greater than those scores and also greater than the SOFA score (21). After the ABC2-SPH score recalibration, we observed improvements in overall performance (Brier score = 0.132) and calibration (slope = 1.048, intercept = 0.378, value of p < 0.001). So, the ABC2-SPHr score may be used to discriminate the risk of IMV in COVID-19 patients <80 years-old.
Since December 2019, over 6.9 million deaths related to COVID-19 have been reported worldwide (30). The unprecedented spread of the virus and the high proportion of severely ill patients created widespread disarray. In this context, the medical community encountered saturated hospitals and strained resources, especially related to IMV, as well as the need to provide accurate information on morbidity and prognosis of the disease to patients and families. Based on this, important ethical questions about intensive care rationing in ICUs had been asked (31). Therefore, it may be helpful to predict which patients are more likely to progress to IMV, in order to subsidize more assertive health decisions for better allocation of human and technological resources, improvement of surveillance, and use of effective therapeutic measures.
Despite severity scores being commonly used in hospital settings [such as SOFA (21), STSS (22), and others], the pandemic required new tools specific for COVID-19, in addition to validation of previous clinical scores for rapid, easy, and precise triage. Despite an increasing number of studies relating to various aspects of severe COVID-19 and its ICU management, only the COVID-IRS (15) and SUM (20) scores were specifically developed for the prediction of IMV in COVID-19 patients. In the original studies of scores developed specifically for COVID-19 patients, the majority of them presented good discrimination for COVID-19 (18, 20, 23, 24), with analyses prior to vaccination and without validation for the Brazilian population. Thus, it becomes useful to validate and recalibrate the ABC2-SPH, the only score developed and validated in the Brazilian population, with high accuracy in predicting hospital mortality.
In the present study, all available scores, such as CALL (17), COVID-IRS (18), CURB-65 (19), PREDI-CO (20), SOFA (21), STSS (22), SUM (23), and 4C Mortality (24), in addition to ABC2-SPH itself, performed worse in our Brazilian cohort than in their original cohorts. The differences in predictive ability may be at least partly explained by differences between the population included in the study and the original derivation cohorts (i.e., geographically distant, ethnically different, with the prevalence of distinct comorbidities, in different health systems and cultures), as already observed by other authors (26, 32), and also for the fact that some of these scores assessed composite outcomes, to try overcome the limitation of having a small sample size. As mentioned earlier, the TRIPOD Guidelines (14, 15) and PROBAST checklist (16) guide at least 100 events for score development. The CALL score derivation score (17), for example, had only 40 events, even using a composite outcome, whereas the COVID-IRS score (18) had 72 events only, and defined the cut-offs based on the data, which may have led to models overfitting (29), limiting their respective generalizations in other cohorts.
Patients who received IMV were predominantly older, women, and had a higher prevalence of underlying comorbidities, as previously described (33–40), of which hypertension, DM, obesity, and chronic kidney disease were the most prevalent. Regarding clinical outcomes, our invasively ventilated patients presented especially higher requirement dialysis, venous thromboembolism, and in-hospital mortality. Overall, in-hospital mortality was 64.0%, similar to those observed for invasively ventilated patients in studies from Argentina (57.7%) (41), Mexico (73.7%) (42), and China (49%) (43).
Considering the finitude of human and logistical resources, during the worst waves the ICUs were completely saturated, requiring the classification of the patients when the probability of survival under critical care treatment, in order to prioritize critical care initiation and continuation for patients who had the highest probability of benefiting from treatment, becoming an ethical necessity to reduce deaths (31). In this context, the ABC2-SPH score discrimination ability was worse in elderly patients, especially octogenarians. When considering only patients aged less than 80 years, and as expected, we observed a better AUROC 0.714 (0.698–0.731).
