- 1Department of Cardiology, The Second People's Hospital of Hefei, Hefei Hospital Affiliated to Anhui Medical University, Hefei, Anhui, China
- 2Graduate School, Bengbu Medical College, Bengbu, Anhui, China
- 3Department of Cardiology, The Lu’an Hospital Affiliated to Anhui Medical University, Lu’an, Anhui, China
- 4Department of Cardiology, The Lu’an People's Hospital, Lu’an, Anhui, China
Objective: This study aimed to investigate the predictive value of a clinical nomogram model based on serum YKL-40 for major adverse cardiovascular events (MACE) during hospitalization in patients with acute ST-segment elevation myocardial infarction (STEMI).
Methods: In this study, 295 STEMI patients from October 2020 to March 2023 in the Second People’s Hospital of Hefei were randomly divided into a training group (n = 206) and a validation group (n = 89). Machine learning random forest model was used to select important variables and multivariate logistic regression was included to analyze the influencing factors of in-hospital MACE in STEMI patients; a nomogram model was constructed and the discrimination, calibration, and clinical effectiveness of the model were verified.
Results: According to the results of random forest and multivariate analysis, we identified serum YKL-40, albumin, blood glucose, hemoglobin, LVEF, and uric acid as independent predictors of in-hospital MACE in STEMI patients. Using the above parameters to establish a nomogram, the model C-index was 0.843 (95% CI: 0.79–0.897) in the training group; the model C-index was 0.863 (95% CI: 0.789–0.936) in the validation group, with good predictive power; the AUC (0.843) in the training group was greater than the TIMI risk score (0.648), p < 0.05; and the AUC (0.863) in the validation group was greater than the TIMI risk score (0.795). The calibration curve showed good predictive values and observed values of the nomogram; the DCA results showed that the graph had a high clinical application value.
Conclusion: In conclusion, we constructed and validated a nomogram based on serum YKL-40 to predict the risk of in-hospital MACE in STEMI patients. This model can provide a scientific reference for predicting the occurrence of in-hospital MACE and improving the prognosis of STEMI patients.
1. Introduction
Acute ST-segment elevation myocardial infarction (STEMI) is an acute severe heart disease caused by the rupture of vulnerable atherosclerotic plaques in coronary vessels. At present, the key to the treatment of STEMI lies in dredging the blocked vessels and restoring the blood supply to the myocardium. Existing treatments are mainly percutaneous coronary intervention (PCI), however, myocardial reperfusion injury often occurs in STEMI patients after PCI with the recovery of myocardial blood supply, leading to the occurrence of major cardiovascular adverse events (MACEs) such as heart failure, malignant arrhythmia, and even sudden death, with a poor prognosis (1, 2). In addition to thrombosis and platelet activation, several studies have shown that the inflammatory response plays an important role in the development and progression of STEMI (3). Therefore, how to predict the occurrence of MACE after PCI in STEMI patients early is of great clinical significance for improving the prognosis.
Serum YKL-40 is a glycoprotein belonging to the chitinase-similar protein family, also known as human glycochondroprotein 39 (HC-gp39) and chitinase 3-like protein 1 (4). YKL-40 can be secreted by a variety of cells, including monocytes, macrophages, neutrophils, chondrocytes, and vascular smooth muscle cells (5–7). To date, serum YKL-40 has been implicated as a novel inflammatory marker contributing to cell proliferation and differentiation, angiogenesis, and tissue remodeling (8). It has been shown that serum YKL-40 is closely associated with the early and late stages of the atherosclerotic process, and YKL-40 induces monocytes to mature into macrophages, which are then secreted by macrophages and activated macrophages at a later stage of differentiation (9).In addition, previous studies have shown that YKL-40 levels are higher in patients with myocardial infarction (10), stable coronary artery disease (11, 12), and heart failure (13). High levels of serum YKL-40 are an independent predictor of MACE after PCI in STEMI patients, and serum YKL-40 can be used as a biomarker to predict the long-term prognosis of STEMI patients after PCI (14, 15). Serum YKL-40 levels predict postoperative myocardial reperfusion and in-hospital MACE in STEMI patients (16).
Currently, the most effective treatment for STEMI patients remains percutaneous coronary intervention. However, the incidence of major adverse cardiac events (MACEs) during hospitalization after PCI varies according to different risk factors for STEMI, particularly in high-risk patients (17, 18). Predictive models will allow physicians to better identify high-risk patients, which will facilitate a more personalized approach to managing these cases.
As a simple and accurate visualization tool, nomograms have been widely used to predict the incidence of each patient endpoint event. Developed a simple and practical nomogram to predict the prognosis of ACS patients with potential clinical value (19). However, few studies predict serum YKL-40 with nomograms and in-hospital MACE in STEMI patients. Therefore, our study aimed to develop and validate a nomogram, combined with clinical characteristics to predict the likelihood of in-hospital MACE in STEMI patients with serum YKL-40, to provide a scientific reference for clinical treatment decisions and the prevention of in-hospital MACE in STEMI patients.
2. Methods
2.1. Study population
We enrolled 295 patients with STEMI who underwent PCI between October 2020 and March 2023 in the Second People’s Hospital of Hefei. Inclusion criteria were STEMI diagnosis (17): (1) chest pain symptoms within 24 h before admission, lasting >30 min; (2) ST-segment elevation and/or abnormal Q waves in ≥2 consecutive leads suggested by electrocardiogram; (3) serum biochemical markers creatine kinase isoenzyme (CK-MB) and/or cardiac troponin T (cTnT) increased within 24 h after the onset of chest pain. Exclusion criteria: (1) malignant tumor; (2) blood system diseases; (3) active infection; (4) chronic inflammatory diseases; (5) severe liver or kidney dysfunction; (6) chronic heart failure; (7) use of steroids or chemotherapeutic drugs. This study was approved by the Ethics Committee of the Second People’s Hospital of Hefei, and all patients signed an informed consent form.
2.2. Detection of serum YKL-40
Five milliliters of cubital venous blood were taken from all STEMI patients before emergency PCI in a common biochemical tube, centrifuged at a centrifugal radius of 15 cm, 2,500 r/min, for 10 min at 4°C, and the separated serum was placed in a sterile EP tube and stored in a −80°C freezer until testing. Specimens were tested in the same batch and thawed thoroughly and uniformly at room temperature for the first time. Serum YKL-40 levels were measured by enzyme-linked immunosorbent assay (ELISA), and a standard curve was drawn to determine the YKL-40 concentration of unknown samples, with intra-batch and inter-batch coefficients of variation of <6 and 8%, respectively. The operation process shall be carried out in strict accordance with the requirements in the instructions for use.
2.3. General data and laboratory tests
General data collection: The general conditions and vital signs of the patients at admission were recorded by consulting the medical records through the electronic case system of the hospital, including age, gender, BMI, diabetes, hypertension, smoking, family history of coronary heart disease, heart rate, systolic blood pressure, diastolic blood pressure, Killip class and Gensini score; the laboratory parameters of fasting tests on the second day after admission included neutrophils, hemoglobin, platelets, total cholesterol, triglycerides, LDL-C, HDL-C, fasting blood glucose, serum creatinine, blood uric acid, and albumin in all patients.
2.4. Definition and grouping of MACE events
MACE defined the primary endpoint event as all-cause mortality. Secondary endpoints were reinfarction, emergency revascularization, cardiac arrest, acute heart failure, cardiogenic shock, malignant arrhythmia (including ventricular tachycardia/ventricular fibrillation, sinus arrest, high-grade or third-degree atrioventricular block), mechanical complications of myocardial infarction, stroke, and severe bleeding (hemoglobin decrease ≥3 g/L). Among them, 113 STEMI patients developed MACE and 182 STEMI patients did not develop MACE events. STEMI patients were randomly divided into a training group (n = 206) and a validation group (n = 89) according to 7:3.
2.5. Random forest model variables selection
Random forest (RF) is a learning method consisting of many individual decision trees that operate as a collection. In the classification task, each tree in RF produces a class prediction, and the class (mode) that votes the most becomes the overall model prediction (18). Each decision tree node uses a subset of features randomly selected from the entire feature list. Increasing the number of trees in the forest to find the number of trees without more significant performance gain. The number of random features to consider in each tree is called MTRYS. Lower Mtry values lead to more differences, fewer associated trees, and yield better stability. In addition, low MTRY also tends to make better use of variables that have moderate effects on response variables. However, lower MTRY values also lead to the worse mean performance of trees as they are constructed based on suboptimal variables. Finally, the minimum size of a terminal node (the number of patients classified at that node) is called the node size. Setting this number more often leads to smaller tree growths. The hyperparameters of all models (MTRYS, minimum number of trees, total number of trees) were adjusted using a grid search method.
2.6. Development and validation of a nomogram
A nomogram was developed based on variables selected by the random forest model and multivariate logistic regression analysis. Receiver operating characteristic (ROC) area under the curve (AUC) and model C-index were used to assess the performance of the model; a calibration curve was drawn to evaluate the calibration of the model; a decision curve was drawn to analyze the net benefit rate of this nomogram model in predicting in-hospital MACE in STEMI patients. ROC curves were plotted to assess the efficacy of nomogram models and TIMI scores in predicting in-hospital MACE in STEMI patients.
2.7. Statistical methods
Statistics and graphs were performed using SPSS 26.0 and GraphPad Prism9.0. Kolmogorov–Smirnov normality test was performed for measurement data, which conformed to the normal distribution and was expressed as mean ± standard deviation and independent sample t-test was used for comparison between the two groups; measurement data without normal distribution were expressed as median M (P25, P75), and Mann–Whitney U test was used for comparison between the two groups; enumeration data adoption rate was expressed, and chi-square test was used for comparison between the two groups; RF model variable selection and nomogram development and validation were statistically analyzed based on R 4.2.2. All statistics were performed using two-sided tests, and p < 0.05 was considered statistically significant.
3. Results
3.1. Comparison of clinical data between the training set and validation set
A total of 295 STEMI patients treated with PCI were included in this study. They were randomly divided into the training group (n = 206) and the validation group (n = 89), 115 males (55.8%) were in the training group, with a mean age of 63.22 ± 13.39; 62 males (69.7%) in the validation group, with a mean age of 62.27 ± 15.01. There was no statistical difference between the training group and the validation group except for gender (p < 0.05), as shown in Table 1.
