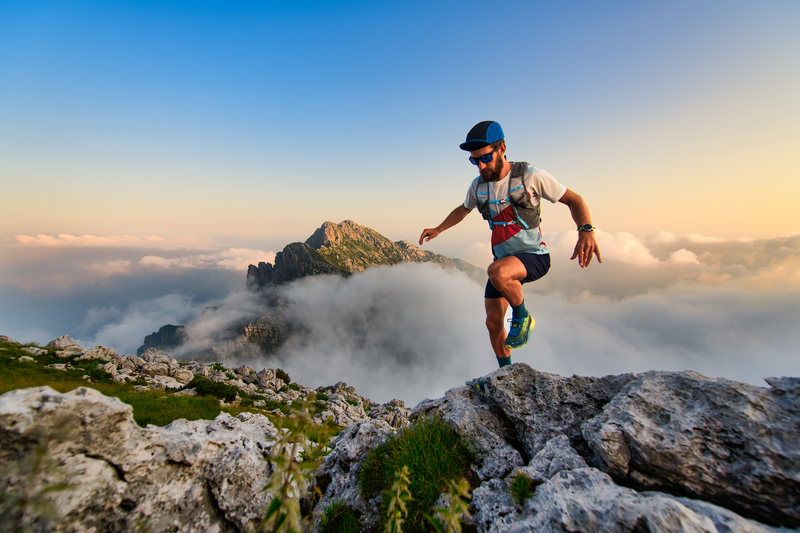
95% of researchers rate our articles as excellent or good
Learn more about the work of our research integrity team to safeguard the quality of each article we publish.
Find out more
ORIGINAL RESEARCH article
Front. Med. , 06 July 2023
Sec. Pulmonary Medicine
Volume 10 - 2023 | https://doi.org/10.3389/fmed.2023.1177786
This article is part of the Research Topic Future Research Questions for Improving COPD Diagnosis and Care View all 13 articles
Background: Providing intensive care is increasingly expensive, and the aim of this study was to construct a risk column line graph (nomograms)for prolonged length of stay (LOS) in the intensive care unit (ICU) for patients with chronic obstructive pulmonary disease (COPD).
Methods: This study included 4,940 patients, and the data set was randomly divided into training (n = 3,458) and validation (n = 1,482) sets at a 7:3 ratio. First, least absolute shrinkage and selection operator (LASSO) regression analysis was used to optimize variable selection by running a tenfold k-cyclic coordinate descent. Second, a prediction model was constructed using multifactorial logistic regression analysis. Third, the model was validated using receiver operating characteristic (ROC) curves, Hosmer-Lemeshow tests, calibration plots, and decision-curve analysis (DCA), and was further internally validated.
Results: This study selected 11 predictors: sepsis, renal replacement therapy, cerebrovascular disease, respiratory failure, ventilator associated pneumonia, norepinephrine, bronchodilators, invasive mechanical ventilation, electrolytes disorders, Glasgow Coma Scale score and body temperature. The models constructed using these 11 predictors indicated good predictive power, with the areas under the ROC curves being 0.826 (95%CI, 0.809–0.842) and 0.827 (95%CI, 0.802–0.853) in the training and validation sets, respectively. The Hosmer-Lemeshow test indicated a strong agreement between the predicted and observed probabilities in the training (χ2 = 8.21, p = 0.413) and validation (χ2 = 0.64, p = 0.999) sets. In addition, decision-curve analysis suggested that the model had good clinical validity.
Conclusion: This study has constructed and validated original and dynamic nomograms for prolonged ICU stay in patients with COPD using 11 easily collected parameters. These nomograms can provide useful guidance to medical and nursing practitioners in ICUs and help reduce the disease and economic burdens on patients.
Chronic obstructive pulmonary disease (COPD) is a common progressive disease with persistent respiratory symptoms and airflow limitation caused by the combination of chronic bronchitis and emphysema (1). It is one of the most common chronic diseases worldwide that can lead to prolonged cough, dyspnea, and fatigue, and accounts for a large proportion of intensive care unit (ICU) admissions (2, 3). In the year 2019, a staggering number of 212.3 million cases of COPD were reported worldwide, leading to 3.3 million fatalities and 74.4 million years of disability adjusted life (4). Furthermore, in the same year, COPD ranked as the third most prevalent cause of death (5). Due to its high prevalence, morbidity, and mortality, COPD is a significant area of concern for both medical care and public health (6). Furthermore, research has shown that COPD imposes a considerable economic and social burden on patients and healthcare systems (7, 8). Efforts to prevent and manage COPD are crucial not only for improving patient outcomes but also for reducing the economic and societal impact of the disease.
An ICU is a place where medical professionals apply modern medical theories and high-technology modern medical equipment to provide specialized centralized monitoring, treatment, and care for critically ill patients, and is an integral part of the healthcare system (9, 10). ICUs provide complex and expensive care (11), and account for a significant portion of the financial expense of healthcare in many countries worldwide (12), for example, in the United States, ICU medical costs account for approximately 13% of hospital costs and 4% of national health expenditure (13). Despite the enormous investment in critical care medicine in many countries, ICUs are often underresourced to meet the needs of critically ill patients, especially in less-developed countries (14). Length of stay (LOS) in the ICU is a key indicator of healthcare efficiency and an important indicator of the quality of critical care provided by a hospital (15, 16). Prolonged ICU stays often result in large resource utilization and thus markedly increased healthcare costs (17). A multistate, multihospital analysis found that ICU utilization rates varied widely among patients hospitalized with COPD. It is therefore particularly important to determine the factors that prolong LOS in the ICU for patients with COPD in order to help accelerate ICU bed turnover, reduce patient care and financial burden, and prevent poor prognoses.