Medical predictive analytics have increased in popularity in recent years to help clinical decision making in various situations and clinical conditions. However, medical manuscripts usually focus the assessment in the AUROC only (also known as C-statistic), and it is often underreported that estimated risks may be unreliable even when the algorithms have good discrimination, especially if calibration is not adequate (44). A recent systematic review mentioned the hundreds of prediction models for COVID-19 as a typical example, most of which are deemed useless due to inappropriate derivation and assessment, with calibration being ignored in the great majority of them (45). This is of utmost importance, as poorly calibrated algorithms may be misleading and potentially harmful for clinical decision-making (44). When assessing the original ABC2-SPH score, there was a systematic miscalibration, with observed rates much higher than the predicted probabilities in low points (i.e., the score underestimated IMV), and observed rates significantly much lower than the predicted probabilities in high points (i.e., the score overestimated IMV). To improve prediction, we performed the recalibration of the ABC2-SPH score, correcting the intercept and the slope of the model to adapt it to patients at risk of IMV (32), with substantial improvement in overall performance and calibration. Thus, the ABC2-SPHr score can be used as a tool to stratify the risk of IMV in Brazilian COVID-19 patients <80 years-old into low, intermediate, high, and very high. Nevertheless, it is important to highlight that prediction models are population-specific and may produce different results in different populations (14). Therefore it is necessary to perform external validation of the ABC2-SPHr score for use in other populations.
Strengths and limitations of the study
Our study contributes to the literature because it is a multicenter study, with a large sample of patients from 32 Brazilian hospitals (including public, private, and philanthropic), from different regions and degrees of complexity, which validated and recalibrated the ABC2-SPH score for prediction of IMV in COVID-19 patients under the age of 80. Additionally, we included comparisons with existing risk stratification scores, ensuring superior performance to the CURB-65 (19), SOFA (21), STSS (22), and SUM (23) scores.
Even with these multiple strengths, The present study presents limitations that should Be addressed. Despite The fact that All hospitals referred there Was adequate supply of IMV during The study period, We cannot assure that All patients Who required IMV In fact received IMV. Therefore, The outcome for this analysis Was receipt of IMV, Not IMV requirement. That Is also Why We opted To recalibrate The score excluding The sample of octogenarians, As frequently doctors have conservative treatment for elderly and/or frail patients, which includes avoiding intubation, and this could Be observed By a worse AUROC curve In this stratum. Additionally, The scores were calculated based On data from a retrospective, observational, and non-randomized study, with data collected from medical records. Therefore, some variables were Not found uniformly, generating missing data. However, In order To reduce this impact, Our data were collected By researchers with extensive training and accompanied closely By a professional with important experience In research. Furthermore, information that depends On a more accurate clinical history, such As The description of comorbidities and details of symptoms, may Not have been obtained.
Next steps
Like other viruses, SARS-CoV-2 evolves over time. The majority of mutations in the SARS-CoV-2 genome have no impact on viral function, but certain variants have garnered widespread attention because of their rapid emergence within populations and evidence for transmission or clinical implications. These are considered variants of concern. The World Health Organization (WHO) has also designated labels for notable variants based on the Greek alphabet: Alpha, Beta Gamma Delta and Omicron (46). The omicron variant and its sublineage have been increasing in prevalence worldwide (47). In August 2023, the World Health Organization classified the EG.5 coronavirus strain as a “variant of interest,” although it did not seem to add public health risks relative to the other currently circulating Omicron descendent lineages (48). So, the current global epidemiology of SARS-CoV-2 is characterized by the continued spread of the Omicon variant. These findings underscore the importance of vaccination to prevent both moderate and severe COVID-19 and to reduce the circulating variant (49). Currently, in the world, around 70% of persons are vaccinated with at least one dose, of a total of 13.3 billion doses administered globally, but there is still great vaccine inequality between countries (30, 50). Therefore, the future severity of the pandemic is not yet known.
As COVID-19 is a dynamic disease, further assessments in the model are required. The outbreak of COVID-19 was accompanied by an unprecedented explosion of scientific evidence, and a living review has found almost 600 prognostic models to predict diverse outcomes in patients with confirmed COVID-19 (51). In the aforementioned systematic review on the methodology of prediction models, Binuya et al. (45) have discussed that the incessant de novo derivation of models instead of refinement of an existing one is a widely recognized issue, and a huge waste of information from previous modeling studies (and we could infer, also a waste of time and money). If a reasonable prediction model is available and produces accurate estimates, the consensus is to build upon such a model and check whether some adjustments (“model updating”) may improve its fit or performance in new data, for example, with recalibration or incorporating a novel marker into the model (45). Thus, further studies should take this into account.
