- 1Department of Tuberculosis, Shanghai Public Health Clinical Center Affiliated to Fudan University, Shanghai, China
- 2Department of Pulmonary Medicine, National Clinical Research Center for Infectious Disease, Shenzhen Third People's Hospital/The Second Affiliated Hospital, School of Medicine, Southern University of Science and Technology, Shenzhen, Guangdong, China
- 3Department of Tuberculosis, Chongqing Public Health Medical Center, Southwest University, Chongqing, China
- 4Department of Tuberculosis, Jiangxi Chest Hospital, Nanchang, Jiangxi, China
- 5Department of Tuberculosis, The Third Hospital of Zhenjiang, Zhenjiang, Jiangsu, China
- 6Department of Radiology, Beijing Chest Hospital, Capital Medical University, Beijing, China
- 7Department of Tuberculosis, Hebei Chest Hospital, Shijiangzhuang, Hebei, China
Background: Chest radiography (chest X-ray or CXR) plays an important role in the early detection of active pulmonary tuberculosis (TB). In areas with a high TB burden that require urgent screening, there is often a shortage of radiologists available to interpret the X-ray results. Computer-aided detection (CAD) software employed with artificial intelligence (AI) systems may have the potential to solve this problem.
Objective: We validated the effectiveness and safety of pulmonary tuberculosis imaging screening software that is based on a convolutional neural network algorithm.
Methods: We conducted prospective multicenter clinical research to validate the performance of pulmonary tuberculosis imaging screening software (JF CXR-1). Volunteers under the age of 15 years, both with or without suspicion of pulmonary tuberculosis, were recruited for CXR photography. The software reported a probability score of TB for each participant. The results were compared with those reported by radiologists. We measured sensitivity, specificity, consistency rate, and the area under the receiver operating characteristic curves (AUC) for the diagnosis of tuberculosis. Besides, adverse events (AE) and severe adverse events (SAE) were also evaluated.
Results: The clinical research was conducted in six general infectious disease hospitals across China. A total of 1,165 participants were enrolled, and 1,161 were enrolled in the full analysis set (FAS). Men accounted for 60.0% (697/1,161). Compared to the results from radiologists on the board, the software showed a sensitivity of 94.2% (95% CI: 92.0–95.8%) and a specificity of 91.2% (95% CI: 88.5–93.2%). The consistency rate was 92.7% (91.1–94.1%), with a Kappa value of 0.854 (P = 0.000). The AUC was 0.98. In the safety set (SS), which consisted of 1,161 participants, 0.3% (3/1,161) had AEs that were not related to the software, and no severe AEs were observed.
Conclusion: The software for tuberculosis screening based on a convolutional neural network algorithm is effective and safe. It is a potential candidate for solving tuberculosis screening problems in areas lacking radiologists with a high TB burden.
Introduction
Tuberculosis (TB) remains one of the leading causes of death worldwide, killing 1.4 million people per year (1). Although the disease is largely curable and preventable, an estimated 2.9 million per 10 million people falling sick with TB were not diagnosed or reported to the World Health Organization (WHO) (2). Therefore, there is a pressing need to improve the early diagnosis of TB disease, thus initiating treatment promptly and reducing the transmission of Mycobacterium tuberculosis. One effective strategy is systemic screening, which should distinguish people with a high possibility of TB from those without. Chest radiography (chest X-ray or CXR) is a widely used and cost-effective screening tool for TB detection, achieving a sensitivity and specificity of 85.0 and 96.0% for TB-related abnormalities, respectively (2–5). While in areas with low resources and a high TB burden requiring mass screening urgently, the interpretation of CXRs is labor-intensive and time-consuming under the circumstances that trained health personnel interpreting the CXRs are very lacking (6–11).
Computer-aided detection (CAD) technologies, especially those with AI algorithms, vastly increase the capacity of image reading and have similar or even better diagnostic accuracy performance compared to human readers (12–14). The technologies make it possible to perform accurate mass screening with fewer resources. Thus, the WHO has recommended CAD as an alternative to human interpretation of CXR for screening and triaging pulmonary TB in individuals aged 15 years or older (2). The edge of CAD is artificial intelligence, especially in the field of deep learning, in which convolutional neural networks (CNNs) are the most promising algorithms for dealing with visual tasks (15–17). JF CXR-1 (version 2) (JF Healthcare, Jiangxi, China) is a simultaneous CXR detection CAD software based on CNNs that detects multiple thorax diseases, such as TB, lung mass, and lung nodules. The software was trained on 14,160 CXRs from township-level hospitals across China. In the testing phase, 13122 CXRs were provided for JF CXR-1 to detect TB. Among them, 31.5% (4127/13122) were pulmonary tuberculosis, 16.3% (2143/13122) were other pulmonary diseases such as pneumonia, pulmonary abscess, lung cancers, etc., and 52.2% (6852/13122) were normal. And JF CXR-1 has achieved an AUC of 0.94, a sensitivity of 0.91 (3,755/4,127), and a specificity of 0.81 (7,286/8,995), which meet the WHO's criteria for the target product profile. To validate its effectiveness and safety in clinical use, we conducted prospective multicenter clinical research in six general infectious disease hospitals in mainland China.
