- 1Department of Psychology, Bar-Ilan University, Ramat Gan, Israel
- 2The Joseph Sagol Neuroscience Center, Sheba Medical Center, Tel HaShomer, Israel
- 3Sackler Faculty of Medicine, Tel Aviv University, Tel Aviv, Israel
- 4Department of Psychiatry, Icahn School of Medicine at Mount Sinai, New York, NY, United States
Background: Adiposity has been previously associated with cognitive impairment and Alzheimer’s disease and related disorders (ADRD). Body mass index (BMI) is the most common measure of global adiposity, but inconsistent results were found since it is a global measurement. BMI does not represent regional fat distribution which differs between sexes, race, and age. Regional fat distribution may contribute differently to cognitive decline and Alzheimer’s disease (AD)-related brain changes. Fat-specific targeted therapies could lead to personalized improvement of cognition. The goal of this systematic review is to explore whether regional fat depots, rather than central obesity, should be used to understand the mechanism underlying the association between adiposity and brain.
Methods: This systematic review included 33 studies in the English language, conducted in humans aged 18 years and over with assessment of regional adiposity, cognitive function, dementia, and brain measures. We included only studies that have assessed regional adiposity using imaging technics and excluded studies that were review articles, abstract only or letters to editor. Studies on children and adolescents, animal studies, and studies of patients with gastrointestinal diseases were excluded. PubMed, PsychInfo and web of science were used as electronic databases for literature search until November 2022.
Results: Based on the currently available literature, the findings suggest that different regional fat depots are likely associated with increased risk of cognitive impairment, brain changes and dementia, especially AD. However, different regional fat depots can have different cognitive outcomes and affect the brain differently. Visceral adipose tissue (VAT) was the most studied regional fat, along with liver fat through non-alcoholic fatty liver disease (NAFLD). Pancreatic fat was the least studied regional fat.
Conclusion: Regional adiposity, which is modifiable, may explain discrepancies in associations of global adiposity, brain, and cognition. Specific regional fat depots lead to abnormal secretion of adipose factors which in turn may penetrate the blood brain barrier leading to brain damage and to cognitive decline.
Background
Adiposity refers to the state of being excessively overweight or obese, which is typically caused by an excessive accumulation of body fat and is strongly associated with type II diabetes (T2D), cardiovascular disease, hypertension, and hyperlipidemia (1). Adiposity has been previously associated with cognitive impairment and Alzheimer’s disease and related disorders (ADRD) (2, 3). Characterization of how adiposity impacts ADRD is necessary because adiposity prevention and treatment could be a safe, efficacious approach to prevent ADRD. Body mass index (BMI) is the most common measure of global adiposity. BMI is calculated as weight (kg) divided by the square of height (m2). Obesity is defined as BMI ≥30 kg/m2. Higher BMI in midlife has been associated with poor cognitive outcomes in late life (4, 5). Poorer performance in executive function (6) as well as working memory (7) and verbal fluency (8) have been consistently associated with higher BMI. We have shown that greater weight variability in midlife is associated with an increased risk of dementia three decades later (4). We have also found evidence for associations of greater variability in BMI over time with faster cognitive decline in late life (9). However, inconsistent results were found in old-age where high BMI has been associated with both higher risk (10, 11) but also lower risk (5, 12–14) for dementia. This non-linear association is often attributed to the fact that weight-loss can precede the development of AD (15–17) along with sarcopenia, the loss of skeletal muscle mass and function (18–21).
One explanation for these discrepancies is that BMI may not be a good measure of adiposity (22) since it represents global, rather than regional fat distribution (23, 24) especially in old age (25, 26). While BMI can assess excessive body fat, it does not account for different regional fat depots and muscle mass. Regional fat depots can be at the origin of different metabolic risks (27) since different fat depots have specific metabolic and hormonal characteristics. Previous data suggest that some obese individuals are metabolically healthy, free from high cardiovascular disease and with a normal metabolic risk profile. Contrariwise, metabolically unhealthy individuals with normal weight can be at high risk of cardiovascular disease (27, 28). Investigation of regional fat in these unique populations may shed light into these discrepancies. For them, little is known about the association of regional fat, brain, and cognition and such research is warranted.
Previous studies have shown that visceral adipose tissue (VAT) rather than obesity calculated by BMI, was associated with cardiovascular disease risk and metabolic syndrome (29, 30) while lower amounts of lower-body fat mass (gluteo-femoral) were also found to be a determinant of cardiometabolic diseases (27, 31). Ectopic fat, which refers to the accumulation of fat in areas where it is not normally found, such as the liver, and pancreas, is strongly associated with obesity and insulin resistance (32, 33). However, previous research has shown that nonalcoholic fatty liver disease (NAFLD) was associated with the metabolic syndrome regardless of central obesity assessed by BMI and insulin resistance (34). These findings suggest that some adipose tissues are deleterious while others have a protective role, indicating that although BMI is a widely used tool to assess central obesity, different regional fat depots may have different roles in cardiovascular risk factors and disease, themselves associated with ADRD (35, 36).
Furthermore, the appropriateness of BMI as a phenotypic marker of adiposity across populations differing in race and ethnicity is now questioned (37). Additionally, BMI does not account for sex differences in excessive fat. Women tend to have more fat than man, but the fat distribution is different between the sexes (38). This leaves critical gaps in knowledge about specific adiposity phenotypes that may differentially affect ADRD risk and neuropathology in old age. Body fat distribution has been linked to cognitive function in old age. Specific anatomical location of stored excess fat, including VAT, subcutaneous adipose tissue (SAT), or fat stored within the organs has been linked to cognition (39–41). Greater VAT was associated with lower delayed memory and language scores suggesting that regional adiposity may be linked to specific cognitive domains (39). There is evidence that the development of cognitive decline seems more strongly related to specific body fat distribution than to BMI (40). Exploring regional adiposity might contribute to the understanding of the mechanisms underlying the relationship of adiposity, cognitive function, and associated brain changes. Indeed, increased fat mass in different abdominal regions contributes to the dysregulation of adipokine secretion, increase of inflammation and release of fatty acids into the circulation (42), different human fat pools could lead to different interventions. Therefore, it is important to use additional measurements and assessments to obtain a more accurate picture of an individual’s overall health and body composition.
Thus, the aim of this review is to explore the association between regional adiposity, different fat depots, cognitive function, and associated brain changes.
Method
Eligibility criteria
The present systematic review included studies of all designs if they were in the English language, conducted in humans aged 18 years and over with assessment of regional adiposity, cognitive function, dementia, and brain changes.
We included studies that have assessed regional adiposity using imaging techniques. There are several methods for measuring regional adipose tissues (43). Bioelectrical impedance analysis has been widely used to assess different fat tissues including VAT (44) but was shown to be less accurate in differentiating between the abdominal fat tissues compared to imaging techniques such as computed tomography (CT) (45), magnetic resonance imaging (MRI) (46) and dual energy x-ray absorptiometry (DEXA) (43, 47). Abdominal Ultrasound (US) is an accurate imaging technique for the detection of fatty liver (48). Therefore, we kept studies that used only imaging techniques to assess regional fat depots. Those include abdominal CT, DEXA, abdominal MRI, and abdominal US.
Studies were excluded if they were review articles, abstract only or letters to editor. Studies on children and adolescents and animal studies were excluded. Studies on patients with severe gastrointestinal diseases were excluded because significant inflammatory changes in the intestine can affect body composition (49, 50).
Search strategy
The review followed the Preferred Reporting Items for Systematic Reviews and Meta-Analyses (PRISMA) guidelines (51). PubMed, PsychInfo, and web of science were used as electronic databases for literature search until November 2022. The search terms were: (“Cognitive decline” OR “cognition” OR “memory” OR “executive function” OR “cognitive” OR “Alzheimer’s disease” OR “Dementia” AND “regional adiposity”) further research was done replacing the term “regional adiposity” with “visceral adiposity,” “subcutaneous adipose tissue,” “hepatic fat,” “fatty liver,” “pancreatic fat,” “pancreatic steatosis,” “fat distribution.”
Study selection
Title and abstract screening were carried out by one researcher (EB), duplicates and articles which did not meet the eligibility criteria were excluded. Articles which did not investigate regional adiposity and cognition or brain changes, were conducted on children or which used adiposity assessment that did not include imaging techniques were excluded. Full text screening was conducted independently by two researchers. Articles that fulfilled the selection criteria after the full text was read, were included in this systematic review. The study selection is shown in the flowchart in Figure 1.
The search strategy resulted in 2621 articles. From these, 2,520 articles were deemed ineligible after title and abstract screening. A hundred and one studies were eligible for full-text screening and 69 were excluded for the following reasons: measure of adiposity not with imaging technique (n = 32), meta-analysis or review (n = 16), no cognitive or brain outcomes (n = 15), not available online (n = 2), participants with inflammatory bowel disease (n = 1), animal study (n = 1), and studies on children or adolescents (n = 2). One study was found after independent research. Hence, 33 observational studies were included in this systematic review.