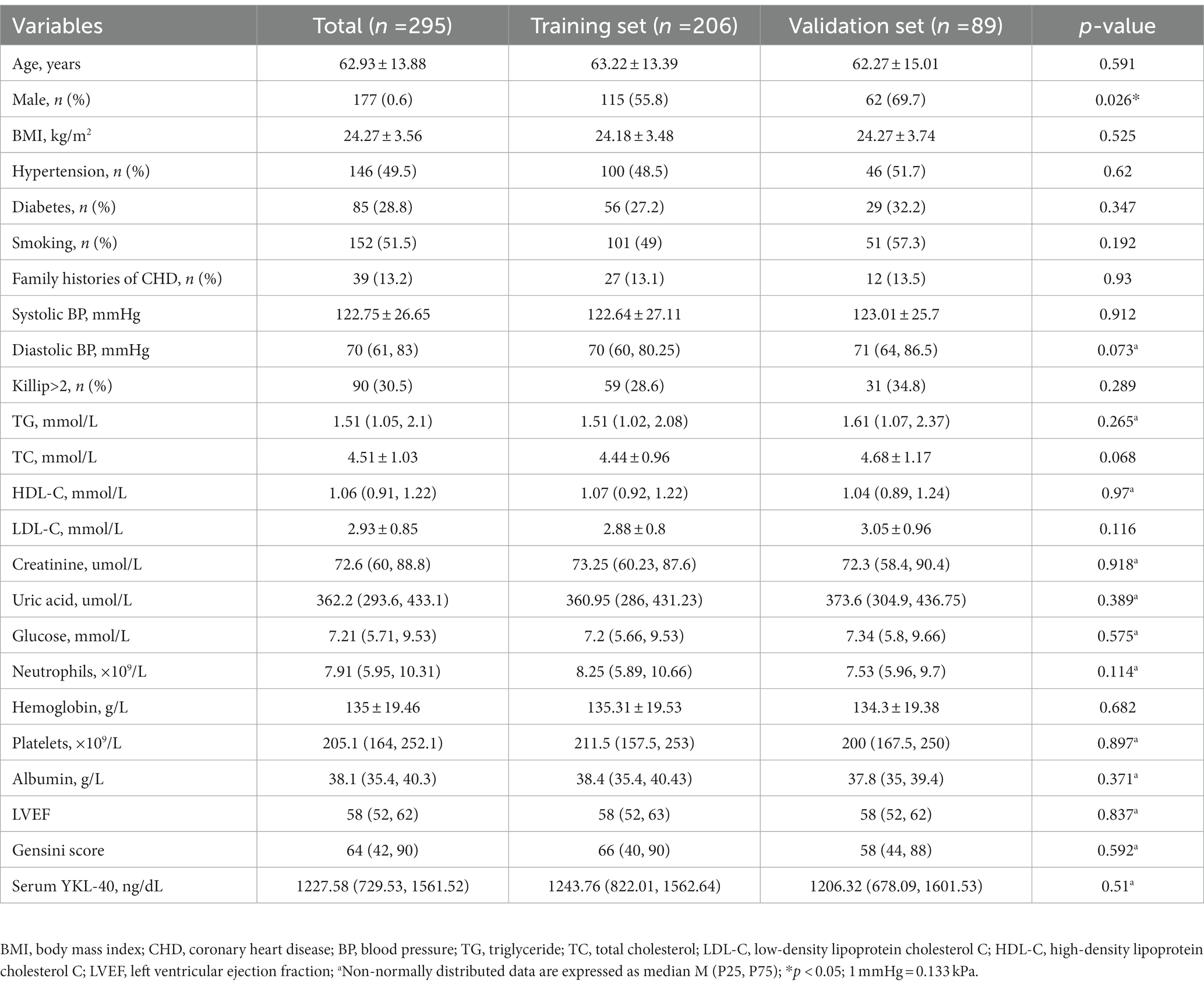
Table 1. Comparison of general clinical data between Training set and Validation Set [Mean ± standard deviation, M (P25,P75), number of cases and percentage (n%)].
3.2. Comparison of STEMI patients with and without MACE events
Compared with the non-MACE group, the serum YKL-40 level was higher in the MACE group (p < 0.05; Table 1); there was a significant difference in age between the two groups; there was a significant difference in systolic blood pressure, diastolic blood pressure, TC, HDL-C, creatinine, uric acid, neutrophils, blood glucose, hemoglobin, and LVEF (p < 0.05); there was no significant difference in gender, BMI, hypertension, diabetes, current smoking, Killip class of family history of coronary heart disease, TG, LDL-C, platelets, albumin, and Gensini score related indicators between the two groups (p > 0.05), as shown in Table 2.
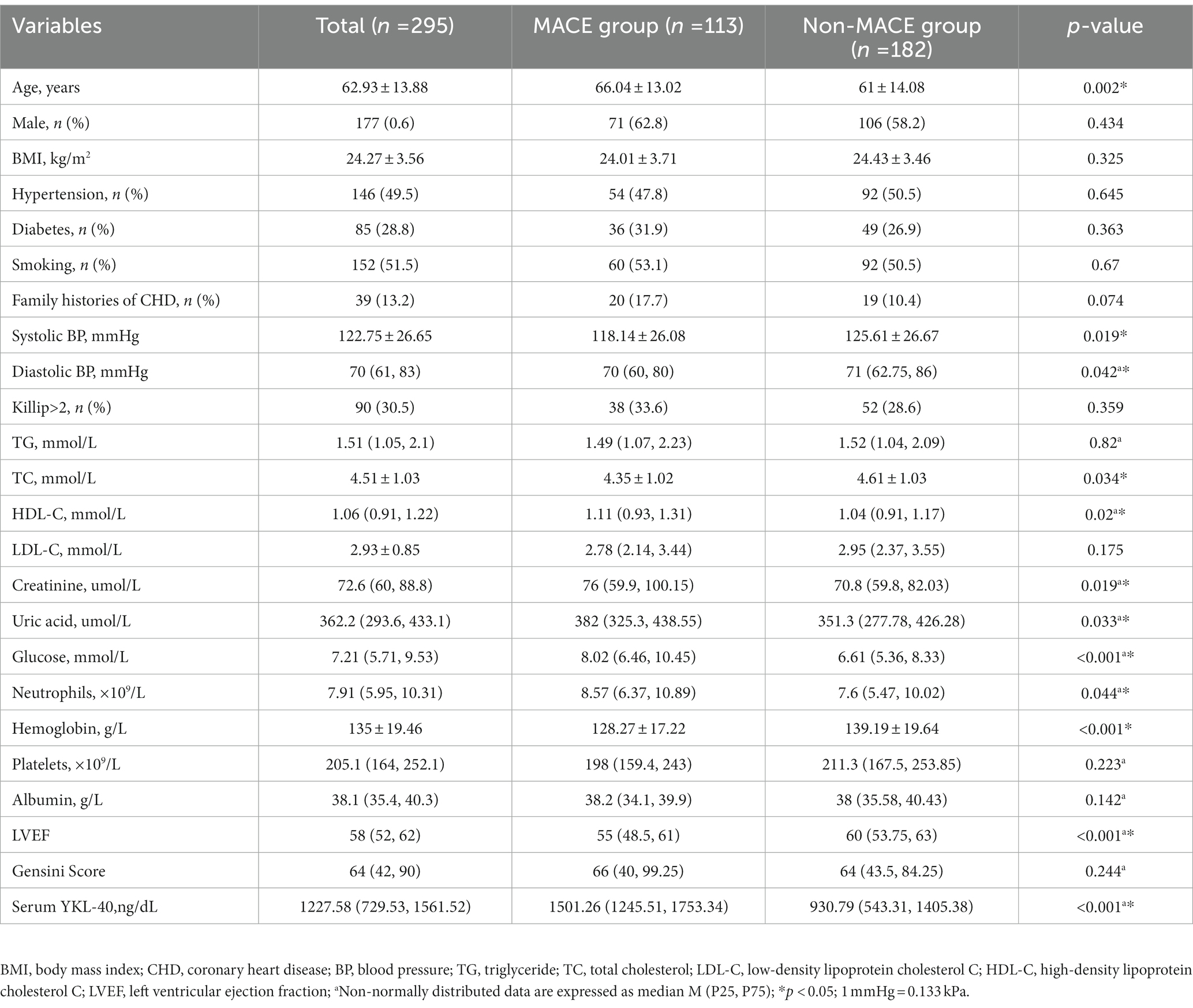
Table 2. Comparison of general clinical data between MACE group and non-MACE group [mean ± standard deviation, M (P25, P75), number of cases and percentage (n%)].
3.3. Development and validation of nomograms based on serum YKL-40 for predicting in-hospital MACE in STEMI patients
3.3.1. Selection of RF model variables and multivariate logistic regression analysis
All variables were included in the RF model to find the optimal RF hyperparameter mtrys of 2, the minimum number of trees of 20, and the total number of trees of 500; the importance ranking of variables was selected using a cross-grid search method (Figure 1); finally the top ten cross-overlapping variables of “MeanDeceAccurscy” and “DecreaseGini” in the importance ranking of RF variables were included in the multivariate logistic regression analysis; the results showed that serum YKL-40, albumin, blood glucose, hemoglobin, LVEF, and uric acid were independent predictors of in-hospital MACE in STEMI patients (Figure 2).
