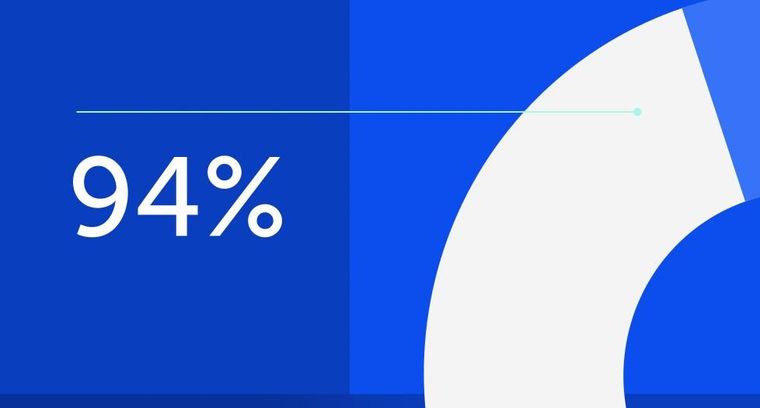
94% of researchers rate our articles as excellent or good
Learn more about the work of our research integrity team to safeguard the quality of each article we publish.
Find out more
REVIEW article
Front. Med., 29 August 2023
Sec. Precision Medicine
Volume 10 - 2023 | https://doi.org/10.3389/fmed.2023.1199605
This article is part of the Research TopicExtending Precision Medicine to Cancer SurvivorshipView all 6 articles
Cancer related cognitive impairment (CRCI) is commonly associated with cancer and its treatments, yet the present binary diagnostic approach fails to capture the full spectrum of this syndrome. Cognitive function is highly complex and exists on a continuum that is poorly characterized by dichotomous categories. Advanced statistical methodologies applied to symptom assessments have demonstrated that there are multiple subclasses of CRCI. However, studies suggest that relying on symptom assessments alone may fail to account for significant differences in the neural mechanisms that underlie a specific cognitive phenotype. Treatment plans that address the specific physiologic mechanisms involved in an individual patient’s condition is the heart of precision medicine. In this narrative review, we discuss how biotyping, a precision medicine framework being utilized in other mental disorders, could be applied to CRCI. Specifically, we discuss how neuroimaging can be used to determine biotypes of CRCI, which allow for increased precision in prediction and diagnosis of CRCI via biologic mechanistic data. Biotypes may also provide more precise clinical endpoints for intervention trials. Biotyping could be made more feasible with proxy imaging technologies or liquid biomarkers. Large cross-sectional phenotyping studies are needed in addition to evaluation of longitudinal trajectories, and data sharing/pooling is highly feasible with currently available digital infrastructures.
Up to 75% of survivors of cancers that originate outside of the central nervous system demonstrate cognitive impairment before, during or following cancer treatments (1–4). CRCI typically presents as difficulties with attention, executive function, memory, and processing speed that can last months or years after the conclusion of treatment (1–5). CRCI can have debilitating effects on survivors’ quality of life including their social and occupational functioning (3, 4, 6–9), and is associated with increased death including cancer-related and all-cause mortality (10–12). Millions of cancer survivors [16.9 million in 2019 alone (13)] are at risk for CRCI.
Cognitive symptoms vary greatly from person to person in terms of domains impacted, as well as symptom severity and trajectory (e.g., late onset, persistent, improving over time). In fact, most symptoms associated with treatment toxicities or cancer pathology are highly variable and unique to each survivor. Thus, recent calls to action have been made for precision survivorship in the field of oncology, where some genetic risks for treatment-related toxicities have been identified in pediatric oncology populations (14). Since 2016, the National Cancer Institute (NCI), has prioritized precision medicine which includes “precision oncology” and “precision survivorship” (15).
The NCI defines precision medicine as using a person’s own biology to diagnose cancer or determine a course of treatment for cancer. Many advancements have been made in precision medicine with respect to cancer diagnostics and treatments, but there has been a lag in applying precision medicine to cancer survivorship, especially for adult cancer survivors (16). Pre-treatment data could be used to determine who is most susceptible to treatment related toxicities, including CRCI.
Precision medicine approaches to CRCI may significantly improve the diagnosis and prognosis of this syndrome. Under or over diagnosing of CRCI incurs significant costs such as negative individual, familial (including impact to the caregiver), and societal burden. The economic benefits of accurate and timely diagnosis have been shown to significantly reduce health care costs. For example, one study showed that health care costs would be reduced by more than half to two-thirds if a diagnosis had been made earlier (17). Patients with CRCI often experience increased stress, anxiety, and depression, loss of employment opportunities, and more social isolation. Patients’ distress can be significantly reduced by health-related perceptions of situational control, validation, and course of deficit, all of which are impossible without a correct diagnosis. Understanding and correctly characterizing the CRCI condition is also imperative for precisely developing and guiding patients toward interventions that can ameliorate their symptoms.
Clinical symptoms of CRCI are currently assessed using standardized neuropsychological tests and/or self-report measures. Guidelines for using neuropsychological tests in CRCI research were published in 2011 (18). These tests provide insight regarding an individual’s cognitive strengths and weaknesses and can assist with diagnosing neuropsychiatric conditions. However, several reports have suggested that these tests may have limited sensitivity, specificity, and reliability for CRCI (19–22). Many of these measures were developed to assess severe neuropathology (21, 23) and therefore may have ceiling effects for CRCI. These tests tend to lack ecological validity (24, 25) and there is also evidence of ethnic or racial biases in standardized neuropsychological testing (26–28).
Guidelines for using self-report measures in CRCI research were published in 2021 (29). Self-report measures reflect daily functioning (30), correlate with mood and psychological factors (24, 30–32), and some were developed specifically for cancer survivor populations (33, 34). Incidences of CRCI are higher when subjective measures are used (3) suggesting they may be more sensitive to this syndrome. However, self-report measures also have several limitations including susceptibility to retrospective recall, response, and state-dependent biases as well as demand characteristics (35–38). Importantly, some studies have found elevated response bias among cancer survivors compared to normative groups (36, 37, 39). Self-reported CRCI has been criticized for rarely correlating with neuropsychological performance (31). However, it is likely that self-report and neuropsychological tests measure different aspects of CRCI and cannot be considered interchangeable (40). Accordingly, evidence suggests that subjective and objective measures of CRCI represent different neural phenotypes. Both objective and subjective measures of CRCI have been shown to correlate with structural and functional brain abnormalities, but these appear to be largely distinct and non-overlapping (41–45).
Neither standardized cognitive tests nor self-report measures can track an individual’s day-to-day variability in cognitive function. Both rely on population-based methods (between group variability) rather than precision-based methods (within person variability). Cognitive functioning is subject to individual variability and real-life demands, thus using one’s own cognitive variability within one’s own environment may be a more ecologically valid and unbiased way of assessing CRCI. A precision health approach to defining CRCI, which is an individually focused approach, is especially beneficial for historically marginalized groups, such as racial/ethnic minorities, as mentioned above.
One of the greatest challenges to clinical management of CRCI is its inconsistent definition within the existing literature. As noted above, CRCI is currently evaluated using neuropsychological test performance. However, these tests rarely provide normative cutoff scores for deficient performance. Therefore, classification of impairment based on these tests in patients with cancer has relied on arbitrary cutoff scores leading to inconsistent results (46). To better harmonize CRCI studies, the International Cognition and Cancer Task Force (ICCTF) suggested a specific metric for determining impairment based on z-scores (18). Few studies have compared the ICCTF definition to other rubrics, although one study showed it was nearly twice as sensitive to impairment compared to other definitions (47). However, different results occur depending on the reference group used for z-score calculation and the size and composition of the testing battery (48, 49).
A major limitation of the z-score and similar approaches, including standardized based regression (50) and reliable change index (22), is that they result in dichotomous classification (yes/not impaired; yes/no declined). Dichotomous classification is not consistent with the complex, continuous nature of brain function. Akin to “black and white thinking,” dichotomization ignores dimensional nuances that could provide greater insight regarding cognitive function (51). In fact, a recent study demonstrated that binary impairment definitions, including the ICCTF criterion, yield unreliable cognitive classifications (52). This is particularly important for CRCI given the historical controversy regarding its existence. Specifically, many patients are classified as “unimpaired” on neuropsychological tests despite experiencing significant difficulties completing everyday cognitive tasks. This inconsistency may suggest that there are latent subgroups of patients whose cognitive changes do not fit into black and white categories.
Several statistical techniques exist that can determine subgroups within the data that are not readily apparent (Table 1). For example, growth mixture modeling, latent profile analyses and clustering have been used to discover CRCI subgroups in a data-driven manner. Growth mixture modeling determines latent subgroups based on different trajectories of an outcome across longitudinal timepoints (69). Prior studies of CRCI using growth mixture modeling have identified up to five latent subgroups of self-reported or objective cognitive function, with the most common finding being three subclasses (53–58).
Once the latent subgroups are identified, characteristics of the subgroups can be examined to determine their clinical significance including factors associated with risk and resilience. For example, using growth mixture modeling, Merriman et al. (54) identified three latent subgroups of subjective CRCI in patients with breast cancer. They labeled the subgroups “more frequent,” “persistent,” and “almost never” based on frequency of cognitive complaints. They found that patients in the “more frequent” subgroup had unique profiles of treatment regimens, genotypes, and psychiatric symptoms compared to the other subgroups (54). Alternatively, covariates can be included in the initial model to examine their impact on the longitudinal outcome (70).
Latent profile analysis also seeks to identify unknown subgroups within a larger population using a probabilistic model that describes the distribution of the data (71). Latent profile analysis is appropriate for cross-sectional and longitudinal data. Like growth mixture modeling, latent profile analysis allows for inclusion of covariates to predict latent subgroup membership. Latent profile analysis has been applied to study CRCI subgroups in both pediatric and adult cancers (59–64).
Other latent general linear model analysis techniques have been used to provide insight regarding the association of self-reported cognitive function and neuropsychological tests in patients with breast cancer (65). However, real-world data do not always meet linear model assumptions. Clustering is another statistical technique useful for discovering latent subgroups which is appropriate for linear or nonlinear cross-sectional or longitudinal data. Clustering is based on distances between data points and aims to identify subgroups within a sample that have both high within-group similarity and high between-group dissimilarity. Very few studies have applied clustering to examine profiles of CRCI. Romero and colleagues identified four subtypes of neuropsychological testing scores using k-means clustering (66). The authors interpreted these findings at the test level in terms of which tests were sensitive to impairment, but their results suggested subgroups of participants with different levels of impairment (66). Another study employed k-means clustering as a sensitivity analysis for their latent profile analysis, both of which identified only a dichotomous solution (59).
These studies represent advances in the literature towards a more precise definition of CRCI. However, the mechanisms that result in various symptoms must be known to develop treatments, particularly the mechanisms that differentiate patients who respond better to one treatment versus another. Mechanistic knowledge cannot be derived from symptom assessments alone given that similar symptoms can result from different pathologies (72). Neuropsychological tests, for example, can identify memory, attention, or other cognitive domain deficits. However, these tests summarize multiple cognitive processes into one score and thus are too broad for identifying the precise neural mechanisms involved. Novel data driven approaches as used in the studies above are more granular and able to classify patients into different subtypes based on behavioral data, but the resultant subtypes do not consider the differences in pathophysiology that would dictate precision treatment.
The biologic mechanisms of cognitive disorders are frequently examined using neuroimaging techniques. Multiple magnetic resonance imaging (MRI) studies have demonstrated that CRCI is a brain-based syndrome characterized by significant changes in brain function and structure. Breast cancer survivors who receive chemotherapy treatment tend to show more pronounced global, cortical gray matter and subcortical white matter volume loss when compared with chemotherapy naïve and noncancer controls [see reviews by Sousa et al. (41), Schroyen et al. (42), and Niu et al. (73)]. McDonald et al. (74) reported the first prospective, longitudinal volumetric MRI study of CRCI. They observed a significant reduction over time in regional gray matter density from pre-chemotherapy to 1-month post-chemotherapy followed by partial improvement over time (74). Gray matter alterations have also been demonstrated in very long-term survivors (75–77). We uniquely used volumetric MRI to estimate cortical brain age and demonstrated that it is significantly increased from pre- to post- breast cancer chemotherapy (78). We also distinctively demonstrated that chemotherapy treated breast cancer survivors are significantly more likely to be classified as having incipient Alzheimer’s disease based on volumetric brain network organization compared to chemotherapy naïve survivors (79).