Data extraction and grouping
Data was extracted from 40 studies by one researcher and then re-checked by a second researcher. Both researchers agreed on including 33 studies in the present study based on inclusion criteria previously stated. Data extraction included the following: author, year published, country of the study, population characteristics including number of participants, number of women, mean age, which regions of adiposity were assessed, the technique of assessment, the measurement methods for cognitive function or brain measures. These findings are shown in Tables 1, 2.
Study characteristics
The data extracted from the 33 studies included in this review (36, 41, 52–82) are presented in Tables 1, 2. All studies had an observational design and were cross-sectional studies except for three longitudinal cognition studies (56, 60, 68). The articles were published between 2009 and 2022. The studies were conducted in the United States, Korea, Canada, Poland, Germany, Singapore, Serbia, Sweden, Turkey, Italy, Iceland and Spain, and included a total of 44,327 participants aged between 18 and 89 years (mean 54.11 years).
Statistical analysis
Results including the association of different regional fat depots with cognition and brain changes are presented narratively. For qualitative analysis, differences in measures between higher regional fat depots and control groups or the appropriate results were reported for individual studies. Data is considered statistically significant if the results reported have a value of p smaller than 0.05.
Results
Visceral adiposity and cognition
According to our search, we have grouped 13 studies assessing VAT and cognitive outcomes, nine (52, 54–59, 61, 62) of them using global cognition measurement such as Mini-mental state examination (MMSE) (83), modified MMSE (3MS) (84) and the Montreal Cognitive Assessment, (MoCA) (85). All studies were cross-sectional (52–55, 57–63) except for two longitudinal (36, 56). Among them, six studies have also assessed SAT and cognitive outcomes (36, 54, 56, 57, 59, 60). While in some studies, absence of significant associations of VAT (36, 55, 56, 58, 59, 62) and SAT (57, 59) with cognitive functioning have been shown, in most of our search, higher VAT was found to be associated with lower cognitive scores (52–54, 57, 60, 61, 63). In studies including both VAT and SAT measures, variable results were found. SAT and VAT were associated with lower verbal memory; VAT was independently associated with lower cognition when accounting for SAT but not the other way around (54). In another study, higher VAT but not higher SAT was associated with poor cognitive functioning (57). Interestingly, sex had opposite effects in these associations. Higher SAT (but not VAT) was associated with worsening cognitive functioning after 7 years in men (56). In contrast, in women higher levels of SAT and VAT were associated with less cognitive decline over the years (56). In another study, higher SAT and subcutaneous thigh fat were associated with a decreased likelihood of dementia in women (36). The impact of regional fat depots on cognitive functions can be found in Table 3.
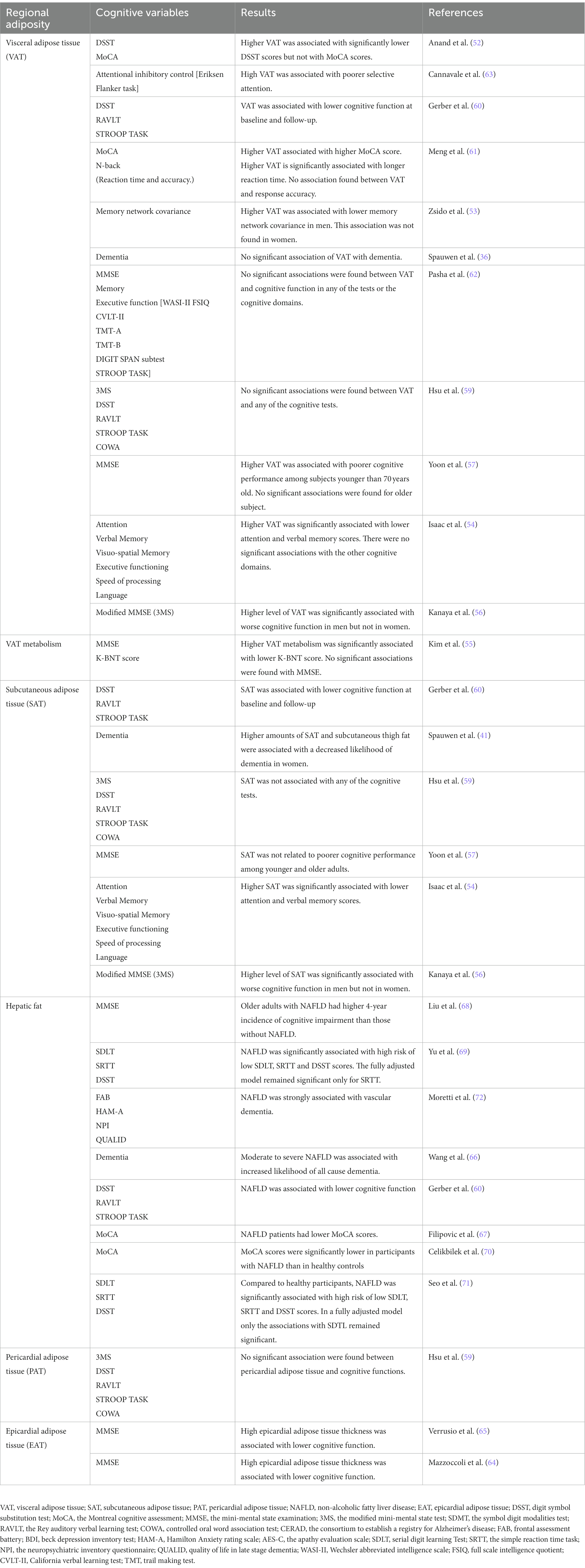
Table 3. Cognition and regional adiposity in studies included in the systematic review: the table is ordered by fat depots from the newest to the oldest publication.
Visceral adiposity and brain changes
Twelve articles reported on the association between VAT and SAT and different brain changes (41, 52–54, 59, 62, 73–78). The association between different brain compartments and regional fat depots can be found in Table 4. All the papers have used structural brain measures via brain MRI (41, 52–54, 59, 62, 73–78), while only one study used also functional measures via functional MRI (fMRI) to assess degree of connectivity (eigenvector centrality, EC) (73). This study showed that high VAT was associated with lower cerebellar structure (gray matter density) as well as lower degree of connectivity of the cerebellum with other brain regions in younger subjects; no associations were found in older individuals (73). These results suggest that the relationship of increased VAT with reduced gray matter density and reduced connectivity in the cerebellum, which is involved in cognitive function, are age-dependent (73). Structural measures have shown that higher VAT and SAT were associated with smaller total brain volume (78). Moreover, elevated VAT was correlated with cortical thinning especially with lower hippocampal volume (54) but not with gray matter and white matter volumes (54, 59). Similarly, higher VAT was linked to smaller temporal lobe and the volume of several other sub-compartments of the brain (76). Six studies (52, 54, 59, 62, 77, 78) have assessed the associations between VAT and White Matter Hyperintensities (WMH) which has been recognized as a risk factor for cognitive impairment and dementia (86). Among them, two have found a significant positive association, namely, associations of high VAT with greater volumes of WMH (62, 77). Similar associations were observed for higher VAT and greater vascular brain injury (52), or lower brain connectivity (fractional anisotropy [FA]), a measure of white matter integrity (74). Higher VAT was associated with higher damage in a brain network implicated in cognitive decline (53), suggesting that VAT is associated with accelerated brain aging (53). In contrast to this relatively broad evidence linking VAT with lower brain volumes and pathologies, two studies found associations of higher VAT with thicker cortex in the posterior cingulate gyrus (75), and with greater left cuneus volume (76).
Visceral adiposity and AD-related pathology
The relationship of VAT with dementia pathology has been examined in two studies (55, 58). Individuals with higher VAT metabolism, a marker of higher VAT dysfunction assessed through PET-CT, compared with individuals with low VAT metabolism, exhibited significantly higher cerebral Aβ burden (55). Those findings suggest that VAT dysfunction could contribute to AD development. In a second study, using brain 18F-fluorodeoxyglucose (FDG) PET as a neurodegenerative biomarker for AD, computing the PALZ score a global index of AD-related hypometabolism, no significant correlation between VAT and the risk of AD pathology was found (58).
Fatty liver and cognition
In the present study, we have identified seven studies examining the relationships of cognition and fatty liver or NAFLD (60, 67–72). In the past literature, we have identified three previous systematic review and meta-analyses (87–89) of observational studies, providing a comprehensive evaluation of the relationship between NAFLD and the risk of dementia or cognitive impairment. However, the studies included in our review include only the ones that have assessed liver fat and NAFLD with imaging technique. In most of the studies, higher severity of NAFLD was associated with increased risk of cognitive impairment (60, 67–71); in some cases, cardiovascular disease attenuated this relationship (60). Strong associations between NAFLD and worsening of cognition in patients meeting the criteria for vascular dementia was found (72). The impact of regional fat depots on cognitive functions can be found in Table 3.