Conclusion
ABC2-SPH risk score demonstrated a poor to fair performance to predict the need for mechanical ventilation in COVID-19 hospitalized patients. However, when compared with other scores, it showed a significantly greater discriminatory capacity, than the CURB-65, STSS, and SUM. This result was potentialized after their recalibration, with a prognostic score that more accurately estimates the probability of IMV in patients aged <80 years old, besides the better discrimination ability than the CURB-65, SOFA, STSS, and SUM scores. Thus ABC2-SPHr risk score is a rapid and easy assessment tool to assist clinicians in decision-making when initiating advanced ventilatory support, and therefore to ensure early life-saving interventions.
Data availability statement
The raw data supporting the conclusions of this article will be made available by the authors, upon reasonable request.
Ethics statement
The study protocol was approved by the Brazilian Research Ethics Commission (CONEP) and is registered under the Certificate of Presentation of Ethical Appreciation (CAAE) number 30350820.5.1001.0008. The studies were conducted in accordance with the local legislation and institutional requirements. The ethics committee/institutional review board waived the requirement of written informed consent for participation from the participants or the participants’ legal guardians/next of kin because Individual informed consent was waived due to the severity of the situation and the use of deidentified data, based on medical chart review only.
Author contributions
CC: Writing – original draft, Writing – review & editing, Visualization, Conceptualization, Investigation, Methodology, Project administration, Supervision. MP: Formal analysis, Methodology, Validation, Writing – review & editing. LR: Formal analysis, Methodology, Validation, Writing – review & editing. AG: Investigation, Writing – review & editing. AJ: Investigation, Writing – review & editing. AF: Investigation, Writing – review & editing. BG: Investigation, Writing – review & editing. BP: Investigation, Writing – review & editing. DP: Investigation, Writing – review & editing. DR: Investigation, Writing – review & editing. FA: Investigation, Writing – review & editing. FV: Investigation, Writing – review & editing. FB: Investigation, Writing – review & editing. GeG: Investigation, Writing – review & editing. GV: Investigation, Writing – review & editing. GiG: Investigation, Writing – review & editing. GN: Investigation, Writing – review & editing. HV: Investigation, Writing – review & editing. IV: Investigation, Writing – review & editing. JA: Investigation. JC: Investigation, Writing – review & editing. JM: Investigation, Writing – review & editing. KR: Investigation, Writing – review & editing. LZ: Investigation, Writing – review & editing. LM: Investigation, Writing – review & editing. LC: Investigation, Writing – review & editing. MaS: Investigation, Writing – review & editing. MCa: Investigation, Writing – review & editing. MB: Investigation, Writing – review & editing. MCu: Investigation, Writing – review & editing. MF: Investigation, Writing – review & editing. RL: Investigation, Writing – review & editing. RM: Investigation, Writing – review & editing. MM: Conceptualization, Data curation, Formal analysis, Funding acquisition, Investigation, Methodology, Project administration, Resources, Supervision, Validation, Visualization, Writing – original draft, Writing – review & editing. PA: Investigation, Writing – review & editing. PD-P: Formal analysis, Methodology, Validation, Writing – review & editing. CC: Investigation, Writing – review & editing. NO: Investigation, Writing – review & editing. AR: Formal analysis, Methodology, Validation, Writing – review & editing.
Funding
The author(s) declare financial support was received for the research, authorship, and/or publication of this article. This study was supported in part by Minas Gerais State Agency for Research and Development (Fundação de Amparo à Pesquisa do Estado de Minas Gerais-FAPEMIG) [grant number APQ-01154-21], National Institute of Science and Technology for Health Technology Assessment (Instituto de Avaliação de Tecnologias em Saúde-IATS)/National Council for Scientific and Technological Development (Conselho Nacional de Desenvolvimento Científico e Tecnológico-CNPq) [grant numbers 465518/2014-1 and 147122/2021-0], and CAPES Foundation (Coordenação de Aperfeiçoamento de Pessoal de Nível Superior) [grant number 88887.507149/2020-00]. AR and MM were supported in part by the Brazilian research agency CNPq (grant numbers 310790/2021-2 and 310561/2021-3, respectively).
Acknowledgments
We would like to thank the health professionals, interns and coordinators of the participating hospitals for supporting this project.