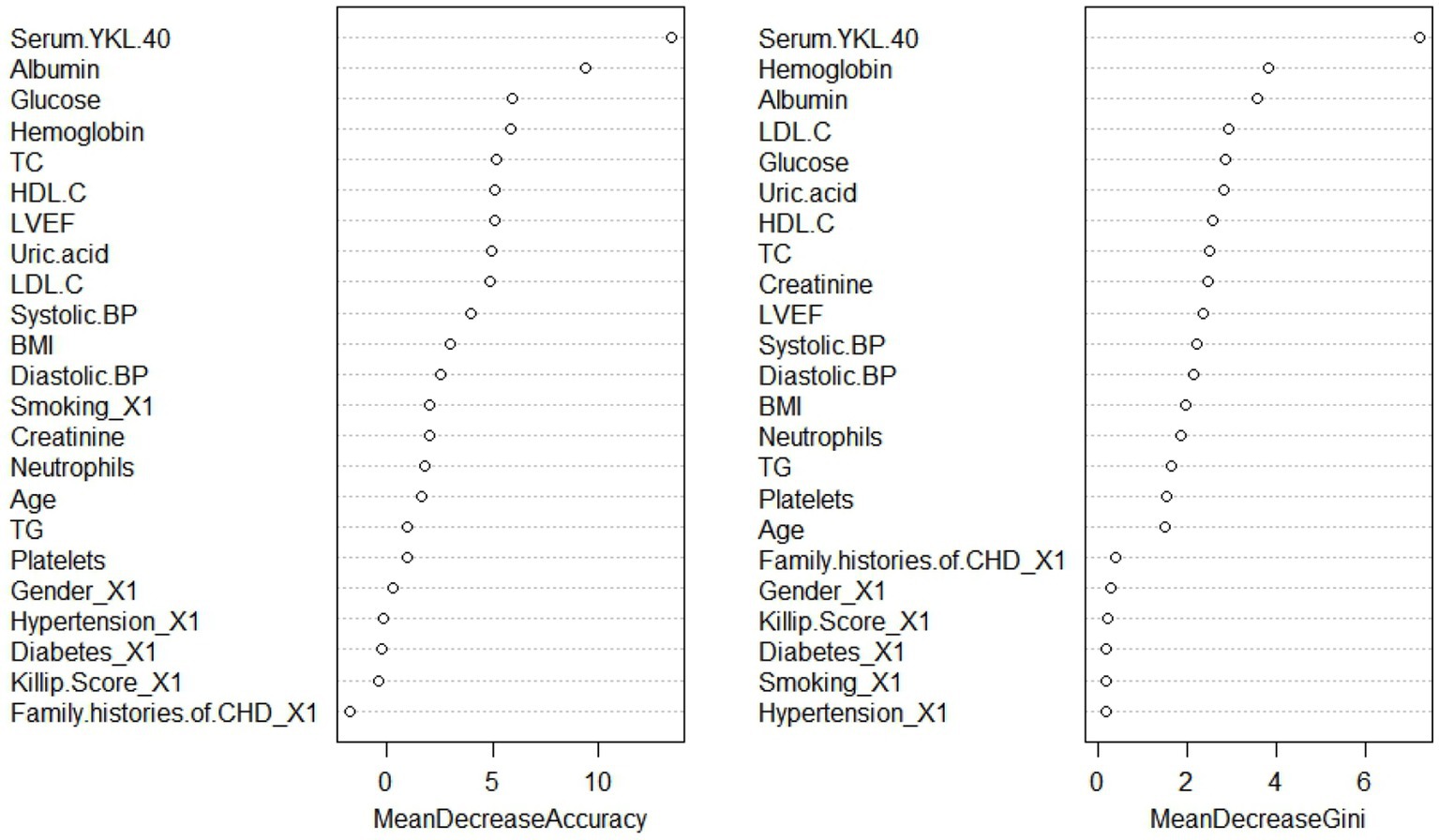
Figure 1. Random forest model variable importance ranking. BMI, body mass index; CHD, coronary heart disease; BP, blood pressure; TG, triglyceride; TC, total cholesterol; LDL-C, low-density lipoprotein cholesterol C; HDL-C, high-density lipoprotein cholesterol C; LVEF, left ventricular ejection fraction.
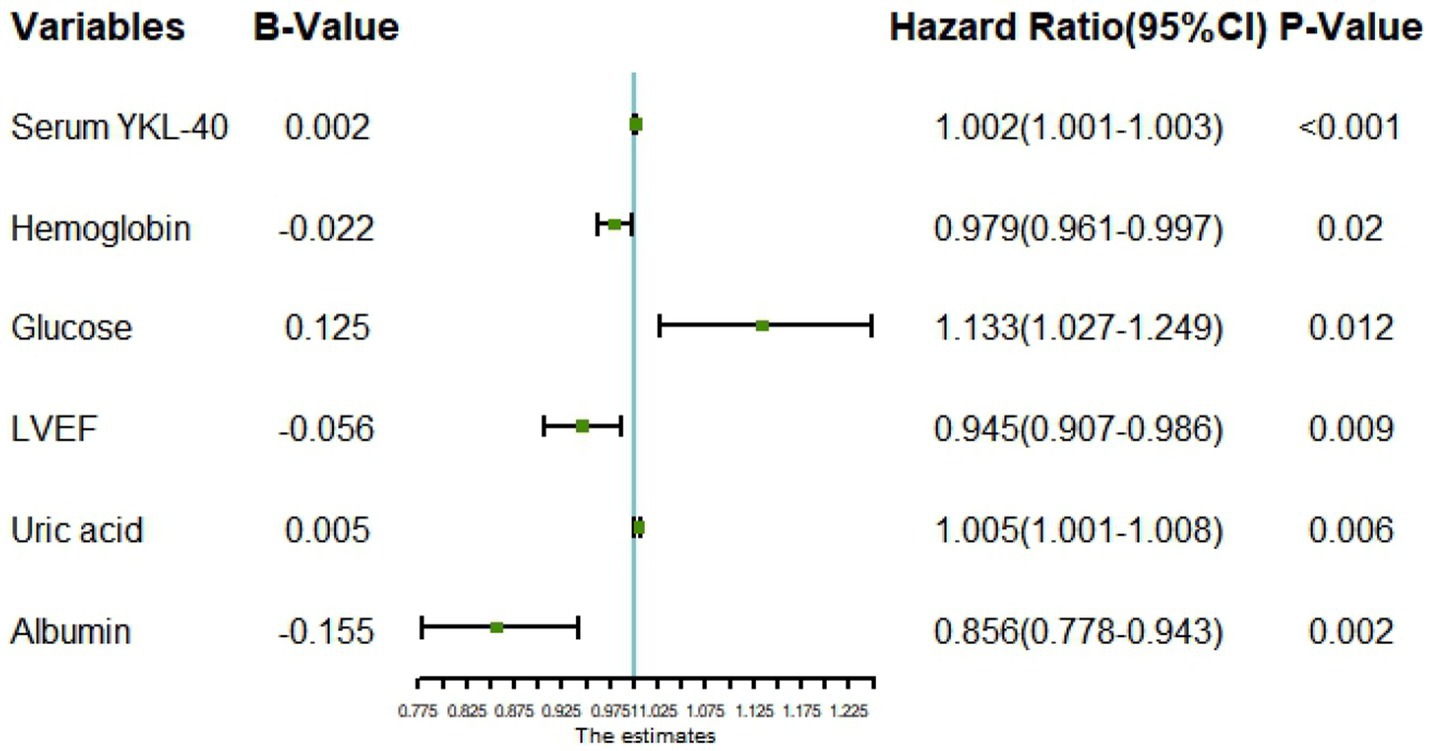
Figure 2. Forest plot for multivariate logistic regression analysis. LVEF, left ventricular ejection fraction.
3.3.2. Development of nomogram based on serum YKL-40
Predictive model nomograms for in-hospital MACE in STEMI patients were plotted with multivariate logistic regression analysis using the “RMS” package in R 4.2.2 with whether STEMI patients developed in-hospital MACE (assigned values: no = 0, yes = 1) as the dependent variable, as shown in Figure 3. Each predictor variable corresponds to a specific score on the horizontal axis of the nomogram score, and the score corresponding to each predictor variable is summed to obtain a total score. Through the total score corresponding to the prediction value of the risk of adverse cardiovascular events at the bottom of the nomogram, it can be seen from the figure that the patients with higher total scores are more likely to have in-hospital MACE.
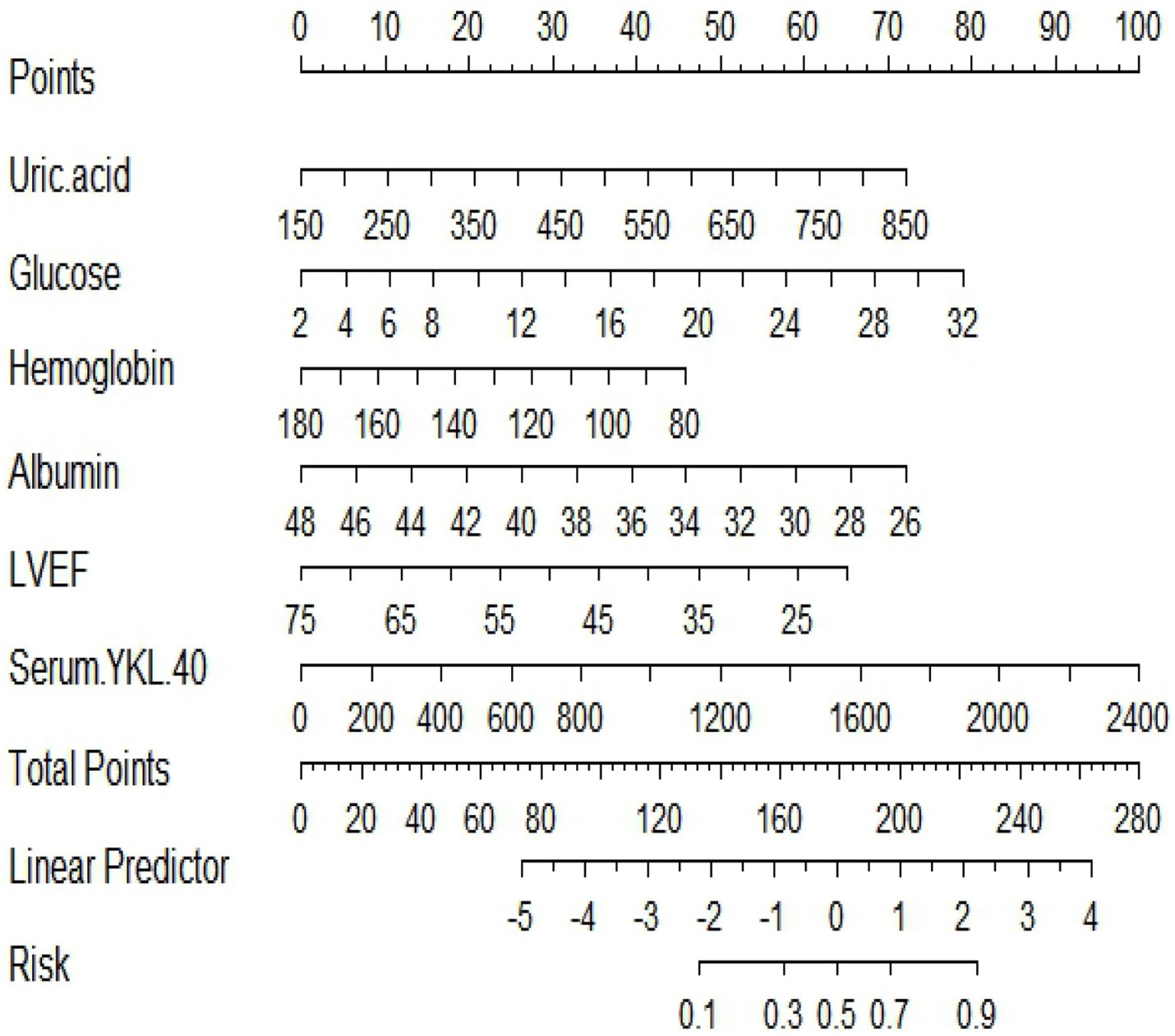
Figure 3. A nomogram based on serum YKL-40 to predict in-hospital major adverse cardiovascular events (MACEs) in patients with ST-segment elevation myocardial infarction (STEMI). LVEF, left ventricular ejection fraction.
