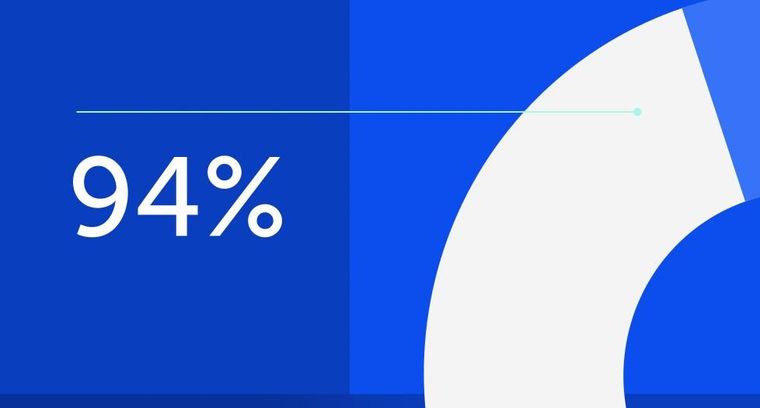
94% of researchers rate our articles as excellent or good
Learn more about the work of our research integrity team to safeguard the quality of each article we publish.
Find out more
ORIGINAL RESEARCH article
Front. Med., 30 June 2023
Sec. Gastroenterology
Volume 10 - 2023 | https://doi.org/10.3389/fmed.2023.1169562
This article is part of the Research TopicGenetics in COVID-19View all 4 articles
Introduction: Coronavirus disease 2019 (COVID-19) is a global pandemic and highly contagious, posing a serious threat to human health. Colorectal cancer (CRC) is a risk factor for COVID-19 infection. Therefore, it is vital to investigate the intrinsic link between these two diseases.
Methods: In this work, bioinformatics and systems biology techniques were used to detect the mutual pathways, molecular biomarkers, and potential drugs between COVID-19 and CRC.
Results: A total of 161 common differentially expressed genes (DEGs) were identified based on the RNA sequencing datasets of the two diseases. Functional analysis was performed using ontology keywords, and pathway analysis was also performed. The common DEGs were further utilized to create a protein-protein interaction (PPI) network and to identify hub genes and key modules. The datasets revealed transcription factors-gene interactions, co-regulatory networks with DEGs-miRNAs of common DEGs, and predicted possible drugs as well. The ten predicted drugs include troglitazone, estradiol, progesterone, calcitriol, genistein, dexamethasone, lucanthone, resveratrol, retinoic acid, phorbol 12-myristate 13-acetate, some of which have been investigated as potential CRC and COVID-19 therapies.
Discussion: By clarifying the relationship between COVID-19 and CRC, we hope to provide novel clues and promising therapeutic drugs to treat these two illnesses.
Severe acute respiratory syndrome coronavirus 2 (SARS-CoV-2) is a betacoronavirus, belonging to the subgenus Sarbecovirus, which is transmitted primarily through the respiratory tract and is highly potent and infectious (1). SARS-CoV-2 has unique features compared with previously known coronavirus genomes, including an optimum affinity for the angiotensin-converting enzyme 2 (ACE2) receptor and a polybasic cleavage site at the S1/S2 spike junction, which determines infectivity and host range (2). In addition, it was shown that SARS-CoV-2 also enters host cells with the primary or auxiliary help of host proteases transmembrane protease serine 2 (TMPRSS2) and FURIN (3), glucose-regulating protein 78 (GRP78) receptor (4), dipeptidyl peptidase 4 (DPP4), (5), cluster of differentiation 147 (CD147) transmembrane protein (6), tyrosine-protein kinase receptor UFO (AXL) (7), phosphatidylinositol 3-phosphate 5-kinase (PIKfyve), two pore channel subtype 2 (TPC2) and cathepsin L (8). The respiratory illness coronavirus disease 2019 (COVID-19) is caused by SARS-CoV-2 (9). Infection with SARS-CoV-2 can stimulate both innate and adaptive immune responses, whereas an uncontrolled inflammatory innate response and a defective adaptive immune response may lead to detrimentally local and systemic tissue damage (9, 10). Patients with COVID-19 frequently experience symptoms such as fever, coughing, vomiting, diarrhea, abdominal pain, shortness of breath, and severe cases that can develop to acute respiratory distress syndrome (ARDS), pneumonia, and multi-organ failure (10, 11). Since its emergence, COVID-19 has continued to affect many parts of the world and threaten millions of lives worldwide (12). Wang et al. have shown that the case fatality rate of COVID-19 patients ranges from 2 to 3% (13). Although the lung is the primary target of coronavirus infection, the digestive system has also been identified to have ACE2, the SARS-CoV-2 functional receptor, suggesting that in addition to the respiratory system, COVID-19 can also infect people through this system (1, 14).
Several studies have been published on the effects of COVID-19 on cancer patients (15–17). A previous study has shown that cancer patients are more vulnerable to infection than individuals without cancer due to the systemic immunosuppressive state created by cancer and anticancer treatment, such as chemotherapy or surgery (17). Yeo et al. further found that cancer was linked to a 2.84-fold greater risk of severe disease in COVID-19 patients and a 2.60-fold increased risk of mortality (18). Colorectal cancer (CRC) is the world’s second-largest cause of cancer death and the third most common malignancy (19). A subgroup analysis based on place of origin showed that Chinese CRC patients had a significant risk of infection, with the global burden of COVID-19 infection in CRC patients being 45.1% (20). According to Burgueño et al., the ileum, colon, and intestinal epithelial cells express ACE2 and TMPRSS2, which permits COVID-19 access into colon cells (21). Immunosuppression, malignancy, and anticancer therapy may play a role in the increased COVID-19 susceptibility in CRC (22). Therefore, it is crucial to explore how COVID-19 influences CRC patients and to search for possible therapeutic agents for CRC patients with COVID-19.
This study attempts to search the shared biological pathways and possible drugs between CRC and COVID-19 by bioinformatics and systems biology techniques. The COVID-19 datasets (GSE196822) and CRC datasets (GSE142279) were from the Gene Expression Omnibus (GEO) database, respectively. Initially, COVID-19 and CRC differentially expressed genes (DEGs) were found, and then common DEGs of the two illnesses were discovered. The obtained common DEGs served as the foundation for subsequent research. Then, employing common DEGs, enrichment analysis and pathway analysis were used to better understand the biological process of gene expression. Protein–protein interaction (PPI) networks were also utilized to gather hub genes based on common DEGs. Transcriptional regulators and miRNAs were then identified using common DEGs. Finally, prospective drugs are offered, as well as gene-disease associations. Figure 1 depicts the overall workflow of this study.
To determine the common gene interrelationship between COVID-19 and CRC, we downloaded RNA-seq datasets of COVID-19 and CRC from the GEO database of the National Center for Biotechnology Information (NCBI)1 (23). The GEO accession ID of COVID-19 was GSE196822, which included RNA-seq profiling from 9 uninfected samples and 34 infected samples using the high throughput sequencing Illumina HiSeq 4,000 (Homo sapiens) platform (24). Similarly, the sequencing of CRC (GSE142279) was gained by high throughput sequencing HiSeq X Ten (Homo sapiens) platform from 20 pairs of colorectal tumors with matched adjacent normal colorectal tissue.
A gene is believed to be expressed differently when there is a transcription level statistically significant difference between distinct test conditions (25). To extract DEGs for datasets GSE196822, we utilized the “DESeq2” package (26) of the R program (version 4.2.2) with False Discover Rate (FDR) < 0.05 and |log2 Fold Change| > 1. The dataset GSE142279 was retrieved by the “limma” package (27) and the criteria for considering the difference in expression as FDR < 0.05 and |log2 Fold Change| > 1. Common DEGs of the two datasets were obtained from the online Venn analysis program Jvenn2 (28).
The Gene Ontology is a globally standardized gene functional classification system with three ontologies: molecular function, cellular component, and biological process. The primary biological functions of DEGs were identified using this analysis (29). By comparing DEGs to the whole genome background, pathway enrichment analysis showed significantly enriched metabolic or signal transduction pathways. Pathway databases included KEGG (Kyoto Encyclopedia of Genes and Genomes), WikiPathways, Reactome, and BioCarta. In this research, we utilized the online gene-set enrichment tool EnrichR3 to investigate the biological processes and signaling pathways linked to common DEGs (30). To quantify the most important functional items and pathways, p-values of 0.05 was employed as standardized metrics.