Fatty liver and brain changes
Five studies have reported the association between structural brain changes and fatty liver (41, 67, 79, 80, 82). Among them only one used a noninvasive functional measure, pseudo-Continuous Arterial Spin Labeling (pCASL) measuring cerebral blood flow (CBF) (79). The results are reported in Table 4. Hepatic fat depots were significantly associated with smaller total cerebral brain volume (79) as well as smaller cingulate gyri and hippocampal volumes (41). NAFLD was associated with a smaller total cerebral volume even after adjustment for VAT, pointing to a relationship between NAFLD and brain aging (80). In this study, no significant associations were observed between NAFLD and hippocampal and WMH volumes, nor with covert brain infarcts (small ischemic cerebral lesions here assessed by abnormal signal intensity) (80). Conversely, NAFLD was significantly associated with the presence of WMHs, even after adjusting for cardiometabolic risk factors (82) in another study. In NAFLD patients with lower cognitive score, the volumes of brain gray and white matter were significantly reduced compared to NAFLD patients with higher cognitive score (67). However, no comparison between the NAFLD patients and the control group without NAFLD volumes was done (67). Finally, higher liver fat shown by lower liver attenuation on abdominal CT was associated with decreased total-CBF and gray matter-CBF and this association remained after adjustment for cardiovascular risk factors (79).
Fatty liver, AD-related pathology, and dementia
One study assessed NAFLD and AD-related neuropathology via PET-CT (81). Prevalent NAFLD was not associated with Aβ or tau PET, the main two pathologies characterizing AD (81). We found only one study investigating associations of NAFLD and dementia risk (66). Moderate-to-severe NAFLD was found to be associated with dementia and AD risk, especially with vascular dementia (66). Moreover, participants with vascular dementia and NAFLD had worse neuropsychological outcomes than participants without NAFLD (66).
Other fat depots
Higher epicardial adipose tissue (EAT) has been associated with poorer cognitive functioning in two studies (64, 65). Pericardial adipose tissue (PAT) has been associated with lower hippocampal white matter but not hippocampal gray matter (59). One study examined associations of pancreatic fat with regional brain volume. Albeit in all analyses higher pancreatic fat was associated with lower hippocampal, cingulate gyri and temporal lobe volumes, none of these associations reached statistical significance (41). To our knowledge, no studies examining relationships of kidney fat depots with cognition or brain changes have been done.
Factors potentially linking fat depots, brain changes and cognition
A few studies have explored inflammatory markers as possible factors linking fat depots, brain changes and cognition. Cannavale et al. (63), hypothesized that inflammatory markers would mediate the negative effect of VAT on selective attention. Indeed, plasma C-reactive protein (CRP) and Interleukin-6 (IL-6) concentrations mediated the relationship between higher VAT and lower attentional inhibitory control, suggesting that systemic inflammation could play a role in the deleterious effects of VAT on cognition (63). Higher levels of SAT and VAT were associated with worsening cognitive function in men even after controlling for metabolic disorders, adipocytokines (adiponectin, IL-6, tumor necrosis factor α [TNF-α], and plasminogen activator inhibitor-1[PAI-1]), and sex hormone levels (estradiol and testosterone) (56). Conversely, there was no association between adiposity and cognitive change in women (56). However, in another study where higher VAT was associated with lower cognitive functioning, estradiol level attenuated the negative consequences of VAT on cognition in women (53). Similar results were found for moderate-to- severe NAFLD, which was associated with increased serum levels of multiple cytokines, i.e., higher IL-6 concentrations partially mediated the association of moderate-to-severe NAFLD with vascular dementia (66).
Higher VAT and hepatic fat remained significantly associated with WMH (62, 82), decreased total-CBF and GM-CBF (79) and smaller brain volumes (41, 80) after adjustment for cardiovascular risk factors. Similarly, VAT was found to be significantly associated with reduced cognitive scores, after adjustment for cardiovascular risk factors, and for MRI-detected vascular brain injury (52). In several studies (60, 68, 69, 71) the association between NAFLD and cognitive impairment varied across the cognitive tests when adjusting for cardiovascular risk factors and diseases. Indeed, the NAFLD-cognitive function association was either attenuated (60, 71) or disappeared (68, 69, 71) when adjusting for these factors.
Discussion
Regional adiposity is associated with brain changes and higher risk of cognitive decline
Based on the current available literature, the findings indicate that different regional fat depots are likely associated with increased risk of cognitive impairment and dementia (36, 41, 52–82). Specifically, VAT (52, 54, 57, 61, 63), EAT (64, 65) and liver fat through NAFLD (60, 67–71, 79) were associated with cognitive impairment. Moreover, regional fat was linked to different brain changes (41, 52–63, 73–78), with a relatively consistent association of different fat depots with cortical volume (41, 54, 59, 73, 76, 78–80), and with white matter disease (62, 74, 77). Both lower cortical volume and white matter disease have been linked to cognitive decline, AD and dementia (90, 91). Interestingly, higher VAT, but not the other regional fat depots, was associated with amyloid β, a core neuropathological feature of AD (55, 81). This could be explained by the excessive secretion of leptin by high VAT, which in turn could inhibit the transport of Amyloid-β precursor protein and promote the fabrication of amyloid β (92). Finally, one of the studies has shown that greater severity of NAFLD was associated with higher risk of dementia (66), while among women higher SAT and thigh fat with lower likelihood of dementia (36). Overall, results from this systematic review suggest that different regional fat depots may lead to different neurobiological alterations and ultimately to different cognitive-related outcomes and dementia. Exploring potential mechanisms underlying the inter-relationships of regional adiposity- brain changes - cognition could lead to targeted and personalized treatments for cognitive-related outcomes.
Metabolic syndrome and insulin resistance may link regional adiposity, brain changes, and cognition
The findings of associations of different regional fat depots with lower cognitive scores are concordant with previous research indicating that adiposity (assessed by BMI) is associated with cognitive impairment and risk of dementia (93). Central adiposity is a core feature of the metabolic syndrome (94) and has been associated with cognitive decline, dementia and neuropathology (94, 95), especially in old age (96). Yet, the metabolic syndrome has been associated with NAFLD and pancreatic fat independently of central obesity and insulin resistance (34, 97). Also, accumulation of VAT was found to be the best predictor for metabolic syndrome in women while it was a poor predictor for men compared to SAT (98). One possibility is that the impaired vascular function resulting from the different conditions of the metabolic syndrome could lead to brain changes that could then lead to cognitive impairment (95).
Another core feature of the metabolic syndrome is insulin resistance which also has been linked to cognitive decline and dementia (99). VAT rather than SAT is more strongly associated with insulin resistance (100) and NAFLD has also been closely linked to insulin resistance (101), showing that different fat compartments may be associated with differential metabolic risk. Although both VAT and fatty liver have been shown to be related to impaired cognition and both are determinants of insulin resistance, their impact is different due to the different roles played by adipokines and hepatokines, respectively (101). Those results imply the importance of assessing regional adiposity rather than central adiposity to understand the specific contribution of excess adiposity to cognition. Therefore, further studies should be done on regional fat depots to better understand the mechanism underlying the association between adiposity, metabolic syndrome, cognition, and brain changes. Investigation of regional fat in metabolically healthy obese population may shed light into these discrepancies (27, 28).
Regional adiposity affects brain volumes and vasculature via systemic inflammation
In the present review, we have gathered data showing that regional fat depots are associated with deleterious brain changes. Regional fat depots including VAT, SAT and fatty liver were associated with smaller cerebral volumes (78–80). Fatty liver and VAT but not SAT were significantly associated with smaller hippocampal volume (41, 54, 59) which is one of the first regions affected by AD (90). Those results are concordant with recent evidence from our group showing associations of higher BMI with thinning of the middle temporal gyrus (102). Overall, the present review indicates that different fat depots can affect different parts of the brain suggesting a potential role of different regional fat depots in brain atrophy and pathology, targeting those fats could then prevent deleterious impact on the brain.
Brain small vessel disease which includes higher WMHs, and lacunar infarcts may lead to cognitive impairment and dementia (91). In the present review, different regional fat depots have also been associated with higher WMHs (62, 77, 82). In addition to its associations with cognitive impairment and AD (86), WMH is prevalent in individuals with insulin resistance, metabolic syndrome and T2D (103), all conditions accompanied by high adiposity (103). Furthermore, adiposity is associated with chronic low-grade systemic inflammation, which increases proinflammatory cytokine secretion (104). Pro-inflammatory cytokines have been linked to greater volumes of WMHs (104) which in turn are linked to cognitive impairment. As suggested by one of the studies reviewed, mechanisms by which VAT exerts a negative influence on cognitive function includes systemic inflammation (63). Therefore, disentangling factors secreted by different fat depots affecting systemic inflammation may shed light into their role in cognitive decline and dementia.
Indeed, different fat depots release different secreted factors, some of which cross readily the blood brain barrier (BBB) and may cause damage, ultimately leading to cognitive decline (56, 63, 66). For example, pro-inflammatory factors such as leptin, IL-6, TNF-α (105, 106) which are secreted by adipocytes can cross the BBB and lead to neuroinflammation, which plays a role in cognitive impairment and AD (107). Conversely, anti-inflammatory adipocytokines such as Adiponectin (108), Interleukin 10 (IL-10) (109, 110), and Apelin (111), are associated with less adiposity and are related to cognition and AD. Neuroinflammation likely causes synaptic remodeling and neurodegeneration resulting in disruption of cognitive functioning possibly resulting from damage brain regions subserving cognition such as the hippocampus (112). Targeting these factors could be an efficacious way to prevent or delay later cognitive decline and AD.