Methods
Study setting and population
The study was conducted at Shanghai Public Health Clinical Center, Beijing Chest Hospital, the Third Hospital of Zhenjiang, Chongqing Public Health Medical Center, Jiangxi Province Chest Hospital, and Hebei Chest Hospital. All of them are designated hospitals for tuberculosis in China, and there are also healthy individuals in the medical examination centers of each hospital. Participants were recruited from the visitors from tuberculosis clinics and medical examination centers of the above hospitals since June 2020. The inclusion criteria were (1) being aged 15 or over, (2) being willing to receive the CXR examination or could provide the image (DICOM format) of the posterior-anterior CXR taken in the late 40 days, and (3) voluntarily participating and providing informed written consent. The exclusion criteria included (1) a history of obsolete pulmonary tuberculosis, (2) neutropenia, (3) infection with the human immunodeficiency virus (HIV), (4) subjects whose CXR images do not meet the diagnostic requirements, (5) those with a history of hematological disorders, (6) those with a history of pulmonary lobectomy, (7) those with a history of mental illness or cognitive disorder, (8) those who are pregnant or breastfeeding, (9) those who had participated in pharmaceutical clinical research within 30 days, and (10) those who had other conditions that investigators consider inappropriate for research participation. All enrolled participants provided informed written consent. This study was approved by the ethics committees of Shanghai Public Health Clinical Center, Beijing Chest Hospital, The Third Hospital of Zhenjiang, Chongqing Public Health Medical Center, Jiangxi Province Chest Hospital, and Hebei Chest Hospital, respectively.
Procedures
Each participant received a complete blood count (CBC) test and an HIV antibody test. Participants with neutropenia or/and HIV infection were excluded according to the results of the blood tests. Then, each of the rest of the participants received a digital posterior-anterior CXR. Even though not all the centers have the same X-ray machines, efforts were made to set the parameters as similar as possible. The CXR images were saved in DICOM format and imported into the AI-based CAD software (JF CXR-1 v2, produced by Jiangxi Zhongke Jiufeng Smart Medical Technology Co., Ltd.). JF-CXR-1 and the radiologist group read every single CXR image independently and were blinded to all the information, including age and sex. The AI algorithm would produce a probability score for each anonymized image to predict its likelihood of being TB-positive.
A score >0.35 indicated a high possibility of tuberculosis, prompting the need for further diagnostic measures such as microbiological tests and a chest CT scan (When the threshold score = 0.35, the combination of sensitivity, specificity, and Kappa value is the best). The radiologist group consisted of eight certified senior radiologists from a third-party organization. They utilized the Diagnosis for Pulmonary Tuberculosis of Chinese Health Industry Standards as the fundamental criteria for TB x-ray screening. All radiologists have at least 10 years of experience in Grade-A tertiary hospitals. The CXR images were read independently by five senior radiologists. Diagnosis suggestions in CXR reports with “suspect TB” or “TB” were considered positive for TB. “Normal” and “other abnormal” CXR were considered negative for TB. The final decision among the five radiologists was determined based on the principle that the minority is subordinate to the majority. If cases where three radiologists shared the same opinion but the other two disagreed, the image would be sent to three other radiologists for arbitration. The final decision of the radiologist group was determined by the three “arbitrators” in a written report. Two months after the CXR examination, the clinical diagnosis information of every participant was collected. TB diagnosis followed China's National TB Diagnosis Guideline (WS288-2017).
Data analysis
All the data were statistically described, including baseline information, effectiveness data, and safety data. The final decision of the radiologist group was set as the reference standard for the software to compare. For effectiveness, the main evaluation indicators were sensitivity and specificity, referring to the results of the radiologists' board. The secondary evaluation indicators were the area under the receiver operating characteristic (ROC) curve (AUC) and the consistency rate with the final diagnosis (including bacteriologically confirmed and clinical diagnoses). If an image was diagnosed as TB or non-TB by both the radiologist group and the AI software, it was defined as true positive (TP) or true negative (TN), respectively. If an image was diagnosed as TB by the radiologist group but non-TB by the software, it was defined as a false negative (FN). Moreover, if an image was diagnosed as non-TB by the radiologist group but TB by the software, it was defined as a false positive (FP). Sensitivity = TP/(TP + FN), specificity = TN/(TN + FP) and the consistency rate = (TP + TN)/(TP + TN + FP + FN). The ROC curve was acquired when the sensitivity was set as the Y-axis, and 1-specificity was set as the X-axis. And the AUC was the area under the ROC.
Sensitivity is the proportion of true positive tests in all patients with a condition. A test or instrument can yield a positive result for a subject with that condition. In our study, it means the test ability of AI software screening out TB on the CXRs compared with the radiologist group.
