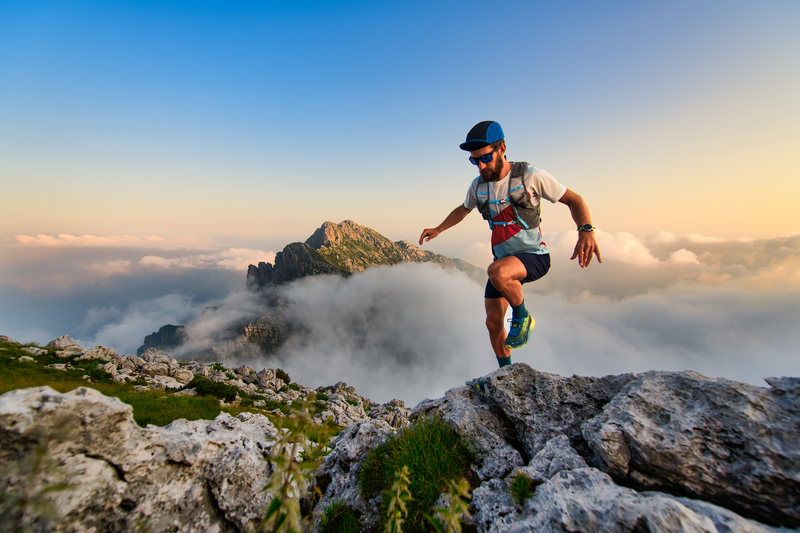
94% of researchers rate our articles as excellent or good
Learn more about the work of our research integrity team to safeguard the quality of each article we publish.
Find out more
EDITORIAL article
Front. Med. , 23 May 2023
Sec. Nuclear Medicine
Volume 10 - 2023 | https://doi.org/10.3389/fmed.2023.1216434
This article is part of the Research Topic Radiomics and Artificial Intelligence in Radiology and Nuclear Medicine View all 13 articles
Editorial on the Research Topic
Radiomics and artificial intelligence in radiology and nuclear medicine
Artificial intelligence (AI) and radiomics algorithms in radiology and nuclear medicine have demonstrated a good performance as diagnostic, predictive or prognostic markers for several diseases with a high potential to be used as clinical tools. However, these algorithms should be further validated in clinical practice to spread their routinary use worldwide.
After the successful publication of a previous Research Topic about Radiomics in Positron Emission Tomography (PET) on Frontiers in Medicine in 2021 (https://www.frontiersin.org/research-topics/15427/artificial-intelligence-in-positron-emission-tomography) a wider and more comprehensive Research Topic on the role of AI and radiomics in radiology and nuclear medicine was launched in 2022. This Research Topic comprises 12 articles.
For successful employment of AI and deep learning algorithms in the clinical practice, explainable artificial intelligence (XAI) could be introduced for several imaging modalities as clearly discussed in the comprehensive review of De Vries et al. including 75 articles (De Vries et al.). However, the authors demonstrated that there is currently no clear consensus on how XAI should be used in order to close the gap between medical professionals and deep learning algorithms for clinical implementation. Furthermore, De Vries et al. also suggested a systematic technical and clinical quality assessment of XAI methods.
Seven articles included in this Research topic are dedicated to the use of AI in oncological imaging.
Radiomic features could be very useful for a better prognostic stratification in patients with glioblastoma. The study of Chiesa et al. including 90 patients with glioblastoma applied a radiomic analysis focusing on healthy tissue ring around the surgical cavity on post-operative magnetic resonance imaging. This study provided a preliminary model for a decision support tool for a customization of the radiation target volume in glioblastoma patients to achieve a margin reduction strategy (Chiesa et al.).
Sun et al. assessed the value of radiomics based on computed tomography (CT) images in the preoperative discrimination between lung invasive adenocarcinomas and non-invasive adenocarcinomas among 1,185 pulmonary nodules. The authors found that radiomics based on CT images showed good predictive performance in discriminating between these tumoral entities, especially in part solid nodule group. Furthermore, radiomics based on contrast enhanced CT images provided no additional value compared to non-contrast enhanced CT images (Sun et al.).
A brief research report evaluated the performance of fluorine-18 fluorodeoxyglucose ([18F]FDG) PET/CT radiomic features to predict overall survival in 50 patients with locally advanced uterine cervical carcinoma. The authors found that standardized uptake value peak (SUVpeak) and the textural feature gray-level run-length matrix (GLRLM) presented the best performance to predict overall survival in patients with cervical cancer undergoing chemo-radiotherapy and brachytherapy (Goncalves de Alencar at al.).
A retrospective study assessed the predictive ability of [18F]FDG PET/CT radiomic features for MYCN, 1p and 11q abnormalities in 122 pediatric patients with neuroblastoma. The authors clearly demonstrated that baseline [18F]FDG PET/CT radiomics is able to predict MYCN amplification and 1p and 11 aberrations in patients with neuroblastoma, thus aiding tumor staging, risk stratification and disease management (Qian et al.).