3.3.3. Evaluation of nomogram model based on serum YKL-40
The C-index of the model in the training set was 0.843 (95% CI, 0.79–0.897), which had good predictive power; the AUC of the nomogram model in predicting in-hospital MACE in STEMI patients was 0.843, indicating that the model had good discrimination, as shown in Figure 4A; the calibration curve showed that the model was not fitted, as shown in Figure 5A; and the clinical decision curve analysis (DCA) showed that the clinical net benefit of the nomogram model was superior to the TIMI risk score between 0.1–99% threshold probability, as shown in Figure 6A.
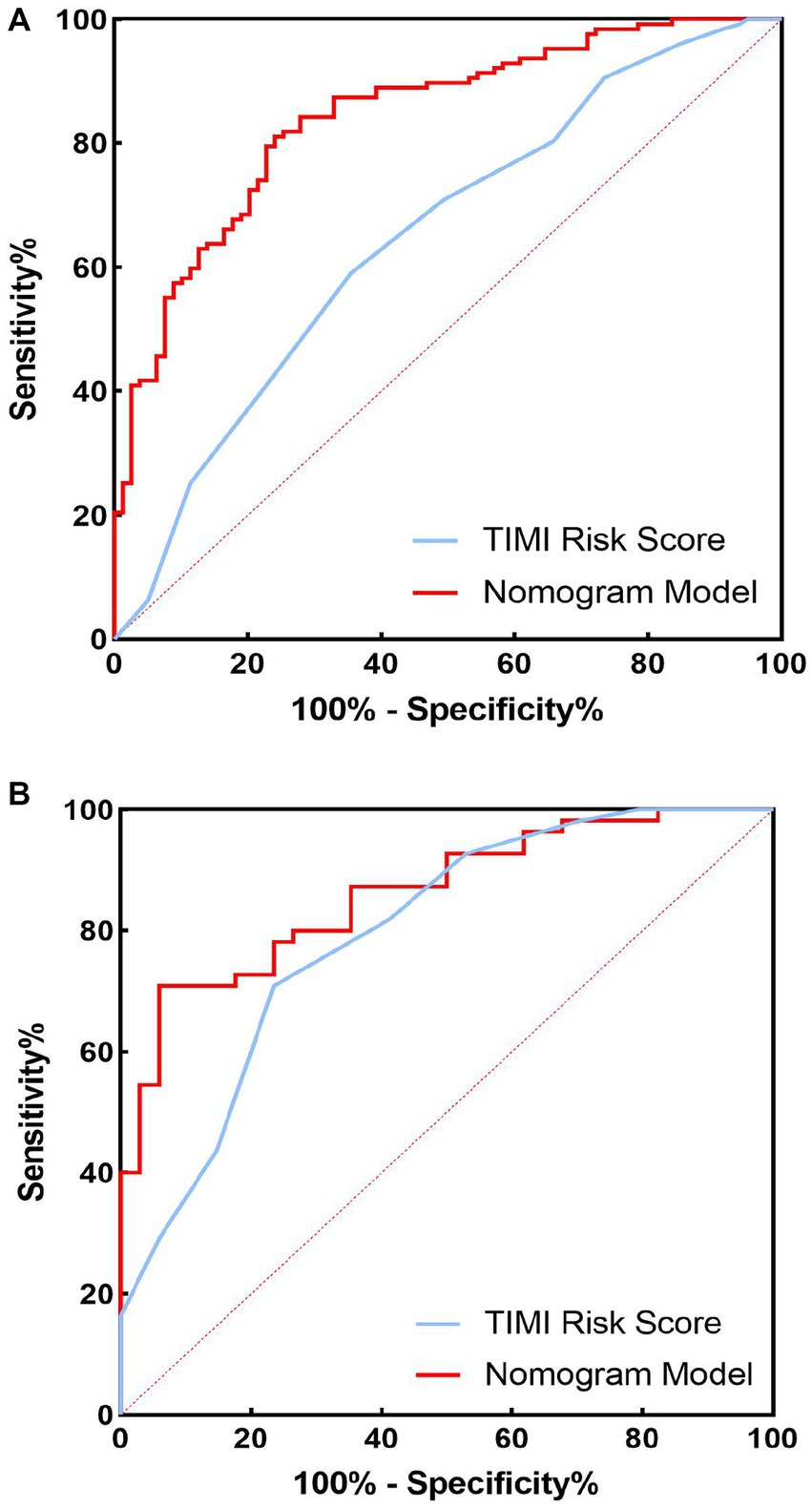
Figure 4. Receiver operating characteristic (ROC) curves of training set and validation set nomogram models and TIMI scores for predicting in-hospital major adverse cardiovascular events (MACEs) in patients with ST-segment elevation myocardial infarction (STEMI). (A) Training set; (B) validation set.
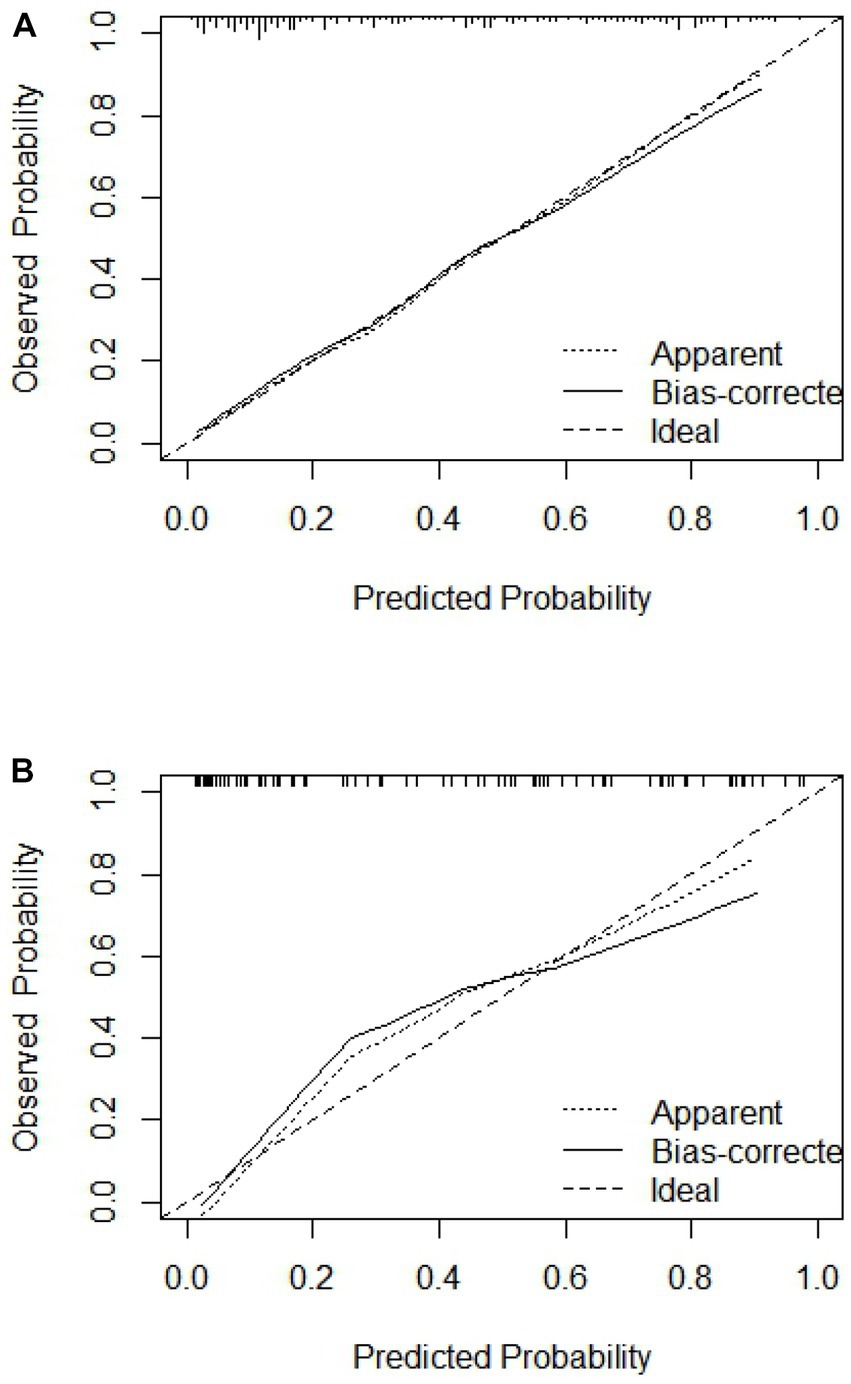
Figure 5. Calibration curve of training set and validation set. (A) Training set; (B) validation set.