PPI is an important part of the cellular biochemical response network and can be used to map the functional and structural knowledge of cellular protein networks (31). STRING4 (version 11.5) was utilized to perform the key assessment of protein–protein interactions based on the common DEGs. With a combined score larger than 0.4, the PPI network was created and visualized using Cytoscape (v.3.9.1). The most entangled nodes in the PPI network are referred to as hub genes. To rank and analyze the major nodes in the PPI network module and identify the key genes, we used the Cytoscape plugin cytoHubba. The top ten hub genes in the PPI network were chosen using the Maximal Clique Centrality (MCC) method (32).
TFs are proteins that bind to specific genes and regulate the transcription rate of genetic information. They built a sophisticated system that regulated genome expression and contributed significantly to molecular understanding (33). The DEGs-TFs interaction network was established using the JASPAR database from the online website NetworkAnalyst. NetworkAnalyst was developed in response to the crucial requirement for analyzing gene expression data within the framework of PPI networks to obtain information on biological mechanisms, functions, and interpretations (34). JASPAR is the most complete database of TF-DNA binding site motifs available to the public (35). MiRNAs that target gene interactions were incorporated to identify miRNAs with the potential to bind to gene transcripts and so negatively impact protein production. The miRTarBase, based on NetworkAnalyst and focused on topological analysis, was utilized to identify DEG-miRNA interaction networks. MiRTarBase is the most famous overall miRNA-target interaction database and an important database for evaluating the validity of miRNA-target gene interaction experiments (36). Cytoscape was used to display the TF-gene and miRNA-gene interaction networks.
Predicting protein-drug interactions (PDI) or identifying pharmacological molecules is an essential part of this research. The Drug Signatures database (DSigDB) database was employed to identify the common DEGs by accessing the enrichment of Enrichr under the Diseases/Drugs function on the online website. DSigDB is a gene set resource for identifying targeted drugs associated with DEGs (37). Further screening of significant drug molecules was performed based on the p-value.
DisGeNET,5 one of the biggest and most thorough databases of human gene-disease connections, integrates and standardizes data on disease-associated genes and variants from various sources while also providing a variety of biomedical aspects of the disease (38). The NetworkAnalyst platform was used to construct a gene-disease interaction network to discover diseases and chronic complications linked to common DEGs (39).
To investigate the interrelationship and impact between COVID-19 and CRC, we collected corresponding human RNA-seq datasets, respectively. We identified a total of 1,668 differentially expressed genes between COVID-19 patients and healthy controls, 839 of which showed up-regulated and 829 down-regulated. At the same time, 1,674 in total DEGs were found between colorectal tumors and normal colorectal tissues in CRC patients, including 751 up-regulated DEGs and 923 down-regulated DEGs (Table 1). We discovered 161 common DEGs from COVID-19 and CRC datasets through cross-comparison analysis using the Jvenn (28), a reputable Venn analysis platform, suggesting that they may be related to the biological link between these two conditions (Figure 2). The results of the differential expression study showed that COVID-19 and CRC interacted and shared certain molecular similarities.
Table 1. Overview of the datasets used in this study along with their geo-features and quantitative measurements.
Enrichr was employed for gene ontology and pathway enrichment analysis to discover the biological significance and enriched pathways of the shared DEGs. The gene ontology enrichment method is widely employed to represent interactions between genes and gene ontology terms. The top ten terms in the categories of biological process, molecular function, and cellular component were gathered in Table 2. Figure 3 presents the total ontological analysis as a linear bar graph for each category. In the biological process, the GO terms are important in relation to neutrophils and interferon-gamma. Pathway analysis demonstrates that the organism responds to its inherent modifications. To assemble the most enriched pathways of the common DEGs, four global databases (KEGG, WikiPathways, Reactome, and BioCarta) were used. Table 3 summarizes the top ten pathways identified by pathway analysis, and the results are presented more intuitively in the bar graph of Figure 4. Acute myeloid leukemia, the immune system, and cyclins and cell cycle regulation pathways are critical in the analysis.
Figure 3. Ontological analysis of shared DEGs: (A) Biological processes, (B) cellular components, and (C) molecular functions.
Figure 4. Pathway enrichment analysis: (A) KEGG, (B) WikiPathways, (C) Reactome, and the (D) BioCarta.
PPI network can visually show the correlation between different proteins, indicating the potential mechanism of protein action. Key proteins that affect how cells and systems function biologically have been discovered due to the assessment and analysis of the PPI network (39). The PPI network was created with STRING based on common DEGs and visualized in Cytoscape. The results are shown in Figure 5, which includes 151 nodes and 286 edges. In the PPI network, the majority of interconnected nodes are referred to as hub genes. A submodule network was further constructed with the latest MCC procedure of the Cytohubba plugin in Cytoscape to analyze potential hub genes. The top 10 genes were listed as the most influential genes, including CDK1, KIFC1, CDCA5, MKI67, UBE2C, PLK1, TPX2, KIF20A, HJURP, CENPA (Figure 6).
Figure 5. PPI network of SARS-CoV-2 and CRC shared DEGs. The octagons in the figure represent DEGs, while the edges represent node interactions. The PPI network comprises 151 nodes and 286 edges and the size and color depth of the octagon indicate the extent to which proteins are connected.
Figure 6. Identification of the hub genes from the PPI network. Here, the highlighted top 10 hub genes and their interactions with other molecules are shown by the red, orange, and yellow nodes (their rank was indicated by a gradient from red to yellow). The network consists of 21 nodes and 117 edges.
We used NetworkAnalyst to identify regulatory TFs and miRNAs to characterize the significant alterations at the transcriptional level and better comprehend the regulatory key proteins. Figures 7, 8 depict the interactions of TF regulators and miRNA regulators with hub genes. Forty-two TFs and 248 miRNA regulatory signals were projected to affect numerous common differential genes, implying that they interact.
Figure 7. The DEG-TF cohesive regulatory interaction network. The diamond shape nodes in this diagram represent TFs, while the circle nodes represent gene symbols interacting with TFs.
Figure 8. The DEGs-miRNAs regulation interaction network. The green circle nodes in the figure denote miRNAs, whereas the yellow circles represent the genes that interact with miRNAs.
Interactions between drugs and proteins play an important role in understanding and recommending structural features for receptor sensitivity. Based on DSigDB, which has the most gene sets connected to drugs/compounds to date, Enrichr was used to screen out the top ten drug molecules with p-values from the common DEGs (Table 4). The ten potential drugs include troglitazone, estradiol, progesterone, calcitriol, genistein, dexamethasone, lucanthone, resveratrol, retinoic acid, phorbol 12-myristate 13-acetate. These drugs have the potential to be employed in the treatment of COVID-19 and CRC. Deciphers the connection between genes and disease begins with the development of technologies for illness therapy. DisGeNET is a platform for managing knowledge that unifies and standardizes information on genes and variants linked to disease from many sources. Based on DisGeNET, gene-disease association analysis was performed by NetworkAnalyst. The results showed that lung neoplasms, autoimmune diseases, liver cirrhosis, unipolar depression, and colonic neoplasms were most concordant with the common DEGs (Figure 9).
Figure 9. The network of gene-disease associations represents diseases linked by common DEGs. The square node indicates the disease, and the circular node defines the subsequent gene symbols.
The COVID-19 epidemic, caused by SARS-CoV-2, has been designated a worldwide emergency due to the damage for its quick spread and high mortality (10). CRC is the largest cause of cancer mortality worldwide, and it is one of the cancers whose incidence is increasing, accounting for 11% of all cancer diagnoses (40). Previous research has shown that cancer patients are more sensitive to infection than individuals without cancer, and Chinese patients with colorectal cancer have a significant risk of infection (18, 20, 41). In this study, we investigated the potential interactions between CRC and COVID-19 using bioinformatics and systems biology techniques. We screened 1,668 and 1,674 DEGs from GSE196822 and GSE142279 datasets, respectively, and identified 161 common DEGs. Then, based on common DEGs, we analyzed GO, pathways, PPI network, hub genes, TF-gene interaction network, miRNA-gene coordination node network, candidate drugs, and gene-disease.
GO terms were selected with mutual DEGs and based on p-values. For the biological process, neutrophil degranulation, neutrophil activation involved in immune response, neutrophil mediated immunity, and regulation of interferon-gamma production were important GO terms. Neutrophils perform an essential part in the course of COVID-19 infection. The immune response of COVID-19 patients with different severity levels includes increased neutrophil degranulation (42). By releasing neutrophil extracellular traps (NETs), neutrophils recruited to infection sites protect against viral challenges (43). NETs are condensed extracellular chromatin filaments composed of nuclear and cytoplasmic proteins, which are associated with many gastrointestinal diseases, including CRC. It was found that NET formation not only increases the proliferation of CRC cells but also stimulates the transfer process (44). Interferon-gamma, an antiviral agent, is an immunomodulator and plays a key role in humoral and cellular immunity. It has also been shown to be possible to try broad-spectrum antiviral therapy including COVID-19 (45). Under cellular component, collagen-containing extracellular matrix and secretory granule lumen were the top two GO terms. In molecular function, RAGE receptor binding and serine-type peptidase activity were the top two GO terms.