The literature (18–22) has suggested that LOS in hospitalized patients with COPD may be influenced by various factors, such as age, smoking history, Charlson Comorbidity Index, comorbidities, malnutrition, mobility, and mechanical ventilation. Some vital-sign parameters such as higher respiratory rate on admission, systolic blood pressure > 140 mmHg, and diastolic blood pressure > 90 mmHg have also been considered as risk factors for LOS (23, 24). However, the factors that influence the length of ICU stay for patients with COPD are not well defined.
Previous nomograms (25) often suffered from small sample sizes, which could limit the generalizability of their results and hinder their applicability in diverse populations of critically ill patients. Moreover, these models relied on predictors that were difficult or time-consuming to collect, making them less practical for real-world clinical application. Additionally, the representation of the entire spectrum of severity among critically ill patients was not always achieved in previous models, potentially affecting their predictive accuracy and clinical usefulness. In this study, we aimed to address these limitations by constructing a novel nomogram for predicting prolonged ICU stays in patients with COPD. We utilized the large and reliable MIMIC-IV (Medical Information Mart for Intensive Care IV) database, which contains comprehensive, deidentified, and well-maintained clinical data on ICU patients, providing an excellent foundation for the development of our predictive model. This study therefore aimed to identify predictors of prolonged LOS in the ICU and attempted to construct a valid predictive model. This could help clinicians and nurses identify patients who require extended ICU stays and develop appropriate interventions to speed up ICU bed turnover and reduce healthcare costs.
The MIMIC-IV database is available primarily for researchers from the Massachusetts Institute of Technology Computational Physiology Laboratory and Collaborative Research Group (26). This database includes demographic information, vital signs, laboratory indicators, medications, and medical-care information. The database has a large sample, comprehensive information, and long-term patient follow-ups, while being free to use and providing a rich resource for critical-care research (27).
This study adhered to the provisions of the Declaration of Helsinki, and ethics approval was provided by the Ethics Committee and Institutional Review Board of the First Affiliated Hospital of Jinan University. Besides, our authors received permission after completing the “Protecting Human Research Participants” web-based training program from the National Institutes of Health (record ID: 45369280). The requirement for obtaining individual patient consent was waived in the present study since it did not have any direct implications on clinical care, and all confidential health data was anonymized to safeguard patient privacy.
The number of 69,211 patients admitted to ICU in MIMIC-IV database from 2008–2019. This study used the Ninth Revisions of the International Classification of Diseases (49,120, 49,121, 49,122, 496) and the Tenth Revisions of the International Classification of Diseases (J44, J440, J441, J449) codes to extract 9,980 patients admitted to the ICU with a COPD diagnosis (including acute exacerbated COPD) from the MIMIC-IV database. The following populations were excluded in this study: (1) patients under the age of 18, (2) patients who had multiple admissions other than the first hospital/ICU admission, (3) patients with ICU stays of less than 1 day, including those who died upon ICU admission, and (4) patients with a hospital stay shorter than their ICU stay. The final population for our study was 4,940 patients. Figure 1 illustrates the detailed patient selection process.
The following data were extracted from the MIMIC-IV database using structured query language (28): (1) general patient data and demographic characteristics: sex, age, weight, height, race, smoking history, marital status, and length of ICU stay; (2) vital signs: body temperature, heart rate, respiratory rate, mean blood pressure, and pulse oximetry-derived oxygen saturation; (3) laboratory test results: pH, glucose, hematocrit, hemoglobin, platelets, white blood cell count, anion gap, bicarbonate, blood urea nitrogen, calcium, chloride, creatinine, sodium, potassium, internal normalized ratio, prothrombin time, partial thromboplastin time(s), PaO2, Lactate, total CO2, PaCO2, lymphocytes, basophils, eosinophils, monocytes, neutrophils and urine output; (4) comorbidities: sepsis, myocardial infarct, congestive heart failure, peripheral vascular disease, cerebrovascular disease, dementia, rheumatic disease, peptic ulcer disease, mild liver disease, uncomplicated diabetes, paraplegia, renal disease, malignant cancer, severe liver disease, metastatic solid tumor, acquired immunodeficiency syndrome, respiratory failure, ventilation associated pneumonia (VAP), hypertension, pneumonia, septic shock, electrolytes disorder and asthma; (5) scores on assessment scales: Charlson comorbidity index, Acute Physiology score III (APSIII), Oxford Acute Severity of Illness score (OASIS), Sequential Organ Failure Assessment (SOFA) score, Glasgow Coma Scale (GCS) score and Braden Scale score; and (6) treatment and medications: renal replacement therapy (RRT), antibiotic, norepinephrine, invasive mechanical ventilation, bronchodilators and glucocorticoids. For vital signs and laboratory test results, we extracted the mean values on the first day of ICU admission. The multiple interpolation (29) was used to process missing data. However, features with a missing rate higher than 20% (height) were removed since we aimed to build a prediction model that can be generalized in the real clinic which should contain accessible data. The missing rate of all extracted variables was shown in Supplementary Figure 1.
After applying the inclusion and exclusion criteria, the final population analyzed comprised 4,940 patients. Given the largeness of the sample, we used the Type 2a scheme TRIPOD (30) (Transparent Reporting of a multivariable prediction model for Individual Prognosis or Diagnosis) for prediction-model building and validation. The data set was first randomly divided into training (n = 3,458) and validation (n = 1,482) sets at a 7:3 ratio. The predictor variables included in the column line graph (nomogram) were selected in two steps. First, we analyzed the data in the training set using least absolute shrinkage and selection operator (LASSO) regression (31, 32) to select the best risk factors for prolonged ICU stay. LASSO analysis (33, 34) is performed by generating a penalty function that is a compression of the coefficients of the variables in the regression model to prevent overfitting and solve the problem of severe covariance. Second, the most important features selected by the LASSO regression from the training set were used in a multifactorial logistic regression analysis. Variables with p < 0.05 were included in the nomogram, and multifactorial analysis was used to predict the respective probabilities of prolonged ICU stay in patients with COPD.