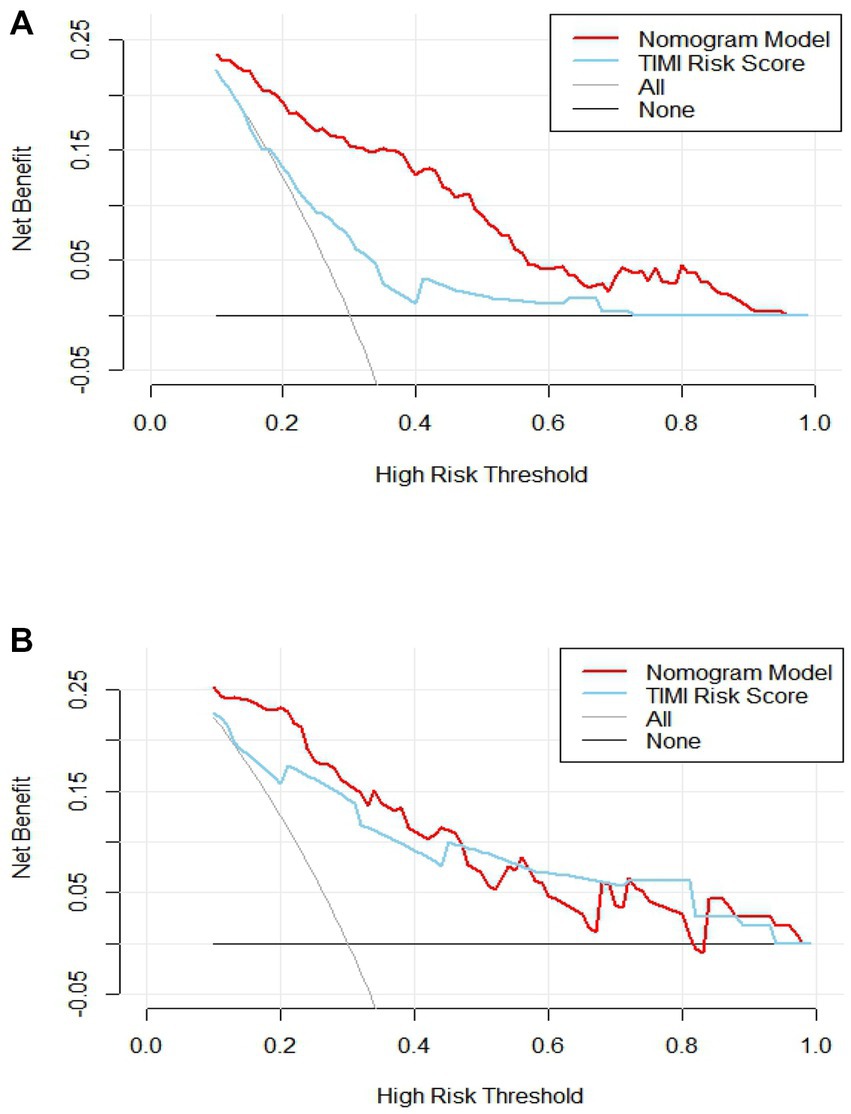
Figure 6. Analysis of clinical decision curve between training set and validation set. (A) Training set; (B) validation set.
3.3.4. Validation of nomogram model based on serum YKL-40
In the training validation set, the model C-index was 0.863 (95% CI: 0.789–0.936), with good predictive power; the AUC of the nomogram model in predicting in-hospital MACE in STEMI patients was 0.795, with good discrimination, as shown in Figure 4B; the calibration curve also showed that the model had good calibration, as shown in Figure 5B; the clinical decision curve analysis (DCA) showed that the nomogram model had better clinical net benefit than TIMI risk score between 0.1 and 45% threshold probability, as shown in Figure 6B.
3.3.5. Comparison of nomogram models with TIMI risk scores
In the training and validation sets, we compared nomogram models with TIMI risk scores. The AUC of the nomogram model in the training set to predict in-hospital MACE in STEMI patients was 0.843 (0.843) greater than the TIMI score (0.648), p < 0.05, as shown in Figure 4A. The AUC of the validated central nomogram model for predicting in-hospital MACE in STEMI patients (0.843) was greater than that of the TIMI score (0.648), p < 0.05, as shown in Figure 4B; the results showed that the nomogram had the strongest discriminant ability.
4. Discussion
Cardiovascular disease (CVD) has become the focus of global public health problems and the greatest threat to human health (20). In recent years, the incidence and mortality of CVD have been increasing year by year, and are also the most important cause of death among urban and rural residents in China (21). The most important disease in CVD is acute myocardial infarction (AMI), which has high mortality and morbidity (22). AMI refers to myocardial cell death or necrosis due to acute, severe, and persistent ischemia and hypoxia after coronary artery occlusion. It is the most severe subtype of coronary heart disease (23). According to the NHANES database from 2013 to 2016, the overall prevalence of AMI in adults aged >20 years in the US is 3% (24). In addition, AMI has a poor prognosis with a 5-year mortality rate as high as 51%, placing a heavy burden on health and socio-economic burden (25). AMI has a wide spectrum of clinical manifestations, including STEMI and NSTEMI, with high morbidity and mortality. STEMI is a typical symptom, mainly caused by acute intracoronary thrombosis and stagnation of coronary flow, and is the most severe type of AMI with a poor prognosis (26), rapid progression, and high in-hospital mortality (27, 28). Therefore, applying the correct treatment is another key factor in improving the prognosis of STEMI and requires early treatment. Effective early reperfusion therapy to save dying myocardium is a critical component of STEMI treatment and is effective in improving survival and prognosis (29). Current treatments for STEMI patients include thrombolytic therapy, PCI, and coronary artery bypass grafting (CABG). According to European and American clinical guidelines, primary PCI has become the preferred reperfusion therapy strategy for STEMI patients (30). However, even if these patients receive timely PCI and/or appropriate antiplatelet therapy, the prognosis remains unsatisfactory, and a large number of STEMI patients still have a high incidence of MACE during hospitalization after PCI, including cardiac death, myocardial reinfarction, malignant arrhythmia, and acute heart failure (31). Therefore, a new biomarker that may predict the risk of in-hospital MACE after PC in STEMI patients is urgently needed, and further improving the management of STEMI patients during hospitalization is essential to achieve better clinical outcomes in STEMI patients.
Serum YKL-40 is a glycoprotein belonging to the chitinase-similar protein family, also known as human glycochondroprotein 39 (HC-gp39) and chitinase 3-like protein 1 (4). YKL-40 can be secreted by a variety of cells, and current studies have confirmed that it is mainly secreted by macrophages in the late stage of differentiation and activated macrophages (7). The acute phase protein YKL-40 is a novel potential biomarker of inflammation in patients with coronary heart disease, and macrophages in atherosclerotic plaques express YKL-40, with the highest expression seen in macrophages with early atherosclerotic lesions. Studies have shown that serum YKL-40 is elevated in patients with acute myocardial infarction and chronic coronary heart disease (11, 32). The results of previous studies showed (33) serum YKL-40 had prognostic significance in AMI patients, plasma YKL-40 was significantly increased in AMI patients and remained higher than that in healthy subjects after 1 month, and was associated with elevated serum BNP, diastolic dysfunction, and long-term increased overall mortality. Yang et al. (14) further investigated patients with acute STEMI who underwent primary percutaneous coronary intervention (PCI) and were followed for 24 months. Found that the incidence of MACE was significantly higher in the high YKL-40 group than in the low YKL-40 group during follow-up. Therefore, high serum YKL-40 levels are an independent predictor of MACE, and serum YKL-40 can be used as a biomarker to predict the long-term prognosis of STEMI patients after PCI.
To better evaluate the prognosis of patients, the random forest model was used to screen the characteristic variables in this study, and finally, the top ten cross-overlapping variables of “MeanDecreaseAccurscy” and “MeanDecreaseGini” in the importance ranking of random forest variables were included in multivariate logistic regression analysis; the results showed that serum YKL-40 (OR: 1.002, p < 0.001), albumin (OR: 0.856, p = 0.002), blood glucose (OR: 1.133, P0.012), hemoglobin (OR: 0.979, p = 0.02), LVEF (OR: 0.945, p = 0.009), and uric acid (OR: 1.005, p = 0.006) were independent predictors of in-hospital MACE in STEMI patients. On this basis, we constructed a nomogram prediction model for in-hospital MACE in STEMI patients.
The results of this study showed that serum YKL-40 levels were significantly increased in patients in the MACE group compared with those in the non-MACE group. By RF and multivariate logistic regression analysis, our study identified serum YKL-40 as an independent risk factor for MACE during hospitalization after PCI in STEMI patients, a result consistent with previous studies (16). Consistent with the existing cardiovascular disease literature (10, 14–16), we found that STEMI patients with higher serum YKL-40 levels tended to be older, male, diabetic, family history of coronary heart disease, anterior myocardial infarction, had a higher proportion of multivessel disease, and greater Gensini score. All these features suggest that elevated serum YKL-40 levels may influence the prognosis of STEMI.
Uric acid is the end product of endogenous and dietary purine metabolism and is produced by purine metabolism by xanthine oxidase. Studies have shown the predictive value of uric acid in the hospitalization of patients with the acute coronary syndrome (34). However, not all epidemiological studies support this hypothesis (35). In some studies, uric acid levels were no longer associated with coronary heart disease after additional adjustments for cardiovascular risk factors (36). Some authors consider hyperuricemia as a risk factor rather than an independent risk factor (37). However, other studies have shown that elevated uric acid levels independently predict adverse clinical outcomes after acute myocardial infarction, including increased coronary lesion severity, and mortality (38). High uric acid levels at admission are an independent prognostic factor for adverse outcomes during hospitalization for PCI in patients with acute myocardial infarction (39). Similarly, our study suggests that serum uric acid is associated with the development of in-hospital MACE after PCI in STEMI patients and is an independent risk factor.
Our study found that hemoglobin was an independent predictor of in-hospital MACE after PCI in STEMI patients by multivariate logistic regression analysis. Anemia is more common in patients hospitalized for cardiovascular disease than in the general population, occurring in 11 to 38% of patients with acute myocardial infarction (40). Hemoglobin (Hb) levels on admission are considered a major determinant of mortality and adverse ischemic and hemorrhagic events in patients with cardiovascular disease, particularly ACS (41–43). Anemia at admission has been reported to affect 25% of patients with acute coronary syndromes and has a negative impact on prognosis (44). In a study by Tsujita et al. involving 3,153 STEMI patients (45), anemia was an independent predictor of reinfarction, hemorrhage, and 1-year mortality.