Associations of adipose-secreted factors with neuropathology and impaired cognitive functioning
Other factors, such as proteins are secreted from different fat depots, and may explain the role of peripheral fats in the brain. For example, Amylin, a hormone synthesized and co-secreted with insulin by pancreatic β-cells, is elevated in obesity and may share similar pathophysiology with Amyloid-β, characteristic of AD neuropathology (113). Also, Glucagon-like peptide-1 (GLP-1), a gut released hormone, which can protect pancreatic β-cells from apoptosis and induce insulin secretion, is attracting attention as a possible link between metabolic syndrome and brain impairment (114, 115). Additional factors, secreted by hepatocytes, the most common cells in the liver, are found to be related to cognition such as plasminogen activator inhibitor 1 (PAI-1) (116), and fetuin (117, 118). Indeed, in the presence of elevated fatty acids (119), circulating fetuin-A can induce insulin resistance (119) and inflammatory signaling (120) which may cause damage to the brain leading to cognitive impairment (117, 118). Therefore, identifying novel factors altered due to excess fat in different abdominal regions and associated with cerebrovascular pathology, neuropathology, and impaired cognitive functioning is crucial for developing fat-specific interventions. Potential mechanisms underlying the inter-relationships of adiposity- brain changes – cognition and therapeutic modalities, is presented in Figure 2.
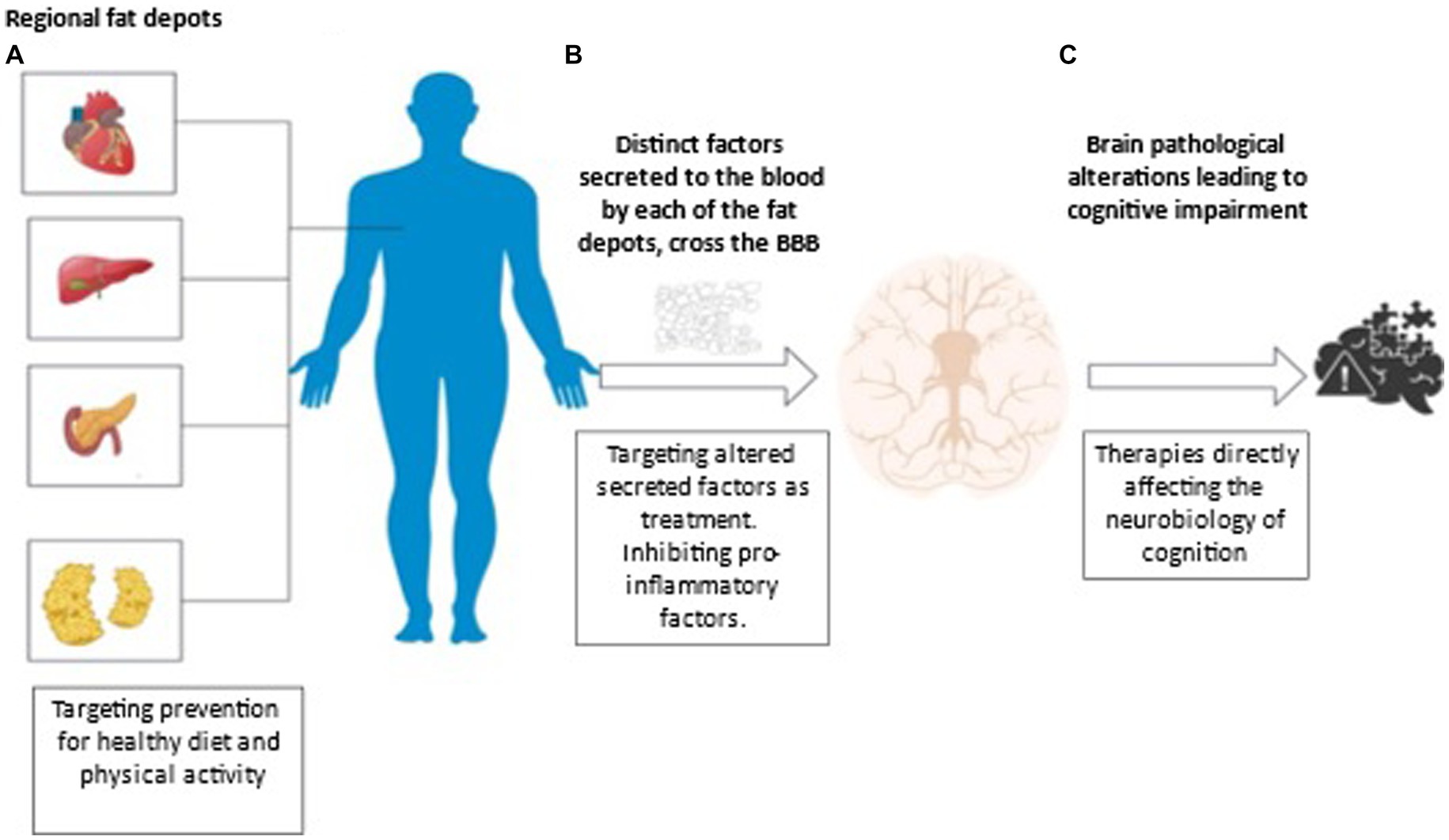
Figure 2. Potential mechanisms underlying the adiposity- brain changes - cognition inter-relationships and therapeutic modalities. (A) Adiposity is commonly used to describe excess body fat or obesity. Different fat depots can be found in the body independently of central obesity. Subcutaneous adiposity refers to the accumulation of fat underneath the skin, in the adipose tissue layer. Visceral adiposity refers to the accumulation of fat in the abdominal cavity, specifically around the organs such as the liver, pancreas, and intestines. Ectopic fat refers to the accumulation of fat in areas where it is not normally found, such as the liver, muscle, and pancreas. Both types of fat are associated with increased risk of metabolic disorders such as insulin resistance, type 2 diabetes, and cardiovascular disease. Understanding the association of adiposity, brain changes and cognitive abilities would provide key insights into the mechanisms by which adiposity impacts cognitive health and to possible treatments. Lifestyle interventions, such as specific diets and physical activity may diminish accumulation of fat depots. (B) Adiposity is associated with chronic low-grade systemic inflammation. Different fat depots can release different proinflammatory cytokines, hormones and enzymes some of which cross readily the blood brain barrier (BBB) and may cause damage ultimately leading to cognitive decline. For example, pro-inflammatory factors such as leptin, secreted by adipocytes, can cross the BBB and lead to neuroinflammation, which plays a major role in cognitive impairment and AD. Conversely, anti-inflammatory adipocytokines such as adiponectin, are associated with less adiposity and are related to better cognitive functioning and lower AD risk. Treatment targeting enhancing protective factors released from adipocytes and diminishing inflammatory factors secreted from fats could be an efficacious way to prevent brain damage and ultimately support healthy cognition. (C) Initial evidence suggests that different fat depots affect a plethora of brain pathologies including cortical volume, the cerebral vasculature, primarily white matter hyperintensities, and neuroinflammation. These pathologies contribute to cognitive impairment and as treatments addressing the neurobiology of cognition evolve, cognitive health may be maintained in spite of accumulation of fat deposits and their respective secreted factors.
Sex differences in regional adiposity, brain changes, and cognition
Finally, sex differences should be taken into consideration as they may also contribute to the fat-brain-cognition axis. Women have overall more fat mass than men. Specifically, women have more SAT, which explains the “pear shape” (38), while they are characterized by lower VAT compared to men (121). Those differences in regional fat depots may lead to different consequences on cognition. Higher levels of VAT were associated with worsening cognitive function in men after adjustment for metabolic disorders, adipocytokines, and sex hormone levels (56). Conversely, there was no association between adiposity and cognitive changes in women (56). Furthermore, while VAT exacerbates the association between aging and poorer brain network covariance in both men and women, estradiol reduces the negative association in women (53). These findings highlight the need to account for sex differences in the investigation of relationships of regional adiposity with brain and cognition.
Limitations
This review provides evidence for the association of different regional fat depots, cognition, and brain changes. However, this study had several limitations. First, the initial intention of our group was to conduct a meta-analysis on regional adiposity and cognition. But, due to the limited number of studies and the variability in methodologies the meta-analysis could not be conclusive as it carried high heterogeneity. The studies have different designs, sample sizes, and cognitive tests as well as different ways of assessing regional fat depots to quantify adiposity, adding complexity to the interpretation of results. Indeed, quantification of the degree of adipose tissues is different in each of the studies, as some quantify by fat volume and others by surface or percentage of fat in the different regions, making it difficult to directly compare the studies. Further prospective studies are needed to establish the relationship between regional fat depots with brain changes and cognition with similar methodologies. Only three studies in this review had longitudinal cognitive decline (56, 60, 68). Considering that the duration of exposure to adiposity may affect the onset and the severity of cognitive impairment, the lack of longitudinal data for regional adiposity is a significant limitation in the field. All studies were observational studies and not clinical trials therefore no causation can be inferred. In some studies, there were no associations between regional fat and cognition. However, many of these studies included relatively young individuals (e.g., 54.11 years, age at baseline for longitudinal studies). In such young ages the range of cognitive functioning is relatively narrow, possibly contributing to the lack of associations. It is important to note that the literature on fat and cognition may suffer from selection bias since older adults with cognitive impairment are less likely to participate in research. Finally, this review focuses specifically on body fat composition, rather than on general body composition, and does not discuss muscle mass and function which are strongly associated with cognitive decline and dementia risk (18–21).