Specificity is the percentage of people without the disease who are correctly excluded by the test. It is important to exclude people with diseases during screening. In our study, it refers to the ability of AI software to rule out non-TB participants. Ideally, a test should provide high sensitivity and specificity. For safety, adverse events (AE) and severe adverse events (SAE) were evaluated for every participant since their CXR was imported into the AI software and stopped 2 weeks later.
Role of the AI developers
The AI developer had no role in study design, data collection, analysis, or manuscript writing, but they provided us with a free account to use the software and free technical support.
Results
Between 06 Nov 2020, and 04 Jun 2021, 1,218 participants were screened, and 53 failed screening. Thus, 1,165 were enrolled, 1,161 were included in the Full Analysis Set (FAS) and Safety Analysis Set (SS) due to one dropout and three eliminations, and 1,150 were included in the Per Protocol Set (PPS) due to 11 protocol deviations (Table 1; Figure 1). The participants' ages ranged from 15 to 86 years, and the average was 44.4 ± 16.4 years. Men accounted for 60.0% (697/1,161), while women accounted for 40.0% (464/1,161).
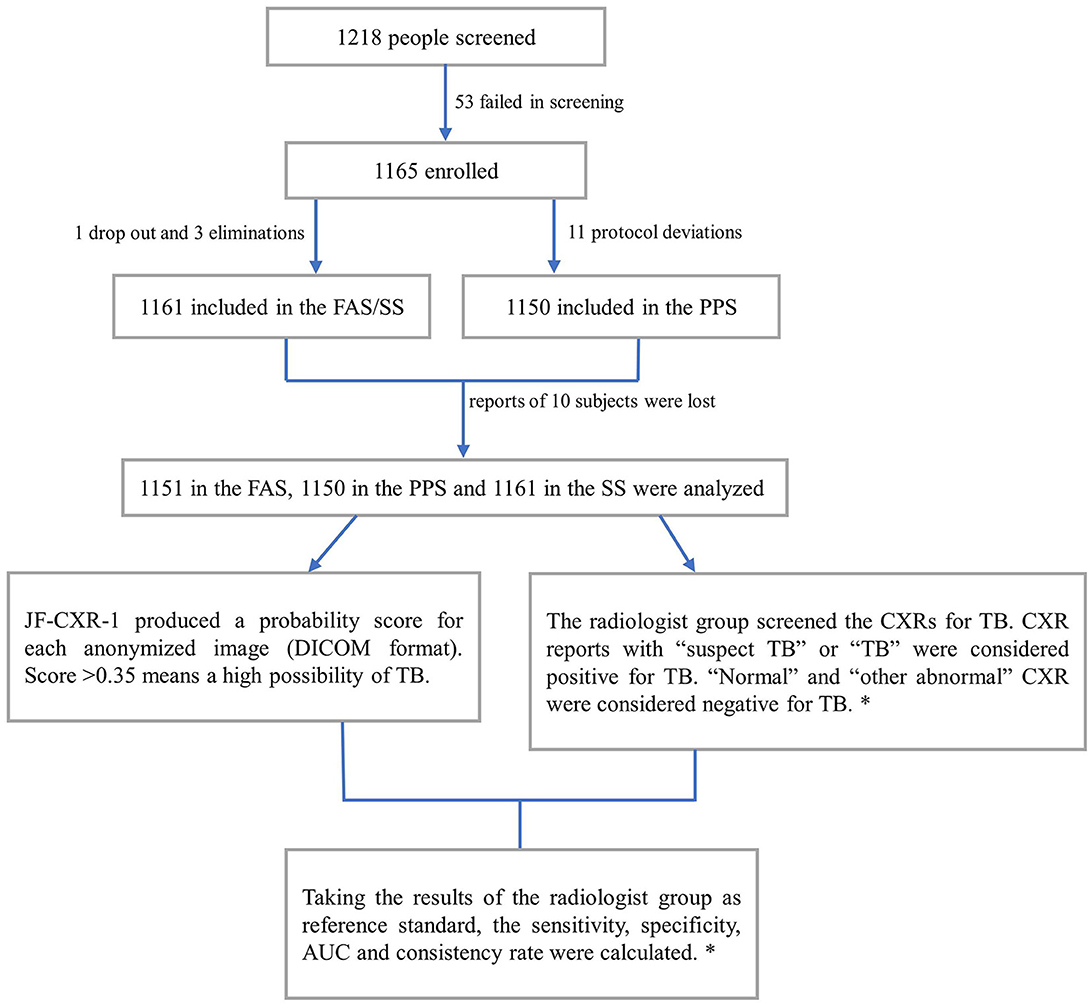
Figure 1. Participant selection process and the diagnostic workflow. FAS, analysis set; SS, safety analysis set; PPS, per protocol set; AUC, area under the receiver operating characteristic curves. *, details are in the methods section.
