- Department of Obstetrics and Gynecology, Shengjing Hospital of China Medical University, Shenyang, Liaoning, China
Background: Numerous studies have suggested that ferroptosis plays a significant role in the development of polycystic ovary syndrome (PCOS), but the mechanism remains unclear.
Methods: In this study, we explored the role of ferroptosis-related genes in the pathogenesis of PCOS using a comprehensive bioinformatics method. First, we downloaded several Gene Expression Omnibus (GEO) datasets and combined them into a meta-GEO dataset. Differential expression analysis was performed to screen for significant ferroptosis-related genes between the normal and PCOS samples. The least absolute shrinkage selection operator regression and support vector machine–recursive feature elimination were used to select the best signs to construct a PCOS diagnostic model. Receiver operating characteristic curve analysis and decision curve analysis were applied to test the performance of the model. Finally, a ceRNA network-related ferroptosis gene was constructed.
Results: Five genes, namely, NOX1, ACVR1B, PHF21A, FTL, and GALNT14, were identified from 10 differentially expressed ferroptosis-related genes to construct a PCOS diagnostic model. Finally, a ceRNA network including 117 lncRNAs, 67 miRNAs, and five ferroptosis-related genes was constructed.
Conclusion: Our study identified five ferroptosis-related genes that may be involved in the pathogenesis of PCOS, which may provide a novel perspective for the clinical diagnosis and treatment of PCOS.
Background
Polycystic ovary syndrome (PCOS) is a complex disease characterized by reproductive, metabolic, and psychological features. It is usually diagnosed during the patients’ reproductive years and mainly presents with hirsutism, acne, irregular menstruation, and infertility (1). There are many theoretical hypotheses regarding the etiology of PCOS, and increasing evidence suggests that PCOS may be a complex polygenic disorder influenced by genetic factors, epigenetic variation, and the environment (2). The prevalence of PCOS ranges from 6 to 20%, depending on the populations studied and the definitions used (3, 4). To date, there is no uniform diagnostic standard or effective treatment for PCOS (5–7). Therefore, exploring the pathogenesis of PCOS can contribute to clinical diagnosis and therapies and improve reproductive outcomes. Numerous studies have shown that iron metabolism is related to endocrine diseases, including PCOS, but the underlying mechanisms remain unclear (8, 9).
Ferroptosis was first described in 2012 as a non-apoptotic, iron-dependent form of cell death, characterized by iron-dependent accumulation of lethal lipid reactive oxygen species (ROS) (10). Iron is essential for cellular biological processes, including growth, proliferation, and metabolism, among the many functions in the body. Balanced iron absorption, systemic transport, cellular uptake, and storage ensure balanced homeostasis of iron metabolism (11, 12). In recent years, ferroptosis is associated with the pathogenesis of various diseases, such as diabetes mellitus, renal failure, cardiomyopathy, neurodegeneration, ischemia–reperfusion injury, and cancer (13–18). Therefore, targeting ferroptosis has become a new research area for the design of therapies and disease prevention measures. Recent studies have shown that ferroptosis is involved in the pathogenesis of PCOS. Zhang et al. (5) reported that circRHBG inhibits ferroptosis in granulosa cell proliferation of PCOS through the circRHBG/miR-515/SLC7A11 axis. Liu et al. (19) revealed that the PCOS model in vivo and granulosa cells subjected to IR have increased ferroptosis levels and that the mechanism of cryptotanshinone in the treatment of PCOS is dependent on its inhibitory effect on cellular ferroptosis. Zhang et al. (20) indicated that ferroptosis proteins were associated with reproductive outcomes of POCS patients with infertility and constructed a FerSig risk prognostic model based on the expression of five independent prognostic ferroptosis proteins (G6PD, GPX4, PCBP1, DPP4, and PCBP2). Taken together, ferroptosis plays a key role in the development of PCOS; thus, comprehensive studies on ferroptosis genes in the pathogenesis of PCOS are urgently needed.
As of now, no studies have focused on the mechanism of ferroptosis-related genes in the pathogenesis of PCOS using comprehensive bioinformatics methods. Therefore, we identified differentially expressed ferroptosis-related genes (DEFRGs) in granulosa cells between normal and PCOS women using a public dataset downloaded from the Gene Expression Omnibus (GEO) database. Least absolute shrinkage and selection operator (LASSO) regression and support vector machine–recursive feature elimination (SVM-RFE) were used to select five hub DEFRGs to construct a PCOS diagnostic model, which was successfully verified in our clinical specimens. We then constructed a ceRNA network associated with the five hub DEFRGs. Our results may help illustrate the potential role of ferroptosis in the pathogenesis of PCOS and provide a novel perspective for the clinical diagnosis and treatment of PCOS.
Materials and methods
Data acquisition
Based on the Affymetrix Human Genome U133A Plus 2.0 Array microarray platform, GSE5850, GSE34526, and GSE102293 gene expressions in granulosa cells from patients with PCOS were downloaded from the GEO database. The GSE5850 dataset consisted of six normal and six PCOS women. The GSE34526 dataset included three healthy controls and seven patients with PCOS. The GSE102293 dataset contained six samples, of which two were from patients with PCOS and four were from normal controls. We then combined and normalized the three datasets into a meta-GEO dataset using the “sva” R package and the “gcrma” R package. A flowchart of the study is presented in Figure 1.
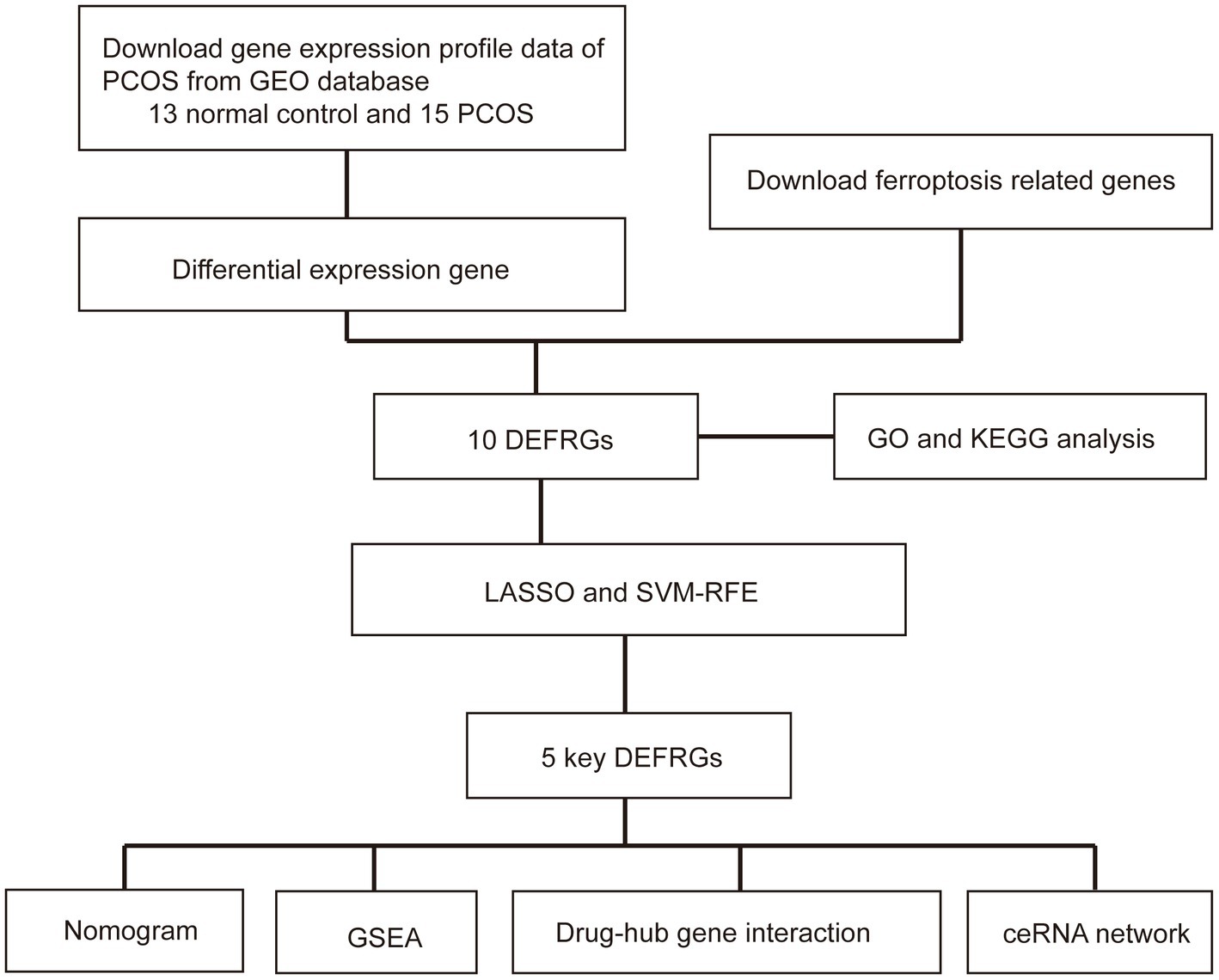
Figure 1. Flow chart of this study. PCOS: polycystic ovary syndrome; GEO: Gene Expression Omnibus; DEFRGs: differentially expressed ferroptosis-related genes; GO: Gene Ontology; KEGG; Kyoto Encyclopedia of Genes and Genomes; GSEA: gene set enrichment analysis; LASSO: least absolute shrinkage and selection operator; SVM-RFE: support vector machine–recursive feature elimination.