Conclusion
This review of 33 studies indicates that different regional fat depots may affect cognition and different regions of the brain. Regional fat depots, especially VAT and hepatic fat, have been associated with cognitive decline, cortical thinning and WMHs. Regional fat depots, rather than central obesity, may better explicate the association between adiposity and brain and may open horizons for new personalized fat-reducing treatments for prevention of cognitive decline.
Author contributions
EB, SG, and MB conceived the presented idea and have made a substantial contribution to the concept and design of the manuscript. EB carried out the literature search from electronic databases, drafted the manuscript, and provided the tables. SG participated with EB to the full-text screening of the articles from the literature search, read and approved the manuscript. MB revised critically the manuscript and approved the version to be published. All authors contributed to the article and approved the submitted version.
Funding
This work was funded by National Institutes of Health grants R01-AG-034087, AG-053446, and AG-051545 (to MB).
Acknowledgments
The authors thank Marina Nissim (Milan Italy Funding). The authors are also grateful for the generosity of the LeRoy Schecter Foundation. Thanks to Marina Nissim for her generosity.
Conflict of interest
The authors declare that the research was conducted in the absence of any commercial or financial relationships that could be construed as a potential conflict of interest.
Publisher’s note
All claims expressed in this article are solely those of the authors and do not necessarily represent those of their affiliated organizations, or those of the publisher, the editors and the reviewers. Any product that may be evaluated in this article, or claim that may be made by its manufacturer, is not guaranteed or endorsed by the publisher.
References
1. Panuganti, KK, Nguyen, M, Kshirsagar, RK, and Doerr, C. Obesity (nursing) In:. StatPearls : StatPearls Publishing (2021)
2. Luchsinger, JA, and Gustafson, DR. Adiposity and Alzheimer’s disease. Curr Opin Clin Nutr Metab Care. (2009) 12:15–21. doi: 10.1097/MCO.0b013e32831c8c71
3. Gustafson, DR, and Luchsinger, JA. High adiposity: risk factor for dementia and Alzheimer’s disease? Alzheimers Res Ther. (2013) 5:57–3. doi: 10.1186/alzrt221
4. Ravona-Springer, R, Schnaider-Beeri, M, and Goldbourt, U. Body weight variability in midlife and risk for dementia in old age. Neurology. (2013) 80:1677–83. doi: 10.1212/WNL.0b013e3182904cee
5. Pedditizi, E, Peters, R, and Beckett, N. The risk of overweight/obesity in mid-life and late life for the development of dementia: a systematic review and meta-analysis of longitudinal studies. Age Ageing. (2016) 45:14–21. doi: 10.1093/ageing/afv151
6. Fitzpatrick, S, Gilbert, S, and Serpell, L. Systematic review: are overweight and obese individuals impaired on behavioural tasks of executive functioning? Neuropsychol Rev. (2013) 23:138–56. doi: 10.1007/s11065-013-9224-7
7. Coppin, G, Nolan-Poupart, S, Jones-Gotman, M, and Small, DM. Working memory and reward association learning impairments in obesity. Neuropsychologia. (2014) 65:146–55. doi: 10.1016/j.neuropsychologia.2014.10.004
8. Cournot, MCMJ, Marquie, JC, Ansiau, D, Martinaud, C, Fonds, H, Ferrieres, J, et al. Relation between body mass index and cognitive function in healthy middle-aged men and women. Neurology. (2006) 67:1208–14. doi: 10.1212/01.wnl.0000238082.13860.50
9. Beeri, MS, Tirosh, A, Lin, HM, Golan, S, Boccara, E, Sano, M, et al. Stability in BMI over time is associated with a better cognitive trajectory in older adults. Alzheimers Dement. (2022) 18:2131–9. doi: 10.1002/alz.12525
10. Gustafson, D, Rothenberg, E, Blennow, K, Steen, B, and Skoog, I. An 18-year follow-up of overweight and risk of Alzheimer disease. Arch Intern Med. (2003) 163:1524–8. doi: 10.1001/archinte.163.13.1524
11. West, RK, Ravona-Springer, R, Heymann, A, Schmeidler, J, Leroith, D, Koifman, K, et al. Waist circumference is correlated with poorer cognition in elderly type 2 diabetes women. Alzheimers Dement. (2016) 12:925–9. doi: 10.1016/j.jalz.2016.03.017
12. Elias, MF, Elias, PK, Sullivan, LM, Wolf, PA, and D’Agostino, RB. Obesity, diabetes and cognitive deficit: the Framingham heart study. Neurobiol Aging. (2005) 26:11–6. doi: 10.1016/j.neurobiolaging.2005.08.019
13. Kuo, HK, Jones, RN, Milberg, WP, Tennstedt, S, Talbot, L, Morris, JN, et al. Cognitive function in normal-weight, overweight, and obese older adults: an analysis of the advanced cognitive training for independent and vital elderly cohort. J Am Geriatr Soc. (2006) 54:97–103. doi: 10.1111/j.1532-5415.2005.00522.x
14. Wotton, CJ, and Goldacre, MJ. Age at obesity and association with subsequent dementia: record linkage study. Postgrad Med J. (2014) 90:547–51. doi: 10.1136/postgradmedj-2014-132571
15. Cova, I, Clerici, F, Rossi, A, Cucumo, V, Ghiretti, R, Maggiore, L, et al. Weight loss predicts progression of mild cognitive impairment to Alzheimer’s disease. PLoS One. (2016) 11:e0151710. doi: 10.1371/journal.pone.0151710
16. Johnson, DK, Wilkins, CH, and Morris, JC. Accelerated weight loss may precede diagnosis in Alzheimer disease. Arch Neurol. (2006) 63:1312–7. doi: 10.1001/archneur.63.9.1312
17. Buchman, AS, Wilson, RS, Bienias, JL, Shah, RC, Evans, DA, and Bennett, DA. Change in body mass index and risk of incident Alzheimer disease. Neurology. (2005) 65:892–7. doi: 10.1212/01.wnl.0000176061.33817.90
18. Beeri, MS, Leugrans, SE, Delbono, O, Bennett, DA, and Buchman, AS. Sarcopenia is associated with incident Alzheimer’s dementia, mild cognitive impairment, and cognitive decline. J Am Geriatr Soc. (2021) 69:1826–35. doi: 10.1111/jgs.17206
19. Oudbier, SJ, Goh, J, Looijaard, SMLM, Reijnierse, EM, Meskers, CGM, and Maier, AB. Pathophysiological mechanisms explaining the association between low skeletal muscle mass and cognitive function. J. Gerontol. (2022) 77:1959–68. doi: 10.1093/gerona/glac121
20. Yang, Y, Xiao, M, Leng, L, Jiang, S, Feng, L, Pan, G, et al. A systematic review and meta-analysis of the prevalence and correlation of mild cognitive impairment in sarcopenia. J Cachexia Sarcopenia Muscle. (2023) 14:45–56. doi: 10.1002/jcsm.13143
21. Brisendine, MH, and Drake, JC. Early stage Alzheimer's disease: are skeletal muscle and exercise the key? J Appl Physiol. (2023) 134:515–20. doi: 10.1152/japplphysiol.00659.2022
22. Janssen, I, Katzmarzyk, PT, and Ross, R. Waist circumference and not body mass index explains obesity-related health risk. Am J Clin Nutr. (2004) 79:379–84. doi: 10.1093/ajcn/79.3.379
23. Staaf, J, Labmayr, V, Paulmichl, K, Manell, H, Cen, J, Ciba, I, et al. Pancreatic fat is associated with metabolic syndrome and visceral fat but not beta-cell function or body mass index in pediatric obesity. Pancreas. (2017) 46:358–65. doi: 10.1097/MPA.0000000000000771
24. Prentice, AM, and Jebb, SA. Beyond body mass index. Obes Rev. (2001) 2:141–7. doi: 10.1046/j.1467-789x.2001.00031.x
25. Tchkonia, T, Morbeck, DE, Von Zglinicki, T, Van Deursen, J, Lustgarten, J, Scrable, H, et al. Fat tissue, aging, and cellular senescence. Aging Cell. (2010) 9:667–84. doi: 10.1111/j.1474-9726.2010.00608.x
26. Kuk, JL, Saunders, TJ, Davidson, LE, and Ross, R. Age-related changes in total and regional fat distribution. Ageing Res Rev. (2009) 8:339–48. doi: 10.1016/j.arr.2009.06.001
27. Stefan, N. Causes, consequences, and treatment of metabolically unhealthy fat distribution. Lancet Diab Endocrinol. (2020) 8:616–27. doi: 10.1016/S2213-8587(20)30110-8
