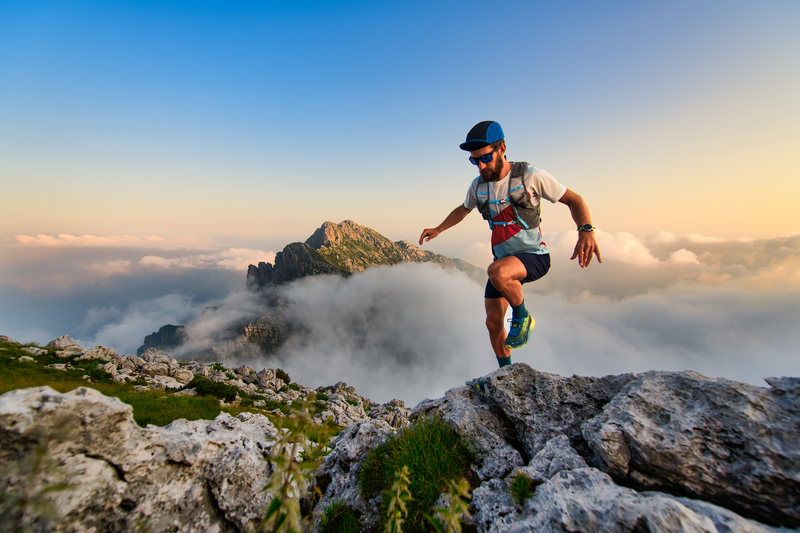
95% of researchers rate our articles as excellent or good
Learn more about the work of our research integrity team to safeguard the quality of each article we publish.
Find out more
ORIGINAL RESEARCH article
Front. Med. , 26 May 2023
Sec. Translational Medicine
Volume 10 - 2023 | https://doi.org/10.3389/fmed.2023.1175483
This article is part of the Research Topic The Bidirectional Relationship between Sleep and Neuroendocrinology View all 7 articles
Objectives: Poor sleep behavior can trigger an inflammatory response and contribute to the development of inflammatory diseases. Cytokines can act as indicators of inflammation and may precede the onset of inflammatory diseases. This study aimed to determine the association between sleep timing parameters (bedtime, sleep duration, sleep debt, and social jetlag) and the levels of nine serum and salivary inflammatory and metabolic biomarkers.
Methods: Data were collected from 352 adolescents aged 16–19 years enrolled in Kuwait’s public high schools. The levels of C-reactive protein (CRP), interleukin-6 (IL-6), interleukin-8 (IL-8), interleukin-10 (IL-10), vascular endothelial growth factor (VEGF), monocyte chemoattractant protein-1 (MCP-1), adiponectin, leptin, and insulin were measured from saliva and serum samples. We conducted mixed-effect multiple linear regression modeling to account for the school variable as a random effect to assess the relationship between the sleep variables and salivary and serum biomarkers. Mediation analysis was conducted to check if BMI was a mediator between bedtime and the biomarkers.
Results: There was a statistically significant elevation in serum IL-6 level associated with later bedtime (0.05 pg./mL, p = 0.01). Adolescents with severe sleep debt of ≥2 h had an increase in salivary IL-6 biomarker levels (0.38 pg./mL, p = 0.01) compared to those who had sleep debt of <1 h. Adolescents with sleep debt of ≥2 h had significantly higher levels of serum CRP (0.61 μg/mL, p = 0.02) than those without sleep debt. Additionally, we found that the inflammatory biomarkers (CRP, IL-6, IL-8, IL-10, VEGF, and MCP-1) and metabolic biomarkers (adiponectin, leptin, and insulin) had more statistically significant associations with the bedtime variables than with sleep duration variables. CRP, IL-6, and IL-8 were associated with sleep debt, and IL-6, VEGF, adiponectin, and leptin levels were associated with social jetlag. BMIz was a full mediator in the relationship between late bedtime and increased serum levels of CRP, IL-6, and insulin.
Conclusion: Adolescents who go to bed at or later than midnight had dysregulated levels of salivary and serum inflammatory biomarkers, suggesting that disrupted circadian rhythm can trigger higher levels of systemic inflammation and potentially exacerbate chronic inflammation and the risk of metabolic diseases.
Sleep and lack of sleep have been reported to play a role in immune processes, such as cellular immune activation and nocturnal and functional secretions of inflammatory biomarkers (1). An inflammatory reaction precedes the development of the metabolic diseases, and certain inflammatory biomarkers can be targeted to prevent the development of inflammatory conditions and organ damage (2). Since biomarker levels are critical in the development of persistent inflammation in certain conditions, it is vital to explore their application as indicators of disease risks and severities and as prognostic markers. There is established evidence confirming a causal relationship between disrupted sleep, circadian rhythm, and the promotion of inflammatory conditions (3). Evaluating the correlation between inflammatory biomarkers and various sleep measurements could help identify which sleep behaviors to target to improve and regulate circadian rhythm, thereby preventing inflammation. The circadian rhythm is significantly influenced by the light–dark cycle and bedtime.