Epidemiological studies have shown that hyperglycemia is an independent risk factor for poor prognosis in patients with acute myocardial infarction regardless of a history of diabetes. Admission stress hyperglycemia has been shown to be associated with increased in-hospital mortality and late follow-up MACE in non-diabetic patients with myocardial infarction (46). Hyperglycemia at admission is strongly associated with mortality, infarct size, impaired left ventricular function, and poor clinical outcome in acute myocardial infarction, and blood glucose at admission is linearly associated with AMI mortality (47). Elevated fasting blood glucose is common in patients with acute myocardial infarction and is significantly associated with increased short-term mortality. In a prospective study, fasting plasma glucose between 6.1 and 6.9 mmol/L was significantly associated with an increased risk of acute myocardial infarction (48). Logistic multivariate regression analysis in this study showed that fasting glucose was an independent risk factor for MACE after PCI in STEMI patients, consistent with previous studies.
Patients with acute myocardial infarction complicated by heart failure (HF) or left ventricular dysfunction have a poor prognosis and are at high risk of rehospitalization and death (49). The use of LVEF measured by echocardiography after acute myocardial infarction to evaluate left ventricular function is an important indicator for predicting clinical prognosis and can well distinguish between low and high risk of cardiac events after acute myocardial infarction. In a study of 417 patients with AMI, LVEF <40% was an independent predictor of the combined end point of death, congestive heart failure, and recurrent AMI 30 years after AMI (50). Another study (51), involving 28,771 patients with HF, left ventricular dysfunction, or both after acute myocardial infarction, showed that the risk of death increased with decreasing LVEF for all types of death. Logistic multivariate regression analysis in this study showed that LVEF was an independent risk factor for MACE after PCI in STEMI patients, consistent with previous studies.
A prospective cohort study (n = 734) divided patients with stable coronary artery disease (CAD) into low serum albumin (baseline albumin concentration <3.5 g/dL, n = 98) and normal albumin (baseline albumin concentration ≥3.5 g/dL, n = 636) groups (52). Low serum albumin concentrations (<3.5 g/dL) have a poor prognosis, with an increased risk of all-cause mortality and cardiovascular events. Another study investigated the effect of low serum albumin levels (n = 35) in 82 patients with the acute coronary syndrome (ACS) (in-hospital mortality 10%, 8 patients) and showed that adverse in-hospital outcomes (death, acute heart failure, cardiogenic shock, and re-infarction 43%) were more common in patients presenting with hypoalbuminemia (53). Additional cohort studies have shown that low serum albumin levels on admission are an independent predictor of long-term all-cause, cardiovascular, and cardiac mortality (54). Similarly, a retrospective study of 1,424 patients with acute myocardial infarction showed a higher incidence of in-hospital MACE in patients with low serum albumin levels (55). Our study found that serum albumin was an independent predictor of in-hospital MACE after PCI in STEMI patients by multivariate logistic regression analysis.
A nomogram is a visual graph composed of line segments of different lengths that are used to predict the probability of a clinical event, is based on a multivariate regression model, and is drawn after integrating multiple clinical indicators. By RF and multivariate logistic regression analysis, we developed a nomogram containing serum YKL-40, hemoglobin, fasting blood glucose, LVEF, uric acid, and serum albumin variables to predict the risk of in-hospital MACE in STEMI patients. For example, serum YKL-40 was 1706.89 ng/dL, hemoglobin 126.7 g/L, fasting blood glucose 3.39 mmol/L, LVEF 52, uric acid 373.2 umol/L, and serum albumin 33.4 g/L in STEMI patients after admission. The nomogram model calculated a total score of 291 and a 70.2% probability of having MACE during hospitalization (Figure 7).
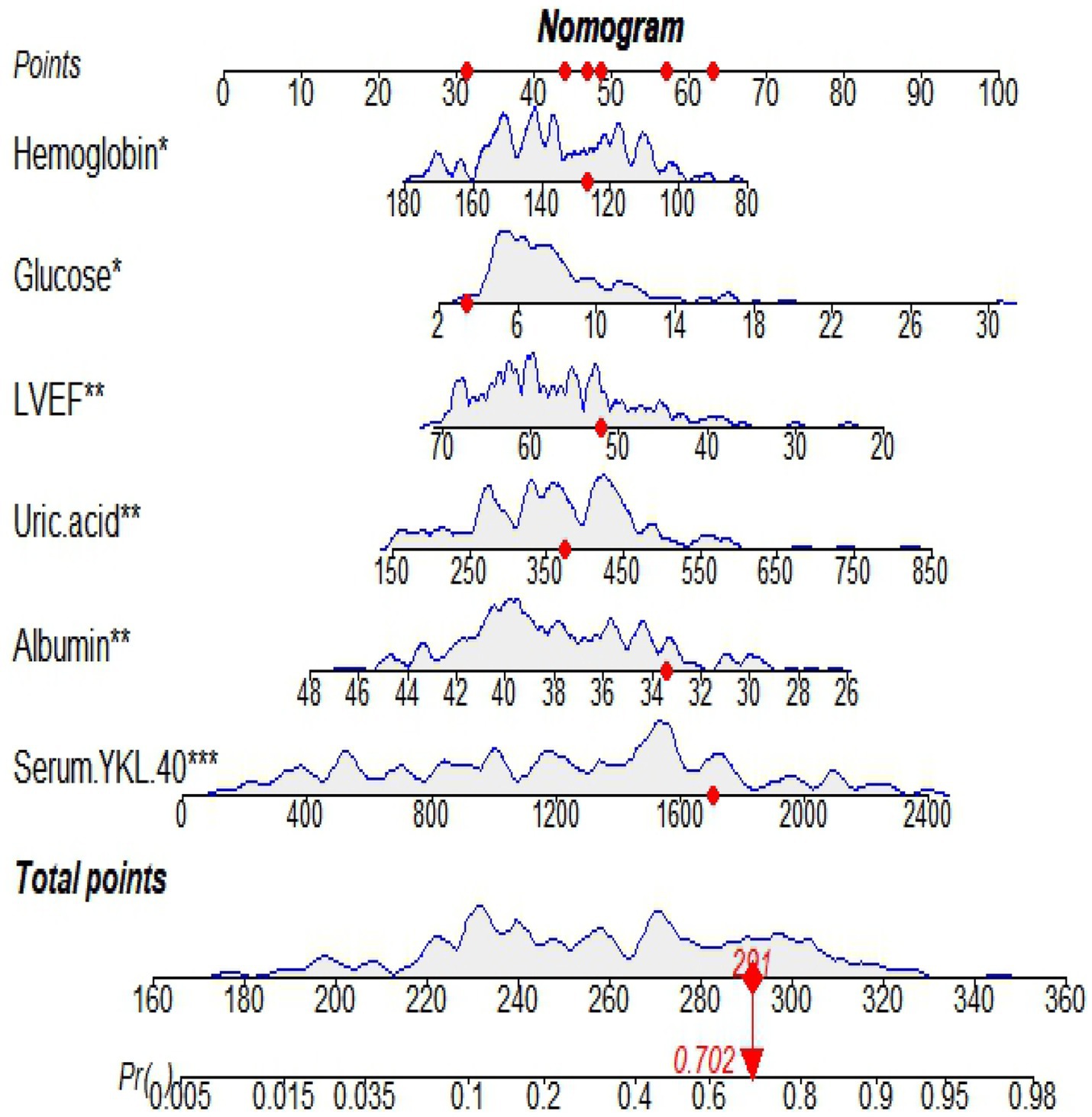
Figure 7. An example of in-hospital MACE nomogram in patients with acute ST-segment elevation myocardial infarction (STEMI).
To further evaluate the predictive value of nomograms for the development of MACE during hospitalization in STEMI patients. We used a risk assessment scoring system to assess whether STEMI patients developed MACE during hospitalization, mainly including the TIMI risk score (56), GRACE score (57), and PAMI risk score (58), of which TIMI risk score is widely used in clinical practice (59, 60). The TIMI score was based on data from the TIMI-Π study, which included patients with STEMI who underwent thrombolytic therapy and mainly analyzed clinical characteristics affecting the risk of death. However, other MACEs also predict adverse outcomes and are more common in clinical practice. Therefore, the AUC of the nomogram model in the training set for predicting in-hospital MACE in STEMI patients was 0.843 (0.843) greater than the TIMI risk score (0.648). Validation of the centralized nomogram model predicted a greater AUC (0.843) than the TIMI risk score (0.648) for in-hospital MACE in STEMI patients; the results indicated that the nomogram had the strongest discriminant power. In parallel, we performed DCA to evaluate the performance of the model. It showed that the net clinical benefit of the nomogram model was better than TIMI risk in both training and validation sets.
This study has some shortcomings. First, this study was a single-center small sample size study without external validation of the model; at the same time, the possibility of selection bias cannot be ruled out. Second, because only studies of MACE during hospitalization after PCI in STEMI patients have been performed, long-term follow-up is needed to assess the long-term prognostic value of serum YKL-40 in STEMI patients in the future.
5. Conclusion
In conclusion, this study suggests that a nomogram model based on serum YKL-40 for predicting the risk of in-hospital MACE in STEMI patients has good discrimination, calibration, and clinical validity and can be used as an effective tool for early clinical prediction of the risk of in-hospital MACE after PCI in STEMI patients.
Data availability statement
The original contributions presented in the study are included in the article/supplementary material, further inquiries can be directed to the corresponding author/s.
Ethics statement
Written informed consent was obtained from the individual(s) for the publication of any potentially identifiable images or data included in this article. The study was approved by the Ethics Committee of the Second People ‘s Hospital of Hefei (Approval No: 2020-ke-058). Informed consent was obtained from all patients.
Author contributions
CF and ZC wrote the main manuscript text. JL, WW, and YW prepared Figures 1–7 and Table 1. JZ put forward constructive comments on the overall conception of the article. We also thank for assistance with data collection. All authors contributed to the article and approved the submitted version.
Funding
This research is funded by the Natural Science Project of Bengbu Medical College in 2020 (2020byzd322).
Acknowledgments
This manuscript has been previously published as a preprint (61).
Conflict of interest
The authors declare that the research was conducted in the absence of any commercial or financial relationships that could be construed as a potential conflict of interest.
Publisher’s note
All claims expressed in this article are solely those of the authors and do not necessarily represent those of their affiliated organizations, or those of the publisher, the editors and the reviewers. Any product that may be evaluated in this article, or claim that may be made by its manufacturer, is not guaranteed or endorsed by the publisher.