Conflict of interest
The authors declare that the research was conducted in the absence of any commercial or financial relationships that could be construed as a potential conflict of interest.
Publisher’s note
All claims expressed in this article are solely those of the authors and do not necessarily represent those of their affiliated organizations, or those of the publisher, the editors and the reviewers. Any product that may be evaluated in this article, or claim that may be made by its manufacturer, is not guaranteed or endorsed by the publisher.
Supplementary material
The Supplementary material for this article can be found online at: https://www.frontiersin.org/articles/10.3389/fmed.2023.1259055/full#supplementary-material
Footnotes
References
1. Chang, R, Elhusseiny, KM, Yeh, YC, and Sun, WZ. COVID-19 ICU and mechanical ventilation patient characteristics and outcomes-a systematic review and meta-analysis. PLoS One. (2021) 16:e0246318. doi: 10.1371/journal.pone.0246318
2. DeCuir, J, Surie, D, Zhu, Y, Gaglani, M, Ginde, AA, Douin, DJ, et al. Effectiveness of monovalent mRNA COVID-19 vaccination in preventing COVID-19–associated invasive mechanical ventilation and death among immunocompetent adults during the omicron variant period — IVY network, 19 U.S. states, February 1, 2022–January 31, 2023. MMWR Morb Mortal Wkly Rep. (2023) 72:463–8. doi: 10.15585/mmwr.mm7217a3
3. Tenforde, MW, Self, WH, Gaglani, M, Ginde, AA, Douin, DJ, Talbot, HK, et al. Effectiveness of mRNA vaccination in preventing COVID-19-associated invasive mechanical ventilation and death-United States, March 2021–January 2022. MMWR Morb Mortal Wkly Rep. (2022) 71:459–65. doi: 10.15585/mmwr.mm7112e1
4. Leazer, S, Collen, J, Alcover, K, Tompkins, E, Ambardar, S, Allard, RJ, et al. Outcomes associated with intensive care and organ support among patients with COVID-19: a systematic review and Meta-analysis. Mil Med. (2023) 188:541–6. doi: 10.1093/milmed/usac143
5. Marcolino, MS, Pires, MC, Ramos, LEF, Silva, RT, Oliveira, LM, Carvalho, RLR, et al. ABC2-SPH risk score for in-hospital mortality in COVID-19 patients: development, external validation and comparison with other available scores. Int J Infect Dis. (2021) 110:281–308. doi: 10.1016/j.ijid.2021.07.049
6. Marcolino, MS, Ziegelmann, PK, Souza-Silva, MVR, Nascimento, IJB, Oliveira, LM, Monteiro, LS, et al. Clinical characteristics and outcomes of patients hospitalized with COVID-19 in Brazil: results from the Brazilian COVID-19 registry. Int J Infect Dis. (2021) 107:300–10. doi: 10.1016/j.ijid.2021.01.019
7. World Health Organization. Testes de diagnóstico para SARS-CoV-2. Geneva: World Health Organization (2020).
8. Armstrong, RA, Kane, AD, and Cook, TM. Outcomes from intensive care in patients with COVID-19: a systematic review and meta-analysis of observational studies. Anaesthesia. (2020) 75:1340–9. doi: 10.1111/anae.15201
9. Karagiannidis, C, Mostert, C, Hentschker, C, Voshaar, T, Malzahn, J, Schillinger, G, et al. Case characteristics, resource use, and outcomes of 10 021 patients with COVID-19 admitted to 920 German hospitals: an observational study. Lancet Respir Med. (2020) 8:853–62. doi: 10.1016/S2213-2600(20)30316-7
10. Richardson, S, Hirsch, JS, Narasimhan, M, Crawford, JM, McGinn, T, Davidson, KW, et al. Presenting characteristics, comorbidities, and outcomes among 5700 patients hospitalized with COVID-19 in the new York City area [published correction appears in JAMA. 2020 May 26; 323 (20): 2098]. JAMA. (2020) 323:2052–9. doi: 10.1001/jama.2020.6775
11. Harris, PA, Taylor, R, Thielke, R, Payne, J, Gonzalez, N, and Conde, JG. Research electronic data capture (REDCap)—a metadata-driven methodology and workflow process for providing translational research informatics support. J Biomed Inform. (2009) 42:377–81. doi: 10.1016/j.jbi.2008.08.010