In the FAS, the reports of 10 subjects were lost. Thus, the results of 1,151 subjects were analyzed. According to the radiologist group, 601 images were positive for TB, while 550 were negative for TB. Compared with the reference standard, the sensitivity of the software was 94.2% (95% CI: 92.0–95.8%), while the specificity was 91.2% (95% CI: 88.5–93.2%). Therefore, the consistency rate was 92.7% (95% CI: 91.1–94.1%) with a Kappa value of 0.854 (P = 0.000) (Table 2). The AUC was 0.98 (Figure 2). After 2 months of clinical evaluation since enrollment, the diagnosis remained unclear for 29 out of 1,161 participants. Among the rest of the 1,132 participants, 687 were diagnosed with tuberculosis, while the others were not. Therefore, taking the final diagnosis (including bacteriologically confirmed and clinically diagnosed) as the ground truth, the sensitivity of the software was 78.9% (75.7–81.8%), the specificity was 89.9% (86.7–92.4%), and the consistency rate was 83.2% (80.9–85.3%) with a Kappa value of 0.662 (p = 0.000) (Table 3).
In the PPS, the results of 1,150 subjects were analyzed. We found that 600 images were positive for TB, while 550 were negative for TB. Compared with the reference standard, the sensitivity of the software was 94.2% (92.0%−95.8%), while the specificity was 91.2% (88.5–93.2%). Therefore, the consistency rate was 92.7% (91.1–94.1%) with a Kappa value of 0.854 (P = 0.000) (Table 4). The AUC was 0.98 (Figure 3). The diagnosis remained unclear for 29 out of 1,161 participants after 2 months of clinical evaluation since enrollment. Among the rest of the 1,121 participants, 582 were diagnosed with tuberculosis, while the other 539 were not. Therefore, taking the final diagnosis (including bacteriologically confirmed and clinically diagnosed) as the ground truth, the sensitivity of the software was 79.3% (76.1–82.2%), the specificity was 90.5% (87.4–92.9%), and the consistency rate was 83.7% (81.4–85.7%) with a Kappa value of 0.671 (p = 0.000) (Table 5). Since it has already been reported that the JF CXR-1 v2 algorithm performed worse among older age groups (>60 years) (13), performance analysis of the tool with both >60 and < 60 years was checked parallelly. In the FAS, 20.2% (233/1,151) of cases were >60 years old, and 79.8% (918/1,151) were ≤60 years old. When the results of CXRs were set as the reference standard, the sensitivity and specificity in the older group were 0.95(125/132) and 0.85(86/101), respectively, and 0.94(426/453) and 0.93(432/465) in the younger group, respectively (Supplementary Table S1). Nevertheless, the sample size was only 233, which was too small to draw a powerful conclusion.
In the safety set (SS), which included 1,161 participants, 0.3% (3/1,161) had AEs, and no SAE was reported. One had his ankle twisted while running, and two had drug-induced dermatitis after initiating anti-tuberculosis treatment. The AEs were mild, generally not bothersome, and unrelated to the software. No SAE was observed.
Discussion
In the era of the application of AI, the state-of-the-art in image recognition is CNNs, which have attracted a number of researchers to develop algorithms to replace human readers to identify tuberculosis in chest CXR images (3, 15, 18–24). However, the majority of the software is only tested with retrospective analysis (3, 13, 14, 17, 19, 25–27) or only with datasets (3, 18, 22, 23). Few studies focus on software evaluation in prospective clinical contexts (28, 29). JF CXR-1 v2 had been certified by the National Medical Products Administration of China for the screening and auxiliary diagnosis of active pulmonary tuberculosis in individuals no younger than 15 years and without immunodeficiency. We conducted this prospective multicenter clinical research to evaluate the performance of JF CXR-1 v2 to recognize tuberculosis in persons without immunodeficiency and aged 15 years or older. As described above, 1,151 subjects in the FAS were analyzed. Compared to the results from radiologists on the board (considered the reference standard), the software showed a sensitivity of 94.2% (95% CI: 92.0–95.8%) and a specificity of 91.2% (95% CI: 88.5–93.2%), and the consistency rate was 92.7% (91.1–94.1%) with a Kappa value of 0.854 (P = 0.000). The AUC was 0.98 (Figure 3). The results were very close in the PPS. The study of Nijiati et al. (17), which evaluated the performance of a trained AI model with CNNs screening TB in chest CXRs in an underdeveloped area, demonstrated its sensitivity, specificity, consistency rate, and AUC of 85.7%, 94.1%, 91.0%, and 0.910, respectively. Noteworthy, Nijiati et al. (17) also took the results of radiologists as a reference standard because their purpose was screening rather than triage. In a prospective study of a pilot active TB onsite screening project (4), where the reference standard in the project was bacteriologically confirmed, and clinically diagnosed TB, JF CXR-1 had sensitivity, specificity, and AUC of 100.0%, 95.7%, and 0.978 at threshold 30, and of 75.0%, 96.8%, and 0.859 at threshold 50. When the same reference standard was used in our study, the sensitivity, specificity, and consistency rates were 78.9% (75.7–81.8%), 89.9% (86.7–92.4%), and 83.2% (80.9–85.3%) with a Kappa value of 0.66 (p = 0.000) in the FAS, and 79.3% (76.1–82.2%), 90.5% (87.4–92.9%), and 83.7% (81.4–85.7%) with a Kappa value of 0.67 (p = 0.000) (Table 5) in the PPS.
