- Department of Respiratory Diseases, The Second Affiliated Hospital of Nanchang University, Nanchang, China
Background: Lung adenocarcinoma (LUAD) is one of the most frequent causes of tumor-related mortality worldwide. Recently, the role of N7-methylguanosine (m7G) in tumors has begun to receive attention, but no investigation on the impact of m7G on LUAD. This study aims to elucidate the significance of m7G on the prognosis and immunotherapy in LUAD.
Methods: Consensus clustering was employed to determine the molecular subtype according to m7G-related regulators extracted from The Cancer Genome Atlas (TCGA) database. Survival, clinicopathological features and tumor mutational burden (TMB) analysis were applied to research molecular characteristics of each subtype. Subsequently, “limma” package was used to screen differentially expressed genes (DEGs) between subtypes. In the TCGA train cohort (n = 245), a prognostic signature was established by univariate Cox regression, lasso regression and multivariate Cox regression analysis according to DEGs and survival analysis was employed to assess the prognosis. Then the prognostic value of the signature was verified by TCGA test cohort (n = 245), TCGA entire cohort (n = 490) and GSE31210 cohort (n = 226). Moreover, the association among immune infiltration, clinical features and the signature was investigated. The immune checkpoints, TMB and tumor immune dysfunction and exclusion (TIDE) were applied to predict the immunotherapy response.
Results: Two novel molecular subtypes (C1 and C2) of LUAD were identified. Compared to C2 subtype, C1 subtype had poorer prognosis and higher TMB. Subsequently, the signature (called the “m7G score”) was constructed according to four key genes (E2F7, FAM83A, PITX3, and HOXA13). The distribution of m7G score were significantly different between two molecular subtypes. The patients with lower m7G score had better prognosis in TCGA train cohort and three verification cohort. The m7G score was intensively related to immune infiltration. Compared with the lower score, the higher m7G score was related to remarkable upregulation of the PD-1 and PD-L1, the higher TMB and the lower TIDE score.
Conclusion: This study established a m7G-related signature for predicting prognosis and immunotherapy in LUAD, which may contribute to the development of new therapeutic strategies for LUAD.
Introduction
Lung adenocarcinoma (LUAD) accounts for the largest proportion in non-small cell lung cancer (NSCLC) (1). Since patients with LUAD suffer from advanced disease or have distant metastasis when first diagnosed, they have a poor prognosis, and the overall 5-year survival rate is still below 20% (2, 3). Impressively, immune checkpoint blockade (ICB) has become a promising therapy strategy for NSCLC (4). However, some patients have a low response rate to ICB treatment, or even drug resistance, thus resulting in disease relapse or dead cases (5, 6). Therefore, it is essential to identify a novel biomarker in LUAD, in order to improve the outcomes of patients and formulate personalized treatment strategies.
Increasing evidence indicates that the initiation and progression of lung cancer depends not only on genetic variation, but also on epigenetic dysregulation (7, 8). As an important part of epigenetic modification, RNA modification is involved in regulating many physiological processes and disease occurrence (9). Besides, dynamic regulation and disruption of these RNA modifications are also related to the tumorigenesis, maintenance and progression of lung cancer (10, 11). Among numerous RNA dynamic modifications, N6-Methyladenosine (m6A), 5-Methylcytosine (m5C), and N7-methylguanosine (m7G) are extremely common (12). Importantly, m7G is the most prevalent modifications of RNA caps (13), which occurs in various RNAs of eukaryotes (14). m7G modification has a significant impact on RNA metabolism, processing and function (15). Nevertheless, the exploration of m7G-related regulators on tumors have only recently begun to receive attention owing to technological limitations. Mis-regulated m7G modification could disturb the translation of many oncogenic transcripts involved in RPTOR/ULK1/autophagy pathway, which contributed to esophageal squamous cell carcinoma oncogenesis (16). EIF4E is regarded as one of m7G-related regulators, whose phosphorylation could increase the translations of oncogene mRNAs to promote prostate cancer tumorigenesis (17). Moreover, one study demonstrated that METTL1 and WDR4 were upregulated in lung cancer samples and vital for the progression (18). Besides, RNA dynamic modification could influence the response function and maturation of tumor immune cells (19). So far, the overall impact of m7G-related regulators on the immunotherapeutic response in LUAD and its relationship with patient prognosis and treatment are still unclear.