The validation of the prediction model consisted of three main processes: discrimination, calibration, and clinical validity. In this study, the areas under the receiver operating characteristic (ROC) curves (area under curve, AUC) were used to determine the discrimination of the model, the Hosmer-Lemeshow test and calibration plots were used to evaluate its calibration, and decision-curve analysis (DCA) was used to assess its clinical validity.
The primary outcome was prolonged ICU length of stay. Detailed ICU admission time and discharge time were recorded for all patients in MIMIC-IV. Length of ICU stay was calculated as the difference between ICU discharge time (icu_outtime) and ICU admission time (icu_intime). Currently, there is no universally accepted definition of prolonged ICU length of stay (35). ICU length of stay >5 days was defined prolonged LOS in the ICU in this study (36) (based on the 75th percentile of ICU LOS in our sample).
We performed a descriptive analysis of the above variables. The baseline data of all patients were grouped by prolonged ICU stay (yes or no). Continuous variables were expressed as medians (25th–75th percentiles); categorical variables were expressed as counts and percentages. The Wilcoxon rank sum test was used to compare group differences for continuous variables, and Chi-squared tests were used to compare categorical variables. For covariance between continuous variables in the logistic regression, we used the variance inflation factor (VIF), with VIF < 4 indicated no multicollinearity among predictors (37).
All data were analyzed and processed using R software (version 4.2.1, https://www.r-project.org/) for statistical analysis and processing (38). Multiple interpolation was performed using the “mice” package, descriptive analysis and comparisons of differences between groups were performed using “tableone” package, LASSO regression analysis was performed using “glmnet” package, multifactor logistic regression analysis was performed using “rms” package, ROC curves were plotted using “pROC” package, and DCA was performed using “rmda” package. A probability value of p < 0.05 was considered statistically significant, and all statistical tests were two-sided.
The number of 4,940 patients were included in this study, and 1,255 (25.4%) prolonged ICU stay (length of stay more than 5 days). The baseline characteristics of all patients were lied in Table 1. The median age of all patients was approximately 72 years old, and 2,660 (53.8%) men were included in the study. Compared with the non-prolonged ICU stay patients, prolonged ICU stay patients generally had more comorbidities, medication, treatment, and tended to have more severe illness severity scores (Table 1). The demographics and clinical characteristics of the patients in the training and validation sets are listed in Supplementary Table 1. There was good comparability between the two groups of patients.
Among the 72 relevant feature variables extracted, 13 potential predictor variables were selected based on the data from the training set (Figures 2A,B) that had nonzero coefficients in the LASSO regression model. Features fitted to construct prediction models were selected by the largest λ at which the mean square error (MSE) is within one standard error of the minimal MSE (Supplementary Table 2). These predictors were sepsis, renal replacement therapy cerebrovascular disease, respiratory failure, ventilator associated pneumonia norepinephrine, bronchodilators, invasive mechanical ventilation, electrolytes disorders, Glasgow Coma Scale score acute physiology score III oxford acute severity of illness score and body temperature. However, in the multivariate logistic regression, two variables (APSIII and OASIS) were excluded because they were not statistically effects (Supplementary Table 3). Finally, we included 11 variables to construct the model.
The results of the multifactorial logistic regression analysis for sepsis, renal replacement therapy (RRT), cerebrovascular disease, respiratory failure, ventilator associated pneumonia (VAP), norepinephrine, bronchodilators, invasive mechanical ventilation, electrolytes disorders, Glasgow Coma Scale score (GCS) and body temperature were listed in Table 2. All of these predictor variables had significant effects, and hence they were used to construct a nomogram of the risk of prolonged LOS in the ICU for patients with COPD, which is presented in Figure 3A. Each predictor variable indicator corresponds to a set of values on the scale with the score scale on the top (points), the scores of all the indicators were summed to obtain the total score, and the position of the total score on the bottom total points scale (total points) corresponds to the probability of having a prolonged LOS in the ICU for patients with COPD. This study also applied the “shiny app” package of R software,1 which is mostly used to help physicians and nurses working in clinical settings to predict risks and individualize patient assessments. As an example to help the reader better understand the nomogram, if a COPD patient with sepsis, respiratory failure, and ventilate associated pneumonia has been treated with bronchodilators, invasive mechanical ventilation, norepinephrine, a Glasgow Coma Score of 9, and a temperature of 38°C during ICU stay. Then the probability of prolonged LOS in his/her ICU was about 90.1% (95%CI, 0.835–0.943; Figure 3B).
Table 2. Multivariate logistic regression analysis of the selected significant clinical characteristics in the training set.
To validate the predictive models, we first performed a VIF test with all variables scoring less than 4. There was no covariance and the model fit was good. The AUC values (equal to C-index) were 0.826 (95%CI, 0.809–0.842) and 0.827 (95%CI, 0.802–0.853) in the training and validation sets, respectively (Figure 4), which indicates good performance. The results of a Hosmer-Lemeshow test indicated strong agreement between the predicted and observed probabilities in the training (χ2 = 8.21, p = 0.413) and validation (χ2 = 8.21, p = 0.413) sets. The calibration curves of the nomograms used to predict the risk of prolonged LOS in the ICU for patients with COPD also indicated good consistency (Figures 5A,B). Together these validation results indicate that the nomograms of the model had good predictive effects.
The blue line in the DCA graph in Figure 6 indicates the scenarios in which this model predicts the occurrence of prolonged LOS in ICUs for patients with COPD. For comparison purposes, the horizontal and diagonal lines represent the two extreme cases: the former represents all samples being negative, while the latter represents all samples being positive. The DCA results indicated that patients with COPD would obtain higher net clinical benefits when using nomograms to predict prolonged ICU stay in both the training and validation sets (Figures 6A,B). For example, in the validation set, assuming a timely intervention for patients with COPD with a 40% risk of developing an prolonged ICU stay, 9 people would benefit for every 100 interventions.