A total of 161 common DEGs were used to search for similar pathways in COVID-19 and CRC. In KEGG pathways, acute myeloid leukemia is significant. Acute myeloid leukemia (AML) is a deadly hematologic cancer. Patients with AML have substantial immunosuppression, and infections are frequently carried on by harsh chemotherapy treatment and disease-related reduced immunity (46). According to the literature, COVID-19 is usually present in a severe clinical form in AML patients, who also frequently have respiratory distress and a very high mortality rate (47). WikiPathways results show that vitamin B12 metabolism and melatonin metabolism and effects are the two pathways of significant importance. In addition to acting as an immunomodulator to regulate cellular immune responses and support hematopoiesis, vitamin B12 can inhibit viral replication in host cells (48, 49). According to Shakeri et al., clinical outcomes in COVID-19 patients are impacted by the host immunological response to viral infections, inflammatory activity, and serum levels of micronutrient deficiencies such as zinc and vitamin B12 deficiency (50). Melatonin is the primary neurohormone secreted by the pineal gland that regulates the sleep–wake cycle. It is also a multifunctional hormone with immunomodulatory, anti-inflammatory, and antiapoptotic properties, as well as having an impact on the metabolism of most organs (51, 52). A randomized clinical trial including 96 COVID-19 patients showed that although melatonin was not associated with a reduction in clinical symptoms and death, melatonin plus standard therapy significantly improved sleep quality and saturation of peripheral oxygen (SpO2) in COVID-19 patients treated for 7 days compared to standard therapy alone. And the effect of melatonin on oxygen delivery and its utilization in tissues may be responsible for the significant improvement in blood oxygen saturation (53). Under Reactome pathways, the immune system and metabolism of angiotensinogen to angiotensins show their importance. When the body is infected with COVID-19, the innate and adaptive immune systems make an effort to eradicate the virus. However, occasionally, the virus interacts with the body in a way that stimulates immune and non-immune cells, resulting in an overactive immunological response (54). Some patients with severe COVID-19 may also experience an overactive cytokine storm or “cytokine release syndrome” caused by an imbalance in the immune system regulation (55). Angiotensinogen, the starting substrate, is cleaved by renin to angiotensin I (Ang I). Ang I is then cleaved by angiotensin-converting enzyme (ACE) to angiotensin II (Ang II), which is metabolized by ACE2 to the beneficial peptide angiotensin 1–7 (Ang 1–7), (56). Importantly, SARS-CoV-2 attacks alveolar cells via ACE2 receptors, causing lung infection and leading to COVID-19 (57). In BioCarta pathways, cyclins and cell cycle regulation pathway is critical. COVID-19 patients showed some specific differential features in the expression patterns of some cell cycle-related genes compared to other respiratory diseases. It is important to note that cell cycle characteristics predominated in blood leukocytes (B, T, and natural killer cells) of COVID-19 patients and correlated with their severity and disease trajectory (58).
The PPI network was constructed by using DEGs genes to realize the shared biological functional characteristics of proteins and predict potential drugs. Based on the PPIs network and the MCC method, the top ten hub genes are CDK1, KIFC1, CDCA5, MKI67, UBE2C, PLK1, TPX2, KIF20A, HJURP, and CENPA. Cyclin-dependent kinase 1 (CDK1) is the sole essential required cyclin-dependent kinase that enables the G2-M transition and regulates G1 progression and G1-S transition (59). It has been demonstrated that not only does CDK1 regulate the cell cycle, but it also plays a part in tumor cell proliferation (60–62). Zhang et al. claimed that CDK1 is overexpressed in CRC cells and is apoptosis-sensitive (61). Furthermore, Tong et al. showed that dipeptidyl peptidase 3 (DPP3)/CDK1 contributes to the advancement of CRC by modulating cell proliferation, apoptosis, and migration (62). Kinesin family member C1 (KIFC1) is involved in multiple biological functions and plays a key role in cancer cell centrosome clustering (63). In CRC patients, KIFC1 can regulate ZW10 interacting kinetochore protein (ZWINT) to promote CRC progression and may induce poor prognosis (64). Cell division cycle associated 5 (CDCA5), originally recognized as a substrate that promotes late complexes, has been reported to be associated with the development and progression of multiple human cancers (65). Shen et al. showed that CDCA5 expression was increased in CRC patients and that overexpression of CDCA5 was associated with poor patient survival. Activation of the extracellular signal-regulated kinase (ERK) pathway may show a beneficial role in the oncogenic activity of CDCA5 (66). MKI67, or proliferation marker protein Ki-67, is a nuclear protein that is expressed in all proliferative vertebrate cells and is commonly used as a biomarker to quantify the fraction of proliferating cells to grade tumors (67). MKI67 expression is a late marker of cell cycle entry and has a key role in many CRC-associated genes (68). Downregulation of MKI67 will inhibit cell growth in CRCs (69). The ubiquitin conjugating enzyme E2 C (UBE2C) modifies cellular short-lived or abnormal proteins. Rectal cancer with overexpressed UBE2C regulates miR-381 expression, encouraging rectal carcinoma cell proliferation and invasion (70). Polo-like kinase 1 (PLK1) is a conserved Ser/Thr kinase that has evolved over time and is well known for its function in controlling the cell cycle. It is mostly expressed during the G2/S and M stages of the cell cycle (71). PLK1, which has been found to be overexpressed in numerous cancers, regulates several important transcription factors and promotes cell proliferation, transformation, and epithelial-to-mesenchymal transition (71, 72). In CRC, upregulated PLK1 signaling is frequently accompanied by dysregulation of cell cycle-related pathways. And abnormal PLK1 signaling has been linked to recurrence and a poor prognosis in CRC patients (73). Targeting protein for Xenopus kinesin-like protein 2 (TPX2) is an indirect target of miR-485-3p prediction and has an important part in mitotic spindle assembly and cancer progression. It has been demonstrated that TPX2 is overexpressed in several cancers and highly expressed in CRC tissues (74). The kinesin family member 20A (KIF20A), which is found on chromosome 5q31.2, is crucial for the occurrence and growth of malignancies. KIF20A is overexpressed in CRC tissues and is significantly linked to patients’ poor prognoses. While silencing KIF20A expression can prevent CRC cell lines from migrating and proliferating by activating the janus kinases (JAKs)/signal transducer and activator of transcription 3 (STAT3) signaling pathway (75). The centromeric protein holliday junction recognition protein (HJURP, or hFLEG1) is essential for the incorporation and maintenance of the histone H3-like variant centromere protein A (CENPA) in centromeres. HJURP knockdown reduces CRC cell migration, proliferation, invasion, and tumorigenicity; therefore, it may be used as a prognostic biomarker and a new target for therapeutic development (76). And CENPA can recruit histone acetyltransferase (HAT) general control of amino acid synthesis (GCN)-5 to the karyopherin α2 subunit gene (KPNA2) promoter region to epigenetically activate its transcriptional activity, resulting in glycolysis and malignant growth in colon cancer (CC) cells (77). Therefore, these hub genes are likely to be potential biomarkers.