With the advances in high-throughput sequencing technique, research on tumor genes is more in-depth, which can help to classify tumors to some content. There are many signatures that assess the prognosis of LUAD according to various subtypes (20–22). However, these signatures are still far from guiding precise treatment, which urgently requires a reliable signature. Here, two molecular subtypes of LUAD were constructed according to the gene expression of m7G-related regulators. We further evaluated the relation between survival, clinical characteristics, immune infiltration and molecular subtype. Then, a novel m7G score was established to quantify the m7G modification patterns, which was proven to be an independent predictor of LUAD prognosis. Moreover, the prognostic signature effectiveness was validated by the internal and external cohort (GSE31210). Furthermore, we elucidated whether this signature could provide reference for clinical immunotherapy and chemotherapy. In conclusion, this study not only provides a novel understanding of molecular subtype by m7G regulators, but also built a robust signature to estimate prognosis and guide individualized treatments in LUAD.
Materials and methods
Lung adenocarcinoma datasets
We obtained gene expression profile, clinical and somatic mutation data of LUAD from The Cancer Genome Atlas (TCGA) database.1 Four hundred ninety LUAD cases were included in the follow-up study after removing patients with survival time less than 30 days. We acquired the external verification cohort (GSE31210) from Gene Expression Omnibus (GEO) database.2 226 cases were finally included after processing as TCGA data. 29 m7G regulators were extracted from previous report (23) and three m7G-related gene sets in MSigDB database3 (Supplementary Table 1).
Landscape of genetic variation and identification molecular subtype
The expression of m7G regulators was extracted from TCGA-LUAD dataset. Then various methods were applied to depict the genetic variation of m7G regulators. The expression of m7G regulators was compared between tumor and normal groups. The mutation map was presented by using “maftools” package. Next, Cox analysis was used to filter genes correlated with LUAD prognosis (P < 0.05).
The “ConsensusClusterPlus” R package was employed to identify molecular subtype by consensus clustering of prognostic gene (parameters: reps = 50, pItem = 0.8, pFeature = 1, clusterAlg = “pam,” distance = “Pearson”) (24). Pam and Pearson distances were used as the clustering algorithm and distance measure, respectively. Furthermore, the sample’s distribution was characterized by principal component analysis (PCA). Moreover, we employed “survival” package to investigate the survival differences among subtypes. Besides, “heatmap” package was applied to explore the relation among molecular subtypes, expression of prognostic gene and clinicopathological features.
Biological function analysis and immune infiltration profile estimation
We investigated the biological process of distinct molecular subtype by Gene Set Enrichment Analysis (GSEA). The “h.all.v7.5.1.symbols.gmt” gene set was obtained from MSigDB database. We applied the CIBERSORT algorithm (25) to assess the immune status among different molecular subtypes. In recent years, tumor mutational burden (TMB) was widely applied to measure the effectiveness of ICB therapy (26). TMB score was calculated by using the somatic mutation data of each patient and then we compared TMB score in different subtypes. In addition, patients were further separated into low and high TMB groups on the basis of the threshold value (27) (10 mutations/megabase) of TMB and then we compared the frequency of high TMB in different subtypes. GSE135222 immunotherapy cohort including 27 cases was obtained from GEO database, which used to verify immunotherapy efficacy of different subtypes.
Screening of differentially expressed genes
We calculated the differentially expressed genes (DEGs) between molecular subtypes by using the Bioconductor “limma” package. The significance criteria were | log2FC| > 1 and false discovery rate (FDR) < 0.01. The upregulated and downregulated of DEGs were visualized by volcano map. The heatmap was also applied to show the distribution of DEGs in different subtypes.