Numerous studies have investigated the relationship between various sleep parameters and inflammatory biomarkers, yielding inconsistent results (4–9). However, a meta-analysis of 72 studies with a combined sample size exceeding 50,000 participants has provided more consistent findings. This analysis revealed that sleep disturbance was associated with higher levels of C-reactive protein (CRP) and interleukin-6 (IL-6), while shorter sleep duration was associated with elevated CRP levels but not IL-6 (7).While most studies have focused on adults, a limited number of investigations have been conducted in adolescents. Some of these studies found a consistent positive relationship between CRP and poor sleep behavior in adolescents, although the association with IL-6 was not always observed in adolescents. These studies attributed the lack of association with IL-6 to the potential masking effect of adjusting for body mass index (BMI) (10). Grandner et al. (4) have proposed several reasons for the conflicting results between sleep and both CRP and IL-6, including type 2 errors (lack of findings despite the presence of a true association). These reasons encompass inconsistent definitions of sleep behaviors, heterogeneity of sleep measurements, inadequate sample sizes, insufficient stratification, selection bias, and inadequate control for confounding and mediating factors (4). Notably, an intervention study on adolescents demonstrated that lower baseline IL-6 and CRP levels were associated with improved treatment responses for behavioral and physical health outcomes following intervention (11). To the best of our knowledge, no studies have investigated the relationship between inflammatory biomarkers in adolescents in the Middle East. Identifying these biomarkers in this our population can provide valuable insights into the development of inflammatory conditions during adolescence. Adolescence is a critical developmental period associated with both significant risks and opportunities for establishing healthy behaviors. The present study aims to validate previous findings regarding selected inflammatory markers associated with poor sleep behavior, with the potential to inform future interventions by identifying biomarkers to measure and sleep behaviors to target.
This study aimed to evaluate the relationship between nine salivary and serum biomarkers such as interleukin (IL)-6, IL-8, IL-10, leptin, C-reactive protein (CRP), insulin, vascular endothelial growth factor (VEGF), and monocyte chemoattractant protein-1 (MCP-1), and different validated sleep parameters such as sleep duration, bedtime, sleep debt, and social jetlag in Kuwaiti adolescents. These biomarkers are implicated in multiple chronic conditions associated with inflammation, such as metabolic syndrome, rheumatoid arthritis, coronary artery disease, inflammatory bowel disease, and cancer (12–14).
This study was a part of a larger cohort study that aimed to evaluate the relationship between salivary biomarkers and hyperglycemia and obesity in adolescents (15). Therefore, the selection of biomarkers was based on their known association with diabetes and other inflammatory conditions.
Furthermore, it is important to acknowledge that the composition of human saliva is influenced by both local and systemic factors. Therefore, we collected saliva samples, taking into account the potential association between sleep and local inflammation (16).
As we investigate the link between sleep and inflammatory biomarkers, we cannot overlook the importance of bodyweight measurements, such as BMI. A wealth of evidence has demonstrated that insufficient sleep is associated with an increased BMI, and higher BMI is linked to inflammatory conditions (17). In this study, we also aimed to evaluate the mediation effect of BMI in the relationship between inflammatory biomarkers and bedtime.
In March and April 2019, we collected data from 352 adolescents who were 16–19 years old and enrolled in Kuwait’s public high schools. The participants for this cross-sectional multi-site study were drawn from Kuwait’s Capital and Hawali governorates.
This study was part of a larger cohort study that aimed to evaluate the relationship between salivary biomarkers and hyperglycemia and obesity in adolescents (15).
The inclusion criteria for this study were senior male and female students enrolled in public high schools in the state of Kuwait who provided their consent to participate. A convenient sampling method was employed to select participants, and a total of 352 children were included in the study based on the allocated budget. The convenience sampling approach was adopted as it depended on the willingness of schools to host the research team for data collection.
In this study, students with medical conditions were not excluded; however, adjustments were made for these conditions during the analysis.
Responses were collected from students using the Qualtrics program (River Park Drive, Provo, UT 84604, United States), on individual iPads (Apple, Cupertino, CA).
This study was conducted during the academic year, and as such, the sleep behavior responses reflected the patterns of the school year. Additionally, data collection took place over a 2-month period, meaning there was no seasonal variation that could have impacted sleep timing.
This study was approved by the Dasman Diabetes Institute Human Ethical Review Committee in Kuwait and the Ministry of Health in Kuwait. We obtained informed consent from the parents or legal guardians and assent form from the adolescents participating in the study at the outset.
All procedures performed in studies involving human participants were as per the institutional and/or national research committee’s ethical standards.
Salivary and serum biomarker levels were the primary dependent continuous variables in this study. Saliva samples were obtained from each participant during the academic year at schools early in the morning before breakfast, after an overnight fast (≥12 h). Salivary collection methodology has been described in an earlier study (18), where subjects were instructed to rinse their mouth with water and to provide 4 mL of an unstimulated whole saliva sample into a conical tube placed in a cup of crushed ice. The samples were processed and centrifuged at 2800 rpm for 20 min at 4°C at the Dasman Biobank and Biochemistry and Molecular Biology Department, Dasman Diabetes Institute Laboratories, Kuwait. Then, supernatants were transferred to a screwcap 2D barcoded storage tube (Thermo Scientific), read using a barcode reader (Thermo Scientific VisionMate™ ST), and frozen at −80°C. The analyzes were conducted at the Forsyth Institute in Cambridge, MA, United States.