The first open evaluation of JF CXR-1 was reported by Qin et al. in 2021 (13). JF CXR-1 was one of the five commercial AI algorithms evaluated for TB triaging in Dhaka, Bangladesh, a high-burden setting. Chest CXRs from 23,954 individuals were included in the analysis, and Xpert was set as the reference standard. However, JF CXR-1 has not met the WHO's Target Product Profile (TPP) of a triage test of at least 90.0% sensitivity and at least 70.0% specificity (30), similar to that of InferRead DR and Lunit INSIGHT. It has been proven that JF-CXR-1 significantly outperformed radiologists. When the sensitivity was fixed at 90.0%, the specificity was 61.1% (60.4–61.8%), while when the specificity was fixed at 70.0%, the sensitivity was 85.0% (83.8–86.2%). Moreover, the AUC was 0.849 (0.843–0.855). It was found to reduce half of the required Xpert tests while maintaining a sensitivity above 90.0%. JF CXR-1 had higher sensitivity for most of the decision thresholds (above ~0.15), which confers it more competence to be a better screening tool. Codlin et al. (25) conducted an independent evaluation of CAD software for TB screening; 12 types of software were included. The performance of each software was compared against both an expert and an intermediate human reader. Xpert results were the reference standard, and half of the 12 software programs, including JF CXR-1, achieved similar results on par with the expert reader. The AUC of JF CXR-1 was 0.77 (0.73–0.81), ranking third among the 12 evaluated CAD software.
Currently, there are 17 available or upcoming AI-CAD products for TB detection, including JF CXR-1. Nevertheless, only two are in the catalog of the Global Drug Facility of the Stop TB Partnership: CAD4TB software (The Netherlands) and InferRead DR Chest (Japan). JF CXR-1 is still under evaluation by the WHO (2020 report). CAD4TB is an example in Qin et al.'s retrospective research (13) of comparing the competence of 5 AI-CAD products for TB triaging, including CAD4TB, InferRead DR, Lunit INSIGHT, JF CXR-1, and qXR. CAD4TB was the top performer with sensitivity, specificity, and AUC of 90.0% (89.0–91.0%), 72.9% (72.3–73.5%), and 0.903, respectively. Besides, 49.0% of the people triaged by CAD4TB would be recalled for confirmatory tests in a program focused on capturing almost all people with tuberculosis, while the percentage was 57.0% by JF CXR-1 (13). In another prospective research, (31) which recruited symptomatic adults in a Pakistani hospital and used a reference mycobacterial culture of two sputa, CAD4TB had a sensitivity, specificity, and AUC of 93.0% (90.0–96.0%), 69.0% (67.0–71.0%), and 0.87 (0.85–0.86), respectively. In brief, the AUC of CAD4TB ranges from 0.71 to 0.94 (14, 32), and nearly all the reference standards were set according to the bacteriological results. The sensitivity, specificity, consistency rate, and AUC of JF CXR-1 in our study were non-inferior to most results from other AI-based software. However, since the reference standard in our study was from the human reader instead of bacteriological results, the application of JF CXR-1 is limited to clinical use. While it could play an important role in screening programs, especially in resource-constrained areas (4), the proportion of bacterially confirmed TB only accounts for 63.0% (1). Moreover, the purpose of screening is to pick out people suspected of having TB and those who need further diagnostic evaluation.
Our study has the advantages of a prospective nature and clinical validation, where the CXR data has not been used for software training and testing. There are also limitations. First, participants with obsolete tuberculosis were not included. Considering the confusing presentation of CXRs between obsolete tuberculosis and active tuberculosis, the efficiency of AI software might be lowered once obsolete tuberculosis is included. Second, because our purpose was to evaluate the screening performance of JF CXR-1, the main reference standard was the results drawn from the radiologist rather than the bacteriological results; the bacteriological screen has not been feasible due to financial reasons in a less developed, high-TB-burden country like China. Third, when it comes to real-world use, whether the software performs better than human readers remains uncertain because the comparison to bacteria was not conducted. Fourth, since the tool has shown poor performance in the age group >60 years (13), future studies are needed to fine-tune the algorithm in this area. The technology of AI is used not only for human resource optimization but also for better output. Therefore, we plan to conduct clinical research covering obsolete pulmonary tuberculosis participants and further evaluate the triage performance (with bacterial results) of JF CXR-1 in the future.