28. Schulze, MB. Metabolic health in normal-weight and obese individuals. Diabetologia. (2019) 62:558–66. doi: 10.1007/s00125-018-4787-8
29. Franzosi, MG. Should we continue to use BMI as a cardiovascular risk factor? Lancet. (2006) 368:624–5. doi: 10.1016/S0140-6736(06)69222-2
30. Després, JP. Is visceral obesity the cause of the metabolic syndrome? Ann Med. (2006) 38:52–63. doi: 10.1080/07853890500383895
31. Manolopoulos, KN, Karpe, F, and Frayn, KN. Gluteofemoral body fat as a determinant of metabolic health. Int J Obes. (2010) 34:949–59. doi: 10.1038/ijo.2009.286
32. Smits, MM, and Van Geenen, EJ. The clinical significance of pancreatic steatosis. Nat Rev Gastroenterol Hepatol. (2011) 8:169–77. doi: 10.1038/nrgastro.2011.4
33. Stefan, N, and Cusi, K. A global view of the interplay between non-alcoholic fatty liver disease and diabetes. Lancet Diab Endocrinol. (2022) 10:284–96. doi: 10.1016/S2213-8587(22)00003-1
34. Yang, KC, Hung, HF, Lu, CW, Chang, HH, Lee, LT, and Huang, KC. Association of non-alcoholic fatty liver disease with metabolic syndrome independently of central obesity and insulin resistance. Sci Rep. (2016) 6:1–10. doi: 10.1038/srep27034
35. Kivipelto, M, Ngandu, T, Fratiglioni, L, Viitanen, M, Kåreholt, I, Winblad, B, et al. Obesity and vascular risk factors at midlife and the risk of dementia and Alzheimer disease. Arch Neurol. (2005) 62:1556–60. doi: 10.1001/archneur.62.10.1556
36. Spauwen, PJ, Murphy, RA, Jónsson, PV, Sigurdsson, S, Garcia, ME, Eiriksdottir, G, et al. Associations of fat and muscle tissue with cognitive status in older adults: the AGES-Reykjavik study. Age Ageing. (2017) 46:250–7. doi: 10.1093/ageing/afw219
37. Heymsfield, SB, Peterson, CM, Thomas, DM, Heo, MS, and Schuna, JM Jr. Why are there race/ethnic differences in adult body mass index-adiposity relationships? A quantitative critical review. Obes Rev. (2016) 17:262–75. doi: 10.1111/obr.12358
38. Karastergiou, K, Smith, SR, Greenberg, AS, and Fried, SK. Sex differences in human adipose tissues–the biology of pear shape. Biol Sex Differ. (2012) 3:1–12. doi: 10.1186/2042-6410-3-13
39. Moh, MC, Low, S, Ng, TP, Wang, J, Ang, SF, Tan, C, et al. Association of traditional and novel measures of central obesity with cognitive performance in older multi-ethnic Asians with type 2 diabetes. Clin Obesity. (2020) 10:e12352. doi: 10.1111/cob.12352
40. Cereda, E, Sansone, V, Meola, G, and Malavazos, AE. Increased visceral adipose tissue rather than BMI as a risk factor for dementia. Age Ageing. (2007) 36:488–91. doi: 10.1093/ageing/afm096
41. Beller, E, Lorbeer, R, Keeser, D, Schoeppe, F, Sellner, S, Hetterich, H, et al. Hepatic fat is superior to BMI, visceral and pancreatic fat as a potential risk biomarker for neurodegenerative disease. Eur Radiol. (2019) 29:6662–70. doi: 10.1007/s00330-019-06276-8
42. Tchkonia, T, Thomou, T, Zhu, YI, Karagiannides, I, Pothoulakis, C, Jensen, MD, et al. Mechanisms and metabolic implications of regional differences among fat depots. Cell Metab. (2013) 17:644–56. doi: 10.1016/j.cmet.2013.03.008
43. Wang, H, Chen, YE, and Eitzman, DT. Imaging body fat: techniques and cardiometabolic implications. Arterioscler Thromb Vasc Biol. (2014) 34:2217–23. doi: 10.1161/ATVBAHA.114.303036
44. Jackson, AS, Pollock, ML, Graves, JE, and Mahar, MT. Reliability and validity of bioelectrical impedance in determining body composition. J Appl Physiol. (1988) 64:529–34. doi: 10.1152/jappl.1988.64.2.529
45. Kim, YJ, Park, JW, Kim, JW, Park, CS, Gonzalez, JPS, Lee, SH, et al. Computerized automated quantification of subcutaneous and visceral adipose tissue from computed tomography scans: development and validation study. JMIR Med Inform. (2016) 4:e4923. doi: 10.2196/medinform.4923
46. Chaudry, O, Grimm, A, Friedberger, A, Kemmler, W, Uder, M, Jakob, F, et al. Magnetic resonance imaging and bioelectrical impedance analysis to assess visceral and abdominal adipose tissue. Obesity. (2020) 28:277–83. doi: 10.1002/oby.22712
47. Borga, M, West, J, Bell, JD, Harvey, NC, Romu, T, Heymsfield, SB, et al. Advanced body composition assessment: from body mass index to body composition profiling. J Investig Med. (2018) 66:1–9. doi: 10.1136/jim-2018-000722
48. Hernaez, R, Lazo, M, Bonekamp, S, Kamel, I, Brancati, FL, Guallar, E, et al. Diagnostic accuracy and reliability of ultrasonography for the detection of fatty liver: a meta-analysis. Hepatology. (2011) 54:1082–90. doi: 10.1002/hep.24452
49. Zhang, M, and Yang, XJ. Effects of a high fat diet on intestinal microbiota and gastrointestinal diseases. World J Gastroenterol. (2016) 22:8905–9. doi: 10.3748/wjg.v22.i40.8905
50. Ghosh, S, and DeCoffe, D. Influence of the gut microbiota and its metabolites on obesity and metabolic disease. Nutrients. (2021) 13:429. doi: 10.3390/nu13020429
51. Moher, D, Liberati, A, Tetzlaff, J, and Altman, DG, PRISMA Group*, T. Preferred reporting items for systematic reviews and meta-analyses: the PRISMA statement. Ann Intern Med. (2009) 151:264–9. doi: 10.7326/0003-4819-151-4-200908180-00135
52. Anand, SS, Friedrich, MG, Lee, DS, Awadalla, P, Després, JP, Desai, D, et al. Evaluation of adiposity and cognitive function in adults. JAMA Netw Open. (2022) 5:–e2146324. doi: 10.1001/jamanetworkopen.2021.46324
53. Zsido, RG, Heinrich, M, Slavich, GM, Beyer, F, Masouleh, SK, Kratzsch, J, et al. Association of estradiol and visceral fat with structural brain networks and memory performance in adults, e196126. JAMA Netw Open. (2019) 2. doi: 10.1001/jamanetworkopen.2019.6126
54. Isaac, V, Sim, S, Zheng, H, Zagorodnov, V, Tai, ES, and Chee, M. Adverse associations between visceral adiposity, brain structure, and cognitive performance in healthy elderly. Front Aging Neurosci. (2011) 3:12. doi: 10.3389/fnagi.2011.00012
55. Kim, S, Yi, HA, Won, KS, Lee, JS, and Kim, HW. Association between visceral adipose tissue metabolism and Alzheimer’s disease pathology. Meta. (2022) 12:258. doi: 10.3390/metabo12030258
56. Kanaya, AM, Lindquist, K, Harris, TB, Launer, L, Rosano, C, Satterfield, S, et al. Total and regional adiposity and cognitive change in older adults: the health, aging and body composition (ABC) study. Arch Neurol. (2009) 66:329–35. doi: 10.1001/archneurol.2008.570
57. Yoon, DH, Choi, SH, Yu, JH, Ha, JH, Ryu, SH, and Park, DH. The relationship between visceral adiposity and cognitive performance in older adults. Age Ageing. (2012) 41:456–61. doi: 10.1093/ageing/afs018
58. Kim, J, Choi, KH, Cho, SG, Kang, SR, Yoo, SW, Kwon, SY, et al. Association of muscle and visceral adipose tissues with the probability of Alzheimer’s disease in healthy subjects. Sci Rep. (2019) 9:949. doi: 10.1038/s41598-018-37244-9
59. Hsu, FC, Yuan, M, Bowden, DW, Xu, J, Smith, SC, Wagenknecht, LE, et al. Adiposity is inversely associated with hippocampal volume in African Americans and European Americans with diabetes. J Diabetes Complicat. (2016) 30:1506–12. doi: 10.1016/j.jdiacomp.2016.08.012
60. Gerber, Y, VanWagner, LB, Yaffe, K, Terry, JG, Rana, JS, Reis, JP, et al. Non-alcoholic fatty liver disease and cognitive function in middle-aged adults: the CARDIA study. BMC Gastroenterol. (2021) 21:1–9. doi: 10.1186/s12876-021-01681-0
61. Meng, H, and Gorniak, SL. Effects of adiposity on postural control and cognition in older adults. Gait Posture. (2020) 82:147–52. doi: 10.1016/j.gaitpost.2020.09.004
62. Panuganti, KK, Nguyen, M, Kshirsagar, RK, and Doerr, C. “Obesity (Nursing),” in StatPearls [Internet]. StatPearls Publishing, (2021).