Serum samples were collected in 7.5 mL of red and black marbled topped tubes with clot activator (SST tubes) from 333 subjects. After collection, the samples were centrifuged at 3000 rpm for 15 min at room temperature, then pipetted into the Eppendorf tubes and stored at −80°C. The frozen samples were transported to the Forsyth Institute under temperature-monitored dry ice. The samples were thawed at 4°C overnight and kept on ice throughout the assay procedure. Biomarker levels were measured in 25 μL of saliva and serum supernatants using multiplex magnetic bead panels on a Luminex 200™ system (Luminex, Austin, TX). Results were evaluated using Bio-Plex Manager™ (Version 5.0; Bio-Rad, Hercules, CA).
The study was conducted during the academic year, so that the sleep timing responses could reflect the school year patterns.
SDWday was calculated by counting the number of hours from the bedtime to the time of waking up on weekdays. The two validated questions (19) used were as follows: “During the past week, at what time did you go to bed on weekdays?” and “During the past week, at what time did you wake up on weekdays?” Data included continuous variables. SDWend was also calculated using the same abovementioned validated questions but for weekends.
This variable was calculated by measuring the average sleep hours per week using the following formula: [(sleep duration weekday × 5) + (sleep duration weekend × 2)]/7 (20). This created a continuous variable.
A bedtime variable was used to evaluate the students’ sleep behavior using the question: “During the past week, at what time did you go to bed on weekdays/weekends?” This created a continuous variable representing bedtime in hours. We used the 24-h clock to record bedtimes (e.g., we coded a bedtime of 1:00 AM as 13:00 and 2:00 AM as 14:00), so that larger values indicated later bedtimes. We categorized bedtime into the following categories: before midnight (<12:00 AM), (from 12:00 to 1:00 AM), and (>1:00 AM).
The BTAverage was calculated using the following formula: [(bedtime weekday × 5) + (bedtime weekend × 2)]/7, creating a continuous variable (21). We further investigated BTAverage as a categorical variable and categorized it into the following: (<12:00 AM), (from 12:00 to 1:00 AM), and (>1:00 AM).
Social jetlag was calculated using the following formula: SJL = bedtime weekends – bedtime weekdays (21). We created a continuous variable in hours, which was dichotomized based on the median as our cut-off value to classify the participants into two groups, with either absence (<1.5 h of SJL) or presence (≥1.5 h) of SJL (22).
Sleep debt was calculated using the formula: SD = SDWend − SDWday. And it was defined as a difference of ≥60 min (23). Severe sleep debt is a difference of ≥90 min (24), but in our study we counted severe sleep debt as >120 min as we had the duration variables in hours and not in minutes.
Nap was a binary variable that captured the habit of taking a nap in the afternoon. The study population was dichotomized into those who napped and those who did not.
Obesity was also analyzed as an independent binary variable, and participants were classified as obese or non-obese adolescents according to the WHO criteria (25). A stadiometer was used to measure height, and a calibrated digital scale was used to measure weight. BMI-for-sex/age z-score (BMIz) was calculated using WHO guidelines (25). Obese subjects had a z-score of ≥2 standard deviations (SD) units from the mean. Overweight subjects had 2 > z-scores ≥1 SD unit above the mean. Normal healthy weight was defined as values of ±1 SD unit. Underweight individuals were defined as those weighing ≤1 SD unit below the mean (25).
Data on age, sex, medical history, dietary habits, and screen time were self-reported. Screen-time was divided into adolescents who engaged in screen-based activities ≤2 h per day vs. those >2 h a day, and it includes the use of computers or smart devices and video games.
A one-sample Kolmogorov–Smirnov test was conducted to test data normality. We transformed the skewed variables using the log 10 function. We conducted mixed-effect linear regression analyzes to adjust for school variable as a random effect and test the association of nine salivary and serum immunometabolic biomarkers as continuous outcome variables with all the sleep timing variables (SDWday, SDWend, SDAverge, BTWday, BTWend, BTAverage, SJL and SD), after adjusting for age, sex, dietary habits, screen time, napping, and medical history. Sex, age, and medical history are essential demographic variables that may influence the relationship between our measured outcomes and exposures. Additionally, nap, screen time, and dietary habits are potential confounding variables that could be associated with the outcomes and exposures, further elucidating their relationship.
To examine the mechanisms by which bedtime behavior influences biomarkers levels, we investigated BMI as a mediator in the associations between BTAverage and the nine salivary and serum biomarkers. Mediation analysis was conducted using structural equation modeling (SEM) (26). Analyzes included the 5,000-bootstrap method of resampling to estimate the confidence intervals for total, direct, and indirect effects. Goodness-of-fit tests were also conducted. Models were tested for multicollinearity. Models were tested for multicollinearity issues and exhibited Variance Inflation Factor (VIF) values of less than 1.6, supporting the absence of multicollinearity among parameters used as predictors. Statistical analyzes were performed on Stata/SE 15.1 (StataCorp, College Station, TX) statistical software. Results for the regression were reported using beta coefficient (β), and their 95% confidence intervals, with 0.05 significance level.
Table 1 shows the participants’ frequency and percent for BTWday, BTWend, and BTAverage, Table 2 presents their characteristics both stratified by average bedtime. Table 3 shows the biomarkers levels stratified by bedtime variables. Table 4 presents the mixed effect linear analysis examining the association between sleep timing variables (sleep duration and bedtime) with salivary and serum immunometabolic biomarkers, adjusting for age, sex, medical history, screen time, napping, and dietary habits. The mean age of adolescents was 17 ± 0.59 years. We found that for every one-hour reduction in SDWDay and SDAverage, the levels of salivary and serum IL-6 increased. There was a statistically significant association between salivary IL-6 level and SDWday (p = 0.02) and between serum IL-6 level and SDWday (p = 0.04) and SDAverage (p = 0.02).