12. Harris, PA, Taylor, R, Minor, BL, Elliott, V, Fernandez, M, O'Neal, L, et al. The REDCap consortium: building an international community of software platform partners. J Biomed Inform. (2019) 95:95. doi: 10.1016/j.jbi.2019.103208
13. Soriano Marcolino, MM, Minelli Figueira, R, Pereira Afonso dos Santos, J, Silva Cardoso, C, Luiz Ribeiro, A, and Alkmim, MB. The experience of a sustainable large scale Brazilian telehealth network. Telemed J E Health. (2016) 22:899–908. doi: 10.1089/tmj.2015.0234
14. Collins, GS, Reitsma, JB, Altman, DG, and Moons, KG. Transparent reporting of a multivariable prediction model for individual prognosis or diagnosis (TRIPOD): the TRIPOD statement. BMJ. (2015) 350:g7594. doi: 10.1136/bmj.g7594
15. Moons, KG, Altman, DG, Reitsma, JB, Ioannidis, JPA, Macaskill, P, Steyerberg, EW, et al. Transparent reporting of a multivariable prediction model for individual prognosis or diagnosis (TRIPOD): explanation and elaboration. Ann Intern Med. (2015) 162:W1–W73. doi: 10.7326/M14-0698
16. Wolff, RF, Moons, KGM, Riley, RD, Whiting, PF, Westwood, M, Collins, GS, et al. PROBAST: a tool to assess the risk of Bias and applicability of prediction model studies. Ann Intern Med. (2019) 170:51–8. doi: 10.7326/M18-1376
17. Ji, D, Zhang, D, Xu, J, Chen, Z, Yang, T, Zhao, P, et al. Prediction for progression risk in patients with COVID-19 pneumonia: the CALL score. Clin Infect Dis. (2020) 71:1393–9. doi: 10.1093/cid/ciaa414
18. Garcia-Gordillo, JA, Camiro-Zúñiga, A, Aguilar-Soto, M, Cuenca, D, Cadena-Fernández, A, Khouri, LS, et al. COVID-IRS: a novel predictive score for risk of invasive mechanical ventilation in patients with COVID-19. PLoS One. (2021) 16:e0248357. doi: 10.1371/journal.pone.0248357
19. Barlow, G, Nathwani, D, and Davey, P. The CURB65 pneumonia severity score outperforms generic sepsis and early warning scores in predicting mortality in community-acquired pneumonia. Thorax. (2007) 62:253–9. doi: 10.1136/thx.2006.067371
20. Bartoletti, M, Giannella, M, Scudeller, L, Tedeschi, S, Rinaldi, M, Bussini, L, et al. Development and validation of a prediction model for severe respiratory failure in hospitalized patients with SARS-CoV-2 infection: a multicentre cohort study (PREDI-CO study). Clin Microbiol Infect. (2020) 26:1545–53. doi: 10.1016/j.cmi.2020.08.003
21. Vincent, JL, Moreno, R, Takala, J, Willatts, S, De Mendonça, A, Bruining, H, et al. The SOFA (Sepsis-related organ failure assessment) score to describe organ dysfunction/failure. Intensive Care Med. (1996) 22:707–10. doi: 10.1007/BF01709751
22. Talmor, D, Jones, AE, Rubinson, L, Howell, MD, and Shapiro, NI. Simple triage scoring system predicting death and the need for critical care resources for use during epidemics. Crit Care Med. (2007) 35:1251–6. doi: 10.1097/01.CCM.0000262385.95721.CC
23. Wojnowski, K, Nettboy, S, Kumar, N, et al. Sum score to predict need for mechanical ventilation in patients with COVID-19. Am Thoracic Soc. (2021):A2636–A 2636. doi: 10.1164/ajrccm-conference.2021.203.1_MeetingAbstracts.A2636
24. Knight, SR, Ho, A, Pius, R, Buchan, I, Carson, G, Drake, TM, et al. Risk stratification of patients admitted to hospital with COVID-19 using the ISARIC WHO clinical characterisation protocol: development and validation of the 4C mortality score. BMJ. (2020) 370:m3339. doi: 10.1136/bmj.m3339
25. DeLong, ER, DeLong, DM, and Clarke-Pearson, DL. Comparing the areas under two or more correlated receiver operating characteristic curves: a nonparametric approach. Biometrics. (1988) 44:837–45. doi: 10.2307/2531595
26. Steyerberg, EW. Clinical prediction models: A practical approach to development, validation, and updating. New York: Springer (2009).