Column line graphs (nomograms) are simple, reliable, and practical forecasting tools (39). They have been widely used in clinical settings to help predictions and decision-making by identify several relevant predictors (40). We have constructed the first risk prediction model for a prolonged ICU stay in patients with COPD that has good predictive effects, with an AUC of 0.827 (95%CI, 0.802–0.853) in the validation set. The prediction model established in this study was also strongly calibrated and had good clinical validity. Sepsis, RRT cerebrovascular disease, respiratory failure, VAP norepinephrine, bronchodilators, invasive mechanical ventilation, electrolytes disorders, GCS and body temperature of patients with COPD can be used as predictors. We can therefore clinically predict the probability of prolonged ICU stays of patients with COPD by obtaining healthy history information, performing physical examinations, drugs and treatment measures. This prediction model can provide guidance for the development of strategies to prevent prolonged ICU length of stay.
Sepsis is a clinical syndrome that poses a life-threatening risk to patients due to the dysregulation of their response to infections, which leads to organ dysfunction (41). Similar to previous studies, sepsis could result in prolonged ICU stays for patients (42, 43). The treatment of sepsis would take a long time and consume a lot of medical resources, which led to a longer stay in the ICU (44). Renal replacement therapy is a therapeutic approach that utilizes blood purification technology to clear solutes, in order to replace the impaired renal function as well as play a protective and supportive role on organ function (45). The use of RRT will prolong ICU stay in our study. Contrary to our conclusion, a meta-analysis showed that early renal replacement therapy was associated with significantly shorter ICU length of stay (46). This disparity may be explained due to differences in data collection. This study was concerned only with the use of RRT with COPD patients instead of the timing of RRT use.
The GCS is composed of the eye-opening response, verbal response, and body movement (47). It is not surprising that the GCS score is a protective factor for longer LOS in patients with COPD (OR = 0.86, 95% CI = 0.84–0.89, p < 0.001), which is consistent with results in clinical practice (48). This is because a lower GCS score is indicative of a more-severe case of impaired consciousness and therefore a longer ICU stay.
Bronchodilators, which include β2-adrenergic agonists, anticholinergics, and theophyllines, are the main measures to control symptoms in patients with COPD (49). Bronchodilators were associated with prolonged ICU stay in this study. Because patients with COPD who are treated with bronchodilators usually experience acute exacerbations, they will have increased dyspnea symptoms and declined respiration function and will require ICU observation for a longer duration than nonusers, resulting in a longer ICU stay. Similarly, cerebrovascular disease, one of the major health problems worldwide (50), is accompanied by multiple forms of neurological dysfunction and altered vascular statuses, including stroke. This undoubtedly increases the risk of patient care and prolonged patient stays. In additional, electrolyte disorders are common in the ICU and can seriously affect the blood supply and metabolism (51). This led to longer ICU stays for patients (52).
Previous studies (53–55) have found that the body temperature of a patient on admission affects their LOS in the ICU. Although those studies were performed on other populations, body temperature was still a predictor for the model in this study. Changes in body temperature is one of the important indicators of condition monitoring, and can affect subsequent treatment and prognosis. Geffroy et al. (56) found that patients with early fever were more likely to have a poor prognosis and hence a prolonged ICU stay.
Similar to the results of previous studies (57–61), invasive mechanical ventilation, respiratory failure and ventilator associated pneumonia increased the LOS in the ICU for patients with COPD. This is due to these patients usually had a more-severe gas exchange impairment, require respiratory support, and have a longer ICU stay. However, unlike previous studies (53), norepinephrine was associated with prolonged LOS in the ICU, and was therefore a risk factor. Possible reasons for this are that patients using norepinephrine have unstable circulatory function and greater variability in their condition and LOS, thus requiring more attention and monitoring by nurses and doctors (48).
Our study aimed to construct a nomogram for predicting prolonged LOS among patients with COPD hospitalized in the ICU. This study differs from the previous study in that our outcome of interest is prolonged LOS, while the other study focused on 30-day mortality prediction (62). Furthermore, we utilized a distinct set of variables to identify predictors specifically relevant to extended LOS among COPD patients in the ICU. Our study contributes to the literature by providing healthcare professionals with a practical tool to stratify patients, optimize resource allocation, and improve patient care. By developing a nomogram that can predict prolonged LOS, we offer clinicians an additional means to identify patients at high risk for prolonged ICU LOS, allowing for more informed decision-making and targeted interventions. Overall, our study represents a significant advancement in understanding and managing COPD patients in the ICU, and has important clinical implications for improving patient outcomes and resource utilization.
Identifying patients at risk of prolonged length of stay may help ICU management and avoid ICU a shortage of ICU beds (53). Considering the specificity and complexity of patients with COPD in the intensive care unit, this study selected 4,940 patients with severe COPD from a large intensive care medicine database for a retrospective population-based study. The predictors selected for this study (e.g., temperature, GCS, RRT, and use of invasive mechanical ventilation) are simpler and more accessible to critical care physicians and nurses than respiratory specialty indicators, and help clinicians in their decision making.