Construction and validation of the m7G related signature
The all patients (n = 490) were randomly separated into train and test cohort according to the ratio of 1:1 by using “caret” package. In train cohort (n = 245), univariate Cox analysis was applied to screen genes related to the survival (P < 0.01). Then, least absolute shrinkage and selection operator (LASSO) regression was employed to further reduce the overfitting genes. Finally, a m7G related signature was established by multivariate Cox analysis, and we also called it m7G score. The previously reported formula (28) was used to calculate m7G score: Σ(gene expression level × corresponding coefficient). Patients was separated into high and low m7G score groups according to median m7G score. Then the sample’s distribution was characterized by PCA. We applied “survival” package to investigate the survival differences between two groups. We also plotted the receiver operating characteristics (ROC) curve to estimate the accuracy of the m7G Related signature by using “timeROC” package. The test cohort (n = 245) and the entire cohort (n = 490) were employed to validate the signature power by using the same analyses. We further used GSE31210 dataset (n = 226) to verify the robustness of the signature.
m7G related signature analysis and nomogram construction
Univariate and multivariate analysis were applied to demonstrate the independent prognosis of the m7G score. Then these results were visualized with the forest plots. We also constructed a nomogram by combining the age, gender, stage and m7G score for clinical practice. Additionally, calibration curve was applied to evaluate the predictive accuracy of the nomogram by using “rms” package.
Analysis of immune infiltration and anti-cancer treatment
We applied different bioinformatics methods including XCELL, TIMER, QUANTISEQ, MCPCOUNTER, EPIC, CIBERSORT-ABS, CIBERSORT, and single-sample gene set enrichment analysis (ssGSEA) (29, 30) to study the relation between m7G score and immune score. Subsequently, we also compared the expression of immune checkpoints between two groups. Mutation maps were manifested by using “maftools” package in two groups. In recent years, in addition to immune checkpoints, tumor immune dysfunction and exclusion (TIDE) was also widely employed to assess the effectiveness of ICB therapy (31). The TMB score was calculated by using the somatic mutation data of each patient and the TIDE score was calculated in the TIDE website4 (P < 0.05). Moreover, the drug sensitivity of each group was estimated by “pRRophetic” package (32). The half-maximal inhibitory concentration (IC50) of drugs was compared through Wilcoxon rank test between different m7G score groups (P < 0.05).
Statistical analysis
R software (version 4.1.0) was employed for all data analysis. Wilcoxon rank test was applied to compare m7G regulators expression between normal and LUAD groups. All above survival distribution was evaluated through survival analysis. The relation among molecular subtype, clinicopathological features and high TMB distribution was estimated by the chi-squared test. Immune infiltration, TMB and TIDE were also compared through Wilcoxon rank test. P < 0.05 was regarded as statistically significant.
Results
Genetic variation profile and m7G modification pattern
The overall research procedure is shown in Figure 1. Twenty-four regulators were manifested significant downregulation or overexpression in different groups according to P-value less than 0.05 (Figure 2A). The result of mutation map showed that EIF4G3 had the highest mutation frequency followed by LARP1 (Figure 2B). The correlation and prognostic significance of m7G regulators were presented in Figure 2C. Four genes including EIF4E3, LARP1, WDR4, and NCBP1 were significantly associated with prognosis. m7G regulators were also showed a remarkable interaction, which was critical for the development of the different m7G modification patterns. The above-mentioned results suggested m7G regulators may relate to tumorigenesis and progression in LUAD.