Differential expression analysis
We downloaded 820 ferroptosis-related genes (FRGs) from the FerrDb V2 website1. FRGs contained genes related to driver markers and suppressors. We identified differentially expressed genes (DEGs) between normal and PCOS women using the “limma” package in R. We then crossed the FRGs with DEGs to obtain DEFRGs for further investigation.
Construction and evaluation of LASSO and SVM-RFE models
The least absolute shrinkage and selection operation and SVM-RFE algorithms were used to obtain key DEFRGs to diagnose PCOS based on the “glmnet” and “e1071” R packages (21). The LASSO algorithm was used to adjust the optimal value of the penalty parameter (λ) using a 10-fold cross-validation. The SVM-RFE algorithm determines the variable by searching for the lambda with the smallest classification error. Finally, key diagnostic genes for PCOS were identified by overlapping the diagnostic data from the two algorithms. Receiver operating characteristic (ROC) curves were drawn to demonstrate the diagnostic performance of the key DEFRGs, and the area under the ROC curve (AUC) was used to verify the efficiency and accuracy of the key diagnostic DEFRGs (7).
Tissue collection
From January 2020 to December 2022, ovarian granulosa cells of 50 PCOS patients, 13 normal ovulatory women undergoing in vitro fertilization (IVF) at the ShengJing Hospital of China Medical University were collected. The diagnosis of PCOS met the Rotterdam 2003 diagnostic criteria: oligoovulation and/or anovulation, hyperandrogenism, and polycystic ovaries. Patients with congenital adrenal hyperplasia, Cushing’s syndrome, or androgen-secreting tumors were excluded. Control patients received IVF treatment for tubal disease, but had normal hormone levels, regular menstrual cycles, and normal ovarian morphology. COCs were isolated via ultrasound-guided vaginal puncture and washed in phosphate-buffered saline (PBS). Granulosa cells were selected from COCs. All the participants were under 40 years of age. This study was approved by the Ethics Committee of the ShengJing Hospital of CMU, and informed consent was obtained from all participants (No. 2020PS198K). The baseline information about all patients with PCOS is shown in Supplementary Table 1.
Quantitative RT-PCR
Total RNA was isolated using TRIzol Reagent (TaKaRa, Shiga, Japan) and then reverse-transcribed into complementary deoxyribonucleic acid (cDNA) synthesis (PrimeScript™ RT Reagent Kit). Real-time qPCR was performed to detect gene expression using 2 × SYBR Green PCR Master Mix (Thermo Fisher Scientific, Waltham, MA, USA). The 2 − ΔΔCt method was used to calculate the relative gene expression. The sequences of the primers used for RT-qPCR are presented in Supplementary Table 2.
Construction of a nomogram model
A nomogram model was conducted to facilitate the clinical application using the “rms” package. The “Points” indicate the score of each factor under different conditions, while the “Total Points” refer to the total score of all factors. Calibration curves were used to measure the predictive accuracy, and decision curve analysis (DCA) curves were used to evaluate the clinical value of the nomogram (22).
Construction of the ceRNA network
To construct a lncRNA–miRNA–mRNA regulatory network based on the five key diagnostic DEFRGs, miRNA–mRNA and miRNA–lncRNAs interactions were predicted using miRWalk2 and miRDB3, respectively. The predicted miRNAs were intersected, and the lncRNA–miRNA–mRNA network was visualized using the Cytoscape software (version 3.8.2) (23).
Functional enrichment analysis
To further explore the mechanism of DEFRGs in PCOS, Gene Ontology (GO) and Kyoto Encyclopedia of Genes and Genomes (KEGG) enrichment analysis were performed using the “clusterProfiler” package in R. Gene set enrichment analysis (GSEA) was conducted using c2.cp.kegg.symbols.gmt and c5.go.symbols.gmt (24).
Statistical analysis
All statistical analyses were performed using the R software (version 4.0.2). We mapped the chromosomal positions of the key DEFRGs using the “RCircos” package in R and calculated the correlation coefficients between the key DEFRGs using the Spearman correlation analysis. The Wilcoxon rank-sum test was used to compare differences between the groups. Statistical significance was set at a two-tailed p-value of <0.05.
Results
Identification of differentially expressed ferroptosis-related genes in PCOS
A total of 401 DEGs were identified between normal and PCOS women by the “limma” package according to the screening criteria of p < 0.05 and log |FC| > 1. We crossed 820 ferroptosis-related genes with 401 DEGs and obtained 10 differentially expressed ferroptosis-related genes (DEFRGs) (NOX1, ACVR1B, PHF21A, PRKCA, IFNA14, PTPN6, FTL, MTF1, PARP15, and GALNT14), which were visualized using a Venn diagram (Figure 2A). The circle diagram displays the chromosomal positions of the 10 DEFRGs (Figure 2B). Finally, the heat map and histogram were used to show the differential expression levels of the 10 DEFRGs between normal controls and patients with PCOS. We found that the expression levels of the 10 DEFRGs were upregulated in PCOS samples compared with those in normal controls (Figures 2C,D). The correlation heat map indicated that the 10 DEFRGs had a strong positive correlation with each other (Figure 3).