27. Rubin, DB, and Wiley, I. Multiple imputation for nonresponse in surveys. Hoboken, N.J: Wiley-Interscience (2004).
28. Safari, S, Baratloo, A, Elfil, M, and Negida, A. Evidence based emergency medicine; part 5 receiver operating curve and area under the curve. Emerg (Tehran). (2016) 4:111–3.
29. Rufibach, K. Use of brier score to assess binary predictions. J Clin Epidemiol. (2010) 63:938–9. doi: 10.1016/j.jclinepi.2009.11.009
30. WHO. (2023). WHO Coronavirus (COVID-19) Dashboard. Available at: https://covid19.who.int/ (Accessed August 18, 2023)
31. Chandel, A, Leazer, S, Alcover, KC, Farley, J, Berk, J, Jayne, C, et al. Intensive care and organ support related mortality in patients with COVID-19: a systematic review and Meta-analysis. Crit Care Explor. (2023) 5:e0876. doi: 10.1097/CCE.0000000000000876
32. Steyerberg, EW, Borsboom, GJJM, van Houwelingen, HC, Eijkemans, MJC, and Habbema, JDF. Validation and updating of predictive logistic regression models: a study on sample size and shrinkage. Stat Med. (2004) 23:2567–86. doi: 10.1002/sim.1844
33. Palaiodimos, L, Kokkinidis, DG, Li, W, Karamanis, D, Ognibene, J, Arora, S, et al. Severe obesity, increasing age and male sex are independently associated with worse in-hospital outcomes, and higher in-hospital mortality, in a cohort of patients with COVID-19 in the Bronx, New York. Metabolism. (2020) 108:154262. doi: 10.1016/j.metabol.2020.154262
34. Zhao, M, Wang, M, Zhang, J, Gu, J, Zhang, P, Xu, Y, et al. Comparison of clinical characteristics and outcomes of patients with coronavirus disease 2019 at different ages. Aging (Albany NY). (2020) 12:10070–86. doi: 10.18632/aging.103298
35. Krishnan, S, Patel, K, Desai, R, Sule, A, Paik, P, Miller, A, et al. Clinical comorbidities, characteristics, and outcomes of mechanically ventilated patients in the state of Michigan with SARS-CoV-2 pneumonia. J Clin Anesth. (2020) 67:110005. doi: 10.1016/j.jclinane.2020.110005
36. Romero Starke, K, Reissig, D, Petereit-Haack, G, Schmauder, S, Nienhaus, A, and Seidler, A. The isolated effect of age on the risk of COVID-19 severe outcomes: a systematic review with meta-analysis. BMJ Glob Health. (2021) 6:e006434. doi: 10.1136/bmjgh-2021-006434
37. Yang, J, Tian, C, Chen, Y, Zhu, C, Chi, H, and Li, J. Obesity aggravates COVID-19: an updated systematic review and meta-analysis. J Med Virol. (2021) 93:2662–74. doi: 10.1002/jmv.26677
38. Deng, L, Zhang, J, Wang, M, and Chen, L. Obesity is associated with severe COVID-19 but not death: a dose-response meta-analysis. Epidemiol Infect. (2021) 149:e144. doi: 10.1017/S0950268820003179
39. Singh, J, Malik, P, Patel, N, Pothuru, S, Israni, A, Chakinala, RC, et al. Kidney disease and COVID-19 disease severity-systematic review and meta-analysis. Clin Exp Med. (2021) 22:125–35. doi: 10.1007/s10238-021-00715-x
40. Ao, G, Wang, Y, Qi, X, Nasr, B, Bao, M, Gao, M, et al. The association between severe or death COVID-19 and solid organ transplantation: a systematic review and meta-analysis. Transplant Rev (Orlando). (2021) 35:100628. doi: 10.1016/j.trre.2021.100628
41. Estenssoro, E, Loudet, CI, Ríos, FG, Kanoore Edul, VS, Plotnikow, G, Andrian, M, et al. Clinical characteristics and outcomes of invasively ventilated patients with COVID-19 in Argentina (SATICOVID): a prospective, multicentre cohort study. Lancet Respir Med. (2021) 9:989–98. doi: 10.1016/S2213-2600(21)00229-0
42. Ñamendys-Silva, SA, Gutiérrez-Villaseñor, A, and Romero-González, JP. Hospital mortality in mechanically ventilated COVID-19 patients in Mexico. Intensive Care Med. (2020) 46:2086–8. doi: 10.1007/s00134-020-06256-3
43. Wu, Z, and McGoogan, JM. Characteristics of and important lessons from the coronavirus disease 2019 (COVID-19) outbreak in China: summary of a report of 72 314 cases from the Chinese Center for Disease Control and Prevention. JAMA. (2020) 323:1239–42. doi: 10.1001/jama.2020.2648