Table 1. Descriptive summary of participants frequency and percentage stratified by bedtime as a categorical variable.
Table 2. Descriptive summary of population characteristics stratified by average bedtime on weekdays and weekends.
Table 3. Descriptive summary of population characteristics, biomarker levels, and sleep variables stratified by average bedtime (before 12:00 AM, 12:00–1:00 AM and, After 1:00 AM).
Table 4. Mixed effect linear regression analysis for the association between sleep timing variables (sleep duration and bedtime) and salivary and serum immunometabolic biomarkers.
Additionally, adjusting for confounders, we demonstrated that for every one-hour increase in BTWend (later bedtime), there was a statistically significant reduction in salivary IL-6 level (−0.11 pg./mL, p = 0.02). As for serum biomarkers, there was a statistically significant elevation in serum IL-6 level associated with BTAverage (0.05 pg./mL, p = 0.01), BTWday (0.04 pg./mL, p = 0.04), and BTWend (0.06 pg./mL, p = 0.003).
Table 5 shows the association of biomarkers with categorical average bedtime on weekdays and weekends (BTAverage). There was a significant elevation in salivary IL-6 level (0.44 pg./mL, p = 0.002) among participants who went to bed ‘from 12:00 to 1:00 AM’ compared to those who went to bed earlier, ‘before 12:00 AM’.
Table 5. Mixed effect linear analysis for the relationship between salivary and serum immunometabolic biomarkers and bedtime for average bedtime as categorical variable.
Table 6 shows the association of biomarkers with other sleep variables (sleep debt and social jetlag). Adolescents with a sleep debt of ≥1 h and a severe sleep debt of ≥2 h had an increase in salivary IL-6 biomarker levels (0.35 pg./mL, p = 0.04; 0.38 pg./mL, p = 0.01), respectively, compared to those who had sleep debt of <1 h. Participants with a sleep debt of ≥1 h had significantly higher levels of serum IL-6 (0.17 pg./mL, p = 0.03) than those without sleep debt or those with sleep debt of <1 h. Students with sleep debt of ≥2 h had significantly higher levels of serum CRP (0.61 μg/mL, p = 0.02) than those without sleep debt or those with sleep debt of <2 h.
Table 6. Mixed effect linear regression analysis for the relationship between salivary and serum immunometabolic biomarkers and the sleep variables, social jetlag and sleep debt.
Only salivary biomarkers showed significant associations with SJL. For every one-hour increase in social jetlag, there was an overall reduction in the following salivary biomarkers IL-6 (−0.14 pg./mL, p < 0.001), adiponectin (−0.03 pg./mL, p = 0.03), and VEGF (−27 pg./mL, p = 0.04). We further investigated the effect of social jetlag with a median cut-off point of 1.5 h of social jetlag. Adolescents with a social jetlag of ≥1.5 h had a reduction in salivary IL-6 (−0.47 pg./mL, p < 0.001) and leptin (−0.06 pg./mL, p = 0.04) levels compared to that no social jetlag or those with a social jetlag of <1.5 h.
Table 7 shows the results of the components of the mediation analysis using the SEM technique. We showed consistent evidence of full mediation by BMI for serum CRP, IL-6, and insulin levels.
Table 7. Mediation effect of BMI on the association between serum and salivary biomarkers and average bedtime.
We assessed multiple sleep parameters, including bedtime, sleep duration, sleep debt, and social jetlag. We observed that the levels of salivary IL-6 and serum IL-6, IL-10, MCP-1, and insulin increase with delayed bedtime. We also found that serum IL-6 level was associated with sleep debt, and that salivary IL-6 level is associated with SJL.
Sleep debt is defined as the difference in sleep duration between weekdays and weekends. Increased sleep debt have a regulatory effect in controlling low-grade systemic inflammation among adults with sleep deprivation on weekdays (27).
Adolescents who had longer weekend catch-up sleep (sleep debt) had higher levels of salivary IL-6 and serum CRP and IL-6. The relationship between increased sleep debt and higher levels of CRP could be explained by multiple factors. First, delayed night sleep can trigger inflammation in different body organs owing to hormonal disruption (28, 29), and night sleep deprivation suppresses immunity, as observed by increased levels of cytokine inflammatory markers (30). Second, the level of CRP is higher in children with obesity (31), and chronic inflammation is a characteristic of obesity, as adipocytes trigger metabolic signals that induce inflammation as indexed by increases in the levels of CRP and other inflammatory markers such as IL6 with disruption of sleep (32).
Furthermore, the evening chronotype (natural tendency to go to bed and wake up later) leads to the accumulation of sleep debt, which is later recovered on weekends, leading to circadian disruption during the week (33) and creating what is called ‘social jetlag’, the most frequent form of circadian rhythm misalignment. Recent studies have investigated social jetlag as a potential risk factor for the development of metabolic diseases (34, 35).
Social jetlag phenomenon occurs when an individual’s sleep and wake times during the workweek differ significantly from those on their days off or during weekend, causing a misalignment of the internal clock with the external environment. This can lead to symptoms similar to those of jet lag, such as fatigue, mood disturbances, and impaired cognitive performance, and potentially chronic inflammatory diseases.