Pathologic white matter changes determined using T2-weighted MRI in patients with cancer exposed to chemotherapy were first described in the nineties (80–82). These changes tended to occur acutely after chemotherapy completion but persisted at 1 year of follow-up (81). Diffusion tensor imaging (DTI) has demonstrated abnormal white matter integrity in the corpus callosum of chemotherapy treated patients with breast cancer compared to noncancer controls (83). Subsequent studies have shown diffuse white matter integrity abnormalities that correlate with cognitive impairment (84, 85), indicating that a demyelinating process may partly underlie CRCI (75). DTI was also uniquely used by our group to computationally simulate the effects of aging on white matter organization, demonstrating that chemotherapy treated breast cancer survivors have lower resilience to brain aging compared to non-cancer controls (86).
Task-based functional MRI (fMRI) studies suggest that certain cognitive tasks are more challenging for patients with breast cancer as they demonstrate expanded recruitment of brain regions to maintain task accuracy. Several lines of research suggest that chemotherapy used in treatment of breast cancer upregulates neural activity (82, 87–91). Brain hypoactivation compared to non-cancer controls has also been reported in several cross-sectional studies (92, 93), yet brain hyperactivation is more often evident over time and is associated with self-reported cognitive complaints (90, 91, 94, 95).
Hyperactivation may therefore help explain the discrepancy that is commonly observed between neuropsychological tests and self-reports of cognitive function. Specifically, patients with breast cancer often demonstrate normal objective task performance but report significant cognitive difficulties (21, 96). Hyperactivation is believed to reflect neural compensation in response to brain injury, which can thus mask the underlying deficit (92). However, patient awareness of the additional neural effort required to maintain performance is reflected in their low self-ratings of cognitive function. Hyperactivation has been consistently observed in association with aging and is greater in individuals with age-related neurodegeneration (97–99).
Resting state fMRI functional connectivity studies provide insight regarding the brain network’s organization in terms of parallel information processing. Some studies show diffuse hyperconnectivity in chemotherapy exposed breast cancer patients and survivors compared to controls (88, 100, 101) while others have shown hypoconnectivity (45, 102–106). Given the brain’s vast complexity, the interpretation of hyper- vs. hypo- activation/connectivity is difficult especially since both can exist simultaneously. Most observations have been made by comparing patients and controls. It is likely that there are subgroups of patients characterized by distinct patterns of brain abnormalities which may help clarify some of the inconsistent findings observed across studies.
Historically, neuropsychiatric conditions have been diagnosed and categorized based on the patient’s symptoms and the level of distress or functional impairment they experience. However, this approach is limited as it fails to capture the wide-ranging neurobiological mechanisms that can underlie similar symptomatology. This poses a significant challenge for the development of precision medicine, which requires a more nuanced understanding of the underlying mechanisms. To improve precision medicine, neuropsychiatric research is currently focusing on diagnoses that emphasize biology. For example, the National Institutes of Health’s Research Domain Criteria (RDoC) (107) and the Bipolar-Schizophrenia Network for Intermediate Phenotypes (B-SNIP) (108) projects aim to define subgroups of patients within a traditional diagnostic category based on neurobiologic profiles (107, 109).
The RDoC framework involves examination of psychological and biological dimensions across various levels of functioning within six primary functional domains. The RDoC framework emphasizes the measurement of behavioral, physiological, and self-reported data to gain a comprehensive understanding of each diagnostic dimension. The primary goal of the RDoC framework is to facilitate new research approaches that will enhance precision medicine for mental health conditions. B-SNIP is a multi-site consortium that aims to establish biotypes of mood and psychotic disorders (110, 111). Their work has resulted in a large, well-described neuropsychiatric population in which the RDoC framework can be applied. This includes examining different aspects of biotype expression such as demographics, symptom clusters, genetic variation, and treatment response.
Machine learning techniques applied to neuroimaging data can be used to identify subgroups with distinct profiles of brain abnormalities (Figure 1). These biotypes represent disease subtypes based on their neural mechanisms. Most biotyping studies to date have relied on resting state functional magnetic fMRI. Resting state fMRI is relatively easy to acquire as it has no behavioral requirements, and it has consistently been shown across multiple studies to be highly sensitive to even subtle neuropathology (112). Resting state fMRI data are used to measure the connectivity of intrinsic functional brain networks (113). The sensitivity of these functional brain networks stems from various factors including their relatively high consumption of physiological resources, their association with gene expression patterns that are important for synaptic function and their involvement in multiple aspects of information processing (114–117).
Figure 1. Overview of biotyping using neuroimaging. Neuroimaging can be used to determine biological subtypes (biotypes) of cancer-related cognitive impairment (CRCI). A subtyping algorithm, such as clustering, is applied to neuroimaging data (volumes, connectivity values, etc.). This results in distinct subtypes of patients who have common patterns of brain function or structure that may represent neural mechanisms of cognitive impairment. Behavioral or clinical characteristics, such as cognitive test performance, are then measured for each subgroup to determine the clinical relevance of the subgroups. Best practice is to then test the biotypes by applying the subtyping algorithm solution to an independent validation sample of patients, measuring the characteristics of interest and comparing these results to those from the original sample. Other validation methods include applying a naive algorithm to the data to determine if the same biotypes are replicated and examining longitudinal trajectories of different biotypes.
Using machine learning and resting state fMRI data, Drysdale and colleagues identified four unique patterns of abnormal intrinsic functional brain connectivity in individuals with major depressive disorder (118). Each of the four biotypes presented with a unique profile of clinical features, highlighting the imprecision of the single diagnostic category used to describe them. Using similar methods, biotypes of other neuropsychiatric and neurological conditions have also been identified, including bipolar disorder, multiple sclerosis, Parkinson’s disease, attention deficit disorder, and schizophrenia (119–124).
To date, there have been few studies of CRCI biotypes. By applying clustering algorithms to resting state brain fMRI functional connectivity data, we previously identified three distinct profiles of abnormal brain connectivity, i.e., biotypes, in chemotherapy treated breast cancer survivors (67). Each biotype was associated with a unique cognitive phenotype as well as distinct demographic and clinical characteristics. We also demonstrated that our biotype algorithm was reproducible in an independent dataset. In a follow-up study (68), we demonstrated that each biotype had unique genetic and psychologic characteristics. We also showed that dichotomous, symptom-based classification combined patients with distinct profiles of abnormal brain connectivity into a single category of impaired cognition (68). In other words, divergent biological mechanisms were ignored by symptom assessment. Additionally, symptom-based assessment was unable to distinguish cognitive, genetic, or psychologic characteristics. Further research is needed to determine the reliability of biotypes across cancer types, if biotypes can predict different CRCI trajectories, and importantly if biotypes are associated with different responses to CRCI interventions.
Another critical barrier to appropriate clinical management of CRCI is the inability to determine which patients will experience this adverse event. Several studies have revealed correlations between CRCI and older age, lower physical and mental activity (i.e., cognitive reserve), higher disease severity and greater chemotherapy treatment intensity (125–127). As described above, neuroimaging studies indicate that the final common biologic pathway of cancer-related cognitive impairment is altered brain structure and function (4, 128, 129). Several studies have shown that baseline neuroimaging can be used to build models that are accurately predictive of future cognitive outcomes. For example, baseline quantitative MRI has been used previously to accurately predict the onset of neurogenerative disorders, post-neurosurgical cognitive status, and cognitive rehabilitation outcome (130–134).
Although traditional inferential statistical approaches can be valuable in this space, machine learning algorithms (135) are more commonly used to build these predictive models because of the high dimensional and often nonlinear nature of quantitative neuroimaging data. Additionally, the priority for predictive models tends to be generalizability rather than inference and machine learning methods emphasize accuracy across samples and conditions. CRCI is a complex, heterogeneous condition with numerous potential contributors and confounds that must be considered in predictive models. The large number of potential demographic and clinical predictors in combination with the high dimensional nature of neuroimaging data warrants large sample sizes which are not always feasible. Compared to traditional statistical methods, machine learning algorithms are often better at handling this “large p, small n” problem (136). Several studies have demonstrated that the inclusion of quantitative neuroimaging metrics significantly improves the accuracy of machine learning predictive models for cognitive outcomes (137–139).
Some studies have shown cognitive impairment and brain injury in patients with breast cancer prior to chemotherapy treatment suggesting that tumor pathogenesis may contribute to CRCI (7, 140–146). These early abnormalities may reflect a vulnerable baseline condition within the brain that is later compounded by anti-cancer treatments, resulting in chronic CRCI. Our group pioneered the use of pre-treatment neuroimaging biomarkers to predict long-term CRCI following breast cancer treatment (147, 148) and other groups have since followed (149). However, this research has relied on binary cognitive outcomes or continuous outcomes with no definition of deficit. Thus, the identification of CRCI biotypes could increase the precision of these prediction algorithms.
As noted above, biotyping can also be used to predict intervention response. Given the complexity of neuropsychiatric disorders, several treatment regimens must often be tried to find the most effective one for a given patient. Interventions for CRCI are currently very limited but several show some promise, including cognitive training, cognitive rehabilitation, physical exercise, and neuromodulation (150–154). However, it is unknown which patients benefit most from which intervention. The traditional trial-and-error approach to prescribing interventions results in unnecessary side effects, time toxicity, increased costs, and delays in symptom management.
The overarching goal of biotyping is to identify disease subtypes that respond differently to various interventions such that precise treatment plans can be made for patients within that subtype. Drysdale et al. demonstrated different outcomes among depression biotypes after transcranial magnetic stimulation treatment (118). Specifically, using biotypes, they identified a priori which patients were most likely to benefit from the intervention. It is likely that patients with CRCI will not homogeneously respond to any one intervention and therefore biotypes could be useful in predicting treatment response. However, few if any CRCI intervention studies to date have included neuroimaging biomarkers.
Once a precision health CRCI diagnosis or prognosis is made, an intervention may be required. Currently, there are no standardized, evidence-based interventions specifically for CRCI. However, several pharmacological and behavioral/integrative interventions have been examined as potential treatments for CRCI. Pharmaceutical treatments have included psychostimulants (155, 156), nicotine patches (157), anemia medications (158), and anti-dementia medications (159). Nguyen and Ehrlich described multiple other drugs that could potentially be repurposed for treating CRCI (160). Preclinical studies have evaluated several novel agents including PAN-811, a ribonucleotide reductase inhibitor (161), mesenchymal stem cells (162–164), functionalized mitochondria (165), dual leucine zipper kinase (166), histone deacetylase 6 inhibitor (167), KU-32, for mitochondrial repair (168), and astaxanthin, an antioxidant (169), among others. However, these treatments have yet to be translated into clinical studies.
There have also been several studies examining behavioral (e.g., psychoeducational, cognitive behavioral therapy, compensatory strategies, cognitive training, cognitive rehabilitation), lifestyle (e.g., physical activity, diet, stress management), neuromodulation (neurofeedback, transcranial magnetic stimulation), and integrative (e.g., acupuncture, music, meditation, yoga) interventions for CRCI (153, 170–176). A meta-analysis of 29 randomized control trials reported that the best options among behavioral interventions for CRCI in descending order of efficacy were: meditation/mindfulness-based stress reduction, combined cognitive training with exercise, cognitive training, cognitive rehabilitation, exercise, cognitive behavioral therapy, qigong, supportive therapy, yoga, and acupuncture (170). Another meta-analysis of nonpharmacological interventions for CRCI reported significant effects on objective measures of attention, immediate recall, and processing speed and subjective cognitive function, depending on intervention type and mode of delivery (177).
Measuring outcomes in CRCI intervention trials based on symptoms alone may yield inaccurate results given the limited sensitivity and specificity these assessments have for CRCI and the lack of reliable impairment cutoffs. For example, several previous CRCI intervention trials have observed few if any significant changes in cognitive testing scores yet demonstrated significant effects on functional brain metrics in breast cancer survivors (178, 179). Stimulant medication trials for CRCI have shown very mixed results (180) despite anecdotal evidence that these medications can be helpful for certain patients with CRCI and fatigue. It is possible that a subgroup of patients benefits from these medications or that the outcome measures used in these trials were not sufficiently sensitive for detecting cognitive changes. Biotypes allow us to identify biologically based classifications that could be used as clinical endpoints for intervention trials and to determine more accurate cut off scores for classifying impairment from symptom assessments.
Animal models are not likely to be specifically useful for biotyping or prediction modeling since these methods rely on the patient’s individual biology and developing animal models for CRCI poses significant challenges due to the intricate nature of replicating the human disease (181). However, for optimal clinical utility, CRCI diagnostic and prediction models require further knowledge regarding which chemotherapies are associated with cognitive deficits. The biological effects exerted by each chemotherapy drug are extensive, and this complexity increases when combined with other medications. This cannot be ethically examined in human patients given that patients cannot be randomized to different treatment regimens. Preclinical models offer the advantage of assessing individual chemotherapeutic agents and elucidating their specific molecular mechanisms. By employing preclinical models, researchers can also better regulate variables such as age, sex, environmental factors, type of cancer, treatment types, and comorbidities [see review by Seigers et al. (182)]. Several recent studies have demonstrated cognitive and neurobiologic deficits in mice treated with doxorubicin, cyclophosphamide, or cisplatin chemotherapy (183–188). Potential mechanisms of CRCI examined in these studies include sphingosine-1-phosphate receptor 1 activation, brain derived neurotrophic factor levels, apolipoprotein E4 genotype, and neuroinflammation [see review by Gibson and Monje (189) for potential mechanisms]. However, few studies have compared different chemotherapy regimens or utilized combination chemotherapies and therefore, significant work is still required in this area.