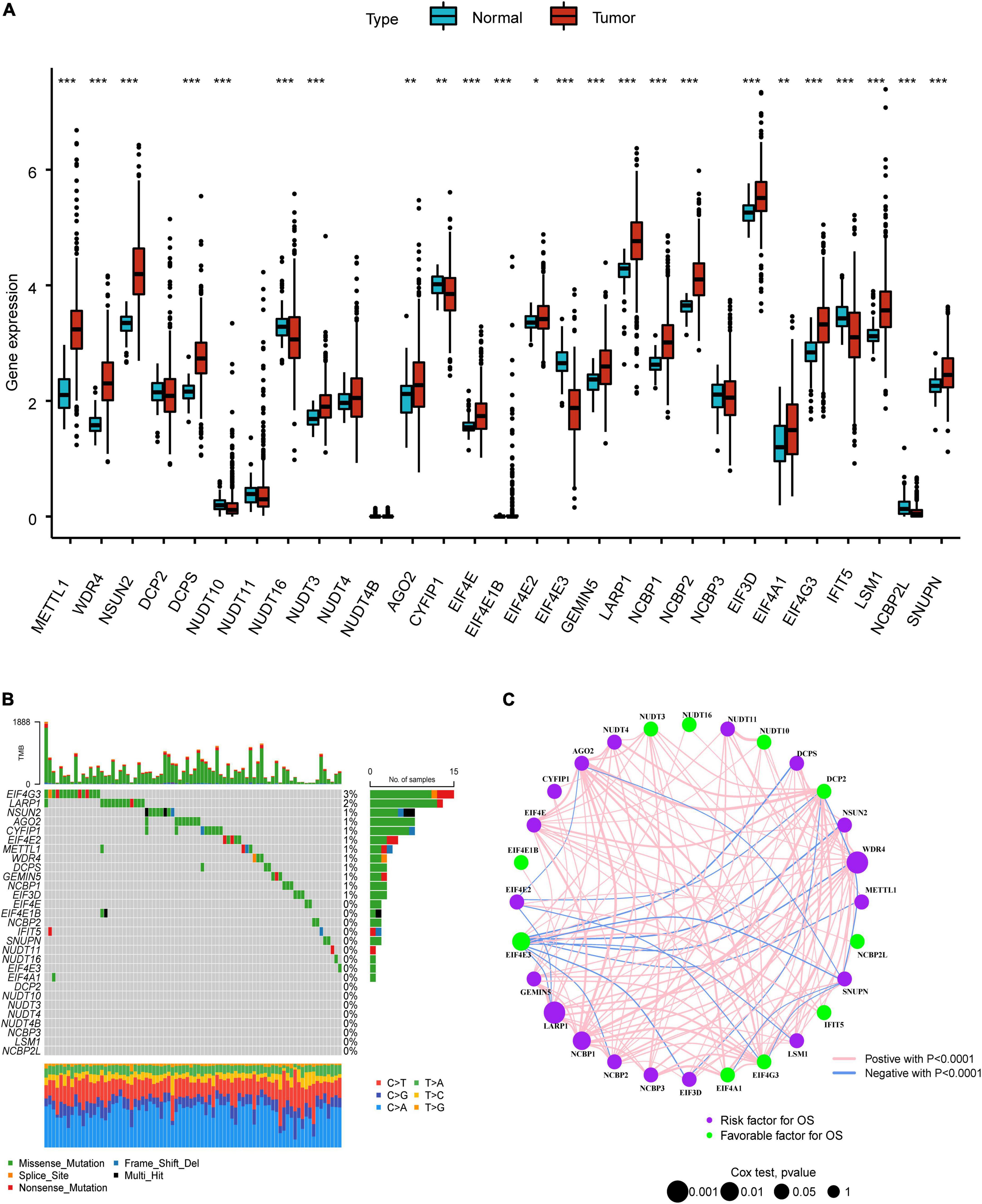
Figure 2. Landscape of genetic variation in LUAD. (A) The difference of m7G regulators expression level in normal and tumor group. (B) The mutation map of m7G regulators in LUAD. (C) The interplay and prognostic significance of m7G regulators. *P < 0.05; **P < 0.01; ***P < 0.001.