This study was based on a large and diverse population of the MIMIC-IV database, but in practice it was subject to several limitations. First, this study had a retrospective single-center design and the sample may be underrepresented. External validation of the line plots was not performed (only internal validation), which may lead to overfitting of the new model, requiring the use of data from other sources to validate the findings. Second, some potentially important factors were not included in our study, making it less comprehensive, including, body mass index (BMI), certain psychosocial factors such as anxiety, depression, and social support. Third, limited by our current capabilities and the extent to which the database was available, we still found that antibiotic and vasopressin use prolonged ICU stays in patients with COPD, which was only expressed simply using categorical variables, which makes the effect of specific dosages on the LOS of patients unclear. Fourth, the included study population meant that important variables such as body temperature ranged from 31°C to 40°C in our nomograms, and there was no way to make good predictions for patients with body temperatures outside this range; this needs to be addressed in future studies. Finally, our research did not specifically examine the repercussions of malnutrition on the intensive care unit length of stay for patients with chronic obstructive pulmonary disease. Malnutrition, a crucial element, has been proven to affect clinical outcomes in individuals with this condition. Regrettably, owing to the absence of exhaustive nutritional data in our patient sample, we could not explore the correlation between malnutrition and length of stay (63). This constraint might have hindered our comprehensive understanding of the intricate factors influencing disease prognosis, potentially leading to an underestimation of nutritional status significance in our conclusions. Consequently, we propose that forthcoming investigations incorporate malnutrition evaluation tools, such as the Mini Nutritional Assessment, Subjective Global Assessment, and Nutritional Risk Screening, to appraise the nutritional condition of patients with chronic obstructive pulmonary disease (64). This approach would yield invaluable insights into malnutrition’s impact on disease progression and outcomes, ultimately aiding in the creation of more focused and efficacious interventions to address this critical aspect of disease management. Moving forward, we intend to encompass as many predictor variables as feasible and validate the model using an external cohort to achieve heightened accuracy in our findings.
Based on the MIMIC-IV database, we have constructed and validated original predictive and dynamic nomograms for prolonged ICU stays in patients with COPD using 11 easily collected parameters The nomograms (available at https://cht19991225.shinyapps.io/Dynamic_nomogram/) will help medical doctors and nursing practitioners to accurately assess the probability of a prolonged ICU stay in specific COPD patients and to distinguish high-risk patients who may require aggressive medical/nursing measures.
The datasets presented in this study can be found in online repositories. The names of the repository/repositories and accession number(s) can be found at: the data were available on the MIMIC-IV website at https://mimic.physionet.org/.
The studies involving human participants were reviewed and approved by the Ethics Committee and Institutional Review Board of the First Affiliated Hospital of Jinan University. Written informed consent for participation was not required for this study in accordance with the national legislation and the institutional requirements.
HC, JLi, and FW created the study protocol, performed the statistical analyses, and wrote the first manuscript draft. JLy and FZ conceived the study, critically revised the manuscript, contributed to data interpretation and manuscript revision. SY and XY assisted with the study design and performed data collection. XH assisted with data collection and manuscript editing. SY confirmed the data and assisted with the statistical analyses. HC maintained the localized database and worked on SQL coding. All authors contributed to the article and approved the submitted version.
The work was supported by Guangdong Provincial Key Laboratory of Traditional Chinese Medicine Informatization (2021B1212040007), Clinical Frontier Technology Program of the First Affiliated Hospital of Jinan University, China (no. JNU1AF-CFTP-2022-a01235), and the Science and Technology Projects in Guangzhou, China (nos. 202201020054 and 2023A03J1032).
The authors declare that the research was conducted in the absence of any commercial or financial relationships that could be construed as a potential conflict of interest.
All claims expressed in this article are solely those of the authors and do not necessarily represent those of their affiliated organizations, or those of the publisher, the editors and the reviewers. Any product that may be evaluated in this article, or claim that may be made by its manufacturer, is not guaranteed or endorsed by the publisher.
The Supplementary material for this article can be found online at: https://www.frontiersin.org/articles/10.3389/fmed.2023.1177786/full#supplementary-material
1. Vogelmeier, CF, Criner, GJ, Martinez, FJ, Anzueto, A, Barnes, PJ, Bourbeau, J, et al. Global strategy for the diagnosis, management, and prevention of chronic obstructive lung disease 2017 report. GOLD executive summary. Am J Respir Crit Care Med. (2017) 195:557–82. doi: 10.1164/rccm.201701-0218PP