Biotyping is a nascent field that warrants replication of results across several dimensions, which is known as deep validation (72, 190). Deep validation steps including applying an existing biotype algorithm to new, independent data without reclustering to determine if biotype expression remains the same as in the original data, clustering independent data with a naïve algorithm to determine if biotypes can be reproduced, and extending cross-sectional biotypes to longitudinal data (72). These methods aim to determine whether the existence of biotypes in a particular condition is reliable, if the original biotyping solution is generalizable, and if biotypes can predict different disease trajectories.
Most biotyping studies to date have employed fMRI neuroimaging. However, neuroimaging it is not currently standard practice for breast or other non-CNS cancers, it is costly and associated with contraindications such as electronic or magnetic biomedical implants, magnetic orthodontia, and claustrophobia, among others. Neuronal activity is associated with increased oxygen delivery and the difference, or contrast between diamagnetic oxygenated hemoglobin and paramagnetic deoxygenated hemoglobin is measured by MRI. This is known as the blood-oxygen-level-dependent (BOLD) contrast and is the standard method used in functional MRI (191). There are other non-invasive technologies that are used to measure brain activity including electroencephalogram and magnetoencephalography. However, functional near-infrared spectroscopy (fNIRS) is the most similar available technology to fMRI. FNIRS also measures the BOLD contrast but does so using near-infrared light. Whereas most biological tissues are transparent to near-infrared light between 700 and 900 nanometers, hemoglobin absorbs and scatters near-infrared light in this range. Oxygenated and deoxygenated hemoglobin absorb near-infrared light at different wavelengths, which constitutes the BOLD contrast for fNIRS measurement (192, 193). FNIRS is highly tolerant to motion and has no environmental restrictions, contraindications or known risks (194).
The primary limitations of fNIRS are that it can only measure cortical tissue and cannot penetrate to subcortical structures (194) and most commercially available fNIRS devices only measure frontal regions. However, it is important to point out that prefrontal cortex is involved in most brain functions (195–197). Compared to chemotherapy naïve patients, chemotherapy-treated patients with breast cancer consistently show significantly changed prefrontal structure and function (74, 79, 82, 85, 198–201). Altered prefrontal regions are among the most predictive of long-term cognitive status in patients with breast cancer (147, 148). Further, in patients with breast cancer, lower prefrontal-executive function is the single best predictor of medication non-adherence (202) and has been associated with decreased quality of life following chemotherapy treatment (203). Thus, prefrontal fNIRS has significant potential as a proxy for whole brain fMRI in the study of CRCI that could be more easily used in-clinic. Surprisingly, no studies to date have applied fNIRS to the study of CRCI.
The correspondence between liquid and neuroimaging biomarkers is another avenue of research for discovering potential proxy technologies that support biotyping. Several lines of evidence suggest that cancer and its therapies accelerate aging processes (77, 86, 204–206). Individuals with age-related neurodegeneration and related cognitive decline tend to demonstrate greater amyloid-beta peptide accumulation and tau hyperphosphorylation compared to cognitively normal individuals (207). Tau and amyloid-beta can be measured from serum or plasma using the commercially available, single-molecule array (SIMOA) assay (208–210). Several studies have demonstrated that blood-based measurements of amyloid-beta or tau using SIMOA are associated with age-related neurogenerative conditions (211–214) as well as other neurologic conditions (215, 216). We uniquely demonstrated that blood-based amyloid beta and tau are highly predictive of cognitive functioning in breast cancer survivors, in combination with age, cytokines, and anthropomorphic measures (217).
Inflammatory biomarkers have long been of interest to researchers studying CRCI. Objective measures of CRCI have been shown to correlate with some inflammatory cytokines (e.g., IL-1β, IL-6, TNF-α, CRP), other proteins (e.g., GM-CSF), neurodegenerative markers (e.g., Aβ − 42, Aβ − 40, tau), and methylation ratios (42). Subjective measures of CRCI have demonstrated both significant and nonsignificant correlations with cytokines (IL-4, IL-1β, IL-6, MCP-1, IGF-1) and no significant correlations with TNF-alpha or CRP (42), suggesting perhaps more complex and inconsistent relationships between inflammatory biomarkers and CRCI.
Circadian biomarkers that can be measured objectively with saliva and blood or biorhythms (e.g., actigraphy) and are implicated in numerous side effects seen in cancer including CRCI. Similarly, to inflammatory biomarkers, data on circadian markers and CRCI relationships have been inconsistent and additionally quite limited in cancer research. For example, a recent study by Ancoli-Israel and colleagues showed that disrupted circadian rhythms were associated with reductions in objectively measured neurocognitive function in their longitudinal study of women with breast cancer (218). However, other studies failed to find any association between circadian rhythmicity and cognition in women with metastatic breast cancer (219). We suggest more research is warranted on the relationships between liquid and rhythm biomarkers in CRCI.
Neurofilament light, NF-L, a marker of neuroaxonal injury, is another protein that has received recent attention in relation to neurodegenerative diseases, including Alzheimer’s dementia (220). Increased blood concentration of NF-L have also been detected in person with frontotemporal dementia (221), Huntington’s disease (222), and Parkinson’s disease (223), suggesting that NF-L may be a biomarker of neurodegenerative processes in general. Blood based assays using SIMOA to detect NF-L are commercially available but no studies of NF-L and CRCI have been conducted to date.
Another emerging biomarker of cognitive decline is known as neuron derived exosomes (NDE). Exosomes are extracellular vesicles that play an integral role in intracellular communication by conveying materials such as RNA and proteins between cells (224). Exosomes are released by a most cell types, including neurons, and can be detected in blood, saliva, and urine (225, 226). NDE’s appear to contribute to cognitive impairment by transporting toxins during pathological conditions (224). This is evidenced by findings of increased NDE levels in individuals with neurodegenerative syndromes (227, 228). Koh et al. provided a comprehensive overview of NDEs as potential biomarkers of CRCI (229).
CRCI research initiatives, including many funding opportunities, have emphasized the need for longitudinal studies as they determine patterns of individual cognitive change which can be more sensitive than a single static cognitive assessment. Longitudinal studies can also provide insight regarding the separate and combined effects of different anti-cancer treatments on cognitive function. However, large cross-sectional studies should not be neglected or discounted as they help establish reliable syndrome phenotypes (230) and are necessary for the initial steps in biotyping. Large multi-site, national studies or pooled studies are needed to validate neuroimaging biomarkers and biotypes of CRCI. Data sharing and pooling can be facilitated by online databases such as EBRAINS,1 the Human Connectome Project,2 and LONI,3 among others.
Finally, most CRCI studies to date have focused on breast cancer although growing evidence indicates cognitive impairment across cancer types (231). A critical component of future research will be the ability to demonstrate that biotypes can be applied to other cancer diagnoses to determine what CRCI is, agnostic to disease. Further research is required to examine biomarkers of CRCI across different cancer types.
CRCI is a prevalent condition that is not fully understood and, as a result, not diagnosed accurately, limiting treatment trials and implementation. Advanced statistical methodology, such as growth mixture modeling, allows for determination of latent subgroups using self-report and longitudinal data, offering significantly more precision in understanding cognitive trajectories and outcomes. However, the main limitation of symptom-driven subtypes classification remains lack of biological data. Neuroimaging can be used to identify brain biomarkers both individually and in clusters to define biological subgroups to diagnosis and predict outcomes. Like symptom-derived subtypes, biotyping accounts for variability in patient functioning by evaluating brain function to define the best taxonomy for patients but does so by utilizing the mechanistic information required for precision medicine.
SK: conception or design. SK, AH, SP, OP, and MW: acquisition, analysis, or interpretation of data, and drafting and revising the work. All authors contributed to the article and approved the submitted version.
This work was supported by funding from the National Institutes of Health (1R01CA226080, 2R01CA172145).
The authors declare that the research was conducted in the absence of any commercial or financial relationships that could be construed as a potential conflict of interest.
The handling editor NS declared a past co-authorship with the author SK.
All claims expressed in this article are solely those of the authors and do not necessarily represent those of their affiliated organizations, or those of the publisher, the editors and the reviewers. Any product that may be evaluated in this article, or claim that may be made by its manufacturer, is not guaranteed or endorsed by the publisher.
1. Lange, M, Joly, F, Vardy, J, Ahles, T, Dubois, M, Tron, L, et al. Cancer-related cognitive impairment: an update on state of the art, detection, and management strategies in cancer survivors. Ann Oncol. (2019) 30:1925–40. doi: 10.1093/annonc/mdz410
2. Boscher, C, Joly, F, Clarisse, B, Humbert, X, Grellard, JM, Binarelli, G, et al. Perceived cognitive impairment in breast Cancer survivors and its relationships with psychological factors. Cancers (Basel). (2020) 12:3000. doi: 10.3390/cancers12103000
3. Janelsins, MC, Kesler, SR, Ahles, TA, and Morrow, GR. Prevalence, mechanisms, and management of cancer-related cognitive impairment. Int Rev Psychiatry. (2014) 26:102–13. doi: 10.3109/09540261.2013.864260
4. Wefel, JS, Kesler, SR, Noll, KR, and Schagen, SB. Clinical characteristics, pathophysiology, and management of noncentral nervous system cancer-related cognitive impairment in adults. CA Cancer J Clin. (2015) 65:123–38. doi: 10.3322/caac.21258
5. Koppelmans, V, de Groot, M, de Ruiter, MB, Boogerd, W, Seynaeve, C, Vernooij, MW, et al. Global and focal white matter integrity in breast cancer survivors 20 years after adjuvant chemotherapy. Hum Brain Mapp. (2014) 35:889–99. doi: 10.1002/hbm.22221
6. Taillibert, S, Voillery, D, and Bernard-Marty, C. Chemobrain: is systemic chemotherapy neurotoxic? Curr Opin Oncol. (2007) 19:623–7. doi: 10.1097/CCO.0b013e3282f0e224
7. Ahles, TA, Saykin, AJ, McDonald, BC, Furstenberg, CT, Cole, BF, Hanscom, BS, et al. Cognitive function in breast cancer patients prior to adjuvant treatment. Breast Cancer Res Treat. (2008) 110:143–52. doi: 10.1007/s10549-007-9686-5
8. Boykoff, N, Moieni, M, and Subramanian, SK. Confronting chemobrain: an in-depth look at survivors' reports of impact on work, social networks, and health care response. J Cancer Surviv. (2009) 3:223–32. doi: 10.1007/s11764-009-0098-x
9. Owusuaa, C, Dijkland, SA, Nieboer, D, van der Heide, A, and van der Rijt, CCD. Predictors of mortality in patients with advanced cancer – a systematic review and meta-analysis. Cancers (Basel). (2022) 14:328. doi: 10.3390/cancers14020328
10. Rostamian, S, le Cessie, S, Marijt, KA, Jukema, JW, Mooijaart, SP, van Buchem, MA, et al. Association of cognitive function with increased risk of cancer death and all-cause mortality: longitudinal analysis, systematic review, and meta-analysis of prospective observational studies. PLoS One. (2022) 17:e0261826. doi: 10.1371/journal.pone.0261826
11. Libert, Y, Dubruille, S, Borghgraef, C, Etienne, AM, Merckaert, I, Paesmans, M, et al. Vulnerabilities in older patients when cancer treatment is initiated: does a cognitive impairment impact the two-year survival? PLoS One. (2016) 11:e0159734. doi: 10.1371/journal.pone.0159734
12. Sattar, S, Haase, K, Tejero, I, Bradley, C, Mariano, C, Kilgour, H, et al. The impact of cognitive impairment on treatment toxicity, treatment completion, and survival among older adults receiving chemotherapy: a systematic review. Cancers (Basel). (2022) 14:1582. doi: 10.3390/cancers14061582
13. American Cancer Society. Cancer Treatment & Survivorship Facts & Figures 2019–2021. Atlanta, GA: (2019). Available at: https://www.cancer.org/research/cancer-facts-statistics/survivor-facts-figures.html (Accessed June 13, 2023).