44. Van Calster, B, McLernon, DJ, van Smeden, M, Wynants, L, and Steyerberg, EW. Calibration: the Achilles heel of predictive analytics. BMC Med. (2019) 17:230. doi: 10.1186/s12916-019-1466-7
45. Binuya, MAE, Engelhardt, EG, Schats, W, Schmidt, MK, and Steyerberg, EW. Methodological guidance for the evaluation and updating of clinical prediction models: a systematic review. BMC Med Res Methodol. (2022) 22:316. doi: 10.1186/s12874-022-01801-8
46. World Health Organization. (2021). Tracking SARS-CoV-2 variants. www.who.int. Available at: https://www.who.int/en/activities/tracking-SARS-CoV-2-variants/ (Accessed August 31, 2023)
47. Tegally, H, Moir, M, Everatt, J, Giovanetti, M, Scheepers, C, Wilkinson, E, et al. Emergence of SARS-CoV-2 omicron lineages BA.4 and BA.5 in South Africa. Nat Med. (2022) 28:1785–90. doi: 10.1038/s41591-022-01911-2
48. WHO. (2023). EG.5 Initial Risk Evaluation. Available at: https://www.who.int/docs/default-source/coronaviruse/09082023eg.5_ire_final.pdf?sfvrsn=2aa2daee_3 (Accessed August 21, 2023)
49. Thompson, MG, Natarajan, K, Irving, SA, Rowley, EA, Griggs, EP, Gaglani, M, et al. Effectiveness of a third dose of mRNA vaccines against COVID-19–associated emergency department and urgent care encounters and hospitalizations among adults during periods of Delta and omicron variant predominance — VISION network, 10 states, August 2021–January 2022. MMWR Morb Mortal Wkly Rep. (2022) 71:139–45. doi: 10.15585/mmwr.mm7104e
50. Our World in Data. (2023). Coronavirus (COVID-19) Vaccinations. Available at: https://ourworldindata.org/covid-vaccinations (Accessed May 29, 2023)
51. Wynants, L, van Calster, B, Collins, GS, Riley, RD, Heinze, G, Schuit, E, et al. Prediction models for diagnosis and prognosis of COVID-19 infection: systematic review and critical appraisal. BMJ. (2020) 369:m1328. doi: 10.1136/bmj.m1328
Glossary
Keywords: COVID-19, intensive care unit, prognosis, invasive mechanical ventilation, risk assessment
Citation: Cimini CCR, Delfino-Pereira P, Pires MC, Ramos LEF, Gomes AGdR, Jorge AdO, Fagundes AL, Garcia BM, Pessoa BP, de Carvalho CA, Ponce D, Rios DRA, Anschau F, Vigil FMB, Bartolazzi F, Grizende GMS, Vietta GG, Goedert GMdS, Nascimento GF, Vianna HR, Vasconcelos IM, Alvarenga JCd, Chatkin JM, Machado Rugolo J, Ruschel KB, Zandoná LB, Menezes LSM, Castro LCd, Souza MD, Carneiro M, Bicalho MAC, Cunha MIA, Sacioto MF, Lutkmeier R, Oliveira NRd, Andrade PGS, Menezes RM, Ribeiro ALP and Marcolino MS (2023) Assessment of the ABC2-SPH risk score to predict invasive mechanical ventilation in COVID-19 patients and comparison to other scores. Front. Med. 10:1259055. doi: 10.3389/fmed.2023.1259055
Edited by:
Sergio E. Rodriguez, Centers for Disease Control and Prevention (CDC), United StatesReviewed by:
Janet Tate, Yale University, United StatesBiagio Pinchera, University of Naples Federico II, Italy
Copyright © 2023 Cimini, Delfino-Pereira, Pires, Ramos, Gomes, Jorge, Fagundes, Garcia, Pessoa, de Carvalho, Ponce, Rios, Anschau, Vigil, Bartolazzi, Grizende, Vietta, Goedert, Nascimento, Vianna, Vasconcelos, Alvarenga, Chatkin, Machado Rugolo, Ruschel, Zandoná, Menezes, Castro, Souza, Carneiro, Bicalho, Cunha, Sacioto, Oliveira, Andrade, Lutkmeier, Menezes, Ribeiro and Marcolino. This is an open-access article distributed under the terms of the Creative Commons Attribution License (CC BY). The use, distribution or reproduction in other forums is permitted, provided the original author(s) and the copyright owner(s) are credited and that the original publication in this journal is cited, in accordance with accepted academic practice. No use, distribution or reproduction is permitted which does not comply with these terms.