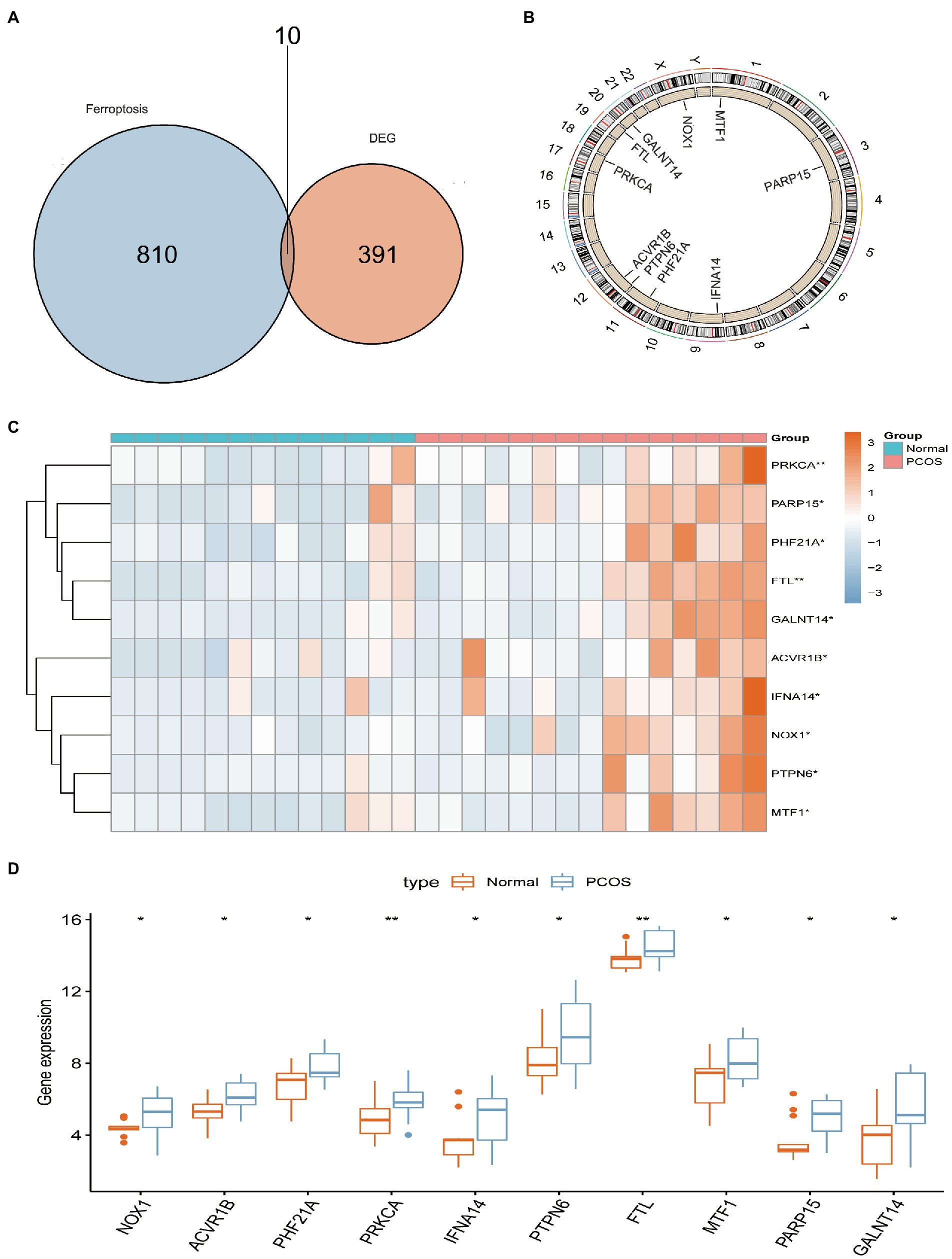
Figure 2. Landscape of 10 DEFRGs in PCOS. (A) Identification of 10 DEFRGs. (B) The chromosomal positions of the 10 DEFRGs. (C) Heat map of the expression of 10 DEFRGs between normal controls and patients with PCOS. (D) Histogram of the expression of 10 DEFRGs between normal controls and patients with PCOS.
Go and KEGG enrichment analysis
GO analysis revealed that 10 DEFRGs mainly enriched in platelet aggregation (GO:0070527), regulation of hemopoiesis (GO:1903706), myeloid cell differentiation (GO:0030099), positive regulation of myeloid cell differentiation (GO:0034109) and homotypic cell-cell adhesion (GO:0034109) (Figure 4A). KEGG analysis showed that the pathways enriched by 10 DEFRGs contained natural killer cell-mediated cytotoxicity, lipid and atherosclerosis, and the AGE-RAGE signaling pathway in diabetic complications (Figure 4B).
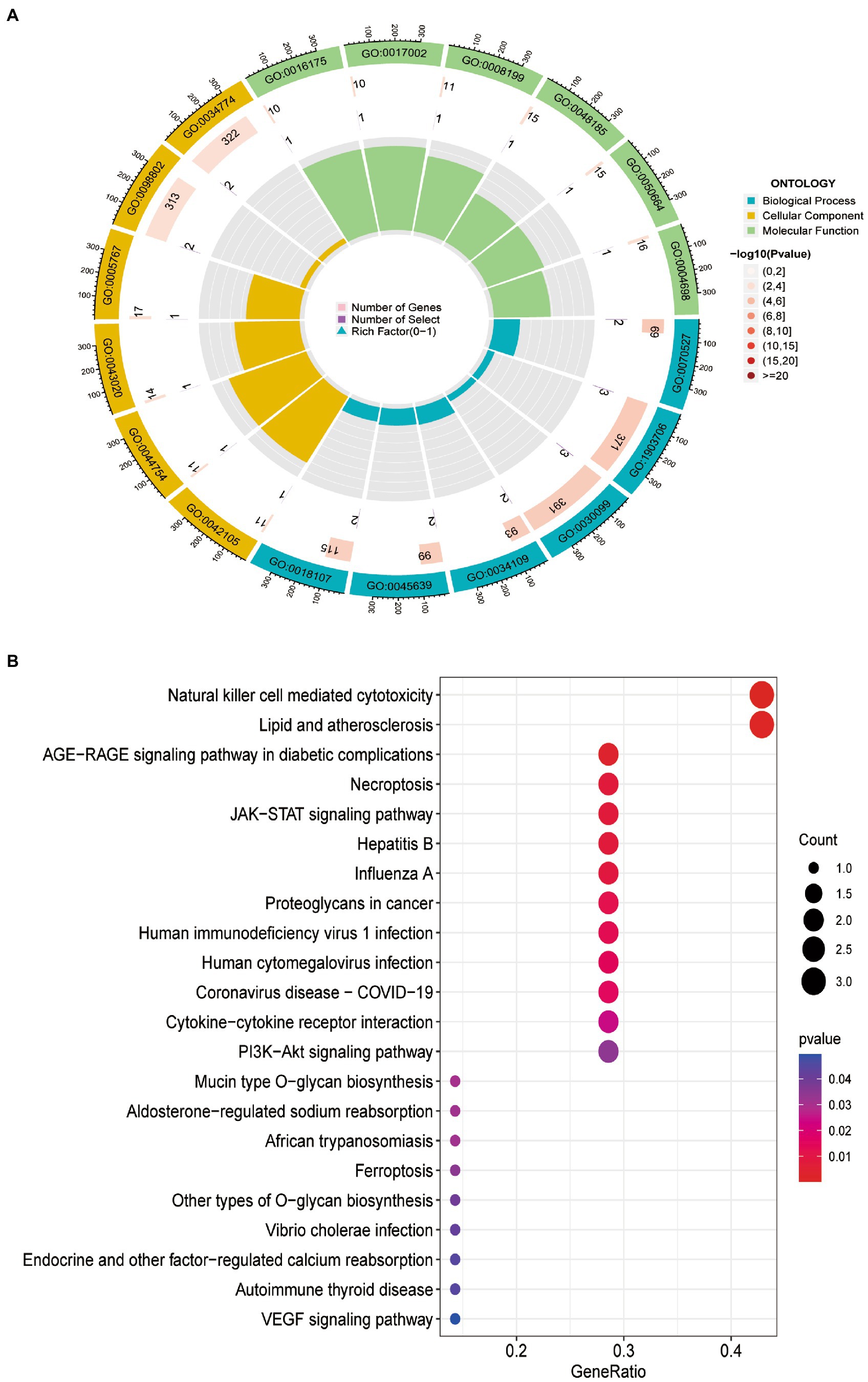
Figure 4. Functional enrichment of 10 DEFRGs. (A) GO enrichment analysis. (B). KEGG enrichment analysis.
Construction and evaluation of LASSO and SVM-RFE models
To screen for dependable diagnostic biomarkers related to PCOS, LASSO regression and the SVM-RFE algorithm were performed to evaluate 10 DEFRGs in PCOS. First, the gene expression profiles of the 10 DEFRGs were fit into LASSO regression based on the least squares method. The results revealed that five potential DEFRGs were selected, while the optimal value of lambda was obtained (Figures 5A,B). The SVM-RFE algorithm retained 10 DEFRGs as effective diagnostic biomarkers (Figures 5C,D). Five overlapping DEFRGs (NOX1, ACVR1B, PHF21A, FTL, and GALNT14) were screened as the key DEFRGs for subsequent research (Figure 5E). The AUC value of the overlapping DEFRGs obtained by the SVM-RFE and LASSO regression models was 0.785, indicating accuracy in predicting PCOS (Figure 5F).