14. Chow, EJ, Antal, Z, Constine, LS, Gardner, R, Wallace, WH, Weil, BR, et al. New agents, emerging late effects, and the development of precision survivorship. J Clin Oncol. (2018) 36:2231–40. doi: 10.1200/JCO.2017.76.4647
15. NCI and the Precision Medicine Initiative. (2017). Available at: https://www.cancer.gov/research/areas/treatment/pmi-oncology. (Accessed July 11, 2022)
16. Brant, JM, and Mayer, DK. Precision medicine: accelerating the science to revolutionize Cancer care. Clin J Oncol Nurs. (2017) 21:722–9. doi: 10.1188/17.CJON.722-729
17. Willmen, T, Volkel, L, Ronicke, S, Hirsch, MC, Kaufeld, J, Rychlik, RP, et al. Health economic benefits through the use of diagnostic support systems and expert knowledge. BMC Health Serv Res. (2021) 21:947. doi: 10.1186/s12913-021-06926-y
18. Wefel, JS, Vardy, J, Ahles, T, and Schagen, SB. International cognition and Cancer task force recommendations to harmonise studies of cognitive function in patients with cancer. Lancet Oncol. (2011) 12:703–8. doi: 10.1016/S1470-2045(10)70294-1
19. Nelson, WL, and Suls, J. New approaches to understand cognitive changes associated with chemotherapy for non-central nervous system tumors. J Pain Symptom Manag. (2013) 46:707–21. doi: 10.1016/j.jpainsymman.2012.11.005
20. Savard, J, and Ganz, PA. Subjective or objective measures of cognitive functioning-What's more important? JAMA Oncol. (2016) 2:1263–4. doi: 10.1001/jamaoncol.2016.2047
21. Horowitz, TS, Suls, J, and Trevino, M. A call for a neuroscience approach to Cancer-related cognitive impairment. Trends Neurosci. (2018) 41:493–6. doi: 10.1016/j.tins.2018.05.001
22. Andreotti, C, Root, JC, Schagen, SB, McDonald, BC, Saykin, AJ, Atkinson, TM, et al. Reliable change in neuropsychological assessment of breast cancer survivors. Psychooncology. (2016) 25:43–50. doi: 10.1002/pon.3799
23. Ahles, TA, and Root, JC. Cognitive effects of Cancer and Cancer treatments. Annu Rev Clin Psychol. (2018) 14:425–51. doi: 10.1146/annurev-clinpsy-050817-084903
24. Hutchinson, AD, Hosking, JR, Kichenadasse, G, Mattiske, JK, and Wilson, C. Objective and subjective cognitive impairment following chemotherapy for cancer: a systematic review. Cancer Treat Rev. (2012) 38:926–34. doi: 10.1016/j.ctrv.2012.05.002
25. Howieson, D. Current limitations of neuropsychological tests and assessment procedures. Clin Neuropsychol. (2019) 33:200–8. doi: 10.1080/13854046.2018.1552762
26. Postal, K. Comment on Cory, 2021: "white privilege in clinical neuropsychology: an invisible 'knapsack' in need of unpacking,". Clin Neuropsychol. (2021) 35:224–6. doi: 10.1080/13854046.2020.1844297
27. Cory, JM. White privilege in neuropsychology: An 'invisible knapsack' in need of unpacking? Clin Neuropsychol. (2021) 35:206–18. doi: 10.1080/13854046.2020.1801845
28. Rivera Mindt, M, Byrd, D, Saez, P, and Manly, J. Increasing culturally competent neuropsychological services for ethnic minority populations: a call to action. Clin Neuropsychol. (2010) 24:429–53. doi: 10.1080/13854040903058960
29. Henneghan, AM, Van Dyk, K, Kaufmann, T, Harrison, R, Gibbons, C, Heijnen, C, et al. Measuring self-reported Cancer-related cognitive impairment: recommendations from the Cancer neuroscience initiative working group. J Natl Cancer Inst. (2021) 113:1625–33. doi: 10.1093/jnci/djab027
30. Costa, DSJ, and Fardell, JE. Why are objective and perceived cognitive function weakly correlated in patients with Cancer? J Clin Oncol. (2019) 37:1154–8. doi: 10.1200/JCO.18.02363
31. Bray, VJ, Dhillon, HM, and Vardy, JL. Systematic review of self-reported cognitive function in cancer patients following chemotherapy treatment. J Cancer Surviv. (2018) 12:537–59. doi: 10.1007/s11764-018-0692-x
32. Henneghan, A, Stuifbergen, A, Becker, H, Kesler, S, and King, E. Modifiable correlates of perceived cognitive function in breast cancer survivors up to 10 years after chemotherapy completion. J Cancer Surviv. (2018) 12:224–33. doi: 10.1007/s11764-017-0661-9
33. Fieo, R, Ocepek-Welikson, K, Kleinman, M, Eimicke, JP, Crane, PK, Cella, D, et al. Measurement equivalence of the patient reported outcomes measurement information system® (PROMIS®) applied cognition - general concerns, short forms in ethnically diverse groups. Psychol Test Assess Model. (2016) 58:255–307.
34. Teresi, JA, and Jones, RN. Methodological issues in examining measurement equivalence in patient reported outcomes measures: methods overview to the two-part series, "measurement equivalence of the patient reported outcomes measurement information system® (PROMIS®) short forms". Psychol Test Assess Model. (2016) 58:37–78.
35. Thong, MSY, Chan, RJ, van den Hurk, C, Fessele, K, Tan, W, Poprawski, D, et al. Going beyond (electronic) patient-reported outcomes: harnessing the benefits of smart technology and ecological momentary assessment in cancer survivorship research. Support Care Cancer. (2021) 29:7–10. doi: 10.1007/s00520-020-05648-x
36. O'Leary, TE, Diller, L, and Recklitis, CJ. The effects of response bias on self-reported quality of life among childhood cancer survivors. Qual Life Res. (2007) 16:1211–20. doi: 10.1007/s11136-007-9231-3
37. Harila, MJ, Salo, J, Lanning, M, Vilkkumaa, I, and Harila-Saari, AH. High health-related quality of life among long-term survivors of childhood acute lymphoblastic leukemia. Pediatr Blood Cancer. (2010) 55:331–6. doi: 10.1002/pbc.22531
38. Burchett, D, and Ben-Porath, YS. Methodological considerations for developing and evaluating response bias indicators. Psychol Assess. (2019) 31:1497–511. doi: 10.1037/pas0000680
39. Sneeuw, KC, Aaronson, NK, Osoba, D, Muller, MJ, Hsu, M-A, Yung, A, et al. The use of significant others as proxy raters of the quality of life of patients with brain cancer. Med Care. (1997) 35:490–506. doi: 10.1097/00005650-199705000-00006
40. Dang, J, King, KM, and Inzlicht, M. Why are self-report and behavioral measures weakly correlated? Trends Cogn Sci. (2020) 24:267–9. doi: 10.1016/j.tics.2020.01.007
41. Sousa, H, Almeida, S, Bessa, J, and Pereira, MG. The developmental trajectory of Cancer-related cognitive impairment in breast Cancer patients: a systematic review of longitudinal neuroimaging studies. Neuropsychol Rev. (2020) 30:287–309. doi: 10.1007/s11065-020-09441-9
42. Schroyen, G, Vissers, J, Smeets, A, Gillebert, CR, Lemiere, J, Sunaert, S, et al. Blood and neuroimaging biomarkers of cognitive sequelae in breast cancer patients throughout chemotherapy: a systematic review. Transl Oncol. (2022) 16:101297. doi: 10.1016/j.tranon.2021.101297
43. Zeng, Y, Cheng, ASK, Song, T, Sheng, X, Zhang, Y, Liu, X, et al. Subjective cognitive impairment and brain structural networks in Chinese gynaecological cancer survivors compared with age-matched controls: a cross-sectional study. BMC Cancer. (2017) 17:796. doi: 10.1186/s12885-017-3793-4
44. Park, HY, Lee, H, Sohn, J, An, SK, Namkoong, K, and Lee, E. Increased resting-state cerebellar-cortical connectivity in breast cancer survivors with cognitive complaints after chemotherapy. Sci Rep. (2021) 11:12105. doi: 10.1038/s41598-021-91447-1
45. Henneghan, AM, and Kesler, SR. Subjective cancer-related cognitive impairments and salience network connectivity in breast cancer survivors. J Cancer Surviv. (2023) 17:967–73. doi: 10.1007/s11764-022-01307-8
46. Shilling, V, Jenkins, V, and Trapala, IS. The (mis)classification of chemo-fog--methodological inconsistencies in the investigation of cognitive impairment after chemotherapy. Breast Cancer Res Treat. (2006) 95:125–9. doi: 10.1007/s10549-005-9055-1
47. Vardy, JL, Stouten-Kemperman, MM, Pond, G, Booth, CM, Rourke, SB, Dhillon, HM, et al. A mechanistic cohort study evaluating cognitive impairment in women treated for breast cancer. Brain Imaging Behav. (2019) 13:15–26. doi: 10.1007/s11682-017-9728-5
48. Schilder, CM, Seynaeve, C, Linn, SC, Boogerd, W, Gundy, CM, Beex, LV, et al. The impact of different definitions and reference groups on the prevalence of cognitive impairment: a study in postmenopausal breast cancer patients before the start of adjuvant systemic therapy. Psychooncology. (2010) 19:415–22. doi: 10.1002/pon.1595
49. Decker, SL, Schneider, WJ, and Hale, JB. Estimating base rates of impairment in neuropsychological test batteries: a comparison of quantitative models. Arch Clin Neuropsychol. (2012) 27:69–84. doi: 10.1093/arclin/acr088
50. Ouimet, LA, Stewart, A, Collins, B, Schindler, D, and Bielajew, C. Measuring neuropsychological change following breast cancer treatment: an analysis of statistical models. J Clin Exp Neuropsychol. (2009) 31:73–89. doi: 10.1080/13803390801992725
51. Greenland, S. Invited commentary: the need for cognitive science in methodology. Am J Epidemiol. (2017) 186:639–45. doi: 10.1093/aje/kwx259
52. Luijendijk, MJ, Feenstra, HEM, Vermeulen, IE, Murre, JMJ, and Schagen, SB. Binary classification threatens the validity of cognitive impairment detection. Neuropsychology. (2023) 37:344–50. doi: 10.1037/neu0000831
53. Allemann-Su, YY, Vetter, M, Koechlin, H, Paul, SM, Cooper, BA, Oppegaard, K, et al. Pre-surgery demographic, clinical, and symptom characteristics associated with different self-reported cognitive processes in patients with breast Cancer. Cancers (Basel). (2022) 14:3281. doi: 10.3390/cancers14133281
54. Merriman, JD, Sereika, SM, Conley, YP, Koleck, TA, Zhu, Y, Phillips, ML, et al. Exploratory study of associations between DNA repair and oxidative stress gene polymorphisms and cognitive problems reported by postmenopausal women with and without breast Cancer. Biol Res Nurs. (2019) 21:50–60. doi: 10.1177/1099800418799964
55. Morin, RT, and Midlarsky, E. Depressive symptoms and cognitive functioning among older adults with cancer(). Aging Ment Health. (2018) 22:1465–70. doi: 10.1080/13607863.2017.1363868
56. Rolfe, MI, Mengersen, K, Beadle, G, Vearncombe, K, Andrew, B, Johnson, HL, et al. Latent class piecewise linear trajectory modelling for short-term cognition responses after chemotherapy for breast cancer patients. J Appl Stat. (2010) 37:725–38. doi: 10.1080/02664760902729641
57. Tometich, DB, Small, BJ, Carroll, JE, Zhai, W, Luta, G, Zhou, X, et al. Pretreatment Psychoneurological symptoms and their association with longitudinal cognitive function and quality of life in older breast Cancer survivors. J Pain Symptom Manag. (2019) 57:596–606. doi: 10.1016/j.jpainsymman.2018.11.015
58. Westrick, AC, Langa, KM, Eastman, M, Ospina-Romero, M, Mullins, MA, and Kobayashi, LC. Functional aging trajectories of older cancer survivors: a latent growth analysis of the US health and retirement study. J Cancer Surviv. (2022). 17:1499–509. doi: 10.1007/s11764-022-01185-0
59. Agelink van Rentergem, JA, Lee Meeuw Kjoe, PR, Vermeulen, IE, and Schagen, SB. Subgroups of cognitively affected and unaffected breast cancer survivors after chemotherapy: a data-driven approach. J Cancer Surviv. (2023). doi: 10.1007/s11764-022-01310-z