References
1. Zhang, Y, Zhang, L, Zheng, H, and Chen, H. Effects of atrial fibrillation on complications and prognosis of patients receiving emergency PCI after acute myocardial infarction. Exp Ther Med. (2018) 16:3574–8. doi: 10.3892/etm.2018.6640
2. Romanov, A, Martinek, M, Pürerfellner, H, Chen, S, De Melis, M, Grazhdankin, I, et al. Incidence of atrial fibrillation detected by continuous rhythm monitoring after acute myocardial infarction in patients with preserved left ventricular ejection fraction: results of the ARREST study. Europace. (2018) 20:263–70. doi: 10.1093/europace/euw344
3. Wang, Q, Ma, J, Jiang, Z, Wu, F, Ping, J, and Ming, L. Association of lymphocyte-to-monocyte ratio with in-hospital and long-term major adverse cardiac and cerebrovascular events in patients with ST-elevated myocardial infarction. Medicine. (2017) 96:e7897. doi: 10.1097/MD.0000000000007897
4. Hakala, BE, White, C, and Recklies, AD. Human cartilage gp-39, a major secretory product of articular chondrocytes and synovial cells, is a mammalian member of a chitinase protein family. J Biol Chem. (1993) 268:25803–10. doi: 10.1016/S0021-9258(19)74461-5
5. Johansen, JS. Studies on serum YKL-40 as a biomarker in diseases with inflammation, tissue remodelling, fibroses and cancer. Dan Med Bull. (2006) 53:172–209. doi: 10.1053/joca.2000.0377
6. Johansen, JS, Olee, T, Price, PA, Hashimoto, S, Ochs, RL, and Lotz, M. Regulation of YKL-40 production by human articular chondrocytes. Arthritis Rheum. (2001) 44:826–37. doi: 10.1002/1529-0131(200104)44:4<826::AID-ANR139>3.0.CO;2-U
7. Johansen, JS, Jensen, BV, Roslind, A, Nielsen, D, and Price, PA. Serum YKL-40, a new prognostic biomarker in cancer patients? Cancer Epidemiol Biomarkers Prev. (2006) 15:194–202. doi: 10.1158/1055-9965.EPI-05-0011
8. Kjaergaard, AD, Johansen, JS, Bojesen, SE, and Nordestgaard, BG. Role of inflammatory marker YKL-40 in the diagnosis, prognosis and cause of cardiovascular and liver diseases. Crit Rev Clin Lab Sci. (2016) 53:396–408. doi: 10.1080/10408363.2016.1190683
9. Rehli, M, Niller, HH, Ammon, C, Langmann, S, Schwarzfischer, L, Andreesen, R, et al. Transcriptional regulation of CHI3L1, a marker gene for late stages of macrophage differentiation. J Biol Chem. (2003) 278:44058–67. doi: 10.1074/jbc.M306792200
10. Fang, C, Chen, Z, Zhang, J, Pan, J, Jin, X, Yang, M, et al. The value of serum YKL-40 and TNF-α in the diagnosis of acute ST-segment elevation myocardial infarction. Cardiol Res Pract. (2022) 2022:1–7. doi: 10.1155/2022/4905954
11. Jin, Y, Cao, JN, Wang, CX, Feng, QT, Ye, XH, Xu, X, et al. High serum YKL-40 level positively correlates with coronary artery disease. Biomark Med. (2017) 11:133–9. doi: 10.2217/bmm-2016-0240
12. Schroder, J, Jakobsen, JC, Winkel, P, Hilden, J, Jensen, GB, Sajadieh, A, et al. Prognosis and reclassification by YKL-40 in stable coronary artery disease. J Am Heart Assoc. (2020) 9:e014634. doi: 10.1161/JAHA.119.014634
13. Chirinos, JA, Orlenko, A, Zhao, L, Basso, MD, Cvijic, ME, Li, Z, et al. Multiple plasma biomarkers for risk stratification in patients with heart failure and preserved ejection fraction. J Am Coll Cardiol. (2020) 75:1281–95. doi: 10.1016/j.jacc.2019.12.069
14. Yang, L, Dong, H, Lu, H, Liao, Y, Zhang, H, Xu, L, et al. Serum YKL-40 predicts long-term outcome in patients undergoing primary percutaneous coronary intervention for ST-segment elevation myocardial infarction. Medicine. (2019) 98:e14920. doi: 10.1097/MD.0000000000014920
15. Hjort, M, Eggers, KM, Lindhagen, L, Baron, T, Erlinge, D, Jernberg, T, et al. Differences in biomarker concentrations and predictions of long-term outcome in patients with ST-elevation and non-ST-elevation myocardial infarction. Clin Biochem. (2021) 98:17–23. doi: 10.1016/j.clinbiochem.2021.09.001
16. Cetin, M, Kocaman, SA, Canga, A, Kırbaş, A, Yılmaz, A, Erdoğan, T, et al. Elevated serum YKL-40 level predicts myocardial reperfusion and in-hospital MACE in patients with STEMI. Herz. (2013) 38:202–9. doi: 10.1007/s00059-012-3671-4
17. Jeong, YJ, Ahn, JM, Hyun, J, Lee, J, Kim, JH, Yang, Y, et al. Ten-year outcomes after drug-eluting stents or bypass surgery for left Main coronary disease in patients with and without diabetes mellitus: the PRECOMBAT extended follow-up study. J Am Heart Assoc. (2021) 10:e019834. doi: 10.1161/JAHA.120.019834
18. Sud, M, Han, L, Koh, M, Abdel-Qadir, H, Austin, PC, Farkouh, ME, et al. Low-density lipoprotein cholesterol and adverse cardiovascular events after percutaneous coronary intervention. J Am Coll Cardiol. (2020) 76:1440–50. doi: 10.1016/j.jacc.2020.07.033
19. Bo, X, Liu, Y, Yang, M, Lu, Z, Zhao, Y, and Chen, L. Development and validation of a nomogram of in-hospital major adverse cardiovascular and cerebrovascular events in patients with acute coronary syndrome. Front Cardiovasc Med. (2021) 8:699023. doi: 10.3389/fcvm.2021.699023
20. Mensah, GA, Roth, GA, and Fuster, V. The global burden of cardiovascular diseases and risk factors: 2020 and beyond. J Am Coll Cardiol. (2019) 74:2529–32. doi: 10.1016/j.jacc.2019.10.009
21. Zhao, D, Liu, J, Wang, M, Zhang, X, and Zhou, M. Epidemiology of cardiovascular disease in China: current features and implications. Nat Rev Cardiol. (2019) 16:203–12. doi: 10.1038/s41569-018-0119-4
22. Reindl, M, Reinstadler, SJ, Feistritzer, HJ, Mayr, A, Klug, G, Marschang, P, et al. Acute myocardial infarction as a manifestation of systemic vasculitis. Wien Klin Wochenschr. (2016) 128:841–3. doi: 10.1007/s00508-016-1051-4
23. Pei, J, Wang, X, Xing, Z, Chen, P, Su, W, Deng, S, et al. Association between admission systolic blood pressure and major adverse cardiovascular events in patients with acute myocardial infarction. PLoS One. (2020) 15:e0234935. doi: 10.1371/journal.pone.0234935
24. Virani, SS, Alonso, A, Benjamin, EJ, Bittencourt, MS, Callaway, CW, Carson, AP, et al. Heart disease and stroke Statistics-2020 update: a report from the American Heart Association. Circulation. (2020) 141:e139–596. doi: 10.1161/CIR.0000000000000757
25. Kochar, A, Chen, AY, Sharma, PP, Pagidipati, NJ, Fonarow, GC, Cowper, PA, et al. Long-term mortality of older patients with acute myocardial infarction treated in US clinical practice. J Am Heart Assoc. (2018) 7:e007230. doi: 10.1161/JAHA.117.007230
26. Guo, W, Yang, D, Wu, D, Liu, H, Chen, S, Liu, J, et al. Hyperuricemia and long-term mortality in patients with acute myocardial infarction undergoing percutaneous coronary intervention. Ann Trans Med. (2019) 7:636. doi: 10.21037/atm.2019.10.110
27. Mathias, W Jr, Tsutsui, JM, Tavares, BG, Fava, AM, Aguiar, MOD, Borges, BC, et al. Sonothrombolysis in ST-segment elevation myocardial infarction treated with primary percutaneous coronary intervention. J Am Coll Cardiol. (2019) 73:2832–42. doi: 10.1016/j.jacc.2019.03.006
28. Chapman, AR, Shah, ASV, Lee, KK, Anand, A, Francis, O, Adamson, P, et al. Long-term outcomes in patients with type 2 myocardial infarction and myocardial injury. Circulation. (2018) 137:1236–45. doi: 10.1161/CIRCULATIONAHA.117.031806
29. Ottani, F, Limbruno, U, Latini, R, Misuraca, L, and Galvani, M. Reperfusion in STEMI patients: still a role for cardioprotection? Minerva Cardioangiol. (2018) 66:452–63. doi: 10.23736/S0026-4725.18.04680-7
30. Ibanez, B, James, S, Agewall, S, Antunes, MJ, Bucciarelli-Ducci, C, Bueno, H, et al. 2017 ESC guidelines for the management of acute myocardial infarction in patients presenting with ST-segment elevation: the task force for the management of acute myocardial infarction in patients presenting with ST-segment elevation of the European Society of Cardiology (ESC). Eur Heart J. (2018) 39:119–77. doi: 10.1093/eurheartj/ehx393
31. Li, J, Li, X, Wang, Q, Hu, S, Wang, Y, Masoudi, FA, et al. ST-segment elevation myocardial infarction in China from 2001 to 2011 (the China PEACE-retrospective acute myocardial infarction study): a retrospective analysis of hospital data. Lancet. (2015) 385:441–51. doi: 10.1016/S0140-6736(14)60921-1
32. Tan, Y, Ji, X, Mo, Z, and Zhou, Y. Serum YKL-40 positively correlates with MMP-9 and CRP in patients with acute ST segment elevation myocardial infarction following emergency treatment. Medicine. (2019) 98:e17950. doi: 10.1097/MD.0000000000017950
33. Pala, S, Sari, M, Kahveci, G, Alizade, E, Arslantas, U, and Uslu, A. Plasma YKL-40 elevation on admission and follow-up is associated with diastolic dysfunction and mortality in patients with acute myocardial infarction. Cardiol Res Pract. (2018) 2018:1–7. doi: 10.1155/2018/8701851
34. Rebora, P, Centola, M, Morici, N, Sacco, A, Occhino, G, Viola, G, et al. Uric acid associated with acute heart failure presentation in acute coronary syndrome patients. Eur J Intern Med. (2022) 99:30–7. doi: 10.1016/j.ejim.2022.01.018