Furthermore, since the application of AI in tuberculosis screening is still under exploration, there are some practical considerations, especially in resource-constrained settings. First, some people might have little trust in AI and worry about data safety. Therefore, people undergoing TB screening should be aware that the screening tool utilizes AI software, and they should be given informed consent before proceeding with the screening process. Second, safety should be guaranteed for AI in healthcare (33). Unlike AI-based clinical decision support (CDS) software, screening software might be safer because of diagnosis procedures after screening. However, the reliability, validity, and stability of the software still need to be checked from time to time. Third, the network infrastructure might still be insufficient in resource-constrained settings, and there is the cost of managing network establishment, maintenance, and repair. There are limitations to CNNs: (1) CNNs have high computational requirements, and (2) since the CNNs have multiple layers, the training process takes a particularly long time if the computer does not have a powerful graphics processing unit (GPU). Even if TB screening with AI could save much money, whether resource-constrained areas could afford other costs or not remains problematic.
In our study, the software for tuberculosis screening based on a convolutional neural network algorithm was effective and safe, with satisfying diagnosis performance. It is a potential candidate for solving tuberculosis screening problems in areas lacking radiologists with a high TB burden.
Data availability statement
The raw data supporting the conclusions of this article will be made available by the authors, without undue reservation.
Ethics statement
The studies involving humans were approved by The Ethics Committee of Shanghai Public Health Clinical Center, The Ethics Committee of Beijing Chest Hospital, The Ethics Committee of the Third Hospital of Zhenjiang, The Ethics Committee of Chongqing Public Health Medical Center, The Ethics Committee of Jiangxi Province Chest Hospital, The Ethics Committee of Hebei Chest Hospital. The studies were conducted in accordance with the local legislation and institutional requirements. Written informed consent for participation in this study was provided by the participants' legal guardians/next of kin. No potentially identifiable images or data are presented in this study.
Author contributions
SL designed the study. YY, LX, and PL were responsible for conducting clinical research at Shanghai Public Health Clinical Center. FY, YW, HP, DH, and NL were responsible for conducting research at Chongqing Public Health Medical Center, Jiangxi Chest Hospital, the Third Hospital of Zhenjiang, Beijing Chest Hospital, Hebei Chest Hospital, and respectively. YY and LX gathered the data from all six centers, did the statistical analysis, and wrote the manuscript. All authors contributed to the article and approved the submitted version.
Funding
This study was supported by grants from the Shanghai Public Health Clinical Center (No. KY-GW-2021-21), the Shenzhen Key Medical Discipline Construction Fund (SZGSP010), the National Clinical Research Center for Infectious Diseases (Tuberculosis) (No. 2020B1111170014), the Shenzhen Clinical Research Center for Tuberculosis [No. (2021) 287 by the Shenzhen Scientific and Technological Innovation], the National Key Research and Development Program of China (2021YFC2301503), and the National Natural and Science Foundation of China (81873884, 82171739, 81770011, and 81900005).
Acknowledgments
We gratefully acknowledge the work of the Shanghai Public Health Clinical Center, Beijing Chest Hospital, The Third Hospital of Zhenjiang, Chongqing Public Health Medical Center, Jiangxi Province Chest Hospital, and Hebei Chest Hospital, all the colleagues, participants, and others who contributed to revealing the scientific truth.
Conflict of interest
The authors declare that the research was conducted in the absence of any commercial or financial relationships that could be construed as a potential conflict of interest.
Publisher's note
All claims expressed in this article are solely those of the authors and do not necessarily represent those of their affiliated organizations, or those of the publisher, the editors and the reviewers. Any product that may be evaluated in this article, or claim that may be made by its manufacturer, is not guaranteed or endorsed by the publisher.
Supplementary material
The Supplementary Material for this article can be found online at: https://www.frontiersin.org/articles/10.3389/fmed.2023.1195451/full#supplementary-material
References
2. WHO Operational Handbook on Tuberculosis. Module 2: Screening - Systematic Screening for Tuberculosis Disease. Geneva: World Health Organizatio. (2021).
3. Hwang EJ, Park S, Jin K-N, Kim JI, Choi SY, Lee JH, et al. Development and validation of a deep learning-based automatic detection algorithm for active pulmonary tuberculosis on chest radiographs. Clin Infect Dis. (2019) 69:739–47. doi: 10.1093/cid/ciy967
4. Liao Q, Feng H, Li Y, Lai X, Pan J, Zhou F, et al. Evaluation of an artificial intelligence (AI) system to detect tuberculosis on chest X-ray at a pilot active screening project in Guangdong, China in 2019. J Xray Sci Technol. (2022) 30:221–30. doi: 10.3233/XST-211019
5. WHO. Chest Radiography in Tuberculosis Detection: Summary of Current WHO Recommendations and Guidance on Programmatic Approaches. Geneva: World Health Organization (2016). Available online at: https://www.who.int/publications/i/item/9789241511506 (accessed January 3, 2023).