*Correspondence: Milena Soriano Marcolino, milenamarc@gmail.com
†ORCID: Christiane Corrêa Rodrigues Cimini, https://orcid.org/0000-0002-1973-1343
Magda Carvalho Pires, https://orcid.org/0000-0003-3312-4002
Lucas Emanuel Ferreira Ramos, https://orcid.org/0000-0001-7844-0581
Angélica Gomides dos Reis Gomes, https://orcid.org/0000-0002-4568-0738
Alzira de Oliveira Jorge, https://orcid.org/0000-0003-1366-1732
Ariovaldo Leal Fagundes, https://orcid.org/0000-0002-0359-1948
Bárbara Machado Garcia, https://orcid.org/0000-0001-6538-9086
Bruno Porto Pessoa, https://orcid.org/0000-0002-4212-519X
Daniela Ponce, https://orcid.org/0000-0002-6178-6938
Danyelle Romana Alves Rios, https://orcid.org/0000-0001-6377-241X
Fernando Anschau, https://orcid.org/0000-0002-2657-5406
Flavia Maria Borges Vigil, https://orcid.org/0000-0002-0651-3566
Frederico Bartolazzi, https://orcid.org/0000-0002-9696-4685
Genna Maira Santos Grizende, https://orcid.org/0000-0001-6891-1087
Giovanna Grunewald Vietta, https://orcid.org/0000-0002-0756-3098
Giulia Maria dos Santos Goedert, https://orcid.org/0000-0002-6672-0276
Guilherme Fagundes Nascimento, https://orcid.org/0000-0001-9064-7067
Heloisa Reniers Vianna, https://orcid.org/0000-0003-1144-6262
Isabela Muzzi Vasconcelos, https://orcid.org/0000-0002-4342-7958
Joice Coutinho de Alvarenga, https://orcid.org/0000-0003-3536-7112
José Miguel Chatkin, https://orcid.org/0000-0002-4343-025X
Juliana Machado Rugolo, https://orcid.org/0000-0003-3984-4959
Karen Brasil Ruschel, https://orcid.org/0000-0002-6362-1889
Liege Barella Zandoná, https://orcid.org/0000-0001-6697-6426
Luanna Silva Monteiro Menezes, https://orcid.org/0000-0002-6621-3338
Luís César de Castro, https://orcid.org/0000-0003-2379-0167
Maíra Dias Souza, https://orcid.org/0000-0003-3546-4000
Marcelo Carneiro, https://orcid.org/0000-0003-3603-1987
Maria Aparecida Camargos Bicalho, https://orcid.org/0000-0001-6298-9377
Maria Izabel Alcântara Cunha, https://orcid.org/0000-0002-4903-1276
Manuela Furtado Sacioto, https://orcid.org/0000-0001-7689-0378
Raquel Lutkmeier, https://orcid.org/0000-0002-9519-261X
Rochele Mosmann Menezes, https://orcid.org/0000-0002-1548-1607
Milena Soriano Marcolino, https://orcid.org/0000-0003-4278-3771