60. Atallah, M, Cooper, B, Munoz, RF, Paul, SM, Anguera, J, Levine, JD, et al. Psychological symptoms and stress are associated with decrements in attentional function in Cancer patients undergoing chemotherapy. Cancer Nurs. (2020) 43:402–10. doi: 10.1097/NCC.0000000000000713
61. Karlson, CW, Sarver, DE, Raiker, JS, Espil, FM, Cox, AD, Elkin, TD, et al. The contribution of neurocognitive functions to academic and psychological outcomes in pediatric cancer: a latent profile analysis. Child Neuropsychol. (2020) 26:881–99. doi: 10.1080/09297049.2020.1734553
62. Peterson, RK, Holingue, C, and Jacobson, LA. Sluggish cognitive tempo profiles in survivors of childhood cancer as compared to children with attention-deficit/hyperactivity disorder. Support Care Cancer. (2022) 30:7553–60. doi: 10.1007/s00520-022-07188-y
63. Sharkey, CM, Mullins, LL, Clawson, AH, Gioia, A, Hawkins, MAW, Chaney, JM, et al. Assessing neuropsychological phenotypes of pediatric brain tumor survivors. Psychooncology. (2021) 30:1366–74. doi: 10.1002/pon.5692
64. Utne, I, Loyland, B, Grov, EK, Rasmussen, HL, Torstveit, AH, Cooper, BA, et al. Distinct attentional function profiles in older adults receiving cancer chemotherapy. Eur J Oncol Nurs. (2018) 36:32–9. doi: 10.1016/j.ejon.2018.08.006
65. Li, Y, Root, JC, Atkinson, TM, and Ahles, TA. Examining the association between patient-reported symptoms of attention and memory dysfunction with objective cognitive performance: a latent regression Rasch model approach. Arch Clin Neuropsychol. (2016) 31:365–77. doi: 10.1093/arclin/acw017
66. Romero-Garcia, R, Owen, M, McDonald, A, Woodberry, E, Assem, M, Coelho, P, et al. Assessment of neuropsychological function in brain tumor treatment: a comparison of traditional neuropsychological assessment with app-based cognitive screening. Acta Neurochir. (2022) 164:2021–34. doi: 10.1007/s00701-022-05162-5
67. Kesler, SR, Petersen, ML, Rao, V, Harrison, RA, and Palesh, O. Functional connectome biotypes of chemotherapy-related cognitive impairment. J Cancer Surviv. (2020) 14:483–93. doi: 10.1007/s11764-020-00863-1
68. Mulholland, MM, Prinsloo, S, Kvale, E, Dula, AN, Palesh, O, and Kesler, SR. Behavioral and biologic characteristics of cancer-related cognitive impairment biotypes. Brain Imaging Behav. (2023) 17:320–8. doi: 10.1007/s11682-023-00774-6
69. Ram, N, and Grimm, KJ. Growth mixture modeling: a method for identifying differences in longitudinal change among unobserved groups. Int J Behav Dev. (2009) 33:565–76. doi: 10.1177/0165025409343765
70. Huang, D, Brecht, ML, Hara, M, and Hser, YI. Influences of a covariate on growth mixture modeling. J Drug Issues. (2010) 40:173–94. doi: 10.1177/002204261004000110
71. Ferguson, SL, G Moore, EW, and Hull, DM. Finding latent groups in observed data: a primer on latent profile analysis in Mplus for applied researchers. Int J Behav Dev. (2019) 44:458–68. doi: 10.1177/0165025419881721
72. Miranda, L, Paul, R, Putz, B, Koutsouleris, N, and Muller-Myhsok, B. Systematic review of functional MRI applications for psychiatric disease subtyping. Front Psych. (2021) 12:665536. doi: 10.3389/fpsyt.2021.665536
73. Niu, R, Du, M, Ren, J, Qing, H, Wang, X, Xu, G, et al. Chemotherapy-induced grey matter abnormalities in cancer survivors: a voxel-wise neuroimaging meta-analysis. Brain Imaging Behav. (2021) 15:2215–27. doi: 10.1007/s11682-020-00402-7
74. McDonald, BC, Conroy, SK, Ahles, TA, West, JD, and Saykin, AJ. Gray matter reduction associated with systemic chemotherapy for breast cancer: a prospective MRI study. Breast Cancer Res Treat. (2010) 123:819–28. doi: 10.1007/s10549-010-1088-4
75. de Ruiter, MB, Reneman, L, Boogerd, W, Veltman, DJ, Caan, M, Douaud, G, et al. Late effects of high-dose adjuvant chemotherapy on white and gray matter in breast cancer survivors: converging results from multimodal magnetic resonance imaging. Hum Brain Mapp. (2012) 33:2971–83. doi: 10.1002/hbm.21422
76. Daniel, E, Deng, F, Patel, SK, Sedrak, MS, Kim, H, Razavi, M, et al. Cortical thinning in chemotherapy-treated older long-term breast cancer survivors. Brain Imaging Behav. (2023) 17:66–76. doi: 10.1007/s11682-022-00743-5
77. Koppelmans, V, de Ruiter, MB, van der Lijn, F, Boogerd, W, Seynaeve, C, van der Lugt, A, et al. Global and focal brain volume in long-term breast cancer survivors exposed to adjuvant chemotherapy. Breast Cancer Res Treat. (2012) 132:1099–106. doi: 10.1007/s10549-011-1888-1
78. Henneghan, A, Rao, V, Harrison, RA, Karuturi, M, Blayney, DW, Palesh, O, et al. Cortical brain age from pre-treatment to post-chemotherapy in patients with breast Cancer. Neurotox Res. (2020) 37:788–99. doi: 10.1007/s12640-019-00158-z
79. Kesler, SR, Rao, V, Ray, WJ, and Rao, A. Alzheimer's disease neuroimaging I. probability of Alzheimer's disease in breast cancer survivors based on gray-matter structural network efficiency. Alzheimers Dement (Amst). (2017) 9:67–75. doi: 10.1016/j.dadm.2017.10.002
80. Brown, MS, Simon, JH, Stemmer, SM, Stears, JC, Scherzinger, A, Cagnoni, PJ, et al. MR and proton spectroscopy of white matter disease induced by high-dose chemotherapy with bone marrow transplant in advanced breast carcinoma. AJNR Am J Neuroradiol. (1995) 16:2013–20.
81. Brown, MS, Stemmer, SM, Simon, JH, Stears, JC, Jones, RB, Cagnoni, PJ, et al. White matter disease induced by high-dose chemotherapy: longitudinal study with MR imaging and proton spectroscopy. AJNR Am J Neuroradiol. (1998) 19:217–21.
82. Ferguson, RJ, McDonald, BC, Saykin, AJ, and Ahles, TA. Brain structure and function differences in monozygotic twins: possible effects of breast cancer chemotherapy. J Clin Oncol. (2007) 25:3866–70. doi: 10.1200/JCO.2007.10.8639
83. Abraham, J, Haut, MW, Moran, MT, Filburn, S, Lemiuex, S, and Kuwabara, H. Adjuvant chemotherapy for breast cancer: effects on cerebral white matter seen in diffusion tensor imaging. Clin Breast Cancer. (2008) 8:88–91. doi: 10.3816/CBC.2008.n.007
84. Deprez, S, Amant, F, Smeets, A, Peeters, R, Leemans, A, Van Hecke, W, et al. Longitudinal assessment of chemotherapy-induced structural changes in cerebral white matter and its correlation with impaired cognitive functioning. J Clin Oncol. (2012) 30:274–81. doi: 10.1200/JCO.2011.36.8571
85. Deprez, S, Amant, F, Yigit, R, Porke, K, Verhoeven, J, Van den Stock, J, et al. Chemotherapy-induced structural changes in cerebral white matter and its correlation with impaired cognitive functioning in breast cancer patients. Hum Brain Mapp. (2011) 32:480–93. doi: 10.1002/hbm.21033
86. Kesler, SR, Watson, CL, and Blayney, DW. Brain network alterations and vulnerability to simulated neurodegeneration in breast cancer. Neurobiol Aging. (2015) 36:2429–42. doi: 10.1016/j.neurobiolaging.2015.04.015
87. Manchon, JF, Dabaghian, Y, Uzor, NE, Kesler, SR, Wefel, JS, and Tsvetkov, AS. Levetiracetam mitigates doxorubicin-induced DNA and synaptic damage in neurons. Sci Rep. (2016) 6:25705. doi: 10.1038/srep25705
88. Chen, VC, Lin, KY, Tsai, YH, and Weng, JC. Connectome analysis of brain functional network alterations in breast cancer survivors with and without chemotherapy. PLoS One. (2020) 15:e0232548. doi: 10.1371/journal.pone.0232548
89. Kesler, SR, Bennett, FC, Mahaffey, ML, and Spiegel, D. Regional brain activation during verbal declarative memory in metastatic breast cancer. Clin Cancer Res. (2009) 15:6665–73. doi: 10.1158/1078-0432.CCR-09-1227
90. McDonald, BC, Conroy, SK, Ahles, TA, West, JD, and Saykin, AJ. Alterations in brain activation during working memory processing associated with breast cancer and treatment: a prospective functional magnetic resonance imaging study. J Clin Oncol. (2012) 30:2500–8. doi: 10.1200/JCO.2011.38.5674
91. Menning, S, de Ruiter, MB, Veltman, DJ, Boogerd, W, Oldenburg, HS, Reneman, L, et al. Changes in brain activation in breast cancer patients depend on cognitive domain and treatment type. PLoS One. (2017) 12:e0171724. doi: 10.1371/journal.pone.0171724
92. Pomykala, KL, de Ruiter, MB, Deprez, S, McDonald, BC, and Silverman, DH. Integrating imaging findings in evaluating the post-chemotherapy brain. Brain Imaging Behav. (2013) 7:436–52. doi: 10.1007/s11682-013-9239-y
93. Saward, JB, Ellis, EG, Cobden, AL, and Caeyenberghs, K. Mapping cognitive deficits in cancer patients after chemotherapy: An activation likelihood estimation meta-analysis of task-related fMRI studies. Brain Imaging Behav. (2022) 16:2320–34. doi: 10.1007/s11682-022-00655-4
94. Zheng, F, Cao, P, Zhou, J, Li, C, and Norris, J. Study on neurologic and cognitive dysfunction in breast Cancer patients undergoing chemotherapy with RS fMRI imaging. World Neurosurg. (2020) 149:388–96. doi: 10.1016/j.wneu.2020.10.088
95. Chen, BT, Jin, T, Patel, SK, Ye, N, Ma, H, Wong, CW, et al. Intrinsic brain activity changes associated with adjuvant chemotherapy in older women with breast cancer: a pilot longitudinal study. Breast Cancer Res Treat. (2019) 176:181–9. doi: 10.1007/s10549-019-05230-y
96. Ganz, PA, Kwan, L, Castellon, SA, Oppenheim, A, Bower, JE, Silverman, DH, et al. Cognitive complaints after breast cancer treatments: examining the relationship with neuropsychological test performance. J Natl Cancer Inst. (2013) 105:791–801. doi: 10.1093/jnci/djt073
97. Terry, DP, Sabatinelli, D, Puente, AN, Lazar, NA, and Miller, LS. A meta-analysis of fMRI activation differences during episodic memory in Alzheimer's disease and mild cognitive impairment. J Neuroimaging. (2015) 25:849–60. doi: 10.1111/jon.12266
98. Li, HJ, Hou, XH, Liu, HH, Yue, CL, He, Y, and Zuo, XN. Toward systems neuroscience in mild cognitive impairment and Alzheimer's disease: a meta-analysis of 75 fMRI studies. Hum Brain Mapp. (2015) 36:1217–32. doi: 10.1002/hbm.22689
99. Yeung, MK, and Chan, AS. A systematic review of the application of functional near-infrared spectroscopy to the study of cerebral hemodynamics in healthy aging. Neuropsychol Rev. (2021) 31:139–66. doi: 10.1007/s11065-020-09455-3
100. Cheng, H, Li, W, Gong, L, Xuan, H, Huang, Z, Zhao, H, et al. Altered resting-state hippocampal functional networks associated with chemotherapy-induced prospective memory impairment in breast cancer survivors. Sci Rep. (2017) 7:45135. doi: 10.1038/srep45135
101. Xuan, H, Gan, C, Li, W, Huang, Z, Wang, L, Jia, Q, et al. Altered network efficiency of functional brain networks in patients with breast cancer after chemotherapy. Oncotarget. (2017) 8:105648–61. doi: 10.18632/oncotarget.22358
102. Dumas, JA, Makarewicz, J, Schaubhut, GJ, Devins, R, Albert, K, Dittus, K, et al. Chemotherapy altered brain functional connectivity in women with breast cancer: a pilot study. Brain Imaging Behav. (2013) 7:524–32. doi: 10.1007/s11682-013-9244-1