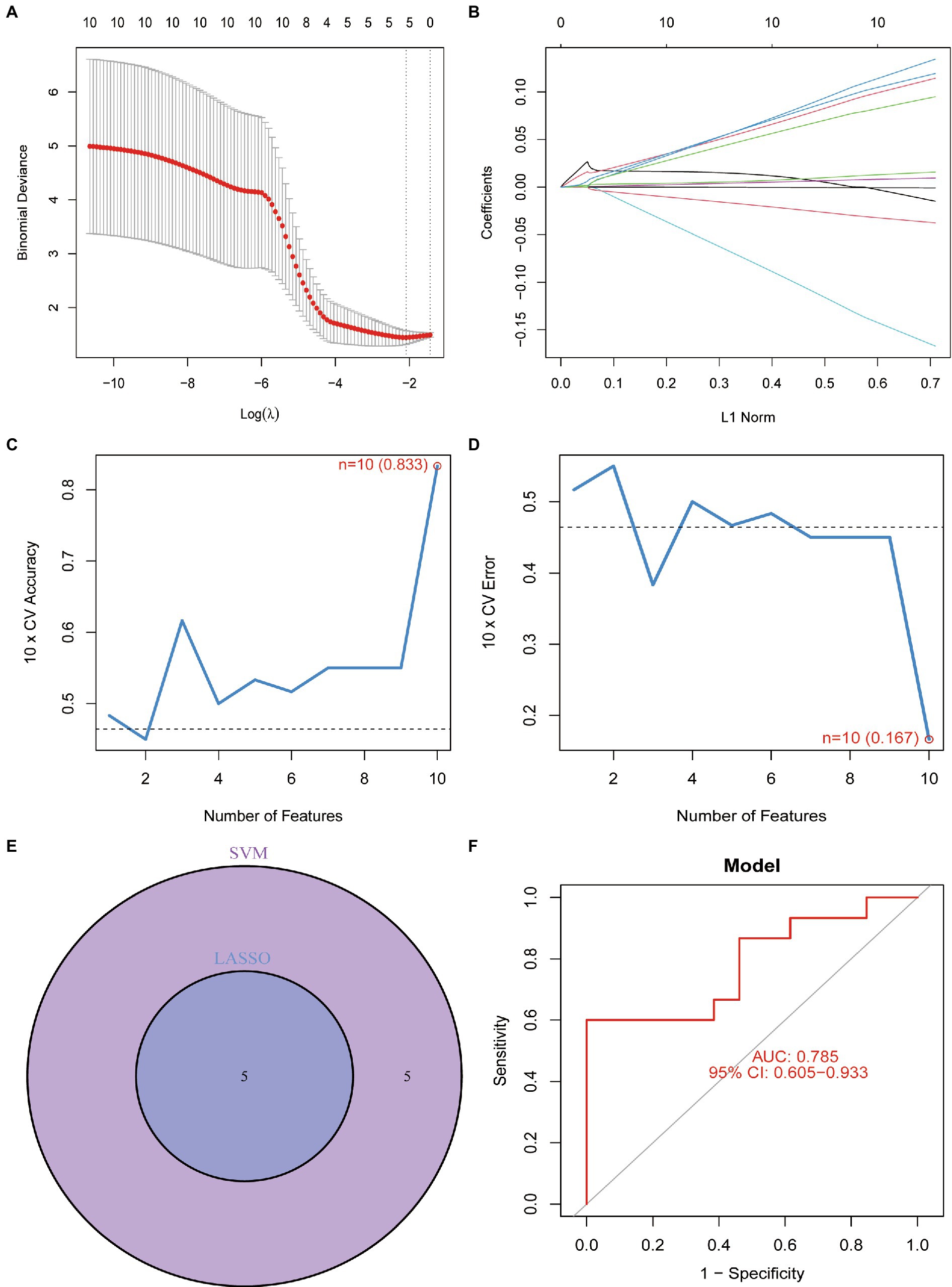
Figure 5. (A) Optimal lambda value was selected in the LASSO regression model based on 10-fold cross-validation. (B) LASSO coefficient profiles of the five co-expressional DEFRGs. (C) Line graph shows the cross-validated accuracy based on different numbers of DEFRGs in the SVM-RFE model. (D) Line graph shows the cross-validated error based on different numbers of DEFRGs in the SVM-RFE model. (E) Screening of five DEFRGs using LASSO and SVM-RFE algorithms. (F) Verification of the diagnostic value of the five DEFRGs by using ROC analysis.
Construction of a nomogram model
To facilitate the clinical diagnosis of PCOS using selected DEFRGs (NOX1, ACVR1B, PHF21A, FTL, and GALNT14), a nomogram model was constructed (Figure 6A). Calibration curves revealed that the practical diagnostic rate for PCOS based on the nomogram model was close to the ideal diagnostic rate, suggesting the accuracy of the nomogram model for the diagnosis of PCOS (Figure 6B). DCA showed that the net benefits generated by the nomogram model were at the high-risk threshold from 0.1 to 1.0, suggesting that the nomogram model had a higher clinical application value in the diagnosis of PCOS (Figure 6C). We then evaluated the nomogram model based on the RNA expression data of the five selected DEFRGs in our clinical specimens assessed using RT-PCR. Calibration and DCA curves successfully verified the accuracy and net clinical benefit of the nomogram model (Figures 6D,E). In addition, differences in the expressions of NOX1, ACVR1B, PHF21A, FTL, and GALNT14 were verified in our clinical specimens. The results indicated that all five DEFRGs were more highly expressed in patients with PCOS than in normal controls, which is in line with our predictions (Figure 7).
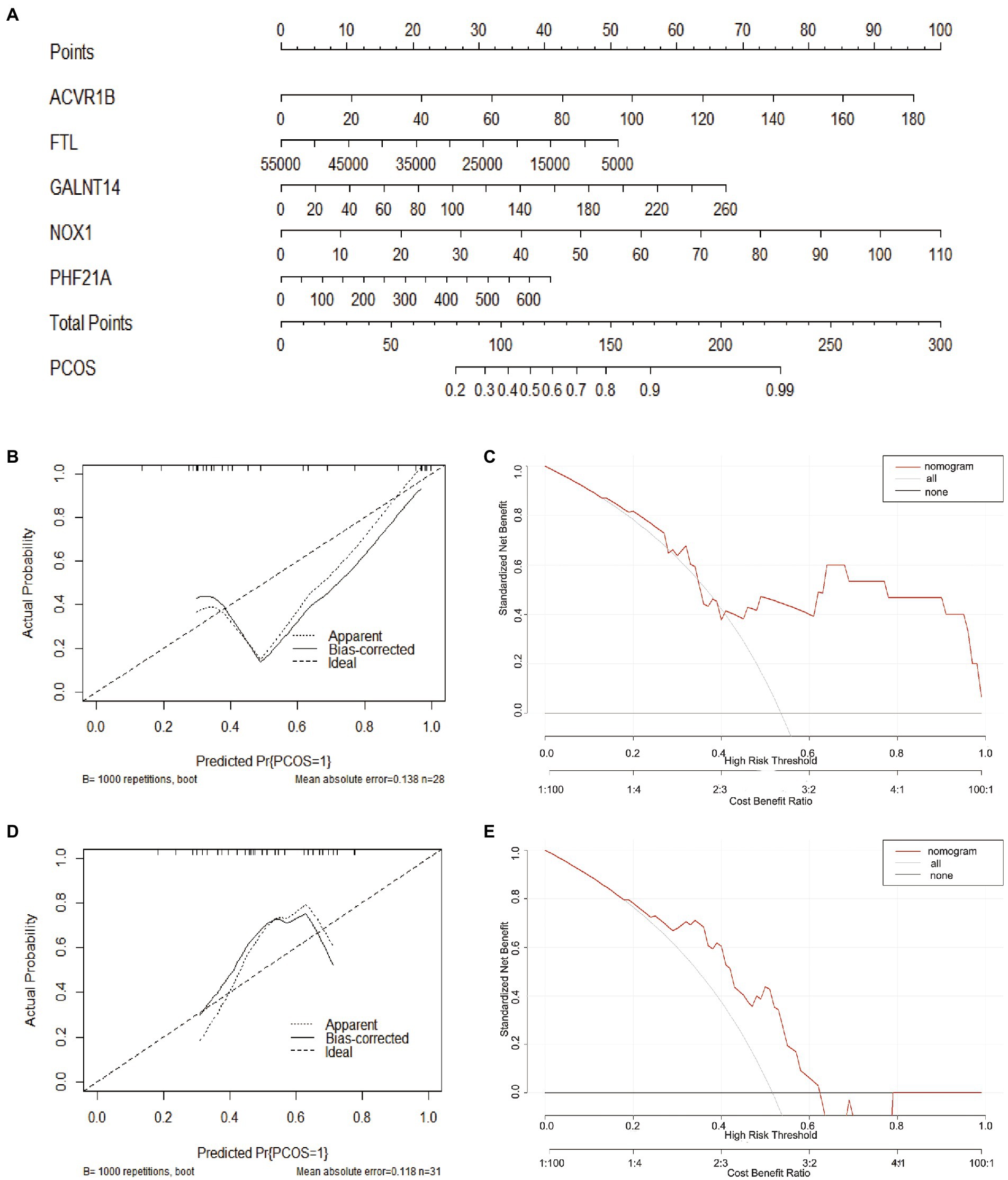
Figure 6. Construction of the nomogram model. (A) Construction of the nomogram model based on five key DEFRGs (NOX1, ACVR1B, PHF21A, FTL, and GALNT14). (B, D) Calibration curve suggests the accuracy of the nomogram model in the diagnosis of PCOS. (C, E) DCA suggests that the nomogram model is of higher clinical application value in the diagnosis of PCOS.