To identify the transcriptional and post-transcriptional regulators of the hub genes, we also explore the interactions between the TFs–gene and miRNAs. Among TFs, NFIC, GATA2, YY1, NFYA, E2F1, PRDM1, MEF2A and SREBF1 are associated with cancer (78–85). Nuclear factor I C (NFIC) belongs to the nuclear factor I family. One study reported that NFIC was a low expression in human Lung Squamous Cell Carcinoma (LUSC) tissues and cell lines, and it inhibited the proliferation of LUSC cells and promoted apoptosis in vitro and in vivo. Additionally, NFIC prevented LUSC cells from migrating and invading other tissues (78). E2F1 is a transcription factor that controls cell cycle progression and was the first member of the E2F transcription factors (E2Fs) to be discovered. It is highly expressed in the majority of cancer cells and prompts the transcription of kinases relevant to the cell cycle. E2F1 regulates the cell cycle in CRC by encouraging spindle construction, where E2F1-induced stathmin1 and transforming acidic coiled-coil-containing protein 3 (TACC3) improve the stability of spindle fiber (82). For DEG-miRNAs, hsa-mir-193b-3p, hsa-mir-192-5p, hsa-mir-215-5p, and hsa-mir-16-5p are associated with cancer. Overexpression of hsa-mir-193b-3p inhibited lung cancer cell invasion and migration and reduced their clonogenic ability. In contrast, hsa-mir-193b-3p inhibition boosted lung cancer’s capacity for metastatic spread and colony formation (86). In cases of colorectal cancer and other malignancies, hsa-mir-192/215-5p can function as a tumor suppressor (87). For hsa-mir-16-5p, in addition to its association with cancer, it was also shown to affect SARS-CoV-2 infection possibly by regulating the ACE2 receptor related network (88, 89). Using data based on single-cell RNA-seq, Li et al. identified hsa-mir-16-5p as one of the probable virus-targeting miRNAs in various cell types in bronchoalveolar lavage fluid samples (89). The previous study also has shown that miRNA hsa-mir-155-5p leads to B cells and T cells activation and exerts immune functions (90). Hsa-mir-34a-5p is one of the promising therapeutics for SARS-CoV-2 (91).
To determine the relationship between significant DEGs and various diseases, we completed a gene-disease analysis. The results showed various types of disease associated with COVID-19, including the brain, blood, skin, heart, liver, joints, and different types of tumors. Brain-related diseases include schizophrenia, unipolar depression, bipolar disorder, epilepsy, and autistic disorder. Individuals with a diagnosis of schizophrenia spectrum disorder were shown to have a higher risk of mortality in a study of adults with SARS-CoV-2 positive test results in a large New York medical system (92). In particular, anxiety disorders are becoming more common than ever before due to the psychosocial impacts of SARS-CoV-2, which in turn may precipitate the onset of a number of co-morbid psychiatric conditions like depression and bipolar disorder (93). In this sense, viral infections are linked to a higher incidence of anxiety and/or depression (94). We also discovered skin problems including contact dermatitis. According to solid data, the COVID-19 pandemic has led to an increase in the prevalence of allergic and irritating contact dermatitis. The most typically reported symptoms are skin dryness, itchiness, and redness (95). Furthermore, Grivas et al. used detailed information from nearly 5,000 patients with COVID-19 and cancer to confirm that COVID-19 severity and mortality are high in cancer patients. This was particularly the case for older age, male sex, non-Hispanic non-white racial/ethnic group, worse eastern cooperative oncology group performance status, hematologic malignancies, and selected laboratory measures (96).
Through the common DEGs, we predicted ten drugs, including troglitazone, estradiol, progesterone, calcitriol, genistein, dexamethasone, lucanthone, resveratrol, retinoic acid, phorbol 12-myristate 13-acetate. Several substances have been evaluated as CRC and COVID-19 therapies. Endogenous reproductive hormones estradiol and progesterone are produced in large amounts in the periphery by the adrenal glands and ovaries, and de novo by the brain. They have a significant physiological impact by controlling inflammatory behaviors and processes (97). In the azoxymethane/dextran sulfate sodium (AOM/DSS) mouse model, estradiol inhibits the development of CRC and downregulated inflammation (98). And progesterone, which could inhibit CRC proliferation by blocking the cell cycle and inducing apoptosis in turn limits malignant tumor progression (99). In COVID-19, women with high estrogen levels have a lower risk of severe symptoms and even lower mortality (100). Calcitriol, the biologically active form of vitamin D, is effective as a treatment for patients with hypocalcemia or secondary hyperparathyroidism caused by renal insufficiency (101). For other roles, calcitriol can inhibit glycolysis and cell growth in human CRC cells, suggesting an inhibitory role in CRC progression (102). And Elamir et al. have demonstrated improved oxygenation in hospitalized COVID-19 patients treated with calcitriol (103). Genistein is one of the main bioactive substances of soybean isoflavones that exert pharmacological functions (104). It can block cancer cell proliferation by various mechanisms, such as up-regulation of p21 levels and inhibition of tyrosine-specific kinase activity (105, 106). In human CRC cells, genistein exerts anti-invasive and anti-proliferative effects by inhibiting cell proliferation and inducing apoptosis (107). Additionally, RNA transcripts and protein synthesis from viruses like rotavirus can be inhibited by genistein (108). Therefore, genistein has the potential to be used as a lead chemical in the evaluation of new anti-CRC and antiviral drug candidates. Dexamethasone is a drug used to treat a variety of inflammatory diseases by effectively inhibiting the release of substances that cause inflammation, and it has been already in clinical use for cancer prevention (109). In addition, for patients who are receiving mechanical ventilation, the corticosteroid dexamethasone can lower the rate of COVID-19-related mortality in the critical care unit by 35%. Despite the varying efficiency of other combination medications in treating COVID-19 associated with ARDS, dexamethasone is widely employed in several COVID-19 therapy regimens (110). Resveratrol as a traditional Chinese medicine chemical monomer can effectively inhibit colon cancer cell growth by the ROS-dependent ferroptosis pathway (111). Retinoic acid is further processed from vitamin A (retinol) by several enzymes and exists in several stereoisomeric forms. In addition to its role in biological processes such as reproduction, differentiation, embryogenesis, and eye development, retinoic acid signaling has been shown to be a possible therapeutic target for CRC (112). Furthermore, McCreary and Sarohan et al. have also shown that resveratrol and retinoic acid may also be new perspectives for the treatment of COVID-19 (113, 114).
This study explored the correlation between CRC and COVID-19 using bioinformatics and systems biology techniques. Through high-throughput sequencing, this study identified COVID-19 and CRC-related biomarkers and potential drugs, which provide new clues for the treatment of both diseases. It is worth noting that there are still some limitations in this study. First, it is impossible to fully recapitulate the underlying genetic linkages using a computational biology technique because of information and method bias. Second, we currently do not have experiments in vivo or in vitro to validate the findings, and specific experiments will be conducted in the next step. Last, there is still a long way to go to translate experimental results into clinical applications.
In this study, the transcriptome datasets of CRC and COVID-19 were used to identify DEGs and elucidated the intrinsic link between the two diseases. A total of 161 common DEGs were found, based on which a PPI network was constructed to recognize the top ten hub genes. These target genes may be biomarkers of CRC or SARS-CoV-2 infection and thus translate into potential drug targets. We also investigated the relationship of these target genes with TFs and miRNAs to better understand their role in disease occurrence and progression. Meanwhile, multiple drug molecules and drug-target interactions of common DEGs were retrieved using the DSigDB database. However, further experiments are needed to verify whether these potential gene targets and drugs can be further used in clinical practice.
The datasets presented in this study can be found in online repositories. The names of the repository/repositories and accession number(s) can be found in the article/supplementary material.
YS, TH, and HP contributed to the conception and design of the study. YS organized the database. TH performed the formal analysis. HP was responsible for the article methodology. AD, TW, JL, XZ, YL, and SX wrote sections of the manuscript. KY conducted the overall supervision and validation. All authors contributed to the article and approved the submitted version.
This work was supported by grants from the National multidisciplinary collaborative diagnosis and treatment capacity building project for major diseases (TJZ202104), the Natural Science Foundation of China (82173248, 82272685, 82002967 and 82202260), the Postdoctoral Science the fellowship of China National Postdoctoral Program for Innative Talents (BX20200225), the Project funded by China Postdoctoral Science Foundation (2022TQ0221), the Science and Technology Major Program of Sichuan Province (2022ZDZX0019), the Sichuan Science and Technology Program (2023YFS0128, 2023NSFSC1874, 2021YJ0420), 1.3.5 project for disciplines of excellence, West China Hospital, Sichuan University (ZYJC18008, ZYGD22006), the Sichuan University postdoctoral interdisciplinary Innovation Fund (10822041A2103).
We thank all of the patients who participated in the study and donated samples, as well as the GEO database for providing the platform.
The authors declare that the research was conducted in the absence of any commercial or financial relationships that could be construed as a potential conflict of interest.
All claims expressed in this article are solely those of the authors and do not necessarily represent those of their affiliated organizations, or those of the publisher, the editors and the reviewers. Any product that may be evaluated in this article, or claim that may be made by its manufacturer, is not guaranteed or endorsed by the publisher.