103. Bruno, J, Hosseini, SM, and Kesler, S. Altered resting state functional brain network topology in chemotherapy-treated breast cancer survivors. Neurobiol Dis. (2012) 48:329–38. doi: 10.1016/j.nbd.2012.07.009
104. Kesler, SR, and Blayney, DW. Neurotoxic effects of anthracycline- vs nonanthracycline-based chemotherapy on cognition in breast Cancer survivors. JAMA Oncol. (2016) 2:185–92. doi: 10.1001/jamaoncol.2015.4333
105. Zeng, Y, Cheng, ASK, Song, T, Sheng, X, Cheng, H, Qiu, Y, et al. Changes in functional brain networks and neurocognitive function in Chinese gynecological cancer patients after chemotherapy: a prospective longitudinal study. BMC Cancer. (2019) 19:386. doi: 10.1186/s12885-019-5576-6
106. Miao, H, Chen, X, Yan, Y, He, X, Hu, S, Kong, J, et al. Functional connectivity change of brain default mode network in breast cancer patients after chemotherapy. Neuroradiology. (2016) 58:921–8. doi: 10.1007/s00234-016-1708-8
107. Insel, T, Cuthbert, B, Garvey, M, Heinssen, R, Pine, DS, Quinn, K, et al. Research domain criteria (RDoC): toward a new classification framework for research on mental disorders. Am J Psychiatry. (2010) 167:748–51. doi: 10.1176/appi.ajp.2010.09091379
108. Tamminga, CA, Ivleva, EI, Keshavan, MS, Pearlson, GD, Clementz, BA, Witte, B, et al. Clinical phenotypes of psychosis in the bipolar-schizophrenia network on intermediate phenotypes (B-SNIP). Am J Psychiatry. (2013) 170:1263–74. doi: 10.1176/appi.ajp.2013.12101339
109. Morris, SE, and Cuthbert, BN. Research domain criteria: cognitive systems, neural circuits, and dimensions of behavior. Dialogues Clin Neurosci. (2012) 14:29–37. doi: 10.31887/DCNS.2012.14.1/smorris
110. Keshavan, MS, Morris, DW, Sweeney, JA, Pearlson, G, Thaker, G, Seidman, LJ, et al. A dimensional approach to the psychosis spectrum between bipolar disorder and schizophrenia: the Schizo-bipolar scale. Schizophr Res. (2011) 133:250–4. doi: 10.1016/j.schres.2011.09.005
111. Keshavan, MS, Clementz, BA, Pearlson, GD, Sweeney, JA, and Tamminga, CA. Reimagining psychoses: an agnostic approach to diagnosis. Schizophr Res. (2013) 146:10–6. doi: 10.1016/j.schres.2013.02.022
112. Kesler, SR. Default mode network as a potential biomarker of chemotherapy-related brain injury. Neurobiol Aging. (2014) 35:S11–9. doi: 10.1016/j.neurobiolaging.2014.03.036
114. Buckner, RL, Krienen, FM, and Yeo, BT. Opportunities and limitations of intrinsic functional connectivity MRI. Nat Neurosci. (2013) 16:832–7. doi: 10.1038/nn.3423
115. Shirer, WR, Ryali, S, Rykhlevskaia, E, Menon, V, and Greicius, MD. Decoding subject-driven cognitive states with whole-brain connectivity patterns. Cereb Cortex. (2012) 22:158–65. doi: 10.1093/cercor/bhr099
116. Richiardi, J, Altmann, A, Milazzo, AC, Chang, C, Chakravarty, MM, Banaschewski, T, et al. Correlated gene expression supports synchronous activity in brain networks. Science. (2015) 348:1241–4. doi: 10.1126/science.1255905
117. Fox, MD, and Greicius, M. Clinical applications of resting state functional connectivity. Front Syst Neurosci. (2010) 4:19. doi: 10.3389/fnsys.2010.00019
118. Drysdale, AT, Grosenick, L, Downar, J, Dunlop, K, Mansouri, F, Meng, Y, et al. Resting-state connectivity biomarkers define neurophysiological subtypes of depression. Nat Med. (2017) 23:28–38. doi: 10.1038/nm.4246
119. Ivleva, EI, Clementz, BA, Dutcher, AM, Arnold, SJM, Jeon-Slaughter, H, Aslan, S, et al. Brain structure biomarkers in the psychosis biotypes: findings from the bipolar-schizophrenia network for intermediate phenotypes. Biol Psychiatry. (2017) 82:26–39. doi: 10.1016/j.biopsych.2016.08.030
120. Meda, SA, Clementz, BA, Sweeney, JA, Keshavan, MS, Tamminga, CA, Ivleva, EI, et al. Examining functional resting-state connectivity in psychosis and its subgroups in the bipolar-schizophrenia network on intermediate phenotypes cohort. Biol Psychiatry Cogn Neurosci Neuroimaging. (2016) 1:488–97. doi: 10.1016/j.bpsc.2016.07.001
121. Barth, B, Mayer-Carius, K, Strehl, U, Kelava, A, Haussinger, FB, Fallgatter, AJ, et al. Identification of neurophysiological biotypes in attention deficit hyperactivity disorder. Psychiatry Clin Neurosci. (2018) 72:836–48. doi: 10.1111/pcn.12773
122. Clementz, BA, Sweeney, JA, Hamm, JP, Ivleva, EI, Ethridge, LE, Pearlson, GD, et al. Identification of distinct psychosis biotypes using brain-based biomarkers. Am J Psychiatry. (2016) 173:373–84. doi: 10.1176/appi.ajp.2015.14091200
123. Wang, L, Cheng, W, Rolls, ET, Dai, F, Gong, W, Du, J, et al. Association of specific biotypes in patients with Parkinson disease and disease progression. Neurology. (2020) 95:e1445–60. doi: 10.1212/WNL.0000000000010498
124. Pontillo, G, Penna, S, Cocozza, S, Quarantelli, M, Gravina, M, Lanzillo, R, et al. Stratification of multiple sclerosis patients using unsupervised machine learning: a single-visit MRI-driven approach. Eur Radiol. (2022) 32:5382–91. doi: 10.1007/s00330-022-08610-z
125. Ahles, TA, Saykin, AJ, McDonald, BC, Li, Y, Furstenberg, CT, Hanscom, BS, et al. Longitudinal assessment of cognitive changes associated with adjuvant treatment for breast cancer: impact of age and cognitive reserve. J Clin Oncol. (2010) 28:4434–40. doi: 10.1200/JCO.2009.27.0827
126. Hosseini, SM, and Kesler, SR. Multivariate pattern analysis of FMRI in breast cancer survivors and healthy women. J Int Neuropsychol Soc. (2014) 20:391–401. doi: 10.1017/S1355617713001173
127. Stouten-Kemperman, MM, de Ruiter, MB, Koppelmans, V, Boogerd, W, Reneman, L, and Schagen, SB. Neurotoxicity in breast cancer survivors >/=10 years post-treatment is dependent on treatment type. Brain Imaging Behav. (2015) 9:275–84. doi: 10.1007/s11682-014-9305-0
128. Kaiser, J, Bledowski, C, and Dietrich, J. Neural correlates of chemotherapy-related cognitive impairment. Cortex. (2014) 54:33–50. doi: 10.1016/j.cortex.2014.01.010
129. Simo, M, Rifa-Ros, X, Rodriguez-Fornells, A, and Bruna, J. Chemobrain: a systematic review of structural and functional neuroimaging studies. Neurosci Biobehav Rev. (2013) 37:1311–21. doi: 10.1016/j.neubiorev.2013.04.015
130. Thompson, DG, Kesler, SR, Sudheimer, K, Mehta, KM, Thompson, LW, Marquett, RM, et al. FMRI activation during executive function predicts response to cognitive behavioral therapy in older, depressed adults. Am J Geriatr Psychiatry. (2015) 23:13–22. doi: 10.1016/j.jagp.2014.02.001
131. Moradi, E, Pepe, A, Gaser, C, Huttunen, H, and Tohka, J, Alzheimer's Disease Neuroimaging I. Machine learning framework for early MRI-based Alzheimer's conversion prediction in MCI subjects. NeuroImage. (2015) 104:398–412. doi: 10.1016/j.neuroimage.2014.10.002
132. Strangman, GE, O'Neil-Pirozzi, TM, Supelana, C, Goldstein, R, Katz, DI, and Glenn, MB. Regional brain morphometry predicts memory rehabilitation outcome after traumatic brain injury. Front Hum Neurosci. (2010) 4:182. doi: 10.3389/fnhum.2010.00182
133. Zhang, D, and Shen, D, Alzheimer's Disease Neuroimaging I. Multi-modal multi-task learning for joint prediction of multiple regression and classification variables in Alzheimer's disease. NeuroImage. (2012) 59:895–907. doi: 10.1016/j.neuroimage.2011.09.069
134. Osipowicz, K, Sperling, MR, Sharan, AD, and Tracy, JI. Functional MRI, resting state fMRI, and DTI for predicting verbal fluency outcome following resective surgery for temporal lobe epilepsy. J Neurosurg. (2016) 124:929–37. doi: 10.3171/2014.9.JNS131422
135. Jordan, MI, and Mitchell, TM. Machine learning: trends, perspectives, and prospects. Science. (2015) 349:255–60. doi: 10.1126/science.aaa8415
136. Strobl, C, Boulesteix, AL, Zeileis, A, and Hothorn, T. Bias in random forest variable importance measures: illustrations, sources and a solution. BMC Bioinformatics. (2007) 8:25. doi: 10.1186/1471-2105-8-25
137. Hoeft, F, McCandliss, BD, Black, JM, Gantman, A, Zakerani, N, Hulme, C, et al. Neural systems predicting long-term outcome in dyslexia. Proc Natl Acad Sci U S A. (2011) 108:361–6. doi: 10.1073/pnas.1008950108
138. Heiss, WD, and Kidwell, CS. Imaging for prediction of functional outcome and assessment of recovery in ischemic stroke. Stroke. (2014) 45:1195–201. doi: 10.1161/STROKEAHA.113.003611
139. Jollans, L, and Whelan, R. The clinical added value of imaging: a perspective from outcome prediction. Biol Psychiatry Cogn Neurosci Neuroimaging. (2016) 1:423–32. doi: 10.1016/j.bpsc.2016.04.005
140. Kesler, SR, Adams, M, Packer, M, Rao, V, Henneghan, AM, Blayney, DW, et al. Disrupted brain network functional dynamics and hyper-correlation of structural and functional connectome topology in patients with breast cancer prior to treatment. Brain Behav. (2017) 7:e00643. doi: 10.1002/brb3.643
141. Patel, SK, Wong, AL, Wong, FL, Breen, EC, Hurria, A, Smith, M, et al. Inflammatory biomarkers, comorbidity, and Neurocognition in women with newly diagnosed breast Cancer. J Natl Cancer Inst. (2015) 107:1–7. doi: 10.1093/jnci/djv131
142. Menning, S, de Ruiter, MB, Veltman, DJ, Koppelmans, V, Kirschbaum, C, Boogerd, W, et al. Multimodal MRI and cognitive function in patients with breast cancer prior to adjuvant treatment--the role of fatigue. Neuroimage Clin. (2015) 7:547–54. doi: 10.1016/j.nicl.2015.02.005
143. Scherling, C, Collins, B, Mackenzie, J, Bielajew, C, and Smith, A. Pre-chemotherapy differences in visuospatial working memory in breast cancer patients compared to controls: an FMRI study. Front Hum Neurosci. (2011) 5:122. doi: 10.3389/fnhum.2011.00122
144. Scherling, C, Collins, B, Mackenzie, J, Bielajew, C, and Smith, A. Prechemotherapy differences in response inhibition in breast cancer patients compared to controls: a functional magnetic resonance imaging study. J Clin Exp Neuropsychol. (2012) 34:543–60. doi: 10.1080/13803395.2012.666227
145. Scherling, C, Collins, B, MacKenzie, J, Lepage, C, Bielajew, C, and Smith, A. Structural brain differences in breast cancer patients compared to matched controls prior to chemotherapy. Int J Biol. (2012) 4:3–25. doi: 10.5539/ijb.v4n2p3
146. Yao, C, Rich, JB, Tannock, IF, Seruga, B, Tirona, K, and Bernstein, LJ. Pretreatment differences in Intraindividual variability in reaction time between women diagnosed with breast Cancer and healthy controls. J Int Neuropsychol Soc. (2016) 22:530–9. doi: 10.1017/S1355617716000126
147. Henneghan, AM, Gibbons, C, Harrison, RA, Edwards, ML, Rao, V, Blayney, DW, et al. Predicting patient reported outcomes of cognitive function using connectome-based predictive modeling in breast Cancer. Brain Topogr. (2020) 33:135–42. doi: 10.1007/s10548-019-00746-4
148. Kesler, SR, Rao, A, Blayney, DW, Oakley-Girvan, IA, Karuturi, M, and Palesh, O. Predicting long-term cognitive outcome following breast Cancer with pre-treatment resting state fMRI and random Forest machine learning. Front Hum Neurosci. (2017) 11:555. doi: 10.3389/fnhum.2017.00555