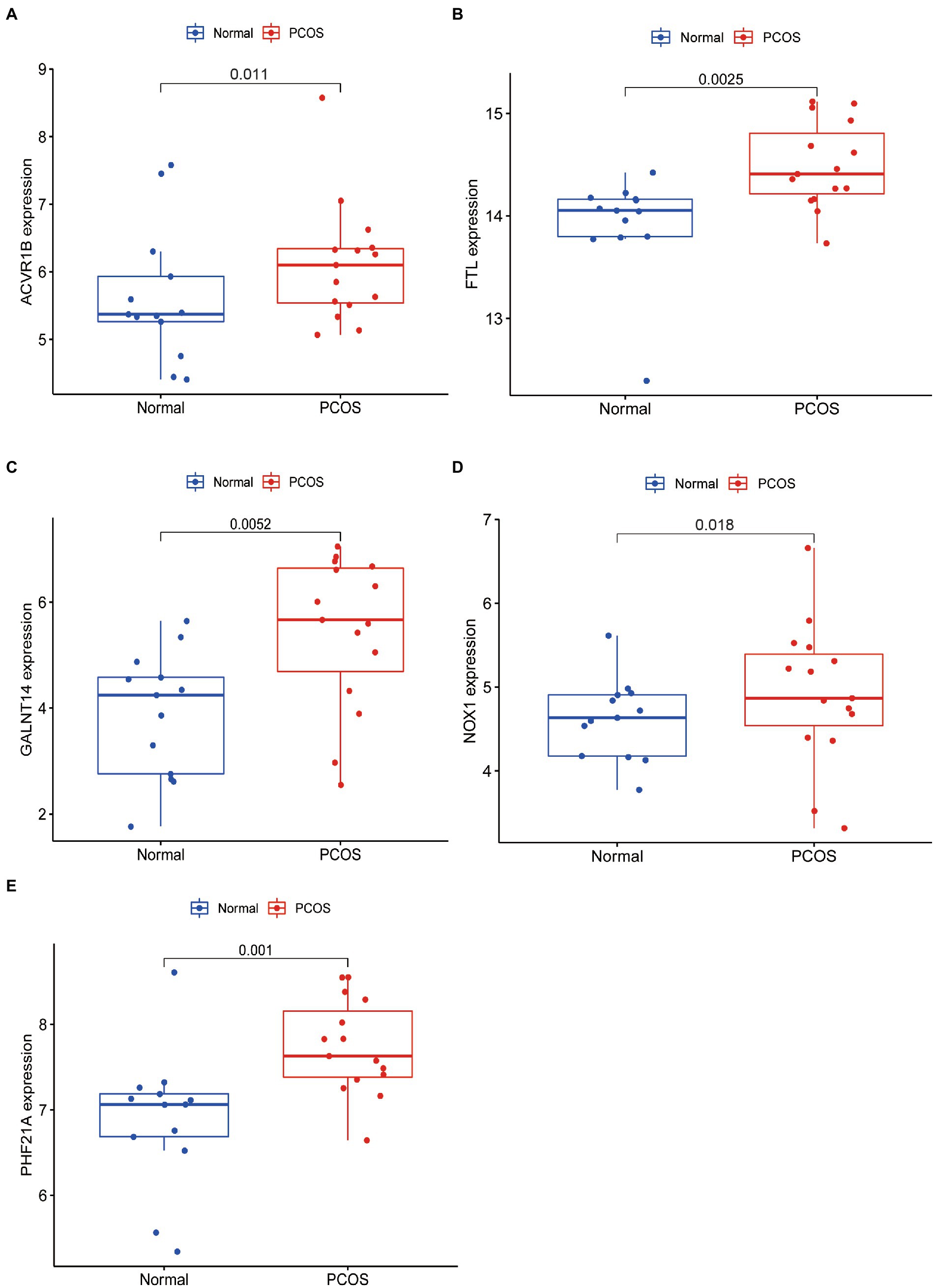
Figure 7. qRT-PCR validated five key DEFRGs differential expression between normal controls and patients with PCOS. (A) ACVR1B; (B) FTL; (C) GALNT14; (D) NOX1; and (E) PHF21A.
Gene set enrichment analysis
We then explored the specific GO function and signaling pathways enriched by the five DEFRGs and the potential mechanisms of the five DEFRGs in the pathogenesis of PCOS. The GSEA results revealed that the main enriched signaling pathways for high NOX1 expression were ALLOGRAFT_REJECTION, ENDOCYTOSIS, FC_GAMMA_R_MEDIATED_PHAGOCYTOSIS, LEISHMANIA_INFECTION, LYSOSOME, and SYSTEMIC_LUPUS_ERYTHEMATOSUS (Figure 8A). The main enriched pathways for high ACVR1B expression were ALLOGRAFT_REJECTION, ANTIGEN_PROCESSING_AND_PRESENTATION, AUTOIMMUNE_THYROID_DISEASE, GRAFT_VERSUS_HOST_DISEASE, LEISHMANIA_INFECTION, and SYSTEMIC_LUPUS_ERYTHEMATOSUS (Figure 8B). The main enriched pathways for high PHF21A expression were ALLOGRAFT_REJECTION, AUTOIMMUNE_THYROID_DISEASE, GRAFT_VERSUS_HOST_DISEASE, LEISHMANIA_INFECTION, SYSTEMIC_LUPUS_ERYTHEMATOSUS, and TYPE_I_DIABETES_MELLITUS (Figure 8C). The main enriched pathways for high FTL expression were ALLOGRAFT_REJECTION, AUTOIMMUNE_THYROID_DISEASE, LEISHMANIA_INFECTION, LYSOSOME, SYSTEMIC_LUPUS_ERYTHEMATOSUS, and TYPE_I_DIABETES_MELLITUS (Figure 8D). Finally, the main enriched pathways for high SNW1 expression were ALLOGRAFT_REJECTION, B_CELL_RECEPTOR_SIGNALING_PATHWAY, INSULIN_SIGNALING_PATHWAY, LEISHMANIA_INFECTION, LYSOSOME, and SYSTEMIC_LUPUS_ERYTHEMATOSUS (Figure 8E).