6. Hoog AHV, Meme HK, van Deutekom H, Mithika AM, Olunga C, Onyino F, et al. High sensitivity of chest radiograph reading by clinical officers in a tuberculosis prevalence survey. Int J Tuberc Lung Dis. (2011) 15:1308–14. doi: 10.5588/ijtld.11.0004
7. Melendez J, Sánchez CI, Philipsen RHHM, Maduskar P, Dawson R, Theron G, et al. An automated tuberculosis screening strategy combining X-ray-based computer-aided detection and clinical information. Sci Rep. (2016) 6:25265. doi: 10.1038/srep25265
8. Pande T, Pai M, Khan FA, Denkinger CM. Use of chest radiography in the 22 highest tuberculosis burden countries. Eur Respir J. (2015) 46:1816–9. doi: 10.1183/13993003.01064-2015
9. Piccazzo R, Paparo F, Garlaschi G. Diagnostic accuracy of chest radiography for the diagnosis of tuberculosis (TB) and its role in the detection of latent TB infection: a systematic review. J Rheumatol Suppl. (2014) 91:32–40. doi: 10.3899/jrheum.140100
10. Pinto LM, Pai M, Dheda K, Schwartzman K, Menzies D, Steingart KR. Scoring systems using chest radiographic features for the diagnosis of pulmonary tuberculosis in adults: a systematic review. Eur Respir J. (2013) 42:480–94. doi: 10.1183/09031936.00107412
11. Van't Hoog A, Viney K, Biermann O, Yang B, Leeflang MM, Langendam MW. Symptom- and chest-radiography screening for active pulmonary tuberculosis in HIV-negative adults and adults with unknown HIV status. Cochr Database Syst Rev. (2022) 3:CD010890. doi: 10.1002/14651858.CD010890.pub2
12. Cao XF Li Y, Xin HN, Zhang HR, Pai M, Gao L. Application of artificial intelligence in digital chest radiography reading for pulmonary tuberculosis screening. Chronic Dis Transl Med. (2021) 7:35–40. doi: 10.1016/j.cdtm.2021.02.001
13. Qin ZZ, Ahmed S, Sarker MS, Paul K, Adel ASS, Naheyan T, et al. Tuberculosis detection from chest x-rays for triaging in a high tuberculosis-burden setting: an evaluation of five artificial intelligence algorithms. Lancet Digit Health. (2021) 3:e543–54. doi: 10.1016/S2589-7500(21)00116-3
14. Qin ZZ, Sander MS, Rai B, Titahong CN, Sudrungrot S, Laah SN, et al. Using artificial intelligence to read chest radiographs for tuberculosis detection: a multi-site evaluation of the diagnostic accuracy of three deep learning systems. Sci Rep. (2019) 9:15000. doi: 10.1038/s41598-019-51503-3
15. Lakhani P, Sundaram B. Deep learning at chest radiography: automated classification of pulmonary tuberculosis by using convolutional neural networks. Radiology. (2017) 284:574–82. doi: 10.1148/radiol.2017162326
16. Ma L, Wang Y, Guo L, Zhang Y, Wang P, Pei X, et al. Developing and verifying automatic detection of active pulmonary tuberculosis from multi-slice spiral CT images based on deep learning. J Xray Sci Technol. (2020) 28:939–51. doi: 10.3233/XST-200662
17. Nijiati M, Zhang Z, Abulizi A, Miao H, Tuluhong A, Quan S, et al. Deep learning assistance for tuberculosis diagnosis with chest radiography in low-resource settings. J Xray Sci Technol. (2021) 29:785–96. doi: 10.3233/XST-210894
18. Nijiati M, Ma J, Hu C, Tuersun A, Abulizi A, Kelimu A, et al. Artificial intelligence assisting the early detection of active pulmonary tuberculosis from chest X-rays: a population-based study. Front Mol Biosci. (2022) 9:874475. doi: 10.3389/fmolb.2022.874475
19. Lee JH, Park S, Hwang EJ, Goo JM, Lee WY, Lee S, et al. Deep learning-based automated detection algorithm for active pulmonary tuberculosis on chest radiographs: diagnostic performance in systematic screening of asymptomatic individuals. Eur Radiol. (2021) 31:1069–80. doi: 10.1007/s00330-020-07219-4
20. Heo S-J, Kim Y, Yun S, Lim S-S, Kim J, Nam C-M, et al. Deep learning algorithms with demographic information help to detect tuberculosis in chest radiographs in annual workers' health examination data. Int J Environ Res Public Health. (2019) 16. doi: 10.3390/ijerph16020250
21. Pasa F, Golkov V, Pfeiffer F, Cremers D, Pfeiffer D. Efficient deep network architectures for fast chest X-ray tuberculosis screening and visualization. Sci Rep. (2019) 9:6268. doi: 10.1038/s41598-019-42557-4
22. Rajaraman S, Zamzmi G, Folio LR, Antani S. Detecting tuberculosis-consistent findings in lateral chest X-rays using an ensemble of CNNs and vision transformers. Front Genet. (2022) 13:864724. doi: 10.3389/fgene.2022.864724