The LUAD patients were classified into two molecular subtypes (C1 and C2) by using “ConsensusClusterPlus” package according to prognostic genes. The intergroup correlation was lowest and intragroup correlation was highest when k = 2 (Figure 3A). Cumulative distribution function (CDF) curve performed the highest partition efficiency when k = 2 (Figures 3B,C). Taken together, two molecular subtypes were established according to the m7G modification pattern, including 245 patients of C1 and 245 patients of C2. The PCA analysis also demonstrated that the two subtypes could be completely distinguished (Figure 3D). The result of Kaplan–Meier analysis showed distinct survival outcome between two subtypes (P < 0.001) (Figure 3E), suggesting that C1 subtype had worse prognosis than C2. Subsequently, the clinical features and gene expression were compared, then we found patients in C1 subtype had poorer tumor stage than C2. EIF4E3 was upregulated in C2 subtype, while LARP1, WDR4, and NCBP1 were upregulated in C1 subtype (Figure 3F).
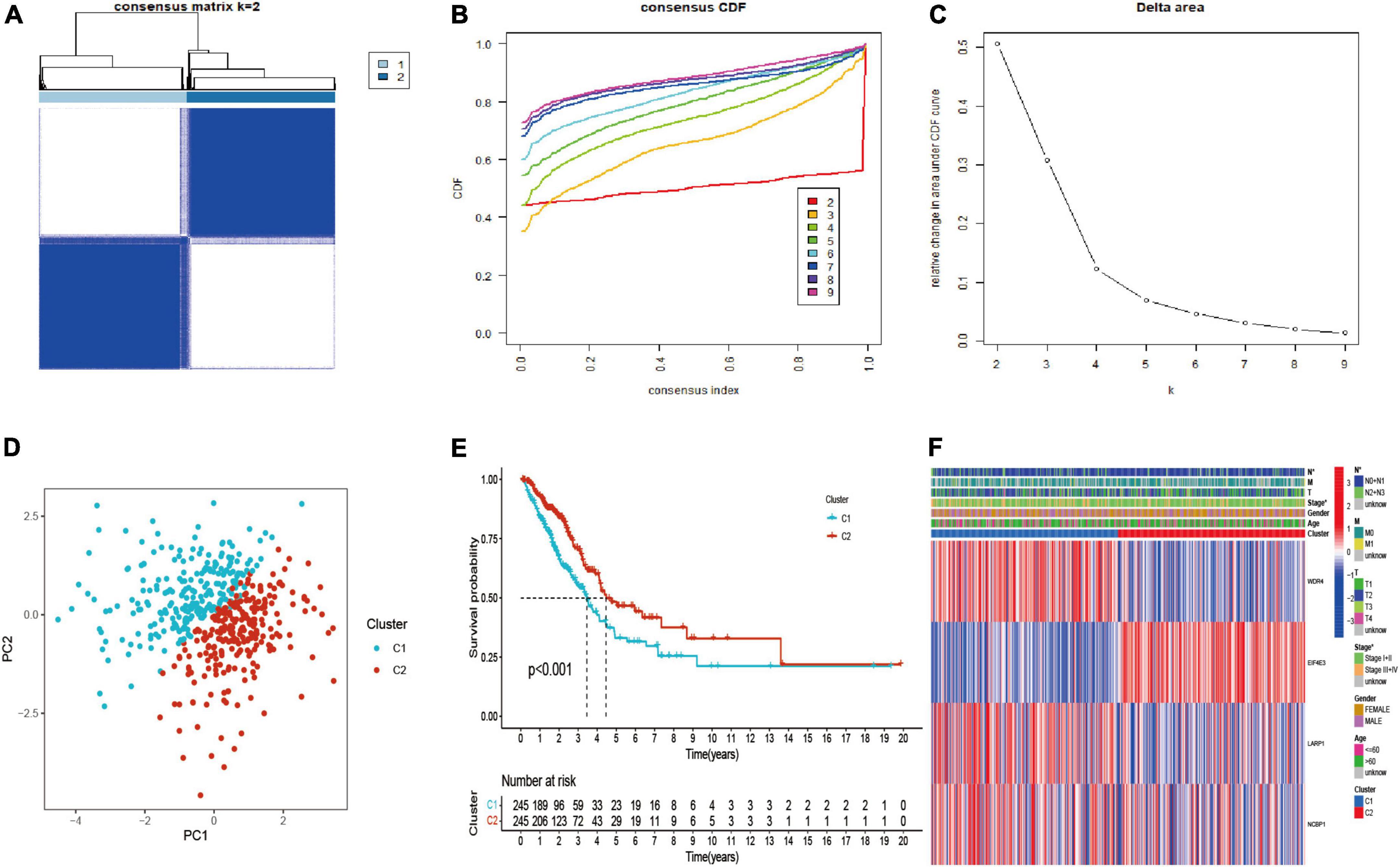
Figure 3. Identification, survival, and clinical characteristics analysis of molecular subtype. (A–C) The optimal value of consensus clustering. (D) Distribution of all patients. (E) Survival analysis in C1 and C2 subtypes. (F) Heatmap of prognostic gene and clinicopathological features between two subtypes. *P < 0.05.