Another retrospective study on 131 patients explored the application of [18F]FDG PET/CT radiomics in the identification and correct classification of spine multiple myeloma lesions and bone metastases. The radiomics model constructed based on [18F]FDG PET/CT images achieved satisfactory diagnostic performance for the classification of multiple myeloma and bone metastases. In addition, the radiomics model showed significant improvement in diagnostic performance compared to human experts and PET conventional parameters (Jin et al.).
A retrospective study from Morland et al. estimated the ability of a new index, uptake formula, including healthy organs standardized uptake values on [18F]FDG PET/CT to predict event free survival in 163 patients with Hodgkin lymphoma. The Uptake Formula showed a similar performance to total metabolic tumor volume in predicting event free survival in Hodgkin lymphoma (Morland et al.).
Ghezzo et al. tested on a cohort of 85 prostate cancer patients a recently proposed convolutional neural network for the automatic segmentation of intraprostatic cancer lesions on prostate specific membrane antigen PET images. The authors demonstrated that the AI model could be used to automatically segment intraprostatic cancer lesions to define the volume of interest for radiomics or deep learning analysis. However, more robust performance is needed for the generation of AI-based decision support technologies to be proposed in clinical practice (Ghezzo et al.).
Beyond oncological indications of imaging methods, AI may be also applied for other indications. For instance, the review article of Yan et al. have summarized the application of radiomics for predicting recurrent pancreatitis, evaluating the clinical severity of pancreatitis, differentiating pancreatitis from pancreatic adenocarcinoma, and functional abdominal pain from pancreatitis, identifying pancreatis, its risk factors and complications (Yan et al.).
Flaus et al. developed a deep learning-based [18F]FDG PET image enhancement method using simulated brain PET to improve visualization of epileptogenic lesions. However, the authors recommended further evaluation to generalize their method and to assess its clinical performance in a larger cohort (Flaus et al.).
Weakly supervised deep learning models have gained increasing popularity in medical image segmentation. However, these models are not suitable for the critical characteristics presented in chest radiographs: the global symmetry of chest radiographs and dependencies between lesions and their positions. In their study, Gu et al. proposed a weakly supervised model, Chest L-Transformer, to take these characteristics into account. The authors demonstrated a significant segmentation performance improvement over the current state-of-the-art while achieving competitive classification performance (Gu et al.).
Lastly, an original article by Quak et al. including 67 patients demonstrated that the degradation of image quality on PET due to a reduction in injected activity at the end of the 68Ge/68Ga generator lifespan can be effectively counterbalanced by using AI-based PET denoising (Quak et al.).
Finally, we would like to underline that AI and radiomics tools are widely used for research purpose in the fields of radiology and nuclear medicine. Nevertheless, large validation protocols and real-life experience are needed to allow an increasing use of these tools in clinical practice, with possible benefit for patients' treatments and outcomes.
SA and GT drafted the manuscript and revised the final version. All authors contributed to the article and approved the submitted version.
The authors declare that the research was conducted in the absence of any commercial or financial relationships that could be construed as a potential conflict of interest.
All claims expressed in this article are solely those of the authors and do not necessarily represent those of their affiliated organizations, or those of the publisher, the editors and the reviewers. Any product that may be evaluated in this article, or claim that may be made by its manufacturer, is not guaranteed or endorsed by the publisher.
Keywords: radiology, artificial intelligence, radiomics, machine learning, nuclear medicine, imaging, deep learning
Citation: Annunziata S and Treglia G (2023) Editorial: Radiomics and artificial intelligence in radiology and nuclear medicine. Front. Med. 10:1216434. doi: 10.3389/fmed.2023.1216434
Received: 03 May 2023; Accepted: 10 May 2023;
Published: 23 May 2023.
Edited and reviewed by: Francesco Cicone, Magna Græcia University, Italy
Copyright © 2023 Annunziata and Treglia. This is an open-access article distributed under the terms of the Creative Commons Attribution License (CC BY). The use, distribution or reproduction in other forums is permitted, provided the original author(s) and the copyright owner(s) are credited and that the original publication in this journal is cited, in accordance with accepted academic practice. No use, distribution or reproduction is permitted which does not comply with these terms.
*Correspondence: Giorgio Treglia, Z2lvcmdpby50cmVnbGlhQGVvYy5jaA==
Disclaimer: All claims expressed in this article are solely those of the authors and do not necessarily represent those of their affiliated organizations, or those of the publisher, the editors and the reviewers. Any product that may be evaluated in this article or claim that may be made by its manufacturer is not guaranteed or endorsed by the publisher.
Research integrity at Frontiers
Learn more about the work of our research integrity team to safeguard the quality of each article we publish.