1. ^https://www.ncbi.nlm.nih.gov/
2. ^http://jvenn.toulouse.inra.fr/app/index.html
1. Ciotti, M , Ciccozzi, M , Terrinoni, A , Jiang, WC , Wang, CB , and Bernardini, S . The Covid-19 pandemic. Crit Rev Clin Lab Sci. (2020) 57:365–88. doi: 10.1080/10408363.2020.1783198
2. Andersen, KG , Rambaut, A , Lipkin, WI , Holmes, EC , and Garry, RF . The proximal origin of Sars-Cov-2. Nat Med. (2020) 26:450–2. doi: 10.1038/s41591-020-0820-9
3. Li, K , Meyerholz, DK , Bartlett, JA , and McCray, PB Jr. The Tmprss 2 inhibitor Nafamostat reduces Sars-Cov-2 pulmonary infection in mouse models of Covid-19. MBio. (2021) 12:e00970–21. doi: 10.1128/mBio.00970-21
4. Allam, L , Ghrifi, F , Mohammed, H , El Hafidi, N , El Jaoudi, R , El Harti, J, et al. Targeting the Grp 78-Dependant Sars-Cov-2 cell entry by peptides and small molecules. Bioinform Biol Insights. (2020) 14:117793222096550. doi: 10.1177/1177932220965505
5. Strollo, R , and Pozzilli, P . Dpp4 inhibition: preventing Sars-Cov-2 infection and/or progression of Covid-19? Diabetes Metab Res Rev. (2020) 36:e3330. doi: 10.1002/dmrr.3330
6. Behl, T , Kaur, I , Aleya, L , Sehgal, A , Singh, S , Sharma, N, et al. Cd147-spike protein interaction in Covid-19: get the ball rolling with a novel receptor and therapeutic target. Sci Total Environ. (2022) 808:152072. doi: 10.1016/j.scitotenv.2021.152072
7. Wang, S , Qiu, Z , Hou, Y , Deng, X , Xu, W , Zheng, T, et al. Axl is a candidate receptor for Sars-Cov-2 that promotes infection of pulmonary and bronchial epithelial cells. Cell Res. (2021) 31:126–40. doi: 10.1038/s41422-020-00460-y
8. Ou, X , Liu, Y , Lei, X , Li, P , Mi, D , Ren, L, et al. Characterization of spike glycoprotein of Sars-Cov-2 on virus entry and its immune cross-reactivity with Sars-Cov. Nat Commun. (2020) 11:1620. doi: 10.1038/s41467-020-15562-9
9. Cao, X . Covid-19: immunopathology and its implications for therapy. Nat Rev Immunol. (2020) 20:269–70. Epub 2020/04/11. doi: 10.1038/s41577-020-0308-3
10. Yang, L , Liu, S , Liu, J , Zhang, Z , Wan, X , Huang, B, et al. Covid-19: Immunopathogenesis and Immunotherapeutics. Signal Transduct Target Ther. (2020) 5:1–8. doi: 10.1038/s41392-020-00243-2
11. Wang, D , Hu, B , Hu, C , Zhu, F , Liu, X , Zhang, J, et al. Clinical characteristics of 138 hospitalized patients with 2019 novel coronavirus–infected pneumonia in Wuhan, China. JAMA. (2020) 323:1061–9. doi: 10.1001/jama.2020.1585
12. Abd El-Raheem, GOH , Elamin, HES , Ahmad, ZMO , and Noma, M . Spatial–temporal trends of COVID-19 infection and mortality in Sudan. Sci Rep. (2022) 12:16822. doi: 10.1038/s41598-022-21137-z
13. Madabhavi, I , Sarkar, M , and Kadakol, N . Covid-19: a review. Monaldi Arch Chest Dis. (2020) 90:248–258. doi: 10.4081/monaldi.2020.1298
14. Ma, C , Dong, W , Shen, B , and Zhang, H . Editorial: Covid-19 and the digestive system. Front Med (Lausanne). (2022) 9:875063. doi: 10.3389/fmed.2022.875063
15. Al-Quteimat, OM , and Amer, AM . The impact of the Covid-19 pandemic on cancer patients. Am J Clin Oncol. (2020) 43:452–5. doi: 10.1097/COC.0000000000000712
16. Naimi, A , Yashmi, I , Jebeleh, R , Imani Mofrad, M , Azimian Abhar, S , Jannesar, Y, et al. Comorbidities and mortality rate in Covid-19 patients with hematological malignancies: a systematic review and Meta-analysis. J Clin Lab Anal. (2022) 36:e24387. doi: 10.1002/jcla.24387
17. Liang, W , Guan, W , Chen, R , Wang, W , Li, J , Xu, K, et al. Cancer patients in Sars-Cov-2 infection: a Nationwide analysis in China. Lancet Oncol. (2020) 21:335–7. doi: 10.1016/S1470-2045(20)30096-6
18. Gao, Y , Liu, M , Shi, S , Chen, Y , Sun, Y , Chen, J, et al. Cancer is associated with the severity and mortality of patients with Covid-19: a systematic review and meta-analysis. MedRxiv. (2020). doi: 10.1101/2020.05.01.20087031
19. Xie, YH , Chen, YX , and Fang, JY . Comprehensive review of targeted therapy for colorectal Cancer. Signal Transduct Target Ther. (2020) 5:22. doi: 10.1038/s41392-020-0116-z
20. Antikchi, MH , Neamatzadeh, H , Ghelmani, Y , Jafari-Nedooshan, J , Dastgheib, SA , Kargar, S, et al. The risk and prevalence of Covid-19 infection in colorectal Cancer patients: a systematic review and Meta-analysis. J Gastrointest Cancer. (2021) 52:73–9. doi: 10.1007/s12029-020-00528-3
21. Burgueño, JF , Reich, A , Hazime, H , Quintero, MA , Fernandez, I , Fritsch, J, et al. Expression of Sars-Cov-2 entry molecules Ace2 and Tmprss2 in the gut of patients with Ibd. Inflamm Bowel Dis. (2020) 26:797–808. doi: 10.1093/ibd/izaa085
22. Parise, R , Li, YE , Nadar, RM , Ramesh, S , Ren, J , Govindarajulu, MY, et al. Health influence of Sars-Cov-2 (Covid-19) on Cancer: a review. Acta Biochim Biophys Sin. (2022) 54:1395–405. doi: 10.3724/abbs.2022147
23. Barrett, T , Wilhite, SE , Ledoux, P , Evangelista, C , Kim, IF , Tomashevsky, M, et al. Ncbi geo: archive for functional genomics data sets—update. Nucleic Acids Res. (2012) 41:D991–5. doi: 10.1093/nar/gks1193
24. Blanco-Melo, D , Nilsson-Payant, BE , Liu, W-C , Uhl, S , Hoagland, D , Møller, R, et al. Imbalanced host response to Sars-Cov-2 drives development of Covid-19. Cells. (2020) 181:1036–1045.e9. doi: 10.1016/j.cell.2020.04.026
25. Fang, H , Sun, Z , Chen, Z , Chen, A , Sun, D , Kong, Y, et al. Bioinformatics and systems-biology analysis to determine the effects of coronavirus disease 2019 on Patients with Allergic Asthma. Front Immunol. (2022) 13:5437. doi: 10.3389/fimmu.2022.988479
26. Love, MI , Huber, W , and Anders, S . Moderated estimation of fold change and dispersion for Rna-Seq data with Deseq2. Genome Biol. (2014) 15:1–21. doi: 10.1186/s13059-014-0550-8
27. Smyth, GK . “limma: Linear Models for Microarray Data,” in Bioinformatics and Computational Biology Solutions Using R and Bioconductor. eds. R. Gentleman, V. J. Carey, W. Huber, R. A. Irizarry, and S. Hedges (New York, NY: Statistics for Biology and Health, Springer).