2. Langsetmo, L, Platt, RW, Ernst, P, and Bourbeau, J. Underreporting exacerbation of chronic obstructive pulmonary disease in a longitudinal cohort. Am J Respir Crit Care Med. (2008) 177:396–401. doi: 10.1164/rccm.200708-1290OC
3. Warwick, M, Fernando, SM, Aaron, SD, Rochwerg, B, Tran, A, Thavorn, K, et al. Outcomes and resource utilization among patients admitted to the intensive care unit following acute exacerbation of chronic obstructive pulmonary disease. J Intensive Care Med. (2021) 36:1091–7. doi: 10.1177/0885066620944865
4. Safiri, S, Carson-Chahhoud, K, Noori, M, Nejadghaderi, SA, Sullman, MJM, Ahmadian Heris, J, et al. Burden of chronic obstructive pulmonary disease and its attributable risk factors in 204 countries and territories, 1990-2019: results from the global burden of disease study 2019. BMJ. (2022) 378:e69679. doi: 10.1136/bmj-2021-069679
5. GBD 2019 Diseases and Injuries Collaborators . Global burden of 369 diseases and injuries in 204 countries and territories, 1990-2019: a systematic analysis for the global burden of disease study 2019. Lancet. (2020) 396:1204–22. doi: 10.1016/S0140-6736(20)30925-9
6. Criner, RN, and Han, MK. COPD care in the 21st century: a public health priority. Respir Care. (2018) 63:591–600. doi: 10.4187/respcare.06276
7. Choi, HS, Yang, DW, Rhee, CK, Yoon, HK, Lee, JH, Lim, SY, et al. The health-related quality-of-life of chronic obstructive pulmonary disease patients and disease-related indirect burdens. Korean J Intern Med. (2020) 35:1136–44. doi: 10.3904/kjim.2018.398
8. Hurst, JR, Siddiqui, MK, Singh, B, Varghese, P, Holmgren, U, and de Nigris, E. A systematic literature review of the humanistic burden of COPD. Int J Chron Obstruct Pulmon Dis. (2021) 16:1303–14. doi: 10.2147/COPD.S296696
9. Marshall, JC, Bosco, L, Adhikari, NK, Connolly, B, Diaz, JV, Dorman, T, et al. What is an intensive care unit? A report of the task force of the world Federation of Societies of intensive and critical care medicine. J Crit Care. (2017) 37:270–6. doi: 10.1016/j.jcrc.2016.07.015
10. Valentin, A, and Ferdinande, P. Recommendations on basic requirements for intensive care units: structural and organizational aspects. Intensive Care Med. (2011) 37:1575–87. doi: 10.1007/s00134-011-2300-7
11. Ward, NS, and Chong, DH. Critical care beds and resource utilization: current trends and controversies. Semin Respir Crit Care Med. (2015) 36:914–20. doi: 10.1055/s-0035-1564876
12. Vincent, JL, Marshall, JC, Namendys-Silva, SA, François, B, Martin-Loeches, I, Lipman, J, et al. Assessment of the worldwide burden of critical illness: the intensive care over nations (ICON) audit. Lancet Respir Med. (2014) 2:380–6. doi: 10.1016/S2213-2600(14)70061-X
13. Halpern, NA, and Pastores, SM. Critical care medicine in the United States 2000-2005: an analysis of bed numbers, occupancy rates, payer mix, and costs. Crit Care Med. (2010) 38:65–71. doi: 10.1097/CCM.0b013e3181b090d0
14. Wu, J, Lin, Y, Li, P, Hu, Y, Zhang, L, and Kong, G. Predicting prolonged length of ICU stay through machine learning. Diagnostics. (2021) 11:2242. doi: 10.3390/diagnostics11122242
15. Verburg, IW, Atashi, A, Eslami, S, Holman, R, Abu-Hanna, A, de Jonge, E, et al. Which models can I use to predict adult ICU length of stay? A Systematic Review. Crit Care Med. (2017) 45:e222–31. doi: 10.1097/CCM.0000000000002054
16. Kilic, M, Yuzkat, N, Soyalp, C, and Gulhas, N. Cost analysis on intensive care unit costs based on the length of stay. Turk J Anaesthesiol Reanim. (2019) 47:142–5. doi: 10.5152/TJAR.2019.80445
17. Herman, C, Karolak, W, Yip, AM, Buth, KJ, Hassan, A, and Legare, JF. Predicting prolonged intensive care unit length of stay in patients undergoing coronary artery bypass surgery—development of an entirely preoperative scorecard. Interact Cardiovasc Thorac Surg. (2009) 9:654–8. doi: 10.1510/icvts.2008.199521
18. Li, M, Cheng, K, Ku, K, Li, J, Hu, H, and Ung, C. Factors influencing the length of hospital stay among patients with chronic obstructive pulmonary disease (COPD) in Macao population: a retrospective study of inpatient health record. Int J Chron Obstruct Pulmon Dis. (2021) 16:1677–85. doi: 10.2147/COPD.S307164
19. Dong, F, Huang, K, Ren, X, Qumu, S, Niu, H, Wang, Y, et al. Factors associated with inpatient length of stay among hospitalised patients with chronic obstructive pulmonary disease, China, 2016-2017: a retrospective study. BMJ Open. (2021) 11:e40560. doi: 10.1136/bmjopen-2020-040560
20. Teixeira, PP, Kowalski, VH, Valduga, K, de Araujo, BE, and Silva, FM. Low muscle mass is a predictor of malnutrition and prolonged hospital stay in patients with acute exacerbation of chronic obstructive pulmonary disease: a longitudinal study. JPEN J Parenter Enteral Nutr. (2021) 45:1221–30. doi: 10.1002/jpen.1998
21. Shay, A, Fulton, JS, and O’Malley, P. Mobility and functional status among hospitalized COPD patients. Clin Nurs Res. (2020) 29:13–20. doi: 10.1177/1054773819836202
22. Ruparel, M, Lopez-Campos, JL, Castro-Acosta, A, Hartl, S, Pozo-Rodriguez, F, and Roberts, CM. Understanding variation in length of hospital stay for COPD exacerbation: European COPD audit. ERJ Open Res. (2016) 2:00034–2015. doi: 10.1183/23120541.00034-2015
23. Tsimogianni, AM, Papiris, SA, Stathopoulos, GT, Manali, ED, Roussos, C, and Kotanidou, A. Predictors of outcome after exacerbation of chronic obstructive pulmonary disease. J Gen Intern Med. (2009) 24:1043–8. doi: 10.1007/s11606-009-1061-2