Analysis of biological functional and immune infiltration
The results of GSEA presented diverse functional pathways between two subtypes. Functional analysis showed E2F_targets, G2M_checkpoint, glycolysis, MITOTIC spindle, MTORC1_signaling, MYC_targets_V1, MYC_targets_V2 were significantly enriched in C1 subtype (Figure 4A). While, there were significantly different pathways were enriched in C2 subtype, such as allograft rejection, complement, inflammatory response, interferon gamma response, IL2_STAT5_signaling, IL6_JAK_STAT3_signaling (Figure 4B).
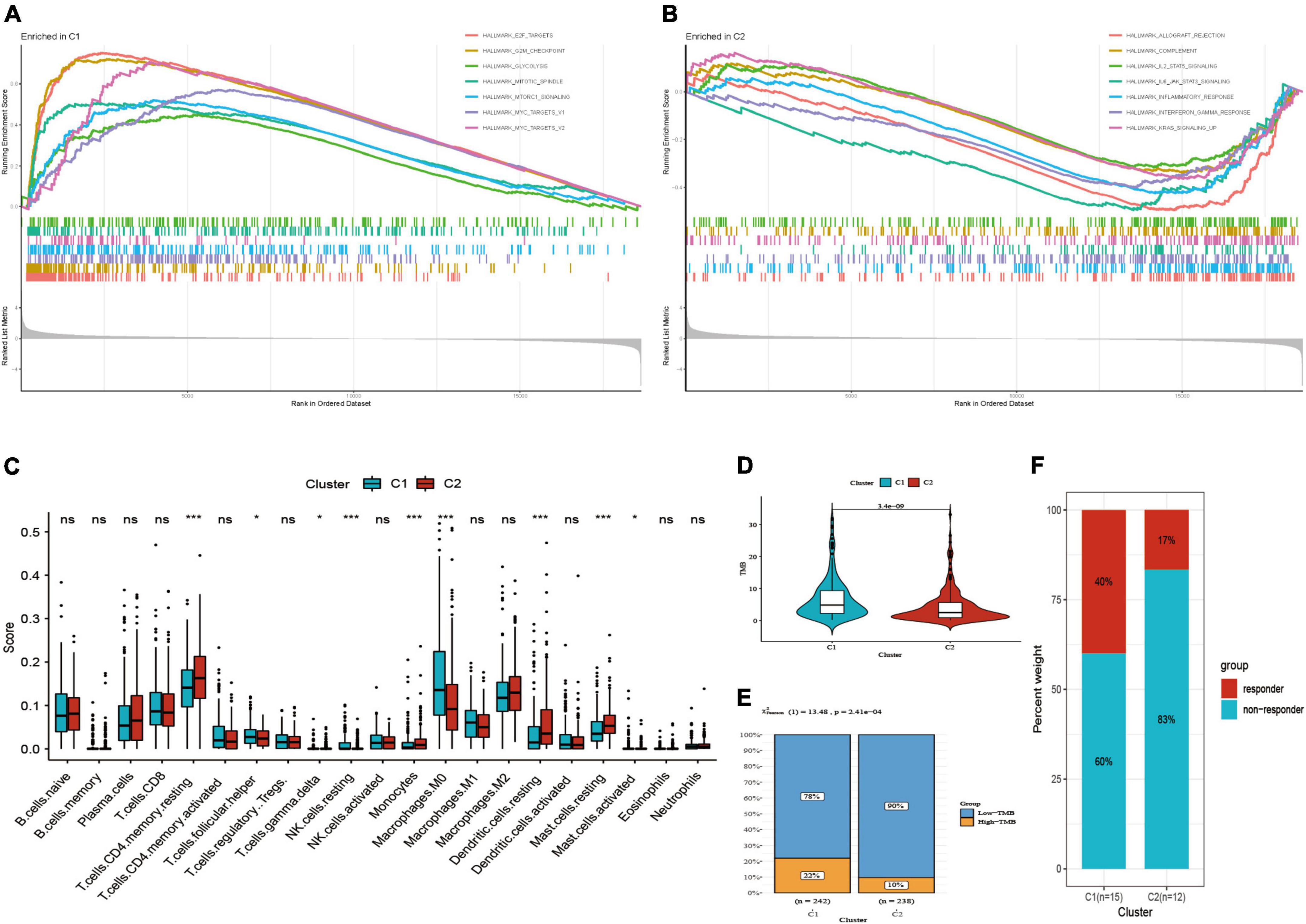
Figure 4. Functional pathways, immune infiltration and TMB analysis. (A) Functional pathways in C1 subtype. (B) Functional pathways in C2 subtype. (C) Comparison of immune score. (D) Comparison of TMB score. (E) Distribution of low and high TMB between two subtypes. (F) Distribution of response to immunotherapy between two subtypes in GSE135222. *P < 0.05; ***P < 0.001; ns: not significant.
Subsequently, we found that two subtypes had markedly different immune infiltration patterns (Figure 4C). The result of CIBERSORT algorithm showed the expression level of T cells follicular helper, resting NK cells, M0 macrophages, activated mast cells were high in C1 subtype, while resting CD4 memory T cells, T cells gamma delta, monocytes, resting dendritic cells, resting mast cells are high in C2 subtype. These suggested that two subtypes may have different immunotherapeutic response, so we further compared TMB score in two subtypes. Then we observed that C1 had higher TMB score as well as the proportion of high-TMB compared to C2 subtype (22 vs. 10%) (Figures 4D,E). GSE135222 immunotherapy cohort was divided into two subtypes by using the same method mentioned above, including 15 cases in C1 subtype and 12 cases in C2 subtype. The results presented that the proportion of response to immunotherapy was higher in C1 subtype than C2 subtype (40 vs. 17%) (Figure 4F).