149. de Ruiter, MB, Reneman, L, Kieffer, JM, Oldenburg, HSA, and Schagen, SB. Brain white matter microstructure as a risk factor for cognitive decline after chemotherapy for breast Cancer. J Clin Oncol. (2021) 39:3908–17. doi: 10.1200/JCO.21.00627
150. Treanor, CJ, McMenamin, UC, O'Neill, RF, Cardwell, CR, Clarke, MJ, Cantwell, M, et al. Non-pharmacological interventions for cognitive impairment due to systemic cancer treatment. Cochrane Database Syst Rev. (2016) 2016:CD011325. doi: 10.1002/14651858.CD011325.pub2
151. Campbell, KL, Zadravec, K, Bland, KA, Chesley, E, Wolf, F, and Janelsins, MC. The effect of exercise on Cancer-related cognitive impairment and applications for physical therapy: systematic review of randomized controlled trials. Phys Ther. (2020) 100:523–42. doi: 10.1093/ptj/pzz090
152. Yan, X, Wei, S, and Liu, Q. Effect of cognitive training on patients with breast cancer reporting cognitive changes: a systematic review and meta-analysis. BMJ Open. (2023) 13:e058088. doi: 10.1136/bmjopen-2021-058088
153. Fernandes, HA, Richard, NM, and Edelstein, K. Cognitive rehabilitation for cancer-related cognitive dysfunction: a systematic review. Support Care Cancer. (2019) 27:3253–79. doi: 10.1007/s00520-019-04866-2
154. Luctkar-Flude, M, and Groll, D. A systematic review of the safety and effect of neurofeedback on fatigue and cognition. Integr Cancer Ther. (2015) 14:318–40. doi: 10.1177/1534735415572886
155. Mar Fan, HG, Clemons, M, Xu, W, Chemerynsky, I, Breunis, H, Braganza, S, et al. A randomised, placebo-controlled, double-blind trial of the effects of d-methylphenidate on fatigue and cognitive dysfunction in women undergoing adjuvant chemotherapy for breast cancer. Support Care Cancer. (2008) 16:577–83. doi: 10.1007/s00520-007-0341-9
156. Kohli, S, Fisher, SG, Tra, Y, Adams, MJ, Mapstone, ME, Wesnes, KA, et al. The effect of modafinil on cognitive function in breast cancer survivors. Cancer. (2009) 115:2605–16. doi: 10.1002/cncr.24287
157. Vega, JN, Albert, KM, Mayer, IA, Taylor, WD, and Newhouse, PA. Nicotinic treatment of post-chemotherapy subjective cognitive impairment: a pilot study. J Cancer Surviv. (2019) 13:673–86. doi: 10.1007/s11764-019-00786-6
158. O'Shaughnessy, JA, Vukelja, SJ, Holmes, FA, Savin, M, Jones, M, Royall, D, et al. Feasibility of quantifying the effects of epoetin alfa therapy on cognitive function in women with breast cancer undergoing adjuvant or neoadjuvant chemotherapy. Clin Breast Cancer. (2005) 5:439–46. doi: 10.3816/CBC.2005.n.002
159. Lawrence, JA, Griffin, L, Balcueva, EP, Groteluschen, DL, Samuel, TA, Lesser, GJ, et al. A study of donepezil in female breast cancer survivors with self-reported cognitive dysfunction 1 to 5 years following adjuvant chemotherapy. J Cancer Surviv. (2016) 10:176–84. doi: 10.1007/s11764-015-0463-x
160. Nguyen, LD, and Ehrlich, BE. Cellular mechanisms and treatments for chemobrain: insight from aging and neurodegenerative diseases. EMBO Mol Med. (2020) 12:e12075. doi: 10.15252/emmm.202012075
161. Jiang, ZG, Winocur, G, Wojtowicz, JM, Shevtsova, O, Fuller, S, and Ghanbari, HA. PAN-811 prevents chemotherapy-induced cognitive impairment and preserves neurogenesis in the hippocampus of adult rats. PLoS One. (2018) 13:e0191866. doi: 10.1371/journal.pone.0191866
162. Chiu, GS, Boukelmoune, N, Chiang, ACA, Peng, B, Rao, V, Kingsley, C, et al. Nasal administration of mesenchymal stem cells restores cisplatin-induced cognitive impairment and brain damage in mice. Oncotarget. (2018) 9:35581–97. doi: 10.18632/oncotarget.26272
163. El-Derany, MO, and Noureldein, MH. Bone marrow mesenchymal stem cells and their derived exosomes resolve doxorubicin-induced chemobrain: critical role of their miRNA cargo. Stem Cell Res Ther. (2021) 12:322. doi: 10.1186/s13287-021-02384-9
164. Sherif, IO, Al-Shaalan, NH, and Sabry, D. Neuroprotective potential of bone marrow-derived mesenchymal stem cells following chemotherapy. Biomedicine. (2021) 9:750. doi: 10.3390/biomedicines9070750
165. Alexander, JF, Mahalingam, R, Seua, AV, Wu, S, Arroyo, LD, Horbelt, T, et al. Targeting the meningeal compartment to resolve Chemobrain and neuropathy via nasal delivery of functionalized mitochondria. Adv Healthc Mater. (2022) 11:e2102153. doi: 10.1002/adhm.202102153
166. Ma, J, Goodwani, S, Acton, PJ, Buggia-Prevot, V, Kesler, SR, Jamal, I, et al. Inhibition of dual leucine zipper kinase prevents chemotherapy-induced peripheral neuropathy and cognitive impairments. Pain. (2021) 162:2599–612. doi: 10.1097/j.pain.0000000000002256
167. McAlpin, BR, Mahalingam, R, Singh, AK, Dharmaraj, S, Chrisikos, TT, Boukelmoune, N, et al. HDAC6 inhibition reverses long-term doxorubicin-induced cognitive dysfunction by restoring microglia homeostasis and synaptic integrity. Theranostics. (2022) 12:603–19. doi: 10.7150/thno.67410
168. Sofis, MJ, Jarmolowicz, DP, Kaplan, SV, Gehringer, RC, Lemley, SM, Garg, G, et al. KU32 prevents 5-fluorouracil induced cognitive impairment. Behav Brain Res. (2017) 329:186–90. doi: 10.1016/j.bbr.2017.03.042
169. El-Agamy, SE, Abdel-Aziz, AK, Wahdan, S, Esmat, A, and Azab, SS. Astaxanthin ameliorates doxorubicin-induced cognitive impairment (Chemobrain) in experimental rat model: impact on oxidative, inflammatory, and apoptotic machineries. Mol Neurobiol. (2018) 55:5727–40. doi: 10.1007/s12035-017-0797-7
170. Zeng, Y, Dong, J, Huang, M, Zhang, JE, Zhang, X, Xie, M, et al. Nonpharmacological interventions for cancer-related cognitive impairment in adult cancer patients: a network meta-analysis. Int J Nurs Stud. (2020) 104:103514. doi: 10.1016/j.ijnurstu.2019.103514
171. Alvarez, J, Meyer, FL, Granoff, DL, and Lundy, A. The effect of EEG biofeedback on reducing postcancer cognitive impairment. Integr Cancer Ther. (2013) 12:475–87. doi: 10.1177/1534735413477192
172. Knotkova, H, Malamud, SC, and Cruciani, RA. Transcranial direct current stimulation (TDCS) improved cognitive outcomes in a cancer survivor with chemotherapy-induced cognitive difficulties. Brain Stimul. (2014) 7:767–8. doi: 10.1016/j.brs.2014.05.007
173. Oldacres, L, Hegarty, J, O'Regan, P, Murphy-Coakley, NM, and Saab, MM. Interventions promoting cognitive function in patients experiencing cancer related cognitive impairment: a systematic review. Psychooncology. (2023) 32:214–28. doi: 10.1002/pon.6073
174. Binarelli, G, Joly, F, Tron, L, Lefevre Arbogast, S, and Lange, M. Management of cancer-related cognitive impairment: a systematic review of computerized cognitive stimulation and computerized physical activity. Cancers (Basel). (2021) 13:5161. doi: 10.3390/cancers13205161
175. Cifu, G, Power, MC, Shomstein, S, and Arem, H. Mindfulness-based interventions and cognitive function among breast cancer survivors: a systematic review. BMC Cancer. (2018) 18:1163. doi: 10.1186/s12885-018-5065-3
176. Shahid, M, and Kim, J. Exercise may affect metabolism in cancer-related cognitive impairment. Meta. (2020) 10:377. doi: 10.3390/metabo10090377
177. Park, JH, Jung, SJ, Lee, LJ, Rhu, J, and Bae, SH. Impact of nonpharmacological interventions on cognitive impairment in women with breast cancer: a systematic review and meta-analysis. Asia Pac J Oncol Nurs. (2023) 10:100212. doi: 10.1016/j.apjon.2023.100212
178. Campbell, KL, Kam, JWY, Neil-Sztramko, SE, Liu Ambrose, T, Handy, TC, Lim, HJ, et al. Effect of aerobic exercise on cancer-associated cognitive impairment: a proof-of-concept RCT. Psychooncology. (2018) 27:53–60. doi: 10.1002/pon.4370
179. Van der Gucht, K, Ahmadoun, S, Melis, M, de Cloe, E, Sleurs, C, Radwan, A, et al. Effects of a mindfulness-based intervention on cancer-related cognitive impairment: results of a randomized controlled functional magnetic resonance imaging pilot study. Cancer. (2020) 126:4246–55. doi: 10.1002/cncr.33074
180. Vega, JN, Dumas, J, and Newhouse, PA. Cognitive effects of chemotherapy and Cancer-related treatments in older adults. Am J Geriatr Psychiatry. (2017) 25:1415–26. doi: 10.1016/j.jagp.2017.04.001
181. Winocur, G, Wojtowicz, JM, Merkley, CM, and Tannock, IF. Environmental enrichment protects against cognitive impairment following chemotherapy in an animal model. Behav Neurosci. (2016) 130:428–36. doi: 10.1037/bne0000155
182. Seigers, R, Schagen, SB, Van Tellingen, O, and Dietrich, J. Chemotherapy-related cognitive dysfunction: current animal studies and future directions. Brain Imaging Behav. (2013) 7:453–9. doi: 10.1007/s11682-013-9250-3
183. Hennings, M, and Fremouw, T. The effect of doxorubicin or cyclophosphamide treatment on auditory brainstem response in mice. Exp Brain Res. (2022) 240:2907–21. doi: 10.1007/s00221-022-06463-y
184. Squillace, S, Niehoff, ML, Doyle, TM, Green, M, Esposito, E, Cuzzocrea, S, et al. Sphingosine-1-phosphate receptor 1 activation in the central nervous system drives cisplatin-induced cognitive impairment. J Clin Invest. (2022) 132:e157738. doi: 10.1172/JCI157738
185. Demby, TC, Rodriguez, O, McCarthy, CW, Lee, YC, Albanese, C, Mandelblatt, J, et al. A mouse model of chemotherapy-related cognitive impairments integrating the risk factors of aging and APOE4 genotype. Behav Brain Res. (2020) 384:112534. doi: 10.1016/j.bbr.2020.112534
186. Demos-Davies, K, Lawrence, J, Rogich, A, Lind, E, and Seelig, D. Cancer treatment induces neuroinflammation and behavioral deficits in mice. Front Behav Neurosci. (2022) 16:1067298. doi: 10.3389/fnbeh.2022.1067298
187. Ng, CAS, Biran, LP, Galvano, E, Mandelblatt, J, Vicini, S, and Rebeck, GW. Chemotherapy promotes astrocytic response to Abeta deposition, but not Abeta levels, in a mouse model of amyloid and APOE. Neurobiol Dis. (2022) 175:105915. doi: 10.1016/j.nbd.2022.105915
188. Usmani, MT, Krattli, RP Jr, El-Khatib, SM, Le, ACD, Smith, SM, Baulch, JE, et al. BDNF augmentation using Riluzole reverses doxorubicin-induced decline in cognitive function and neurogenesis. Neurotherapeutics. (2023) 20:838–52. doi: 10.1007/s13311-022-01339-z
189. Gibson, EM, and Monje, M. Emerging mechanistic underpinnings and therapeutic targets for chemotherapy-related cognitive impairment. Curr Opin Oncol. (2019) 31:531–9. doi: 10.1097/CCO.0000000000000578
190. Walter, M, Alizadeh, S, Jamalabadi, H, Lueken, U, Dannlowski, U, Walter, H, et al. Translational machine learning for psychiatric neuroimaging. Prog Neuro-Psychopharmacol Biol Psychiatry. (2019) 91:113–21. doi: 10.1016/j.pnpbp.2018.09.014
191. Logothetis, NK, and Pfeuffer, J. On the nature of the BOLD fMRI contrast mechanism. Magn Reson Imaging. (2004) 22:1517–31. doi: 10.1016/j.mri.2004.10.018
192. Pinti, P, Tachtsidis, I, Hamilton, A, Hirsch, J, Aichelburg, C, Gilbert, S, et al. The present and future use of functional near-infrared spectroscopy (fNIRS) for cognitive neuroscience. Ann N Y Acad Sci. (2020) 1464:5–29. doi: 10.1111/nyas.13948