28. Bardou, P , Mariette, J , Escudié, F , Djemiel, C , and Klopp, C . Jvenn: an interactive venn diagram viewer. BMC Bioinformatics. (2014) 15:1–7. doi: 10.1186/1471-2105-15-293
29. Peng, J , Wang, H , Lu, J , Hui, W , Wang, Y , and Shang, X . Identifying term relations cross different gene ontology categories. BMC Bioinformatics. (2017) 18:67–74. doi: 10.1186/s12859-017-1959-3
30. Kuleshov, MV , Jones, MR , Rouillard, AD , Fernandez, NF , Duan, Q , Wang, Z, et al. Enrichr: a comprehensive gene set enrichment analysis web server 2016 update. Nucleic Acids Res. (2016) 44:W90–7. doi: 10.1093/nar/gkw377
31. De Las, RJ , and Fontanillo, C . Protein–protein interactions essentials: key concepts to building and analyzing Interactome networks. PLoS Comput Biol. (2010) 6:e1000807. doi: 10.1371/journal.pcbi.1000807
32. Chin, C-H , Chen, S-H , Wu, H-H , Ho, C-W , Ko, M-T , and Lin, C-Y . Cytohubba: identifying hub objects and sub-networks from complex Interactome. BMC Syst Biol. (2014) 8:1–7. doi: 10.1186/1752-0509-8-S4-S11
33. Lambert, SA , Jolma, A , Campitelli, LF , Das, PK , Yin, Y , Albu, M, et al. The human transcription factors. Cells. (2018) 172:650–65. doi: 10.1016/j.cell.2018.01.029
34. Zhou, G , Soufan, O , Ewald, J , Hancock, RE , Basu, N , and Xia, J . Networkanalyst 3.0: a visual analytics platform for comprehensive gene expression profiling and Meta-analysis. Nucleic Acids Res. (2019) 47:W234–41. doi: 10.1093/nar/gkz240
35. Li, X-y , Wang, J-b , An, H-b , Wen, M-z , You, J-x , and Yang, X-t . Effect of Sars-Cov-2 infection on asthma patients. Front Med. (2022) 9:9. doi: 10.3389/fmed.2022.928637
36. Sethupathy, P , Corda, B , and Hatzigeorgiou, AG . Tarbase: a comprehensive database of experimentally supported animal Microrna targets. RNA. (2006) 12:192–7. doi: 10.1261/rna.2239606
37. Yoo, M , Shin, J , Kim, J , Ryall, KA , Lee, K , Lee, S, et al. Dsigdb: drug signatures database for gene set analysis. Bioinformatics. (2015) 31:3069–71. doi: 10.1093/bioinformatics/btv313
38. Salnikova, LE , Chernyshova, EV , Anastasevich, LA , and Larin, SS . Gene-and disease-based expansion of the knowledge on inborn errors of immunity. Front Immunol. (2019) 10:2475. doi: 10.3389/fimmu.2019.02475
39. Lu, L , Liu, LP , Gui, R , Dong, H , Su, YR , Zhou, XH, et al. Discovering common Pathogenetic processes between Covid-19 and Sepsis by bioinformatics and system biology approach. Front Immunol. (2022) 13:975848. Epub 2022/09/20. doi: 10.3389/fimmu.2022.975848
40. Sawicki, T , Ruszkowska, M , Danielewicz, A , Niedźwiedzka, E , Arłukowicz, T , and Przybyłowicz, KE . A review of colorectal Cancer in terms of epidemiology, risk factors, development, symptoms and diagnosis. Cancers. (2021) 13:2025. doi: 10.3390/cancers13092025
41. Shen, Q , Wang, J , and Zhao, L . To investigate the internal association between Sars-Cov-2 infections and cancer through bioinformatics. Math Biosci Eng. (2022) 19:11172–94. doi: 10.3934/mbe.2022521
42. Bibert, S , Guex, N , Lourenco, J , Brahier, T , Papadimitriou-Olivgeris, M , Damonti, L, et al. Transcriptomic signature differences between Sars-Cov-2 and influenza virus infected patients. Front Immunol. (2021) 12:666163. doi: 10.3389/fimmu.2021.666163
43. Jenne, CN , Wong, CH , Zemp, FJ , McDonald, B , Rahman, MM , Forsyth, PA, et al. Neutrophils recruited to sites of infection protect from virus challenge by releasing neutrophil extracellular traps. Cell Host Microbe. (2013) 13:169–80. doi: 10.1016/j.chom.2013.01.005
44. Khan, U , Chowdhury, S , Billah, MM , Islam, KMD , Thorlacius, H , and Rahman, M . Neutrophil extracellular traps in colorectal Cancer progression and metastasis. Int J Mol Sci. (2021) 22:7260. doi: 10.3390/ijms22147260
45. Ozcelik, F , Tanoglu, A , Çıracı, MZ , and Ozcelik, IK . Use of immune modulator interferon-gamma to support combating Covid-19 pandemic. Int J Coronaviruses. (2020) 1:1–15. doi: 10.14302/issn.2692-1537.ijcv-20-3345
46. Ferrara, F , and Schiffer, CA . Acute Myeloid Leukaemia in Adults. Lancet. (2013) 381:484–95. doi: 10.1016/S0140-6736(12)61727-9
47. Marchesi, F , Salmanton-García, J , Emarah, Z , Piukovics, K , Nucci, M , López-García, A, et al. Covid-19 in adult acute myeloid leukemia patients: a long-term Followup study from the European Hematology Association survey (Epicovideha). Haematologica. (2023) 108:22–33. doi: 10.3324/haematol.2022.280847
48. Maggini, S , Pierre, A , and Calder, PC . Immune function and micronutrient requirements change over the life course. Nutrients. (2018) 10:1531. doi: 10.3390/nu10101531
49. Herrmann, W , and Obeid, R . Causes and early diagnosis of vitamin B12 deficiency. Dtsch Arztebl Int. (2008) 105:680–5. doi: 10.3238/arztebl.2008.0680
50. Shakeri, H , Azimian, A , Ghasemzadeh-Moghaddam, H , Safdari, M , Haresabadi, M , Daneshmand, T, et al. Evaluation of the relationship between serum levels of zinc, vitamin B12, vitamin D, and clinical outcomes in patients with Covid-19. J Med Virol. (2022) 94:141–6. doi: 10.1002/jmv.27277
51. Auld, F , Maschauer, EL , Morrison, I , Skene, DJ , and Riha, RL . Evidence for the efficacy of melatonin in the treatment of primary adult sleep disorders. Sleep Med Rev. (2017) 34:10–22. doi: 10.1016/j.smrv.2016.06.005
52. Zhang, R , Wang, X , Ni, L , Di, X , Ma, B , Niu, S, et al. Covid-19: melatonin as a potential adjuvant treatment. Life Sci. (2020) 250:117583. doi: 10.1016/j.lfs.2020.117583
53. Mousavi, SA , Heydari, K , Mehravaran, H , Saeedi, M , Alizadeh-Navaei, R , Hedayatizadeh-Omran, A, et al. Melatonin effects on sleep quality and outcomes of Covid-19 patients: An open-label, randomized, controlled trial. J Med Virol. (2022) 94:263–71. doi: 10.1002/jmv.27312
54. Xu, Z , Shi, L , Wang, Y , Zhang, J , Huang, L , Zhang, C, et al. Pathological findings of Covid-19 associated with acute respiratory distress syndrome. Lancet Respir Med. (2020) 8:420–2. doi: 10.1016/S2213-2600(20)30076-X
55. Eisen, HN , and Chakraborty, AK . Evolving concepts of specificity in immune reactions. Proc Natl Acad Sci. (2010) 107:22373–80. doi: 10.1073/pnas.1012051108
56. Patel, VB , Zhong, J-C , Grant, MB , and Oudit, GY . Role of the Ace2/angiotensin 1–7 Axis of the renin–angiotensin system in heart failure. Circ Res. (2016) 118:1313–26. doi: 10.1161/CIRCRESAHA.116.307708
57. Su, W-L , Lu, K-C , Chan, C-Y , and Chao, Y-C . Covid-19 and the lungs: a review. J Infect Public Health. (2021) 14:1708–14. doi: 10.1016/j.jiph.2021.09.024
58. Prado, CAS , Fonseca, DLM , Singh, Y , Filgueiras, IS , Baiocchi, GC , Placa, DR, et al. Title: integrative systems immunology uncovers molecular networks of the cell cycle that stratify Covid-19 severity. J Med Virol. (2023) 95:e28450. doi: 10.1002/jmv.28450
59. Enserink, JM , and Kolodner, RD . An overview of Cdk1-controlled targets and processes. Cell Div. (2010) 5:11–41. doi: 10.1186/1747-1028-5-11
60. Yang, W , Cho, H , Shin, H-Y , Chung, J-Y , Kang, ES , Lee, E-j, et al. Accumulation of cytoplasmic Cdk1 is associated with Cancer growth and survival rate in epithelial ovarian Cancer. Oncotarget. (2016) 7:49481–97. doi: 10.18632/oncotarget.10373