Students with a social jetlag of ≥1.5 h had lower salivary and serum VEGF levels. Salivary VEGF is not an exudate from the bloodstream; in humans, it is secreted from the parotid glands (36–38). Several studies in adults showed that sleep restriction among adults is associated with a higher risk of oral inflammatory disease, such as periodontitis (39, 40). Two studies found that higher secretion of salivary VEGF is prevalent in healthy individuals, and that lower secretion is prevalent in those with periodontitis (41, 42). These results suggest the protective nature of salivary VEGF in maintaining healthy oral mucosa and periodontal tissues (41).
The increase in salivary IL-6 levels noted with multiple sleep parameters in our study may reflect localized oral inflammation (39, 43). Our earlier prospective longitudinal study of Kuwaiti children showed that inadequate sleep can induce hyperglycemia, leading to gingivitis, which may progress to periodontitis over time (40).
Further, our results showed an overall increase in serum insulin is associated with later bedtimes. Evidence shows that sleep deprivation triggers an increase in insulin resistance (44). Insulin resistance develops owing to high levels of insulin secretion, which leads to the development of type 2 diabetes. A strong body of evidence shows a positive association between insulin and sleep deprivation independent of age, sex, ethnicity, BMI, and physical activity (45).
Further examination of BMI in the mediation analysis showed that increased BMI was a full mediator in the relationship between increased CRP, IL-6, and insulin levels and bedtime. Although several studies have addressed the associations between higher levels of inflammatory cytokines and the risk of increased bodyweight, to our knowledge, this is the first study to examine the mediation effect of increased BMI in the relationship between sleep timing and systemic inflammation as indexed by inflammatory biomarkers. We showed that later bedtime was associated with IL6, IL8, insulin, CRP, and MCP-1, and short sleep duration was only associated with IL6. Being a full mediator, increased BMI is involved in the causal pathway that can trigger inflammation and subsequent comorbidities.
Studies show that disrupted circadian rhythms in adolescents increased the levels of inflammatory cytokines and can predict metabolic syndrome (46). Circadian disruption (47, 48) has received increasing research attention. Circadian rhythms are critical for the optimal function of the immune system, and their disruption can affect host immune responses and increase susceptibility and development of pathology (49). Circadian rhythms are regulated by the sleep–wake and dark–light cycles. At the molecular level, circadian rhythms are driven by the molecular circadian clock, which is found in nearly every cell in the body (50). Circadian rhythms are mainly disrupted by irregular sleep–wake schedules and other environmental factors, such as light exposure at night and eating food late at night, resulting in cellular and organ dysfunction, including metabolic pathology (50). Understanding the interaction between circadian rhythms manifested by poor sleep behavior and systemic inflammation could provide new insights for intervention to improve lifestyle and prevent consequences of metabolic syndrome.
This study had some limitations. Owing to the cross-sectional design, we were unable to infer a causal relationship. We did not utilize randomized sample recruitment in this study, which may leave it vulnerable to selection bias. Additionally, the data on sleep duration were self-reported; hence, information was susceptible to recall bias.
Another limitation of this study is that we did not collect data on insomnia and sleep-disordered breathing and local inflammation, which could have played roles in the levels of inflammatory salivary biomarkers.
We observed that the levels of salivary IL-6 and serum IL-6, IL-10, MCP-1, and insulin increase with delayed bedtime. Adolescents who had longer weekend catch-up sleep had higher levels of salivary IL-6 and serum CRP and IL-6. These changes in biomarker levels with sleep timing behavior support the hypothesis that late bedtime and disrupted circadian rhythm promote local and systemic inflammation that can trigger chronic metabolic diseases.
In conclusion, while conventional wisdom has emphasized the role of diet and physical activity in preventing inflammatory and metabolic diseases, emerging studies suggest that improving sleep may also be a valuable approach. Although controlling diet and physical activity can be challenging, addressing sleep behaviors holds promise for mitigating inflammation and reducing the risk of metabolic diseases. The present study serves as a crucial preliminary investigation in identifying specific sleep behaviors to target and inflammatory biomarkers to measure, thereby offering potential strategies for improving health outcomes in this population. Further research in this area is warranted to develop comprehensive interventions that encompass sleep as a key component in disease prevention and management. By recognizing the importance of sleep in promoting health and preventing inflammation and metabolic diseases, healthcare providers and individuals alike can prioritize sleep as a valuable aspect of overall well-being.
The raw data supporting the conclusions of this article will be made available by the authors, without undue reservation.
This study was approved by the Dasman Diabetes Institute Human Ethical Review Committee in Kuwait and the Ministry of Health in Kuwait. We obtained informed consent from the parents or legal guardians and assent form from the adolescents participating in the study at the outset. All procedures performed in studies involving human participants were as per the institutional and/or national research committee’s ethical standards. Written informed consent to participate in this study was provided by the participants’ legal guardian/next of kin.
HA and AA contributed to writing the original draft of the manuscript, data analysis, and data interpretation. SB-H contributed to the study methodology, data interpretation, and critical revision of the manuscript. FA-M, MA, SD, and JA contributed to the data collection, project administration, and they revised the manuscript. MT contributed to the conception of the study, study methodology, and critically revised the manuscript. NR contributed to the data analysis and data interpretation. MF contributed to the data analysis and critical revision of the manuscript. All authors provided final approval and agreed to be accountable for all aspects of the work.