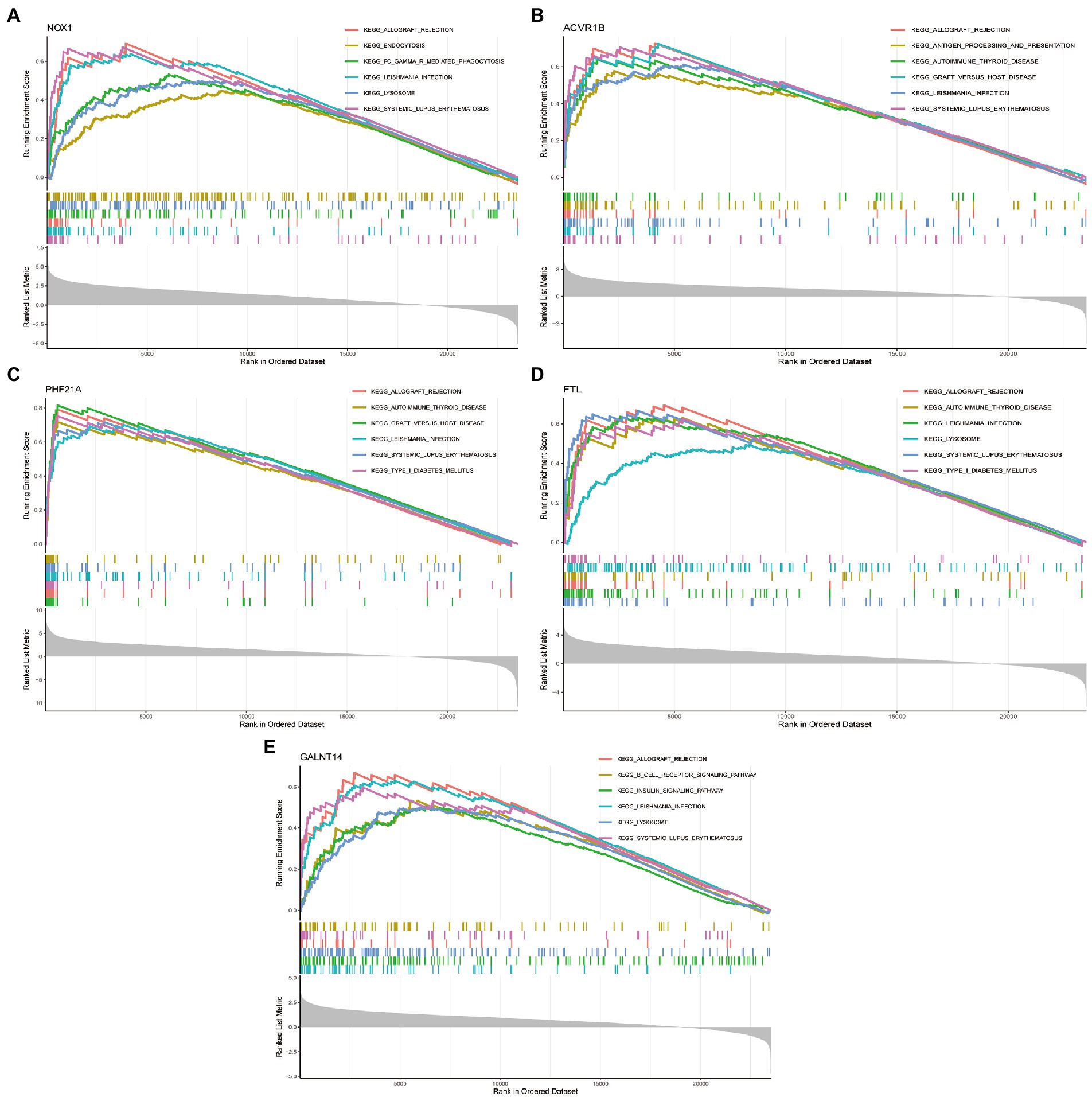
Figure 8. GSEA enrichment analysis showing signaling pathways enriched by five DEFRGs. (A) NOX1; (B) ACVR1B; (C) PHF21A; (D) FTL; and (E) GALNT14.
Construction of the ceRNA network
A lncRNA–miRNA–mRNA network was constructed based on the five key DEFRGs. First, the microRNAs (miRNAs) interacting with the five key DEFRGs were obtained based on the miRWalk database.4 The miRNAs that interacted with lncRNAs were acquired from the miRNA target prediction database.5 The predicted miRNAs were intersected, and the lncRNA–miRNA–mRNA network included five key DEFRGs, 117 lncRNAs, and 67 miRNAs visualized using Cytoscape software (Figure 9).
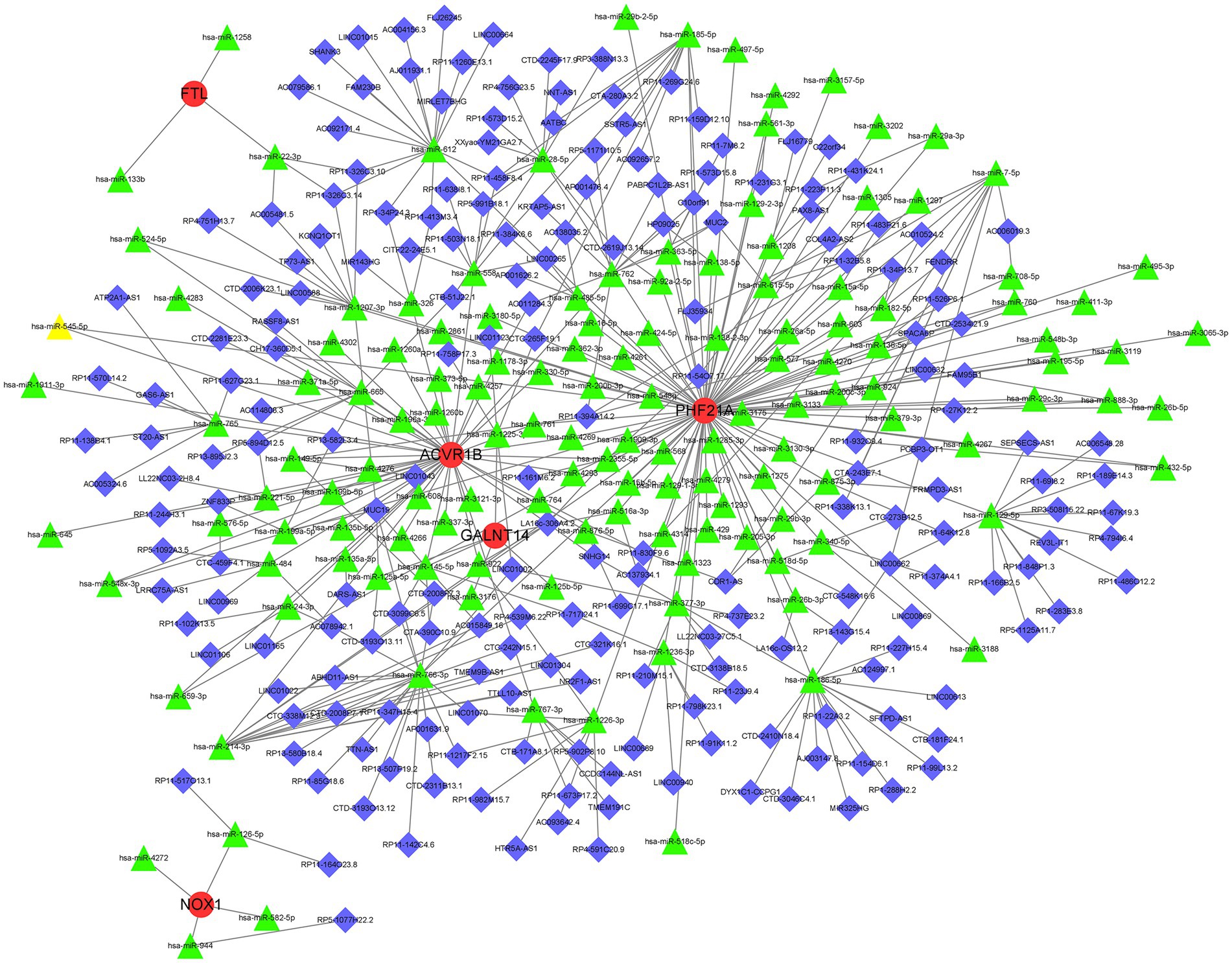
Figure 9. Representative lncRNA–miRNA–mRNA network was obtained by Cytoscape software. Red, green, and blue represent mRNA, miRNA, and lncRNA, respectively.
Discussion
In this study, we acquired 10 upregulated DEFRGs based on GEO datasets to explore their roles in the pathogenesis of PCOS using a series of differential expression analyses. GO and KEGG enrichment analyses of 10 DEFRGs indicated that these genes are involved in some pathways associated with immunity and ferroptosis, suggesting that immunity and ferroptosis may be involved in the pathogenesis of PCOS. In addition, five key DEFRGs involved in the diagnosis of PCOS were screened using LASSO regression and the SVM-RFE algorithm. Finally, we constructed a lncRNA–miRNA–mRNA network associated with the five DEFRGs. Increasing evidence indicates that the lncRNA–miRNA–mRNA network plays a critical role in the development of PCOS. For example, Liu G et al. (25) revealed that the lncRNA PVT1/MicroRNA-17-5p/PTEN network was related to the secretion of E2 and P4, proliferation, and apoptosis of granulosa cells in PCOS. Guo et al. (26) illustrated that HOTAIRM1 could sponge miR-433-5p to promote PIK3CD expression, thereby regulating the growth and apoptosis of granulose cells in PCOS. However, the ceRNA network found in our study has not yet been studied. In summary, our study illustrates the potential role of ferroptosis in the pathogenesis of PCOS from the perspective of comprehensive bioinformatics analysis and provides a novel perspective for the clinical diagnosis and treatment of PCOS.