61. Zhang, P , Kawakami, H , Liu, W , Zeng, X , Strebhardt, K , Tao, K, et al. Targeting Cdk1 and Mek/Erk overcomes apoptotic resistance in Braf-mutant human colorectal Cancerdual targeting Cdk1 and Mek in Braf-mutant colorectal Cancer. Mol Cancer Res. (2018) 16:378–89. doi: 10.1158/1541-7786.MCR-17-0404
62. Tong, Y , Huang, Y , Zhang, Y , Zeng, X , Yan, M , Xia, Z, et al. Dpp3/Cdk1 contributes to the progression of colorectal Cancer through regulating cell proliferation, cell apoptosis, and cell migration. Cell Death Dis. (2021) 12:529. doi: 10.1038/s41419-021-03796-4
63. Han, J , Wang, F , Lan, Y , Wang, J , Nie, C , Liang, Y, et al. Kifc1 regulated by Mir-532-3p promotes epithelial-to-Mesenchymal transition and metastasis of hepatocellular carcinoma Via Gankyrin/Akt signaling. Oncogene. (2019) 38:406–20. doi: 10.1038/s41388-018-0440-8
64. Akabane, S , Oue, N , Sekino, Y , Asai, R , Thang, PQ , Taniyama, D, et al. Kifc1 regulates Zwint to promote tumor progression and spheroid formation in colorectal Cancer. Pathol Int. (2021) 71:441–52. doi: 10.1111/pin.13098
65. Zhang, N , and Pati, D . Sororin is a master regulator of sister chromatid cohesion and separation. Cell Cycle. (2012) 11:2073–83. doi: 10.4161/cc.20241
66. Shen, A , Liu, L , Chen, H , Qi, F , Huang, Y , Lin, J, et al. Cell division cycle associated 5 promotes colorectal Cancer progression by activating the Erk signaling pathway. Oncogenesis. (2019) 8:19. doi: 10.1038/s41389-019-0123-5
67. Cidado, J , Wong, HY , Rosen, DM , Cimino-Mathews, A , Garay, JP , Fessler, AG, et al. Ki-67 is required for maintenance of Cancer stem cells but not cell proliferation. Oncotarget. (2016) 7:6281–93. doi: 10.18632/oncotarget.7057
68. Sobecki, M , Mrouj, K , Colinge, J , Gerbe, F , Jay, P , Krasinska, L, et al. Cell-cycle regulation accounts for variability in Ki-67 expression Levelski-67 expression and the cell cycle. Cancer Res. (2017) 77:2722–34. doi: 10.1158/0008-5472.CAN-16-0707
69. Zeng, Q , Lei, F , Chang, Y , Gao, Z , Wang, Y , Gao, Q, et al. An oncogenic gene, Snrpa1, regulates Pik3r1, Vegfc, Mki67, Cdk1 and other genes in colorectal Cancer. Biomed Pharmacother. (2019) 117:109076. doi: 10.1016/j.biopha.2019.109076
70. Niu, L , Gao, C , and Li, Y . Identification of potential Core genes in colorectal carcinoma and key genes in colorectal Cancer liver metastasis using bioinformatics analysis. Sci Rep. (2021) 11:23938. doi: 10.1038/s41598-021-03395-5
71. Iliaki, S , Beyaert, R , and Afonina, IS . Polo-like kinase 1 (Plk1) signaling in Cancer and beyond. Biochem Pharmacol. (2021) 193:114747. doi: 10.1016/j.bcp.2021.114747
72. Su, S , Chhabra, G , Singh, CK , Ndiaye, MA , and Ahmad, N . Plk1 inhibition-based combination therapies for Cancer management. Transl Oncol. (2022) 16:101332. Epub 2022/01/02. doi: 10.1016/j.tranon.2021.101332
73. Yu, Z , Deng, P , Chen, Y , Liu, S , Chen, J , Yang, Z, et al. Inhibition of the Plk1-coupled cell cycle machinery overcomes resistance to Oxaliplatin in colorectal Cancer. Adv Sci (Weinh). (2021) 8:e2100759. doi: 10.1002/advs.202100759
74. Taherdangkoo, K , Nezhad, SRK , and Hajjari, M . Mir-485-3p suppresses colorectal Cancer Via targeting Tpx2. Bratisl Lek Listy. (2020) 121:302–7. doi: 10.4149/BLL_2020_048
75. Zhang, Q , Di, J , Ji, Z , Mi, A , Li, Q , Du, X, et al. Kif20a predicts poor survival of patients and promotes colorectal Cancer tumor progression through the Jak/Stat3 signaling pathway. Dis Markers. (2020) 2020:1–11. doi: 10.1155/2020/2032679
76. Kang, DH , Woo, J , Kim, H , Kim, SY , Ji, S , Jaygal, G, et al. Prognostic relevance of Hjurp expression in patients with surgically resected colorectal Cancer. Int J Mol Sci. (2020) 21:7928. doi: 10.3390/ijms21217928
77. Liang, Y-C , Su, Q , Liu, Y-J , Xiao, H , and Yin, H-Z . Centromere protein a (Cenpa) regulates metabolic reprogramming in the Colon Cancer cells by transcriptionally activating Karyopherin subunit alpha 2 (Kpna2). Am J Pathol. (2021) 191:2117–32. doi: 10.1016/j.ajpath.2021.08.010
78. Zhang, H , Luo, Z , Tang, J , Tian, J , Xiao, Y , Sun, C, et al. Transcription factor Nfic functions as a tumor suppressor in lung squamous cell carcinoma progression by modulating Lncrna Casc2. Cell Cycle. (2022) 21:63–73. doi: 10.1080/15384101.2021.1995130
79. Katerndahl, CDS , Rogers, ORS , Day, RB , Cai, MA , Rooney, TP , Helton, NM, et al. Tumor suppressor function of Gata2 in acute Promyelocytic leukemia. Blood. (2021) 138:1148–61. doi: 10.1182/blood.2021011758
80. Hays, E , and Bonavida, B . Yy1 regulates Cancer cell immune resistance by modulating Pd-L1 expression. Drug Resist Updat. (2019) 43:10–28. doi: 10.1016/j.drup.2019.04.001
81. Han, F , Zhang, L , Liao, S , Zhang, Y , Qian, L , Hou, F, et al. The interaction between S100a2 and Kpna2 mediates Nfya nuclear import and is a novel therapeutic target for colorectal Cancer metastasis. Oncogene. (2022) 41:657–70. doi: 10.1038/s41388-021-02116-6
82. Fang, Z , Lin, M , Chen, S , Liu, H , Zhu, M , Hu, Y, et al. E2f1 promotes cell cycle progression by stabilizing spindle Fiber in colorectal Cancer cells. Cell Mol Biol Lett. (2022) 27:90. doi: 10.1186/s11658-022-00392-y
83. Li, Q , Zhang, L , You, W , Xu, J , Dai, J , Hua, D, et al. Prdm1/Blimp1 induces Cancer immune evasion by modulating the Usp22-Spi 1-Pd-L1 Axis in hepatocellular carcinoma cells. Nat Commun. (2022) 13:7677. doi: 10.1038/s41467-022-35469-x
84. Xiao, Q , Gan, Y , Li, Y , Fan, L , Liu, J , Lu, P, et al. Mef2a transcriptionally Upregulates the expression of Zeb2 and Ctnnb1 in colorectal Cancer to promote tumor progression. Oncogene. (2021) 40:3364–77. doi: 10.1038/s41388-021-01774-w
85. Li, LY , Yang, Q , Jiang, YY , Yang, W , Jiang, Y , Li, X, et al. Interplay and cooperation between Srebf1 and master transcription factors regulate lipid metabolism and tumor-promoting pathways in squamous Cancer. Nat Commun. (2021) 12:4362. Epub 2021/07/18. doi: 10.1038/s41467-021-24656-x
86. Choi, KH , Shin, CH , Lee, WJ , Ji, H , and Kim, HH . Dual-Strand tumor suppressor Mir-193b-3p and-5p inhibit malignant phenotypes of lung Cancer by suppressing their common targets. Biosci Rep. (2019) 39:BSR20190634. doi: 10.1042/BSR20190634
87. Toolabi, N , Daliri, FS , Mokhlesi, A , and Talkhabi, M . Identification of key regulators associated with Colon Cancer prognosis and pathogenesis. J Cell Commun Signal. (2022) 16:115–27. Epub 2021/03/27. doi: 10.1007/s12079-021-00612-8
88. Ghafouri-Fard, S , Khoshbakht, T , Hussen, BM , Abdullah, ST , Taheri, M , and Samadian, M . A review on the role of Mir-16-5p in the carcinogenesis. Cancer Cell Int. (2022) 22:342. doi: 10.1186/s12935-022-02754-0
89. Li, C , Wang, R , Wu, A , Yuan, T , Song, K , Bai, Y, et al. Sars-Cov-2 as potential Microrna sponge in Covid-19 patients. BMC Med Genet. (2022) 15:94. doi: 10.1186/s12920-022-01243-7
90. Rodriguez, A , Vigorito, E , Clare, S , Warren, MV , Couttet, P , Soond, DR, et al. Requirement of Bic/Microrna-155 for Normal immune function. Science. (2007) 316:608–11. doi: 10.1126/science.1139253
91. Sacar Demirci, MD , and Adan, A . Computational analysis of Microrna-mediated interactions in Sars-Cov-2 infection. PeerJ. (2020) 8:e9369. doi: 10.7717/peerj.9369