This study was funded by the Dasman Diabetes Institute, Kuwait (Grant # RA/065/2011 and RA/005/2011); the Kuwait Foundation for the Advancement of Sciences, Kuwait (Grant # PR19-13MM-01); and the Forsyth Institute, Cambridge, MA, United States (Grant # FSI-CP02). This work would not have been possible without the support of the Kuwait School Oral Health Program, Ministry of Health in Kuwait.
The authors are especially thankful to Fatima Alwahaib and Sultan Hanna for their assistance in accessing and coordinating with the school administrations for the data collection. They would also like to thank all the parents and their children who participated in the study.
The authors declare that the research was conducted in the absence of any commercial or financial relationships that could be construed as a potential conflict of interest.
All claims expressed in this article are solely those of the authors and do not necessarily represent those of their affiliated organizations, or those of the publisher, the editors and the reviewers. Any product that may be evaluated in this article, or claim that may be made by its manufacturer, is not guaranteed or endorsed by the publisher.
The Supplementary material for this article can be found online at: https://www.frontiersin.org/articles/10.3389/fmed.2023.1175483/full#supplementary-material
1. Irwin, M. Effects of sleep and sleep loss on immunity and cytokines. Brain Behav Immun. (2002) 16:503–12. doi: 10.1016/s0889-1591(02)00003-x
2. Chase, HP, Cooper, S, Osberg, I, Stene, LC, Barriga, K, Norris, J, et al. Elevated C-reactive protein levels in the development of type 1 diabetes. Diabetes. (2004) 53:2569–73. doi: 10.2337/diabetes.53.10.2569
3. Kizaki, T, Sato, S, Shirato, K, Sakurai, T, Ogasawara, J, Izawa, T, et al. Effect of circadian rhythm on clinical and pathophysiological conditions and inflammation. Crit Rev Immunol. (2015) 35:261–75. doi: 10.1615/CritRevImmunol.2015014925
4. Grandner, MA, Sands-Lincoln, MR, Pak, VM, and Garland, SN. Sleep duration, cardiovascular disease, and proinflammatory biomarkers. Nat Sci Sleep. (2013) 5:93–107. doi: 10.2147/NSS.S31063
5. Nowakowski, S, Matthews, KA, von Känel, R, Hall, MH, and Thurston, RC. Sleep characteristics and inflammatory biomarkers among midlife women. Sleep. (2018) 41:zsy049. doi: 10.1093/sleep/zsy049
6. Chiang, J-K. Short duration of sleep is associated with elevated high-sensitivity C-reactive protein level in Taiwanese adults: a cross-sectional study. J Clin Sleep Med. (2014) 10:743–9. doi: 10.5664/jcsm.3862
7. Irwin, MR, Olmstead, R, and Carroll, JE. Sleep disturbance, sleep duration, and inflammation: a systematic review and meta-analysis of cohort studies and experimental sleep deprivation. Biol Psychiatry. (2016) 80:40–52. doi: 10.1016/j.biopsych.2015.05.014
8. Dzierzewski, JM, Donovan, EK, Kay, DB, Sannes, TS, and Bradbrook, KE. Sleep inconsistency and markers of inflammation. Front Neurol. (2020) 11:1042. doi: 10.3389/fneur.2020.01042
9. Leng, Y, Ahmadi-Abhari, S, Wainwright, NW, Cappuccio, FP, Surtees, PG, Luben, R, et al. Daytime napping, sleep duration and serum C reactive protein: a population-based cohort study. BMJ Open. (2014) 4:e006071. doi: 10.1136/bmjopen-2014-006071
10. Uy, JP, Dieffenbach, M, Leschak, CJ, Eisenberger, NI, Fuligni, AJ, and Galván, A. Sleep duration moderates the associations between immune markers and corticolimbic function during stress in adolescents. Neuropsychologia. (2022) 176:108374. doi: 10.1016/j.neuropsychologia.2022.108374
11. Dolsen, EA, and Harvey, AG. IL-6, sTNF-R2, and CRP in the context of sleep, circadian preference, and health in adolescents with eveningness chronotype: cross-sectional and longitudinal treatment effects. Psychoneuroendocrinology. (2021) 129:105241. doi: 10.1016/j.psyneuen.2021.105241
12. Gabay, C. Interleukin-6 and chronic inflammation. Arthritis Res Ther. (2006) 8 Suppl 2:S3. doi: 10.1186/ar1917
13. Kaczynski, T, Wronski, J, Gluszko, P, Kryczka, T, Miskiewicz, A, Górski, B, et al. Salivary interleukin 6, interleukin 8, interleukin 17A, and tumour necrosis factor alpha levels in patients with periodontitis and rheumatoid arthritis. Cent Eur J Immunol. (2019) 44:269–76. doi: 10.5114/ceji.2019.89601
14. Maggio, M, Guralnik, JM, Longo, DL, and Ferrucci, L. Interleukin-6 in aging and chronic disease: a magnificent pathway. J Gerontol A Biol Sci Med Sci. (2006) 61:575–84. doi: 10.1093/gerona/61.6.575