24. Diaz-Peromingo, JA, Grandes-Ibanez, J, Fandino-Orgeira, JM, Barcala-Villamarin, P, and Garrido-Sanjuan, JA. Predicting factors contributing to length of stay in hospitalized chronic obstructive pulmonary disease (COPD) patients: the role of the emergency room. Acta Med Austriaca. (2004) 47:29–32. doi: 10.14712/18059694.2018.62
25. Yang, L, Li, M, Shu, J, Yang, Y, and Huang, Q. A risk prediction model for prolonged length of stay in patients with acute exacerbations of chronic obstructive pulmonary disease: a retrospective study of 225 patients in a single center in Kunming. China Med Sci Monit. (2022) 28:e934392. doi: 10.12659/MSM.934392
26. Johnson, A, Bulgarelli, L, Shen, L, Gayles, A, Shammout, A, Horng, S, et al. MIMIC-IV, a freely accessible electronic health record dataset. Sci Data. (2023) 10:1. doi: 10.1038/s41597-022-01899-x
27. Yang, J, Li, Y, Liu, Q, Li, L, Feng, A, Wang, T, et al. Brief introduction of medical database and data mining technology in big data era. J Evid Based Med. (2020) 13:57–69. doi: 10.1111/jebm.12373
28. Wu, WT, Li, YJ, Feng, AZ, Li, L, Huang, T, Xu, AD, et al. Data mining in clinical big data: the frequently used databases, steps, and methodological models. Mil Med Res. (2021) 8:44. doi: 10.1186/s40779-021-00338-z
29. Morris, TP, White, IR, and Royston, P. Tuning multiple imputation by predictive mean matching and local residual draws. BMC Med Res Methodol. (2014) 14:75. doi: 10.1186/1471-2288-14-75
30. Collins, GS, Reitsma, JB, Altman, DG, and Moons, KG. Transparent reporting of a multivariable prediction model for individual prognosis or diagnosis (TRIPOD): the TRIPOD statement. BMJ. (2015) 350:g7594. doi: 10.1136/bmj.g7594
31. Hu, JY, Wang, Y, Tong, XM, and Yang, T. When to consider logistic LASSO regression in multivariate analysis? Eur J Surg Oncol. (2021) 47:2206. doi: 10.1016/j.ejso.2021.04.011
32. Lyu, J, Li, Z, Wei, H, Liu, D, Chi, X, Gong, DW, et al. A potent risk model for predicting new-onset acute coronary syndrome in patients with type 2 diabetes mellitus in Northwest China. Acta Diabetol. (2020) 57:705–13. doi: 10.1007/s00592-020-01484-x
33. Mullah, M, Hanley, JA, and Benedetti, A. LASSO type penalized spline regression for binary data. BMC Med Res Methodol. (2021) 21:83. doi: 10.1186/s12874-021-01234-9
34. Lin, Q, Zhao, Z, and Liu, JS. Sparse sliced inverse regression via Lasso. J Am Stat Assoc. (2019) 114:1726–39. doi: 10.1080/01621459.2018.1520115
35. Huang, YC, Huang, SJ, Tsauo, JY, and Ko, WJ. Definition, risk factors and outcome of prolonged surgical intensive care unit stay. Anaesth Intensive Care. (2010) 38:500–5. doi: 10.1177/0310057X1003800314
36. Quintana, JM, Unzurrunzaga, A, Garcia-Gutierrez, S, Gonzalez, N, Lafuente, I, Bare, M, et al. Predictors of hospital length of stay in patients with exacerbations of COPD: a cohort study. J Gen Intern Med. (2015) 30:824–31. doi: 10.1007/s11606-014-3129-x
37. Kim, JH . Multicollinearity and misleading statistical results. Korean J Anesthesiol. (2019) 72:558–69. doi: 10.4097/kja.19087
38. Chan, B . Data Analysis Using R Programming. Adv Exp Med Biol. (2018) 1082:47–122. doi: 10.1007/978-3-319-93791-5_2
39. Balachandran, VP, Gonen, M, Smith, JJ, and DeMatteo, RP. Nomograms in oncology: more than meets the eye. Lancet Oncol. (2015) 16:e173–80. doi: 10.1016/S1470-2045(14)71116-7
40. Liang, G, Chen, X, Zha, X, and Zhang, F. A nomogram to improve predictability of small-incision Lenticule extraction surgery. Med Sci Monit. (2017) 23:5168–75. doi: 10.12659/MSM.904598
41. Taeb, AM, Hooper, MH, and Marik, PE. Sepsis: current definition, pathophysiology, diagnosis, and management. Nutr Clin Pract. (2017) 32:296–308. doi: 10.1177/0884533617695243
42. Vincent, JL, Sakr, Y, Sprung, CL, Ranieri, VM, Reinhart, K, Gerlach, H, et al. Sepsis in European intensive care units: results of the SOAP study. Crit Care Med. (2006) 34:344–53. doi: 10.1097/01.CCM.0000194725.48928.3A
43. Arabi, Y, Venkatesh, S, Haddad, S, Al, SA, and Al, MS. A prospective study of prolonged stay in the intensive care unit: predictors and impact on resource utilization. Int J Qual Health Care. (2002) 14:403–10. doi: 10.1093/intqhc/14.5.403
44. Salomao, R, Ferreira, BL, Salomao, MC, Santos, SS, Azevedo, L, and Brunialti, M. Sepsis: evolving concepts and challenges. Braz J Med Biol Res. (2019) 52:e8595. doi: 10.1590/1414-431x20198595
45. Nash, DM, Przech, S, Wald, R, and O'Reilly, D. Systematic review and meta-analysis of renal replacement therapy modalities for acute kidney injury in the intensive care unit. J Crit Care. (2017) 41:138–44. doi: 10.1016/j.jcrc.2017.05.002
46. Naorungroj, T, Neto, AS, Yanase, F, Eastwood, G, Wald, R, Bagshaw, SM, et al. Time to initiation of renal replacement therapy among critically ill patients with acute kidney injury: a current systematic review and Meta-analysis. Crit Care Med. (2021) 49:e781–92. doi: 10.1097/CCM.0000000000005018
47. Teasdale, G, Maas, A, Lecky, F, Manley, G, Stocchetti, N, and Murray, G. The Glasgow coma scale at 40 years: standing the test of time. Lancet Neurol. (2014) 13:844–54. doi: 10.1016/S1474-4422(14)70120-6
48. Deng, Y, Liu, S, Wang, Z, Wang, Y, Jiang, Y, and Liu, B. Explainable time-series deep learning models for the prediction of mortality, prolonged length of stay and 30-day readmission in intensive care patients. Front Med. (2022) 9:933037. doi: 10.3389/fmed.2022.933037
49. Oostenbrink, JB, Rutten-van, MM, Monz, BU, and FitzGerald, JM. Probabilistic Markov model to assess the cost-effectiveness of bronchodilator therapy in COPD patients in different countries. Value Health. (2005) 8:32–46. doi: 10.1111/j.1524-4733.2005.03086.x