193. Scholkmann, F, Kleiser, S, Metz, AJ, Zimmermann, R, Mata Pavia, J, Wolf, U, et al. A review on continuous wave functional near-infrared spectroscopy and imaging instrumentation and methodology. NeuroImage. (2014) 85:6–27. doi: 10.1016/j.neuroimage.2013.05.004
194. Scarapicchia, V, Brown, C, Mayo, C, and Gawryluk, JR. Functional magnetic resonance imaging and functional near-infrared spectroscopy: insights from combined recording studies. Front Hum Neurosci. (2017) 11:419. doi: 10.3389/fnhum.2017.00419
195. Leh, SE, Petrides, M, and Strafella, AP. The neural circuitry of executive functions in healthy subjects and Parkinson's disease. Neuropsychopharmacology. (2010) 35:70–85. doi: 10.1038/npp.2009.88
196. Bast, T. The hippocampal learning-behavior translation and the functional significance of hippocampal dysfunction in schizophrenia. Curr Opin Neurobiol. (2011) 21:492–501. doi: 10.1016/j.conb.2011.01.003
197. Squire, LR, van der Horst, AS, McDuff, SG, Frascino, JC, Hopkins, RO, and Mauldin, KN. Role of the hippocampus in remembering the past and imagining the future. Proc Natl Acad Sci U S A. (2010) 107:19044–8. doi: 10.1073/pnas.1014391107
198. de Ruiter, MB, Reneman, L, Boogerd, W, Veltman, DJ, van Dam, FS, Nederveen, AJ, et al. Cerebral hyporesponsiveness and cognitive impairment 10 years after chemotherapy for breast cancer. Hum Brain Mapp. (2011) 32:1206–19. doi: 10.1002/hbm.21102
199. Inagaki, M, Yoshikawa, E, Matsuoka, Y, Sugawara, Y, Nakano, T, Akechi, T, et al. Smaller regional volumes of brain gray and white matter demonstrated in breast cancer survivors exposed to adjuvant chemotherapy. Cancer. (2007) 109:146–56. doi: 10.1002/cncr.22368
200. Harrison, RA, Rao, V, and Kesler, SR. The association of genetic polymorphisms with neuroconnectivity in breast cancer patients. Sci Rep. (2021) 11:6169. doi: 10.1038/s41598-021-85768-4
201. Kesler, SR, Wefel, JS, Hosseini, SM, Cheung, M, Watson, CL, and Hoeft, F. Default mode network connectivity distinguishes chemotherapy-treated breast cancer survivors from controls. Proc Natl Acad Sci U S A. (2013) 110:11600–5. doi: 10.1073/pnas.1214551110
202. Stilley, CS, Bender, CM, Dunbar-Jacob, J, Sereika, S, and Ryan, CM. The impact of cognitive function on medication management: three studies. Health Psychol. (2010) 29:50–5. doi: 10.1037/a0016940
203. Reid-Arndt, SA, Yee, A, Perry, MC, and Hsieh, C. Cognitive and psychological factors associated with early posttreatment functional outcomes in breast cancer survivors. J Psychosoc Oncol. (2009) 27:415–34. doi: 10.1080/07347330903183117
204. Alfano, CM, Peng, J, Andridge, RR, Lindgren, ME, Povoski, SP, Lipari, AM, et al. Inflammatory cytokines and comorbidity development in breast Cancer survivors versus noncancer controls: evidence for accelerated aging? J Clin Oncol. (2017) 35:149–56. doi: 10.1200/JCO.2016.67.1883
205. Sanoff, HK, Deal, AM, Krishnamurthy, J, Torrice, C, Dillon, P, Sorrentino, J, et al. Effect of cytotoxic chemotherapy on markers of molecular age in patients with breast cancer. J Natl Cancer Inst. (2014) 106:dju057. doi: 10.1093/jnci/dju057
206. Ahles, TA. Brain vulnerability to chemotherapy toxicities. Psychooncology. (2012) 21:1141–8. doi: 10.1002/pon.3196
207. Lloret, A, Fuchsberger, T, Giraldo, E, and Vina, J. Molecular mechanisms linking amyloid beta toxicity and tau hyperphosphorylation in Alzheimer′s disease. Free Radic Biol Med. (2015) 83:186–91. doi: 10.1016/j.freeradbiomed.2015.02.028
208. Andreasson, U, Blennow, K, and Zetterberg, H. Update on ultrasensitive technologies to facilitate research on blood biomarkers for central nervous system disorders. Alzheimers Dement (Amst). (2016) 3:98–102. doi: 10.1016/j.dadm.2016.05.005
209. Rissin, DM, Kan, CW, Campbell, TG, Howes, SC, Fournier, DR, Song, L, et al. Single-molecule enzyme-linked immunosorbent assay detects serum proteins at subfemtomolar concentrations. Nat Biotech. (2010) 28:595–9. doi: 10.1038/nbt.1641
210. Song, L, Lachno, DR, Hanlon, D, Shepro, A, Jeromin, A, Gemani, D, et al. A digital enzyme-linked immunosorbent assay for ultrasensitive measurement of amyloid-beta 1-42 peptide in human plasma with utility for studies of Alzheimer's disease therapeutics. Alzheimers Res Ther. (2016) 8:58. doi: 10.1186/s13195-016-0225-7
211. Mattsson, N, Zetterberg, H, Janelidze, S, Insel, PS, Andreasson, U, Stomrud, E, et al. Plasma tau in Alzheimer disease. Neurology. (2016) 87:1827–35. doi: 10.1212/WNL.0000000000003246
212. Zetterberg, H, Wilson, D, Andreasson, U, Minthon, L, Blennow, K, Randall, J, et al. Plasma tau levels in Alzheimer's disease. Alzheimers Res Ther. (2013) 5:9. doi: 10.1186/alzrt163
213. Janelidze, S, Stomrud, E, Palmqvist, S, Zetterberg, H, van Westen, D, Jeromin, A, et al. Plasma beta-amyloid in Alzheimer's disease and vascular disease. Sci Rep. (2016) 6:26801. doi: 10.1038/srep26801
214. Dage, JL, Wennberg, AMV, Airey, DC, Hagen, CE, Knopman, DS, Machulda, MM, et al. Levels of tau protein in plasma are associated with neurodegeneration and cognitive function in a population-based elderly cohort. Alzheimers Dement. (2016) 12:1226–34. doi: 10.1016/j.jalz.2016.06.001
215. Bogoslovsky, T, Wilson, D, Chen, Y, Hanlon, D, Gill, J, Jeromin, A, et al. Increases of plasma levels of glial fibrillary acidic protein, tau, and amyloid beta up to 90 days after traumatic brain injury. J Neurotrauma. (2017) 34:66–73. doi: 10.1089/neu.2015.4333
216. Olivera, A, Lejbman, N, Jeromin, A, French, LM, Kim, HS, Cashion, A, et al. Peripheral Total tau in military personnel who sustain traumatic brain injuries during deployment. JAMA Neurol. (2015) 72:1109–16. doi: 10.1001/jamaneurol.2015.1383
217. Henneghan, A, Haley, AP, and Kesler, S. Exploring relationships among peripheral amyloid Beta, tau, cytokines, cognitive function, and psychosomatic symptoms in breast Cancer survivors. Biol Res Nurs. (2020) 22:126–38. doi: 10.1177/1099800419887230
218. Ancoli-Israel, S, Liu, L, Natarajan, L, Rissling, M, Neikrug, AB, Youngstedt, SD, et al. Reductions in sleep quality and circadian activity rhythmicity predict longitudinal changes in objective and subjective cognitive functioning in women treated for breast cancer. Support Care Cancer. (2022) 30:3187–200. doi: 10.1007/s00520-021-06743-3
219. Abercrombie, HC, Giese-Davis, J, Sephton, S, Epel, ES, Turner-Cobb, JM, and Spiegel, D. Flattened cortisol rhythms in metastatic breast cancer patients. Psychoneuroendocrinology. (2004) 29:1082–92. doi: 10.1016/j.psyneuen.2003.11.003
220. Ashton, NJ, Leuzy, A, Lim, YM, Troakes, C, Hortobagyi, T, Hoglund, K, et al. Increased plasma neurofilament light chain concentration correlates with severity of post-mortem neurofibrillary tangle pathology and neurodegeneration. Acta Neuropathol Commun. (2019) 7:5. doi: 10.1186/s40478-018-0649-3
221. Rohrer, JD, Woollacott, IO, Dick, KM, Brotherhood, E, Gordon, E, Fellows, A, et al. Serum neurofilament light chain protein is a measure of disease intensity in frontotemporal dementia. Neurology. (2016) 87:1329–36. doi: 10.1212/WNL.0000000000003154
222. Byrne, LM, Rodrigues, FB, Blennow, K, Durr, A, Leavitt, BR, Roos, RAC, et al. Neurofilament light protein in blood as a potential biomarker of neurodegeneration in Huntington's disease: a retrospective cohort analysis. Lancet Neurol. (2017) 16:601–9. doi: 10.1016/S1474-4422(17)30124-2
223. Hansson, O, Janelidze, S, Hall, S, Magdalinou, N, Lees, AJ, Andreasson, U, et al. Blood-based NfL: a biomarker for differential diagnosis of parkinsonian disorder. Neurology. (2017) 88:930–7. doi: 10.1212/WNL.0000000000003680
224. Huo, L, Du, X, Li, X, Liu, S, and Xu, Y. The emerging role of neural cell-derived exosomes in intercellular communication in health and neurodegenerative diseases. Front Neurosci. (2021) 15:738442. doi: 10.3389/fnins.2021.738442
225. Zhong, J, Ren, X, Liu, W, Wang, S, Lv, Y, Nie, L, et al. Discovery of novel markers for identifying cognitive decline using neuron-derived exosomes. Front Aging Neurosci. (2021) 13:696944. doi: 10.3389/fnagi.2021.696944
226. Faure, J, Lachenal, G, Court, M, Hirrlinger, J, Chatellard-Causse, C, Blot, B, et al. Exosomes are released by cultured cortical neurones. Mol Cell Neurosci. (2006) 31:642–8. doi: 10.1016/j.mcn.2005.12.003
227. Arioz, BI, Tufekci, KU, Olcum, M, Durur, DY, Akarlar, BA, Ozlu, N, et al. Proteome profiling of neuron-derived exosomes in Alzheimer's disease reveals hemoglobin as a potential biomarker. Neurosci Lett. (2021) 755:135914. doi: 10.1016/j.neulet.2021.135914
228. Ohmichi, T, Mitsuhashi, M, Tatebe, H, Kasai, T, Ali El-Agnaf, OM, and Tokuda, T. Quantification of brain-derived extracellular vesicles in plasma as a biomarker to diagnose Parkinson's and related diseases. Parkinsonism Relat Disord. (2019) 61:82–7. doi: 10.1016/j.parkreldis.2018.11.021
229. Koh, YQ, Tan, CJ, Toh, YL, Sze, SK, Ho, HK, Limoli, CL, et al. Role of exosomes in Cancer-related cognitive impairment. Int J Mol Sci. (2020) 21:82755. doi: 10.3390/ijms21082755
230. Cornblath, EJ, Lydon-Staley, DM, and Bassett, DS. Harnessing networks and machine learning in neuropsychiatric care. Curr Opin Neurobiol. (2019) 55:32–9. doi: 10.1016/j.conb.2018.12.010
Keywords: cancer, cognition, neuroimaging, biotypes, precision medicine
Citation: Kesler SR, Henneghan AM, Prinsloo S, Palesh O and Wintermark M (2023) Neuroimaging based biotypes for precision diagnosis and prognosis in cancer-related cognitive impairment. Front. Med. 10:1199605. doi: 10.3389/fmed.2023.1199605
Received: 03 April 2023; Accepted: 15 August 2023;
Published: 29 August 2023.
Edited by:
Noha Sharafeldin, University of Alabama at Birmingham, United StatesReviewed by:
Radhika Gangaraju, University of Alabama at Birmingham, United StatesCopyright © 2023 Kesler, Henneghan, Prinsloo, Palesh and Wintermark. This is an open-access article distributed under the terms of the Creative Commons Attribution License (CC BY). The use, distribution or reproduction in other forums is permitted, provided the original author(s) and the copyright owner(s) are credited and that the original publication in this journal is cited, in accordance with accepted academic practice. No use, distribution or reproduction is permitted which does not comply with these terms.
*Correspondence: Shelli R. Kesler, c3JrZXNsZXJAYXVzdGluLnV0ZXhhcy5lZHU=
Disclaimer: All claims expressed in this article are solely those of the authors and do not necessarily represent those of their affiliated organizations, or those of the publisher, the editors and the reviewers. Any product that may be evaluated in this article or claim that may be made by its manufacturer is not guaranteed or endorsed by the publisher.
Research integrity at Frontiers
Learn more about the work of our research integrity team to safeguard the quality of each article we publish.