In the present study, five key DEFRGs (NOX1, ACVR1B, PHF21A, FTL, and GALNT14) were identified as the most significant genes related to PCOS pathogenesis, and an accurate and clinically valuable nomogram model was constructed. Ferroptosis is a type of cell death caused by the accumulation of lipid peroxidation products and lethal ROS from iron metabolism (27, 28). The mitochondrial respiratory chain and NADPH oxidase of the NADPH oxidase (NOX) family are major sources of reactive oxygen species (ROS) in human neuronal cells, cardiomyocytes, and keratinocytes (29–31). NOX1, a member of the NOX family, promotes ROS release and ferroptosis (32). Zhang et al. (33) found that ferric ammonium citrate (FAC) increased the ferric content in a human granulosa-like tumor cell line (KGN) by activating the transferrin receptor (TFRC). Iron uptake then mediates the activation of NOX1 signaling, which induces the release of ROS and mitochondrial damage. Therefore, the inhibitory effects of TFRC/NOX1 signaling on follicular genesis may be a potential treatment for PCOS. polypeptide N-acetylgalactosaminyltransferase 14 (GALNT14) is a member of the acetylgalactosaminyltransferase family that can initiate protein O-glycosylation by transferring the GalNAc residue of UDP-GalNAc to the hydroxyl group of Ser or Thr (34). Li et al. (35) indicated that GALNT14 regulates ferroptosis and apoptosis in ovarian cancer by targeting the EGFR/mTOR pathway. Ferritin is the only protein capable of storing substantial amounts of iron, and it plays a key role in regulating cellular iron metabolism. The heavy (H) and light (L) chain subunits of ferritin (FTH and FTL, respectively) are responsible for intracellular iron storage (36). Therefore, FTH1 and FTL levels are positively correlated with ferroptosis and can function as biomarkers of ferroptosis. ACVR1B, also known as ALK-4, acts as a transducer of activin-like ligands that belong to the growth and differentiation factors of the TGF-β superfamily of signaling proteins (37). Kota Fujiki et al. (38) reported that cadmium-and-erabine-induced cell death, including ferroptosis in renal proximal tubular epithelial cells, can be inhibited by blocking the ALK4/5 signaling pathway, suggesting that ALK-4 is related to ferroptosis. PHD finger protein 21A (PHF21A) is also known as BHC80. To date, no relevant studies have reported the mechanism of PHF21A involvement in iron deficiency and PCOS. We selected five key DEFRGs related to PCOS pathogenesis, which will be further elucidated in in vitro and in vivo experiments.
This study has some limitations. First, our research results were based on bioinformatics analysis. Further basic and clinical experiments are required to verify these results. Second, owing to the limitations of the data in the public database, the sample size included in our study was not large enough, and the research results may deviate from the real situation.
Conclusion
In the present study, we identified several ferroptosis-related genes that are strongly associated with PCOS pathogenesis, which may provide a novel perspective for the clinical diagnosis and treatment of PCOS. However, further studies are necessary to explore the mechanisms of ferroptosis and its role in PCOS pathogenesis.
Data availability statement
The datasets presented in this study can be found in online repositories. The names of the repository/repositories and accession number(s) can be found in the article/Supplementary material.
Ethics statement
The study was approved by the Ethics Committee of the ShengJing Hospital of CMU, and informed consent was obtained from all participants. The patients/participants provided their written informed consent to participate in this study.
Author contributions
SL, XJ, HG, and FB conceived and designed the study, developed the methodology, analyzed and interpreted the data, wrote, reviewed, and revised the manuscript. All authors contributed to the article and approved the submitted version.
Funding
This work was supported by 345 Talent Project of Shengjing Hospital of China Medical University (No. M0695); Shenyang Young and Middle-aged Science and Technology Innovation Talents Support Program, Project (No. RC210436); Joint Program of Applied Basic Research of Liaoning Province, Project; Key Laboratory of Reproductive and Genetic Medicine (China Medical University); National Health Commission.
Acknowledgments
We thank the authors for providing the GEO public datasets. We thank LetPub (www.letpub.com) for linguistic assistance during the preparation of this manuscript.
Conflict of interest
The authors declare that the research was conducted in the absence of any commercial or financial relationships that could be construed as a potential conflict of interest.
Publisher’s note
All claims expressed in this article are solely those of the authors and do not necessarily represent those of their affiliated organizations, or those of the publisher, the editors and the reviewers. Any product that may be evaluated in this article, or claim that may be made by its manufacturer, is not guaranteed or endorsed by the publisher.
Supplementary material
The Supplementary material for this article can be found online at: https://www.frontiersin.org/articles/10.3389/fmed.2023.1120693/full#supplementary-material
Footnotes
1. ^http://www.zhounan.org/ferrdb/current/
2. ^http://mirwalk.umm.uni-heidelberg.de/
References
1. Visser, JA. The importance of metabolic dysfunction in polycystic ovary syndrome. Nat Rev Endocrinol. (2021) 17:77–8. doi: 10.1038/s41574-020-00456-z
2. Walters, KA, Gilchrist, RB, Ledger, WL, Teede, HJ, Handelsman, DJ, and Campbell, RE. New perspectives on the pathogenesis of PCOS: neuroendocrine origins. Trends Endocrinol Metab. (2018) 29:841–52. doi: 10.1016/j.tem.2018.08.005
3. Balen, AH, Morley, LC, Misso, M, Franks, S, Legro, RS, Wijeyaratne, CN, et al. The management of anovulatory infertility in women with polycystic ovary syndrome: an analysis of the evidence to support the development of global WHO guidance. Hum Reprod Update. (2016) 22:687–708. doi: 10.1093/humupd/dmw025
4. Escobar-Morreale, HF. Polycystic ovary syndrome: definition, aetiology, diagnosis and treatment. Nat Rev Endocrinol. (2018) 14:270–84. doi: 10.1038/nrendo.2018.24
5. Zhang, D, Yi, S, Cai, B, Wang, Z, Chen, M, Zheng, Z, et al. Involvement of ferroptosis in the granulosa cells proliferation of PCOS through the circRHBG/miR-515/SLC7A11 axis. Ann Transl Med. (2021) 9:1348. doi: 10.21037/atm-21-4174
6. Christ, JP, and Falcone, T. Bariatric surgery improves Hyperandrogenism, menstrual irregularities, and metabolic dysfunction among women with polycystic ovary syndrome (PCOS). Obes Surg. (2018) 28:2171–7. doi: 10.1007/s11695-018-3155-6
7. Tagliaferri, V, Romualdi, D, Scarinci, E, Cicco, S, Florio, CD, Immediata, V, et al. Melatonin treatment may be able to restore menstrual Cyclicity in women with PCOS: a pilot study. Reprod Sci. (2018) 25:269–75. doi: 10.1177/1933719117711262
8. Yin, J, Hong, X, Ma, J, Bu, Y, and Liu, R. Serum trace elements in patients with polycystic ovary syndrome: a systematic review and meta-analysis. Front Endocrinol (Lausanne). (2020) 11:572384. doi: 10.3389/fendo.2020.572384