92. Nemani, K , Li, C , Olfson, M , Blessing, EM , Razavian, N , Chen, J, et al. Association of Psychiatric Disorders with mortality among patients with Covid-19. JAMA Psychiat. (2021) 78:380–6. doi: 10.1001/jamapsychiatry.2020.4442
93. Jansen Van Vuren, E , Steyn, SF , Brink, CB , Möller, M , Viljoen, FP , and Harvey, BH . The neuropsychiatric manifestations of Covid-19: interactions with Psy Chiatric illness and pharmacological treatment. Biomed Pharmacother. (2021) 135:111200. doi: 10.1016/j.biopha.2020.111200
94. Coughlin, SS . Anxiety and depression: linkages with viral diseases. Public Health Rev. (2012) 34:1–17. doi: 10.1007/BF03391675
95. Babino, G , Argenziano, G , and Balato, A . Impact in contact dermatitis during and after Sars-Cov2 pandemic. Curr Treat Options Allergy. (2022) 9:19–26. doi: 10.1007/s40521-022-00298-2
96. Grivas, P , Khaki, AR , Wise-Draper, TM , French, B , Hennessy, C , Hsu, CY, et al. Association of Clinical Factors and Recent Anticancer Therapy with Covid-19 severity among patients with Cancer: a report from the Covid-19 and Cancer consortium. Ann Oncol. (2021) 32:787–800. doi: 10.1016/j.annonc.2021.02.024
97. Paul, SM , Pinna, G , and Guidotti, A . Allopregnanolone: from molecular pathophysiology to therapeutics. Hist Perspect Neurobiol Stress. (2020) 12:100215. doi: 10.1016/j.ynstr.2020.100215
98. Son, HJ , Sohn, SH , Kim, N , Lee, H-N , Lee, SM , Nam, RH, et al. Effect of estradiol in an Azoxymethane/dextran sulfate sodium-treated mouse model of colorectal Cancer: implication for sex difference in colorectal Cancer development. Cancer Res Treat. (2019) 51:632–48. doi: 10.4143/crt.2018.060
99. Zhang, Y-L , Wen, X-D , Guo, X , Huang, S-Q , Wang, T-T , Zhou, P-T, et al. Progesterone suppresses the progression of colonic carcinoma by increasing the activity of the Gadd45α/Jnk/C-Jun Signalling pathway. Oncol Rep. (2021) 45:1–13. doi: 10.3892/or.2021.8046
100. Ramírez-de-Arellano, A , Gutiérrez-Franco, J , Sierra-Diaz, E , and Pereira-Suárez, AL . The role of estradiol in the immune response against Covid-19. Hormones. (2021) 20:657–67. doi: 10.1007/s42000-021-00300-7
101. Bilezikian, JP , Bikle, D , Hewison, M , Lazaretti-Castro, M , Formenti, AM , Gupta, A, et al. Mechanisms in endocrinology: vitamin D and Covid-19. Eur J Endocrinol. (2020) 183:R133–47. doi: 10.1530/EJE-20-0665
102. Huang, C-Y , Weng, Y-T , Li, P-C , Hsieh, N-T , Li, C-I , Liu, H-S, et al. Calcitriol suppresses Warburg effect and cell growth in human Colorect Al Cancer cells. Life (Basel). (2021) 11:963. doi: 10.3390/life11090963
103. Elamir, YM , Amir, H , Lim, S , Rana, YP , Lopez, CG , Feliciano, NV, et al. A randomized pilot study using Calcitriol in hospitalized Covid-19 patients. Bone. (2022) 154:116175. doi: 10.1016/j.bone.2021.116175
104. Liu, J , Zhang, L , Gao, J , Zhang, B , Liu, X , Yang, N, et al. Discovery of Genistein derivatives as potential Sars-Cov-2 Main protease inhibitors by virtual screening, molecular dynamics simulations and Admet analysis. Front Pharmacol. (2022) 13:961154. doi: 10.3389/fphar.2022.961154
105. Park, C , Cha, H-J , Lee, H , Hwang-Bo, H , Ji, SY , Kim, MY, et al. Induction of G2/M cell cycle arrest and apoptosis by Genistein in human bladder Cancer T24 cells through inhibition of the Ros-dependent Pi3k/Akt signal transduction pathway. Antioxidants. (2019) 8:327. doi: 10.3390/antiox8090327
106. Uckun, F , Evans, W , Forsyth, C , Waddick, K , Tuel-Ahlgren, L , Chelstrom, L, et al. Biotherapy of B-cell precursor leukemia by targeting Genistein to Cd19-associated tyrosine kinases. Science. (1995) 267:886–91. doi: 10.1126/science.7531365
107. Liu, X , Lan, Y , Zhang, L , Ye, X , Shen, Q , Mo, G, et al. Genistein exerts anti-colorectal Cancer actions: clinical reports, com Putational and validated findings. Aging (Albany NY). (2023) 15:3678–89. doi: 10.18632/aging.204702
108. Huang, H , Liao, D , Liang, L , Song, L , and Zhao, W . Genistein inhibits rotavirus replication and Upregulates Aqp4 expression in rotavirus-infected Caco-2 cells. Arch Virol. (2015) 160:1421–33. doi: 10.1007/s00705-015-2404-4
109. Ma, S , Song, W , Xu, Y , Si, X , Zhang, D , Lv, S, et al. Neutralizing tumor-promoting inflammation with polypeptide-dexamethasone conjugate for microenvironment modulation and colorectal cancer therapy. Biomaterials. (2020) 232:119676. doi: 10.1016/j.biomaterials.2019.119676
110. Namazi, N . The effectiveness of dexamethasone as a combination therapy for Covid-19. Acta Pharma. (2022) 72:345–58. doi: 10.2478/acph-2022-0030
111. Zhang, Z , Ji, Y , Hu, N , Yu, Q , Zhang, X , Li, J, et al. Ferroptosis-induced anticancer effect of resveratrol with a biomimetic Nano-delivery system in colorectal Cancer treatment. Asian J Pharm Sci. (2022) 17:751–66. doi: 10.1016/j.ajps.2022.07.006
112. Wester, RA , van Voorthuijsen, L , Neikes, HK , Dijkstra, JJ , Lamers, LA , Frölich, S, et al. Retinoic acid signaling drives differentiation toward the absorptive L Ineage in colorectal cancer. iScience. (2021) 24:103444. doi: 10.1016/j.isci.2021.103444
113. McCreary, MR , Schnell, PM , and Rhoda, DA . Randomized double-blind placebo-controlled proof-of-concept trial of resveratrol for outpatient treatment of mild coronavirus disease (Covid-19). Sci Rep. (2022) 12:10978. doi: 10.1038/s41598-022-13920-9
Keywords: COVID-19, colorectal cancer, differentially expressed genes, gene ontology, protein–protein interaction, hub gene, drug molecule
Citation: Song Y, Huang T, Pan H, Du A, Wu T, Lan J, Zhou X, Lv Y, Xue S and Yuan K (2023) The influence of COVID-19 on colorectal cancer was investigated using bioinformatics and systems biology techniques. Front. Med. 10:1169562. doi: 10.3389/fmed.2023.1169562
Received: 19 February 2023; Accepted: 15 June 2023;
Published: 30 June 2023.
Edited by:
Elisa Frullanti, University of Siena, ItalyReviewed by:
Peyman Kheirandish Zarandi, Islamic Azad University, IranCopyright © 2023 Song, Huang, Pan, Du, Wu, Lan, Zhou, Lv, Xue and Yuan. This is an open-access article distributed under the terms of the Creative Commons Attribution License (CC BY). The use, distribution or reproduction in other forums is permitted, provided the original author(s) and the copyright owner(s) are credited and that the original publication in this journal is cited, in accordance with accepted academic practice. No use, distribution or reproduction is permitted which does not comply with these terms.
*Correspondence: Kefei Yuan, eWtmMTNAMTYzLmNvbQ==
†These authors have contributed equally to this work
Disclaimer: All claims expressed in this article are solely those of the authors and do not necessarily represent those of their affiliated organizations, or those of the publisher, the editors and the reviewers. Any product that may be evaluated in this article or claim that may be made by its manufacturer is not guaranteed or endorsed by the publisher.
Research integrity at Frontiers
Learn more about the work of our research integrity team to safeguard the quality of each article we publish.