23. Nafisah SI, Muhammad G. Tuberculosis detection in chest radiograph using convolutional neural network architecture and explainable artificial intelligence. Neural Comput Appl. (2022) 19:1–21. doi: 10.1007/s00521-022-07258-6
24. Lee S, Yim J-J, Kwak N, Lee YJ, Lee J-K, Lee JY, et al. Deep learning to determine the activity of pulmonary tuberculosis on chest radiographs. Radiology. (2021) 301:435–42. doi: 10.1148/radiol.2021210063
25. Codlin AJ, Dao TP, Vo LNQ, Forse RJ, Van Truong V, Dang HM, et al. Independent evaluation of 12 artificial intelligence solutions for the detection of tuberculosis. Sci Rep. (2021) 11:23895. doi: 10.1038/s41598-021-03265-0
26. Tavaziva G, Harris M, Abidi SK, Geric C, Breumninger M, Dheda K, et al. Chest X-ray analysis with deep learning-based software as a triage test for pulmonary tuberculosis: an individual patient data meta-analysis of diagnostic accuracy. Clin Infect Dis. (2022) 74:1390–400. doi: 10.1093/cid/ciab639
27. Zhou W, Cheng G, Zhang Z, Zhu L, Jaeger S, Lure FYM, et al. Deep learning-based pulmonary tuberculosis automated detection on chest radiography: large-scale independent testing. Quant Imaging Med Surg. (2022) 12:2344–55. doi: 10.21037/qims-21-676
28. Harris M, Qi A, Jeagal L, Torabi N, Menzies D, Korobitsyn A, et al. A systematic review of the diagnostic accuracy of artificial intelligence-based computer programs to analyze chest x-rays for pulmonary tuberculosis. PLoS ONE. (2019) 14:e0221339. doi: 10.1371/journal.pone.0221339
29. Tavaziva G, Majidulla A, Nazish A, Saeed S, Benedetti A, Khan AJ, et al. Diagnostic accuracy of a commercially available, deep learning-based chest X-ray interpretation software for detecting culture-confirmed pulmonary tuberculosis. Int J Infect Dis. (2022) 122:15–20. doi: 10.1016/j.ijid.2022.05.037
30. WHO. High Priority Target Product Profiles For New Tuberculosis Diagnostics: Report of a Consensus Meeting. Geneva: World Health Organization (2014). Available online at: https://www.who.int/publications/i/item/WHO-HTM-TB-2014.18 (accessed January 3, 2023).
31. Khan FA, Majidulla A, Tavaziva G, Nazish A, Abidi SK, Benedetti A, et al. Chest x-ray analysis with deep learning-based software as a triage test for pulmonary tuberculosis: a prospective study of diagnostic accuracy for culture-confirmed disease. Lancet Digit Health. (2020) 2:e573–81. doi: 10.1016/S2589-7500(20)30221-1
32. Kulkarni S, Jha S. Artificial intelligence, radiology, and tuberculosis: a review. Acad Radiol. (2020) 27:71–5. doi: 10.1016/j.acra.2019.10.003
Keywords: tuberculosis, screening, radiography, deep learning, convolutional neural network algorithm, computer-aided detection (CAD), diagnosis
Citation: Yang Y, Xia L, Liu P, Yang F, Wu Y, Pan H, Hou D, Liu N and Lu S (2023) A prospective multicenter clinical research study validating the effectiveness and safety of a chest X-ray-based pulmonary tuberculosis screening software JF CXR-1 built on a convolutional neural network algorithm. Front. Med. 10:1195451. doi: 10.3389/fmed.2023.1195451
Received: 28 March 2023; Accepted: 24 July 2023;
Published: 15 August 2023.
Edited by:
Ramalingam Bethunaickan, National Institute of Research in Tuberculosis (ICMR), IndiaReviewed by:
Chinnaiyan Ponnuraja, National Institute of Research in Tuberculosis (ICMR), IndiaUmashankar Vetrivel, Indian Council of Medical Research (ICMR), India
Alangudi Palaniappan Natarajan, National Institute of Research in Tuberculosis (ICMR), India
Copyright © 2023 Yang, Xia, Liu, Yang, Wu, Pan, Hou, Liu and Lu. This is an open-access article distributed under the terms of the Creative Commons Attribution License (CC BY). The use, distribution or reproduction in other forums is permitted, provided the original author(s) and the copyright owner(s) are credited and that the original publication in this journal is cited, in accordance with accepted academic practice. No use, distribution or reproduction is permitted which does not comply with these terms.
*Correspondence: Shuihua Lu, lushuihua66@126.com
†These authors share first authorship