9. Escobar-Morreale, HF. Iron metabolism and the polycystic ovary syndrome. Trends Endocrinol Metab. (2012) 23:509–15. doi: 10.1016/j.tem.2012.04.003
10. Dixon, SJ, Lemberg, KM, Lamprecht, MR, Skouta, R, Zaitsev, EM, Gleason, CE, et al. Ferroptosis: an iron-dependent form of nonapoptotic cell death. Cells. (2012) 149:1060–72. doi: 10.1016/j.cell.2012.03.042
11. Yan, HF, Zou, T, Tuo, QZ, Xu, S, Li, H, Belaidi, AA, et al. Ferroptosis: mechanisms and links with diseases. Signal Transduct Target Ther. (2021) 6:49. doi: 10.1038/s41392-020-00428-9
12. Yao, X, Li, W, Fang, D, Xiao, C, Wu, X, Li, M, et al. Emerging roles of energy metabolism in Ferroptosis regulation of tumor cells. Adv Sci (Weinh). (2021) 8:e2100997. doi: 10.1002/advs.202100997
13. Mou, Y, Wang, J, Wu, J, He, D, Zhang, C, Duan, C, et al. Ferroptosis, a new form of cell death: opportunities and challenges in cancer. J Hematol Oncol. (2019) 12:34. doi: 10.1186/s13045-019-0720-y
14. Tang, S, and Xiao, X. Ferroptosis and kidney diseases. Int Urol Nephrol. (2020) 52:497–503. doi: 10.1007/s11255-019-02335-7
15. Mahoney-Sánchez, L, Bouchaoui, H, Ayton, S, Devos, D, Duce, JA, and Devedjian, JC. Ferroptosis and its potential role in the physiopathology of Parkinson’s disease. Prog Neurobiol. (2021) 196:101890. doi: 10.1016/j.pneurobio.2020.101890
16. Elgendy, SM, Alyammahi, SK, Alhamad, DW, Abdin, SM, and Omar, HA. Ferroptosis: an emerging approach for targeting cancer stem cells and drug resistance. Crit Rev Oncol Hematol. (2020) 155:103095. doi: 10.1016/j.critrevonc.2020.103095
17. Li, M, Xin, S, Gu, R, Zheng, L, Hu, J, Zhang, R, et al. Novel diagnostic biomarkers related to oxidative stress and macrophage Ferroptosis in atherosclerosis. Oxidative Med Cell Longev. (2022) 2022:1–18. doi: 10.1155/2022/8917947
18. Stancic, A, Velickovic, K, Markelic, M, Grigorov, I, Saksida, T, Savic, N, et al. Involvement of Ferroptosis in diabetes-induced liver pathology. Int J Mol Sci. (2022) 23:9309. doi: 10.3390/ijms23169309
19. Liu, H, Xie, J, Fan, L, Xia, Y, Peng, X, Zhou, J, et al. Cryptotanshinone protects against PCOS-induced damage of ovarian tissue via regulating oxidative stress, mitochondrial membrane potential, inflammation, and apoptosis via regulating Ferroptosis. Oxidative Med Cell Longev. (2022) 2022:1–21. doi: 10.1155/2022/8011850
20. Zhang, J, Ding, N, Xin, W, Yang, X, and Wang, F. Quantitative proteomics reveals that a prognostic signature of the endometrium of the polycystic ovary syndrome women based on Ferroptosis proteins. Front Endocrinol (Lausanne). (2022) 13:871945. doi: 10.3389/fendo.2022.871945
21. Chen, S, Jiang, Y, Qi, X, Song, P, Tang, L, and Liu, H. Bioinformatics analysis to obtain critical genes regulated in subcutaneous adipose tissue after bariatric surgery. Adipocytes. (2022) 11:550–61. doi: 10.1080/21623945.2022.2115212
22. Jin, X, Wang, J, Ge, L, and Hu, Q. Identification of immune-related biomarkers for sciatica in peripheral blood. Front Genet. (2021) 12:781945. doi: 10.3389/fgene.2021.781945
23. Pan, X, and Bi, F. A potential immune-related long non-coding RNA prognostic signature for ovarian cancer. Front Genet. (2021) 12:694009. doi: 10.3389/fgene.2021.694009
24. Huang, C, Chen, L, Zhang, Y, Wang, L, Zheng, W, Peng, F, et al. Predicting AURKA as a novel therapeutic target for NPC: a comprehensive analysis based on bioinformatics and validation. Front Genet. (2022) 13:926546. doi: 10.3389/fgene.2022.926546
25. Liu, G, Liu, S, Xing, G, and Wang, F. lncRNA PVT1/MicroRNA-17-5p/PTEN Axis regulates secretion of E2 and P4, proliferation, and apoptosis of ovarian Granulosa cells in PCOS. Mol Ther Nucleic Acids. (2020) 20:205–16. doi: 10.1016/j.omtn.2020.02.007
26. Guo, H, Li, T, and Sun, X. LncRNA HOTAIRM1, miR-433-5p and PIK3CD function as a ceRNA network to exacerbate the development of PCOS. J Ovarian Res. (2021) 14:19. doi: 10.1186/s13048-020-00742-4
27. Li, J, Cao, F, Yin, HL, Huang, ZJ, Lin, ZT, Mao, N, et al. Ferroptosis: past, present and future. Cell Death Dis. (2020) 11:88. doi: 10.1038/s41419-020-2298-2
28. Song, J, Liu, T, Yin, Y, Zhao, W, Lin, Z, Yin, Y, et al. The deubiquitinase OTUD1 enhances iron transport and potentiates host antitumor immunity. EMBO Rep. (2021) 22:e51162. doi: 10.15252/embr.202051162
29. Cadenas, S. ROS and redox signaling in myocardial ischemia-reperfusion injury and cardioprotection. Free Radic Biol Med. (2018) 117:76–89. doi: 10.1016/j.freeradbiomed.2018.01.024
30. Olguín-Albuerne, M, and Morán, J. Redox signaling mechanisms in nervous system development. Antioxid Redox Signal. (2018) 28:1603–25. doi: 10.1089/ars.2017.7284
31. Emmert, H, Fonfara, M, Rodriguez, E, and Weidinger, S. NADPH oxidase inhibition rescues keratinocytes from elevated oxidative stress in a 2D atopic dermatitis and psoriasis model. Exp Dermatol. (2020) 29:749–58. doi: 10.1111/exd.14148
32. Kain, HS, Glennon, EKK, Vijayan, K, Arang, N, Douglass, AN, Fortin, CL, et al. Liver stage malaria infection is controlled by host regulators of lipid peroxidation. Cell Death Differ. (2020) 27:44–54. doi: 10.1038/s41418-019-0338-1
33. Zhang, L, Wang, F, Li, D, Yan, Y, and Wang, H. Transferrin receptor-mediated reactive oxygen species promotes ferroptosis of KGN cells via regulating NADPH oxidase 1/PTEN induced kinase 1/acyl-CoA synthetase long chain family member 4 signaling. Bioengineered. (2021) 12:4983–94. doi: 10.1080/21655979.2021.1956403
34. Bennett, EP, Mandel, U, Clausen, H, Gerken, TA, and Fritz, TA. Control of Mucin-type O-glycosylation—a classification of the polypeptide GalNAc-transferase gene family. Glycobiology. (2012) 22:736–56. doi: 10.1093/glycob/cwr182
35. Li, HW, Liu, MB, Jiang, X, Song, T, Feng, SX, Wu, JY, et al. GALNT14 regulates ferroptosis and apoptosis of ovarian cancer through the EGFR/mTOR pathway. Fut. Oncol. (2022) 18:149–61. doi: 10.2217/fon-2021-0883
36. Honarmand Ebrahimi, K, Hagedoorn, PL, and Hagen, WR. Unity in the biochemistry of the iron-storage proteins ferritin and bacterioferritin. Chem Rev. (2015) 115:295–326. doi: 10.1021/cr5004908
37. Boueiz, A, Pham, B, Chase, R, Lamb, A, Lee, S, Naing, ZZC, et al. Integrative genomics analysis identifies ACVR1B as a candidate causal gene of emphysema distribution. Am J Respir Cell Mol Biol. (2019) 60:388–98. doi: 10.1165/rcmb.2018-0110OC
Keywords: ferroptosis, PCOS, SVM-RFE, LASSO, bioinformatics
Citation: Lin S, Jin X, Gu H and Bi F (2023) Relationships of ferroptosis-related genes with the pathogenesis in polycystic ovary syndrome. Front. Med. 10:1120693. doi: 10.3389/fmed.2023.1120693
Edited by:
Simcha Yagel, Hadassah Medical Center, IsraelReviewed by:
Jin Yu, Shanghai Jiao Tong University, Shanghai, ChinaZhong Xingming, Guangdong Provincial Family Planning Hospital, China
Copyright © 2023 Lin, Jin, Gu and Bi. This is an open-access article distributed under the terms of the Creative Commons Attribution License (CC BY). The use, distribution or reproduction in other forums is permitted, provided the original author(s) and the copyright owner(s) are credited and that the original publication in this journal is cited, in accordance with accepted academic practice. No use, distribution or reproduction is permitted which does not comply with these terms.
*Correspondence: Fangfang Bi, ✉ YmlmYW5nZmFuZzE2OEAxNjMuY29t