63. Cannavale, CN, Bailey, M, Edwards, CG, Thompson, SV, Walk, AM, Burd, NA, et al. Systemic inflammation mediates the negative relationship between visceral adiposity and cognitive control. Int J Psychophysiol. (2021) 165:68–75. doi: 10.1016/j.ijpsycho.2021.03.010
64. Mazzoccoli, G, Dagostino, MP, Vinciguerra, M, Ciccone, F, Paroni, G, Seripa, D, et al. An association study between epicardial fat thickness and cognitive impairment in the elderly. Am J Phys Heart Circ Phys. (2014) 307:H1269–76. doi: 10.1152/ajpheart.00175.2014
65. Verrusio, W, Renzi, A, Magro, VM, Musumeci, M, Andreozzi, P, and Cacciafesta, M. Association between epicardial fat thickness and cognitive function in elderly. A preliminary study. Ann Ist Super Sanita. (2019) 55:59–62. doi: 10.4415/ANN_19_01_11
66. Wang, Y, Li, Y, Liu, K, Han, X, Dong, Y, Wang, X, et al. Nonalcoholic fatty liver disease, serum cytokines, and dementia among rural-dwelling older adults in China: a population-based study. Eur J Neurol. (2022) 29:2612–21. doi: 10.1111/ene.15416
67. Filipović, B, Marković, O, Đurić, V, and Filipović, B. Cognitive changes and brain volume reduction in patients with nonalcoholic fatty liver disease. Can J Gastroenterol Hepatol. (2018) 2018:1–6. doi: 10.1155/2018/9638797
68. Pasha, EP, Birdsill, A, Parker, P, Elmenshawy, A, Tanaka, H, and Haley, AP. “Visceral adiposity predicts subclinical white matter hyperintensities in middle-aged adults,” in Obes Res Clin Pract. (2017) 11:177–187.
69. Yu, Q, He, R, Jiang, H, Wu, J, Xi, Z, He, K, et al. Association between metabolic dysfunction-associated fatty liver disease and cognitive impairment. J Clin Transl Hepatol. (2022) 10:1034–41. doi: 10.14218/JCTH.2021.00490
70. Celikbilek, A, Celikbilek, M, and Bozkurt, G. Cognitive assessment of patients with nonalcoholic fatty liver disease. Eur J Gastroenterol Hepatol. (2018) 30:944–50. doi: 10.1097/MEG.0000000000001131
71. Seo, SW, Gottesman, RF, Clark, JM, Hernaez, R, Chang, Y, Kim, C, et al. Nonalcoholic fatty liver disease is associated with cognitive function in adults. Neurology. (2016) 86:1136–42. doi: 10.1212/WNL.0000000000002498
72. Moretti, R, Giuffré, M, Crocè, LS, Gazzin, S, and Tiribelli, C. Nonalcoholic fatty liver disease and altered neuropsychological functions in patients with subcortical vascular dementia. J Personal Med. (2022) 12:1106. doi: 10.3390/jpm12071106
73. Raschpichler, M, Straatman, K, Schroeter, ML, Arelin, K, Schlögl, H, Fritzsch, D, et al. Abdominal fat distribution and its relationship to brain changes: the differential effects of age on cerebellar structure and function: a cross-sectional, exploratory study. BMJ Open. (2013) 3:e001915. doi: 10.1136/bmjopen-2012-001915
74. Cárdenas, D, Madinabeitia, I, Vera, J, de Teresa, C, Alarcón, F, Jiménez, R, et al. Better brain connectivity is associated with higher total fat mass and lower visceral adipose tissue in military pilots. Sci Rep. (2020) 10:610. doi: 10.1038/s41598-019-57345-3
75. Kaur, S, Gonzales, MM, Strasser, B, Pasha, E, McNeely, J, Tanaka, H, et al. Central adiposity and cortical thickness in midlife. Psychosom Med. (2015) 77:671–8. doi: 10.1097/PSY.0000000000000202
76. Lee, H, Seo, HS, Kim, RE, Lee, SK, Lee, YH, and Shin, C. Obesity and muscle may have synergic effect more than independent effects on brain volume in community-based elderly. Eur Radiol. (2021) 31:2956–66. doi: 10.1007/s00330-020-07407-2
77. Kim, KW, Seo, H, Kwak, MS, and Kim, D. Visceral obesity is associated with white matter hyperintensity and lacunar infarct. Int J Obes. (2017) 41:683–8. doi: 10.1038/ijo.2017.13
78. Debette, S, Beiser, A, Hoffmann, U, DeCarli, C, O’Donnell, CJ, Massaro, JM, et al. Visceral fat is associated with lower brain volume in healthy middle-aged adults. Ann Neurol. (2010) 68:136–44. doi: 10.1002/ana.22062
79. VanWagner, LB, Terry, JG, Chow, LS, Alman, AC, Kang, H, Ingram, KH, et al. Nonalcoholic fatty liver disease and measures of early brain health in middle-aged adults: the CARDIA study. Obesity. (2017) 25:642–51. doi: 10.1002/oby.21767
80. Weinstein, G, Zelber-Sagi, S, Preis, SR, Beiser, AS, DeCarli, C, Speliotes, EK, et al. Association of nonalcoholic fatty liver disease with lower brain volume in healthy middle-aged adults in the Framingham study. JAMA Neurol. (2018) 75:97–104. doi: 10.1001/jamaneurol.2017.3229
81. Weinstein, G, O’Donnell, A, Davis-Plourde, K, Zelber-Sagi, S, Ghosh, S, DeCarli, CS, et al. Non-alcoholic fatty liver disease, liver fibrosis, and regional amyloid-β and tau pathology in middle-aged adults: the Framingham study. J Alzheimers Dis. (2022) 86:1371–83. doi: 10.3233/JAD-215409
82. Jang, H, Kang, D, Chang, Y, Kim, Y, Lee, JS, Kim, KW, et al. Non-alcoholic fatty liver disease and cerebral small vessel disease in Korean cognitively normal individuals. Sci Rep. (2019) 9:1814. doi: 10.1038/s41598-018-38357-x
83. Folstein, MF, Robins, LN, and Helzer, JE. The mini-mental state examination. Arch Gen Psychiatry. (1983) 40:812. doi: 10.1001/archpsyc.1983.01790060110016