15. Alqaderi, H, Hegazi, F, Al-Mulla, F, Chiu, CJ, Kantarci, A, Al-Ozairi, E, et al. Salivary biomarkers as predictors of obesity and intermediate hyperglycemia in adolescents. Front. Public Health. (2022) 10:10. doi: 10.3389/fpubh.2022.800373
16. Zhang, CZ, Cheng, XQ, Li, JY, Zhang, P, Yi, P, Xu, X, et al. Saliva in the diagnosis of diseases. Int J Oral Sci. (2016) 8:133–7. doi: 10.1038/ijos.2016.38
17. Miller, MA, and Cappuccio, FP. Inflammation, sleep, obesity and cardiovascular disease. Curr Vasc Pharmacol. (2007) 5:93–102. doi: 10.2174/157016107780368280
18. Goodson, JM, Kantarci, A, Hartman, ML, Denis, GV, Stephens, D, Hasturk, H, et al. Metabolic disease risk in children by salivary biomarker analysis. PLoS One. (2014) 9:e98799. doi: 10.1371/journal.pone.0098799
19. Nascimento-Ferreira, MV, Collese, TS, de Moraes, ACF, Rendo-Urteaga, T, Moreno, LA, and Carvalho, HB. Validity and reliability of sleep time questionnaires in children and adolescents: a systematic review and meta-analysis. Sleep Med Rev. (2016) 30:85–96. doi: 10.1016/j.smrv.2015.11.006
20. Abreu, GA, Silva, T, Teixeira, LR, and Bloch, KV. Quality analysis of self-reported information on sleep duration in schoolchildren in the study of cardiovascular risk factors in adolescents (ERICA). Cad Saude Publica. (2019) 35:e00152918. doi: 10.1590/0102-311X00152918
21. Jankowski, KS. Social jet lag: sleep-corrected formula. Chronobiol Int. (2017) 34:531–5. doi: 10.1080/07420528.2017.1299162
22. Sudy, AR, Ella, K, Bodizs, R, and Kaldi, K. Association of Social Jetlag with Sleep Quality and Autonomic Cardiac Control during Sleep in Young healthy men. Front Neurosci. (2019) 13:950. doi: 10.3389/fnins.2019.00950
23. Roenneberg, T, Kuehnle, T, Juda, M, Kantermann, T, Allebrandt, K, Gordijn, M, et al. Epidemiology of the human circadian clock. Sleep Med Rev. (2007) 11:429–38. doi: 10.1016/j.smrv.2007.07.005
24. Bin, YS, Marshall, NS, and Glozier, N. Sleeping at the limits: the changing prevalence of short and long sleep durations in 10 countries. Am J Epidemiol. (2013) 177:826–33. doi: 10.1093/aje/kws308
25. De Onis, M, and Lobstein, T. Defining obesity risk status in the general childhood population: Which cut-offs should we use? 5. New York: Taylor & Francis, (2010). pp. 458–460.
26. Gunzler, D, Chen, T, Wu, P, and Zhang, H. Introduction to mediation analysis with structural equation modeling. Shanghai Arch Psychiatry. (2013) 25:390–4. doi: 10.3969/j.issn.1002-0829.2013.06.009
27. Han, KM, Lee, HJ, Kim, L, and Yoon, HK. Association between weekend catch-up sleep and high-sensitivity C-reactive protein levels in adults: a population-based study. Sleep. (2020) 43:zsaa010. doi: 10.1093/sleep/zsaa010
28. Hall, MH, Lee, L, and Matthews, KA. Sleep duration during the school week is associated with C-reactive protein risk groups in healthy adolescents. Sleep Med. (2015) 16:73–8. doi: 10.1016/j.sleep.2014.10.005
29. Yu, JH, Yun, C-H, Ahn, JH, Suh, S, Cho, HJ, Lee, SK, et al. Evening chronotype is associated with metabolic disorders and body composition in middle-aged adults. J Clin Endocrinol Metabol. (2015) 100:1494–502. doi: 10.1210/jc.2014-3754
30. Irwin, MR. Why sleep is important for health: a psychoneuroimmunology perspective. Annu Rev Psychol. (2015) 66:143–72. doi: 10.1146/annurev-psych-010213-115205
31. Todendi, PF, Possuelo, LG, Klinger, EI, Reuter, CP, Burgos, MS, Moura, DJ, et al. Low-grade inflammation markers in children and adolescents: influence of anthropometric characteristics and CRP and IL6 polymorphisms. Cytokine. (2016) 88:177–83. doi: 10.1016/j.cyto.2016.09.007
32. Gregor, MF, and Hotamisligil, GS. Inflammatory mechanisms in obesity. Annu Rev Immunol. (2011) 29:415–45. doi: 10.1146/annurev-immunol-031210-101322
33. Roenneberg, T, Allebrandt, KV, Merrow, M, and Vetter, C. Social jetlag and obesity. Curr Biol. (2012) 22:939–43. doi: 10.1016/j.cub.2012.03.038
34. Feupe, SF, Frias, PF, Mednick, SC, McDevitt, EA, and Heintzman, ND. Nocturnal continuous glucose and sleep stage data in adults with type 1 diabetes in real-world conditions. J Diabetes Sci Technol. (2013) 7:1337–45. doi: 10.1177/193229681300700525
35. Reutrakul, S, Thakkinstian, A, Anothaisintawee, T, Chontong, S, Borel, AL, Perfect, MM, et al. Sleep characteristics in type 1 diabetes and associations with glycemic control: systematic review and meta-analysis. Sleep Med. (2016) 23:26–45. doi: 10.1016/j.sleep.2016.03.019