50. Hu, X, De Silva, TM, Chen, J, and Faraci, FM. Cerebral vascular disease and neurovascular injury in ischemic stroke. Circ Res. (2017) 120:449–71. doi: 10.1161/CIRCRESAHA.116.308427
51. Lindner, G, Herschmann, S, Funk, GC, Exadaktylos, AK, Gygli, R, and Ravioli, S. Sodium and potassium disorders in patients with COPD exacerbation presenting to the emergency department. BMC Emerg Med. (2022) 22:49. doi: 10.1186/s12873-022-00607-7
52. Inabnit, LS, Blanchette, C, and Ruban, C. Comorbidities and length of stay in chronic obstructive pulmonary disease patients. COPD. (2018) 15:355–60. doi: 10.1080/15412555.2018.1513470
53. Zampieri, FG, Ladeira, JP, Park, M, Haib, D, Pastore, CL, Santoro, CM, et al. Admission factors associated with prolonged (>14 days) intensive care unit stay. J Crit Care. (2014) 29:60–5. doi: 10.1016/j.jcrc.2013.09.030
54. Reaven, NL, Lovett, JE, and Funk, SE. Brain injury and fever: hospital length of stay and cost outcomes. J Intensive Care Med. (2009) 24:131–9. doi: 10.1177/0885066608330211
55. Diringer, MN, Reaven, NL, Funk, SE, and Uman, GC. Elevated body temperature independently contributes to increased length of stay in neurologic intensive care unit patients. Crit Care Med. (2004) 32:1489–95. doi: 10.1097/01.CCM.0000129484.61912.84
56. Geffroy, A, Bronchard, R, Merckx, P, Seince, PF, Faillot, T, Albaladejo, P, et al. Severe traumatic head injury in adults: which patients are at risk of early hyperthermia? Intensive Care Med. (2004) 30:785–90. doi: 10.1007/s00134-004-2280-y
57. Limsuwat, C, Mankongpaisarnrung, C, Dumrongmongcolgul, N, and Nugent, K. Factors influencing the length of hospital stay in patients with acute exacerbations of chronic obstructive pulmonary disease admitted to intensive care units. Qual Manag Health Care. (2014) 23:86–93. doi: 10.1097/QMH.0000000000000024
58. Rouze, A, Cottereau, A, and Nseir, S. Chronic obstructive pulmonary disease and the risk for ventilator-associated pneumonia. Curr Opin Crit Care. (2014) 20:525–31. doi: 10.1097/MCC.0000000000000123
59. Koulenti, D, Parisella, FR, Xu, E, Lipman, J, and Rello, J. The relationship between ventilator-associated pneumonia and chronic obstructive pulmonary disease: what is the current evidence? Eur J Clin Microbiol Infect Dis. (2019) 38:637–47. doi: 10.1007/s10096-019-03486-2
60. Terzano, C, Colamesta, V, Unim, B, Romani, S, Meneghini, A, Volpe, G, et al. Chronic obstructive pulmonary disease (COPD) exacerbation: impact of comorbidities on length and costs during hospitalization. Eur Rev Med Pharmacol Sci. (2017) 21:3680–9.
61. Gadre, SK, Duggal, A, Mireles-Cabodevila, E, Krishnan, S, Wang, XF, Zell, K, et al. Acute respiratory failure requiring mechanical ventilation in severe chronic obstructive pulmonary disease (COPD). Medicine. (2018) 97:e487. doi: 10.1097/MD.0000000000010487
62. Peng, JC, Gong, WW, Wu, Y, Yan, TY, and Jiang, XY. Development and validation of a prognostic nomogram among patients with acute exacerbation of chronic obstructive pulmonary disease in intensive care unit. BMC Pulm Med. (2022) 22:306. doi: 10.1186/s12890-022-02100-0
63. Ruiz, AJ, Buitrago, G, Rodriguez, N, Gómez, G, Sulo, S, Gómez, C, et al. Clinical and economic outcomes associated with malnutrition in hospitalized patients. Clin Nutr. (2019) 38:1310–6. doi: 10.1016/j.clnu.2018.05.016
64. Bauer, JM, Vogl, T, Wicklein, S, Trogner, J, Muhlberg, W, and Sieber, CC. Comparison of the Mini nutritional assessment, subjective global assessment, and nutritional risk screening (NRS 2002) for nutritional screening and assessment in geriatric hospital patients. Z Gerontol Geriatr. (2005) 38:322–7. doi: 10.1007/s00391-005-0331-9
Keywords: chronic obstructive pulmonary disease, intensive care unit, length of stay, nomograms, prolonged intensive care unit stays
Citation: Cheng H, Li J, Wei F, Yang X, Yuan S, Huang X, Zhou F and Lyu J (2023) A risk nomogram for predicting prolonged intensive care unit stays in patients with chronic obstructive pulmonary disease. Front. Med. 10:1177786. doi: 10.3389/fmed.2023.1177786
Received: 02 March 2023; Accepted: 15 June 2023;
Published: 06 July 2023.
Edited by:
Justin Garner, Royal Brompton Hospital, United KingdomReviewed by:
Ali Alagoz, University of Health Sciences, TürkiyeCopyright © 2023 Cheng, Li, Wei, Yang, Yuan, Huang, Zhou and Lyu. This is an open-access article distributed under the terms of the Creative Commons Attribution License (CC BY). The use, distribution or reproduction in other forums is permitted, provided the original author(s) and the copyright owner(s) are credited and that the original publication in this journal is cited, in accordance with accepted academic practice. No use, distribution or reproduction is permitted which does not comply with these terms.
*Correspondence: Fuling Zhou, emhvdWZ1bGluZ0B3aHUuZWR1LmNu; Jun Lyu, bHl1anVuMjAyMEBqbnUuZWR1LmNu
†These authors have contributed equally to this work
Disclaimer: All claims expressed in this article are solely those of the authors and do not necessarily represent those of their affiliated organizations, or those of the publisher, the editors and the reviewers. Any product that may be evaluated in this article or claim that may be made by its manufacturer is not guaranteed or endorsed by the publisher.
Research integrity at Frontiers
Learn more about the work of our research integrity team to safeguard the quality of each article we publish.