84. Teng, E, and Chui, H. The modified mini-mental state examination (3MS). Can J Psychiatr. (1987) 41:114–21.
85. Nasreddine, ZS, Phillips, NA, Bédirian, V, Charbonneau, S, Whitehead, V, Collin, I, et al. The Montreal cognitive assessment, MoCA: a brief screening tool for mild cognitive impairment. J Am Geriatr Soc. (2005) 53:695–9. doi: 10.1111/j.1532-5415.2005.53221.x
86. Garnier-Crussard, A, Bougacha, S, Wirth, M, Dautricourt, S, Sherif, S, Landeau, B, et al. White matter hyperintensity topography in Alzheimer’s disease and links to cognition. Alzheimers Dement. (2022) 18:422–33. doi: 10.1002/alz.12410
87. Lu, LY, Wu, MY, Kao, YS, and Hung, CH. Non-alcoholic fatty liver disease and the risk of dementia: a meta-analysis of cohort studies. Clin Mol Hepatol. (2022) 28:931–2. doi: 10.3350/cmh.2022.0259
88. George, ES, Sood, S, Daly, RM, and Tan, SY. Is there an association between non-alcoholic fatty liver disease and cognitive function? Syst Rev BMC Geriatr. (2022) 22:47. doi: 10.1186/s12877-021-02721-w
89. Wang, L, Sang, B, and Zheng, Z. Risk of dementia or cognitive impairment in non-alcoholic fatty liver disease: a systematic review and meta-analysis. Front Aging Neurosci. (2022) 14:985109. doi: 10.3389/fnagi.2022.985109
90. Kaye, JA, Swihart, T, Howieson, D, Dame, A, Moore, MM, Karnos, T, et al. Volume loss of the hippocampus and temporal lobe in healthy elderly persons destined to develop dementia. Neurology. (1997) 48:1297–304. doi: 10.1212/WNL.48.5.1297
91. Vermeer, SE, Prins, ND, den Heijer, T, Hofman, A, Koudstaal, PJ, and Breteler, MM. Silent brain infarcts and the risk of dementia and cognitive decline. N Engl J Med. (2003) 348:1215–22. doi: 10.1056/NEJMoa022066
92. Fewlass, DC, Noboa, K, Pi-Sunyer, FX, Johnston, JM, Yan, SD, and Tezapsidis, N. Obesity-related leptin regulates Alzheimer's Aβ. FASEB J. (2004) 18:1870–8. doi: 10.1096/fj.04-2572com
93. Qizilbash, N, Gregson, J, Johnson, ME, Pearce, N, Douglas, I, Wing, K, et al. BMI and risk of dementia in two million people over two decades: a retrospective cohort study. Lancet Diabetes Endocrinol. (2015) 3:431–6. doi: 10.1016/S2213-8587(15)00033-9
94. Yaffe, K. Metabolic syndrome and cognitive disorders: is the sum greater than its parts? Alzheimer Dis Assoc Disord. (2007) 21:167–71. doi: 10.1097/WAD.0b013e318065bfd6
95. Lee, EB, and Mattson, MP. The neuropathology of obesity: insights from human disease. Acta Neuropathol. (2014) 127:3–28. doi: 10.1007/s00401-013-1190-x
96. Assuncao, N, Sudo, FK, Drummond, C, de Felice, FG, and Mattos, P. Metabolic syndrome and cognitive decline in the elderly: a systematic review. PLoS One. (2018) 13:e0194990. doi: 10.1371/journal.pone.0194990
97. Goodpaster, BH, Krishnaswami, S, Harris, TB, Katsiaras, A, Kritchevsky, SB, Simonsick, EM, et al. Obesity, regional body fat distribution, and the metabolic syndrome in older men and women. Arch Intern Med. (2005) 165:777–83. doi: 10.1001/archinte.165.7.777
98. Pickhardt, PJ, Jee, Y, O’Connor, SD, and del Rio, AM. Visceral adiposity and hepatic steatosis at abdominal CT: association with the metabolic syndrome. Am J Roentgenol. (2012) 198:1100–7. doi: 10.2214/AJR.11.7361
99. Beeri, MS, and Bendlin, BB. The link between type 2 diabetes and dementia: from biomarkers to treatment. Lancet Diabetes Endocrinol. (2020) 8:736–8. doi: 10.1016/S2213-8587(20)30267-9
100. Liu, L, Feng, J, Zhang, G, Yuan, X, Li, F, Yang, T, et al. Visceral adipose tissue is more strongly associated with insulin resistance than subcutaneous adipose tissue in Chinese subjects with pre-diabetes. Curr Med Res Opin. (2018) 34:123–9. doi: 10.1080/03007995.2017.1364226
101. Stefan, N, Schick, F, Birkenfeld, AL, Häring, HU, and White, MF. The role of hepatokines in NAFLD. Cell Metab. (2023) 35:236–52. doi: 10.1016/j.cmet.2023.01.006
102. West, RK, Livny, A, Ravona-Springer, R, Bendlin, BB, Heymann, A, Leroith, D, et al. Higher BMI is associated with smaller regional brain volume in older adults with type 2 diabetes. Diabetologia. (2020) 63:2446–51. doi: 10.1007/s00125-020-05264-8
103. Alfaro, FJ, Gavrieli, A, Saade-Lemus, P, Lioutas, VA, Upadhyay, J, and Novak, V. White matter microstructure and cognitive decline in metabolic syndrome: a review of diffusion tensor imaging. Metabolism. (2018) 78:52–68. doi: 10.1016/j.metabol.2017.08.009
104. Khanna, D, Khanna, S, Khanna, P, Kahar, P, and Patel, BM. Obesity: a chronic low-grade inflammation and its markers. Cureus. (2022) 14:e22711. doi: 10.7759/cureus.22711
105. Trayhurn, P, and Wood, IS. Adipokines: inflammation and the pleiotropic role of white adipose tissue. Br J Nutr. (2004) 92:347–55. doi: 10.1079/BJN20041213
106. Fantuzzi, G. Adipose tissue, adipokines, and inflammation. J Allergy Clin Immunol. (2005) 115:911–9. doi: 10.1016/j.jaci.2005.02.023
107. Heneka, MT, Carson, MJ, El Khoury, J, Landreth, GE, Brosseron, F, Feinstein, DL, et al. Neuroinflammation in Alzheimer’s disease. Lancet Neurol. (2015) 14:388–405. doi: 10.1016/S1474-4422(15)70016-5
108. Nigro, E, Scudiero, O, Monaco, ML, Palmieri, A, Mazzarella, G, Costagliola, C, et al. New insight into adiponectin role in obesity and obesity-related diseases. Biomed Res Int. (2014) 2014:1–14. doi: 10.1155/2014/658913
109. Juge-Aubry, CE, Somm, E, Giusti, V, Pernin, A, Chicheportiche, R, Verdumo, C, et al. Adipose tissue is a major source of interleukin-1 receptor antagonist: upregulation in obesity and inflammation. Diabetes. (2003) 52:1104–10. doi: 10.2337/diabetes.52.5.1104
110. Esposito, K, Pontillo, A, Giugliano, F, Giugliano, G, Marfella, R, Nicoletti, G, et al. Association of low interleukin-10 levels with the metabolic syndrome in obese women. J Clin Endocrinol Metabol. (2003) 88:1055–8. doi: 10.1210/jc.2002-021437
111. Dai, L, Smith, PM, Kuksis, M, and Ferguson, AV. Apelin acts in the subfornical organ to influence neuronal excitability and cardiovascular function. J Physiol. (2013) 591:3421–32. doi: 10.1113/jphysiol.2013.254144
112. Nguyen, JC, Killcross, AS, and Jenkins, TA. Obesity and cognitive decline: role of inflammation and vascular changes. Front Neurosci. (2014) 8:375. doi: 10.3389/fnins.2014.00375
113. Despa, F, and DeCarli, C. Amylin: what might be its role in Alzheimer’s disease and how could this affect therapy? Expert Rev Proteomics. (2013) 10:403–5. doi: 10.1586/14789450.2013.841549
114. Cabou, C, and Burcelin, R. GLP-1, the gut-brain, and brain-periphery axes. Rev Diabetic Stud. (2011) 8:418–31. doi: 10.1900/RDS.2011.8.418
115. Robinson, A, Lubitz, I, Atrakchi-Baranes, D, Licht-Murava, A, Katsel, P, Leroith, D, et al. Combination of insulin with a GLP1 agonist is associated with better memory and normal expression of insulin receptor pathway genes in a mouse model of Alzheimer’s disease. J Mol Neurosci. (2019) 67:504–10. doi: 10.1007/s12031-019-1257-9
116. Oh, J, Lee, HJ, Song, JH, Park, SI, and Kim, H. Plasminogen activator inhibitor-1 as an early potential diagnostic marker for Alzheimer’s disease. Exp Gerontol. (2014) 60:87–91. doi: 10.1016/j.exger.2014.10.004
117. Laughlin, GA, McEvoy, LK, Barrett-Connor, E, Daniels, LB, and Ix, JH. Fetuin-a, a new vascular biomarker of cognitive decline in older adults. Clin Endocrinol. (2014) 81:134–40. doi: 10.1111/cen.12382
118. Smith, ER, Nilforooshan, R, Weaving, G, and Tabet, N. Plasma fetuin-a is associated with the severity of cognitive impairment in mild-to-moderate Alzheimer’s disease. J Alzheimers Dis. (2011) 24:327–33. doi: 10.3233/JAD-2011-101872
119. Stefan, N, and Häring, HU. Circulating fetuin-a and free fatty. Acids interact to predict insulin resistance in humans. Nat Med. (2013) 19:394–5. doi: 10.1038/nm.3116
120. Pal, D, Dasgupta, S, Kundu, R, Maitra, S, Das, G, Mukhopadhyay, S, et al. Fetuin-a acts as an endogenous ligand of TLR4 to promote lipid-induced insulin resistance. Nat Med. (2012) 18:1279–85. doi: 10.1038/nm.2851
Keywords: regional adiposity, fat distribution, visceral, liver, cognition, brain, dementia, Alzheimer’s disease
Citation: Boccara E, Golan S and Beeri MS (2023) The association between regional adiposity, cognitive function, and dementia-related brain changes: a systematic review. Front. Med. 10:1160426. doi: 10.3389/fmed.2023.1160426
Edited by:
Takao Yamasaki, Minkodo Minohara Hospital, JapanReviewed by:
Stavros I. Dimitriadis, University of Barcelona, SpainGiuseppe Paolisso, University of Campania Luigi Vanvitelli, Italy
Norbert Stefan, University of Tübingen, Germany
Michele Bastide, Universitéde Lille, France
Copyright © 2023 Boccara, Golan and Beeri. This is an open-access article distributed under the terms of the Creative Commons Attribution License (CC BY). The use, distribution or reproduction in other forums is permitted, provided the original author(s) and the copyright owner(s) are credited and that the original publication in this journal is cited, in accordance with accepted academic practice. No use, distribution or reproduction is permitted which does not comply with these terms.
*Correspondence: Ethel Boccara, Ethel.boccara@sheba.health.gov.il
†Present address: Michal Schnaider Beeri, The Herbert and Jacqueline Krieger Klein Alzheimer’s Research Center, Brain Health Institute, Rutgers University, New Brunswick–Piscataway, NJ, United States