36. Booth, V, Young, S, Cruchley, A, Taichman, NS, and Paleolog, E. Vascular endothelial growth factor in human periodontal disease. J Periodontal Res. (1998) 33:491–9. doi: 10.1111/j.1600-0765.1998.tb02349.x
37. Yamaguchi, T, and Takahashi, T. Effect of salivarectomy on the growth of gastrointestinal mucosa and urinary secretion of epidermal growth factor in rats. Gastroenterol Jpn. (1989) 24:626–31. doi: 10.1007/BF02774160
38. Mandel ID. The functions of saliva. J Dent Res. (1987) 66:623–7. doi: 10.1177/00220345870660S103
39. Romandini, M, Gioco, G, Perfetti, G, Deli, G, Staderini, E, and Lafori, A. The association between periodontitis and sleep duration. J Clin Periodontol. (2017) 44:490–501. doi: 10.1111/jcpe.12713
40. Alqaderi, H, Tavares, M, Hartman, M, and Goodson, JM. Effect of sleep and salivary glucose on gingivitis in children. J Dent Res. (2016) 95:1387–93. doi: 10.1177/0022034516661509
41. Sosnin, DY, Gileva, OS, Sivak, EY, Daurova, FY, Gibadullina, NV, and Korotin, SV. The content of vascular endothelial grow factor in saliva and serum in patients with periodontitis. Klin Lab Diagn. (2019) 64:663–8. doi: 10.18821/0869-2084-2019-64-11-663-668
42. Yilmaz Sastim, C, Gursoy, M, Kononen, E, Kasurinen, A, Norvio, S, Gürsoy, UK, et al. Salivary and serum markers of angiogenesis in periodontitis in relation to smoking. Clin Oral Investig. (2021) 25:1117–26. doi: 10.1007/s00784-020-03411-4
43. Alqaderi, H, Goodson, JM, and Agaku, I. Association between sleep and severe periodontitis in a nationally representative adult US population. J Periodontol. (2020) 91:767–74. doi: 10.1002/JPER.19-0105
44. Spiegel, K, Knutson, K, Leproult, R, Tasali, E, and Van Cauter, E. Sleep loss: a novel risk factor for insulin resistance and type 2 diabetes. J Appl Physiol (1985). (2005):2008–19. doi: 10.1152/japplphysiol.00660.2005
45. Brady, EM, Bodicoat, DH, Hall, AP, Khunti, K, Yates, T, Edwardson, C, et al. Sleep duration, obesity and insulin resistance in a multi-ethnic UK population at high risk of diabetes. Diabetes Res Clin Pract. (2018) 139:195–202. doi: 10.1016/j.diabres.2018.03.010
46. Rohleder, N, Aringer, M, and Boentert, M. Role of interleukin-6 in stress, sleep, and fatigue. Ann N Y Acad Sci. (2012) 1261:88–96. doi: 10.1111/j.1749-6632.2012.06634.x
47. Stranges, S, and Miller, M. Meta-analysis of short sleep duration and obesity in children and adults. Sleep. (2008) 31:619–26. doi: 10.1093/sleep/31.5.619
48. Hasler, G, Buysse, DJ, Klaghofer, R, Gamma, A, Ajdacic, V, Eich, D, et al. The association between short sleep duration and obesity in young adults: a 13-year prospective study. Sleep. (2004) 27:661–6. doi: 10.1093/sleep/27.4.661
49. Bishehsari, F, Voigt, RM, and Keshavarzian, A. Circadian rhythms and the gut microbiota: from the metabolic syndrome to cancer. Nat Rev Endocrinol. (2020) 16:731–9. doi: 10.1038/s41574-020-00427-4
Keywords: sleep, cytokin, saliva, serum, inflammation
Citation: Alqaderi H, Abdullah A, Finkelman M, Abufarha M, Devarajan S, Abubaker J, Ramesh N, Tavares M, Al-Mulla F and Bin-Hasan S (2023) The relationship between sleep and salivary and serum inflammatory biomarkers in adolescents. Front. Med. 10:1175483. doi: 10.3389/fmed.2023.1175483
Received: 27 February 2023; Accepted: 09 May 2023;
Published: 26 May 2023.
Edited by:
Deborah Suchecki, Federal University of São Paulo, BrazilReviewed by:
Rakhmat Ari Wibowo, Gadjah Mada University, IndonesiaCopyright © 2023 Alqaderi, Abdullah, Finkelman, Abufarha, Devarajan, Abubaker, Ramesh, Tavares, Al-Mulla and Bin-Hasan. This is an open-access article distributed under the terms of the Creative Commons Attribution License (CC BY). The use, distribution or reproduction in other forums is permitted, provided the original author(s) and the copyright owner(s) are credited and that the original publication in this journal is cited, in accordance with accepted academic practice. No use, distribution or reproduction is permitted which does not comply with these terms.
*Correspondence: Hend Alqaderi, aGVuZF9hbHFhZGVyaUBoc2RtLmhhcnZhcmQuZWR1
Disclaimer: All claims expressed in this article are solely those of the authors and do not necessarily represent those of their affiliated organizations, or those of the publisher, the editors and the reviewers. Any product that may be evaluated in this article or claim that may be made by its manufacturer is not guaranteed or endorsed by the publisher.
Research integrity at Frontiers
Learn more about the work of our research integrity team to safeguard the quality of each article we publish.