35. Ndrepepa, G. Uric acid and cardiovascular disease. Clin Chim Acta. (2018) 484:150–63. doi: 10.1016/j.cca.2018.05.046
36. Antman, EM, Hand, M, Armstrong, PW, Bates, ER, Green, LA, Halasyamani, LK, et al. 2007 focused update of the ACC/AHA 2004 guidelines for the management of patients with ST-elevation myocardial infarction: a report of the American College of Cardiology/American Heart Association task force on practice guidelines. J Am Coll Cardiol. (2008) 51:210–47. doi: 10.1016/j.jacc.2007.10.001
37. Nakahashi, T, Tada, H, Sakata, K, Yoshida, T, Tanaka, Y, Nomura, A, et al. The association between serum uric acid and mortality in patients with acute coronary syndrome after percutaneous coronary intervention. Int Heart J. (2022) 63:447–53. doi: 10.1536/ihj.21-764
38. Zhang, S, Liu, X, Song, B, Yu, H, Zhang, X, and Shao, Y. Impact of serum uric acid levels on the clinical prognosis and severity of coronary artery disease in patients with acute coronary syndrome and hypertension after percutaneous coronary intervention: a prospective cohort study. BMJ Open. (2022) 12:e052031. doi: 10.1136/bmjopen-2021-052031
39. Bhalerao, AV, Tahir, SM, and Agarwal, R. Risk stratification and in-hospital outcome in patients with acute coronary syndrome. J Fam Med Prim Care. (2022) 11:2780–8. doi: 10.4103/jfmpc.jfmpc_1805_21
40. Colombo, MG, Kirchberger, I, Amann, U, Heier, M, Thilo, C, Kuch, B, et al. Association between admission anemia and long-term mortality in patients with acute myocardial infarction: results from the MONICA/KORA myocardial infarction registry. BMC Cardiovasc Disord. (2018) 18:50. doi: 10.1186/s12872-018-0785-5
41. Ohana-Sarna-Cahan, L, and Atar, S. Clinical outcomes of patients with acute coronary syndrome and moderate or severe chronic anaemia undergoing coronary angiography or intervention. Eur Heart J Acute Cardiovasc Care. (2018) 7:646–51. doi: 10.1177/2048872617707959
42. Brener, SJ, Mehran, R, Dangas, GD, Ohman, EM, Witzenbichler, B, Zhang, Y, et al. Relation of baseline hemoglobin levels and adverse events in patients with acute coronary syndromes (from the acute catheterization and urgent intervention triage strategY and harmonizing outcomes with RevasculariZatiON and stents in acute myocardial infarction trials). Am J Cardiol. (2017) 119:1710–6. doi: 10.1016/j.amjcard.2017.02.052
43. Yazji, K, Abdul, F, Elangovan, S, Ul Haq, MZ, Ossei-Gerning, N, Morris, K, et al. Baseline anemia in patients undergoing percutaneous coronary intervention after an acute coronary syndrome-a paradox of high bleeding risk, high ischemic risk, and complex coronary disease. J Interv Cardiol. (2017) 30:491–9. doi: 10.1111/joic.12406
44. Kunadian, V, Mehran, R, Lincoff, AM, Feit, F, Manoukian, SV, Hamon, M, et al. Effect of anemia on frequency of short- and long-term clinical events in acute coronary syndromes (from the acute catheterization and urgent intervention triage strategy trial). Am J Cardiol. (2014) 114:1823–9. doi: 10.1016/j.amjcard.2014.09.023
45. Tsujita, K, Nikolsky, E, Lansky, AJ, Dangas, G, Fahy, M, Brodie, BR, et al. Impact of anemia on clinical outcomes of patients with ST-segment elevation myocardial infarction in relation to gender and adjunctive antithrombotic therapy (from the HORIZONS-AMI trial). Am J Cardiol. (2010) 105:1385–94. doi: 10.1016/j.amjcard.2010.01.001
46. Eskandari, R, Matini, P, Emami, S, and Rezaei, Y. Association between admission blood glucose and prognosis in non-diabetic patients with first-ever acute myocardial infarction. Rom J Intern Med. (2022) 60:34–41. doi: 10.2478/rjim-2021-0033
47. Bjarnason, TA, Hafthorsson, SO, Kristinsdottir, LB, Oskarsdottir, ES, Johnsen, A, and Andersen, K. The prognostic effect of known and newly detected type 2 diabetes in patients with acute coronary syndrome. Eur Heart J Acute Cardiovasc Care. (2020) 9:608–15. doi: 10.1177/2048872619849925
48. Park, C, Guallar, E, Linton, JA, Lee, DC, Jang, Y, Son, DK, et al. Fasting glucose level and the risk of incident atherosclerotic cardiovascular diseases. Diabetes Care. (2013) 36:1988–93. doi: 10.2337/dc12-1577
49. Metra, M, Cotter, G, El-Khorazaty, J, Davison, BA, Milo, O, Carubelli, V, et al. Acute heart failure in the elderly: differences in clinical characteristics, outcomes, and prognostic factors in the VERITAS study. J Card Fail. (2015) 21:179–88. doi: 10.1016/j.cardfail.2014.12.012
50. Schwammenthal, E, Adler, Y, Amichai, K, Sagie, A, Behar, S, Hod, H, et al. Prognostic value of global myocardial performance indices in acute myocardial infarction: comparison to measures of systolic and diastolic left ventricular function. Chest. (2003) 124:1645–51. doi: 10.1378/chest.124.5.1645
51. Hall, TS, von Lueder, TG, Zannad, F, Rossignol, P, Duarte, K, Chouihed, T, et al. Relationship between left ventricular ejection fraction and mortality after myocardial infarction complicated by heart failure or left ventricular dysfunction. Int J Cardiol. (2018) 272:260–6. doi: 10.1016/j.ijcard.2018.07.137
52. Chien, SC, Chen, CY, Leu, HB, Su, CH, Yin, WH, Tseng, WK, et al. Association of low serum albumin concentration and adverse cardiovascular events in stable coronary heart disease. Int J Cardiol. (2017) 241:1–5. doi: 10.1016/j.ijcard.2017.04.003
53. Hartopo, AB, Gharini, PP, and Setianto, BY. Low serum albumin levels and in-hospital adverse outcomes in acute coronary syndrome. Int Heart J. (2010) 51:221–6. doi: 10.1536/ihj.51.221
54. Xia, M, Zhang, C, Gu, J, Chen, J, Wang, LC, Lu, Y, et al. Impact of serum albumin levels on long-term all-cause, cardiovascular, and cardiac mortality in patients with first-onset acute myocardial infarction. Clin Chim Acta. (2018) 477:89–93. doi: 10.1016/j.cca.2017.12.014
55. Yoshioka, G, Tanaka, A, Nishihira, K, Natsuaki, M, Kawaguchi, A, Watanabe, N, et al. Prognostic impact of follow-up serum albumin after acute myocardial infarction. ESC Heart Failure. (2021) 8:5456–65. doi: 10.1002/ehf2.13640
56. Morrow, DA, Antman, EM, Charlesworth, A, Cairns, R, Murphy, SA, de Lemos, JA, et al. TIMI risk score for ST-elevation myocardial infarction: a convenient, bedside, clinical score for risk assessment at presentation: an intravenous nPA for treatment of infarcting myocardium early II trial substudy. Circulation. (2000) 102:2031–7. doi: 10.1161/01.CIR.102.17.2031
57. Eagle, KA, Lim, MJ, Dabbous, OH, Pieper, KS, Goldberg, RJ, Van de Werf, F, et al. A validated prediction model for all forms of acute coronary syndrome: estimating the risk of 6-month postdischarge death in an international registry. JAMA. (2004) 291:2727–33. doi: 10.1001/jama.291.22.2727
58. Addala, S, Grines, CL, Dixon, SR, Stone, GW, Boura, JA, Ochoa, AB, et al. Predicting mortality in patients with ST-elevation myocardial infarction treated with primary percutaneous coronary intervention (PAMI risk score). Am J Cardiol. (2004) 93:629–32. doi: 10.1016/j.amjcard.2003.11.036
59. Vicent, L, Velásquez-Rodríguez, J, Valero-Masa, MJ, Díez-Delhoyo, F, González-Saldívar, H, Bruña, V, et al. Predictors of high Killip class after ST segment elevation myocardial infarction in the era of primary reperfusion. Int J Cardiol. (2017) 248:46–50. doi: 10.1016/j.ijcard.2017.07.038
60. De Luca, G, Suryapranata, H, Vant Hof, AW, De Boer, MJ, Hoorntje, JC, Dambrink, JH, et al. Prognostic assessment of patients with acute myocardial infarction treated with primary angioplasty: implications for early discharge. Circulation. (2004) 109:2737–43. doi: 10.1161/01.CIR.0000131765.73959.87
Keywords: serum YKL-40, acute ST-elevation myocardial infarction, STEMI, adverse cardiovascular events, MACE, nomogram
Citation: Fang C, Li J, Wang W, Wang Y, Chen Z and Zhang J (2023) Establishment and validation of a clinical nomogram model based on serum YKL-40 to predict major adverse cardiovascular events during hospitalization in patients with acute ST-segment elevation myocardial infarction. Front. Med. 10:1158005. doi: 10.3389/fmed.2023.1158005
Edited by:
Valentina Parisi, University of Naples Federico II, ItalyReviewed by:
Teresa Magalhães, New University of Lisbon, PortugalAlexander E. Berezin, Zaporizhia State Medical University, Ukraine
Copyright © 2023 Fang, Li, Wang, Wang, Chen and Zhang. This is an open-access article distributed under the terms of the Creative Commons Attribution License (CC BY). The use, distribution or reproduction in other forums is permitted, provided the original author(s) and the copyright owner(s) are credited and that the original publication in this journal is cited, in accordance with accepted academic practice. No use, distribution or reproduction is permitted which does not comply with these terms.
*Correspondence: Zhenfei Chen, 1601994492@qq.com
†These authors have contributed equally to this work