Screening differentially expressed genes between m7G subtypes and construction of m7G related signature
Based on “limma” package, we identified 1,014 DEGs between m7G Subtypes, including 534 upregulated genes and 480 downregulated genes. Then the significant DEGs were visualized with volcano map (Figure 5A). The expression profiles of DEGs between C1 and C2 subtype were visualized with heatmap (Figure 5B). We utilized the train cohort to establish m7G related prognostic signature (n = 245). First, 201 genes correlated with patient prognosis were found by univariate analysis (Supplementary Table 2). Then we further applied LASSO regression to filter eight genes for the subsequent multivariate analysis (Figure 5C). Finally, four key genes including E2F7, FAM83A, PITX3, and HOXA13 were identified by using multivariate Cox regression (Figure 5D). The m7G score was calculated with the following formula: 0.5171 × E2F7 (mRNA level) + 0.1888 × FAM83A (mRNA level) + 1.5576 × PITX3 (mRNA level) + 0.5210 × HOXA13 (mRNA level). Then we split patients into high and low m7G score groups according to approach mentioned above (Figures 5E–G). The relative expressions of E2F7, FAM83A, PITX3, and HOXA13 in two groups were presented in Figure 5H. Patients had significant poor survival in high m7G score group (Figure 5I). The areas under the curves (AUC) for predicting survival rates at 1-, 3-, and 5-year were 0.736, 0.732, and 0.672, respectively (Figure 5J).
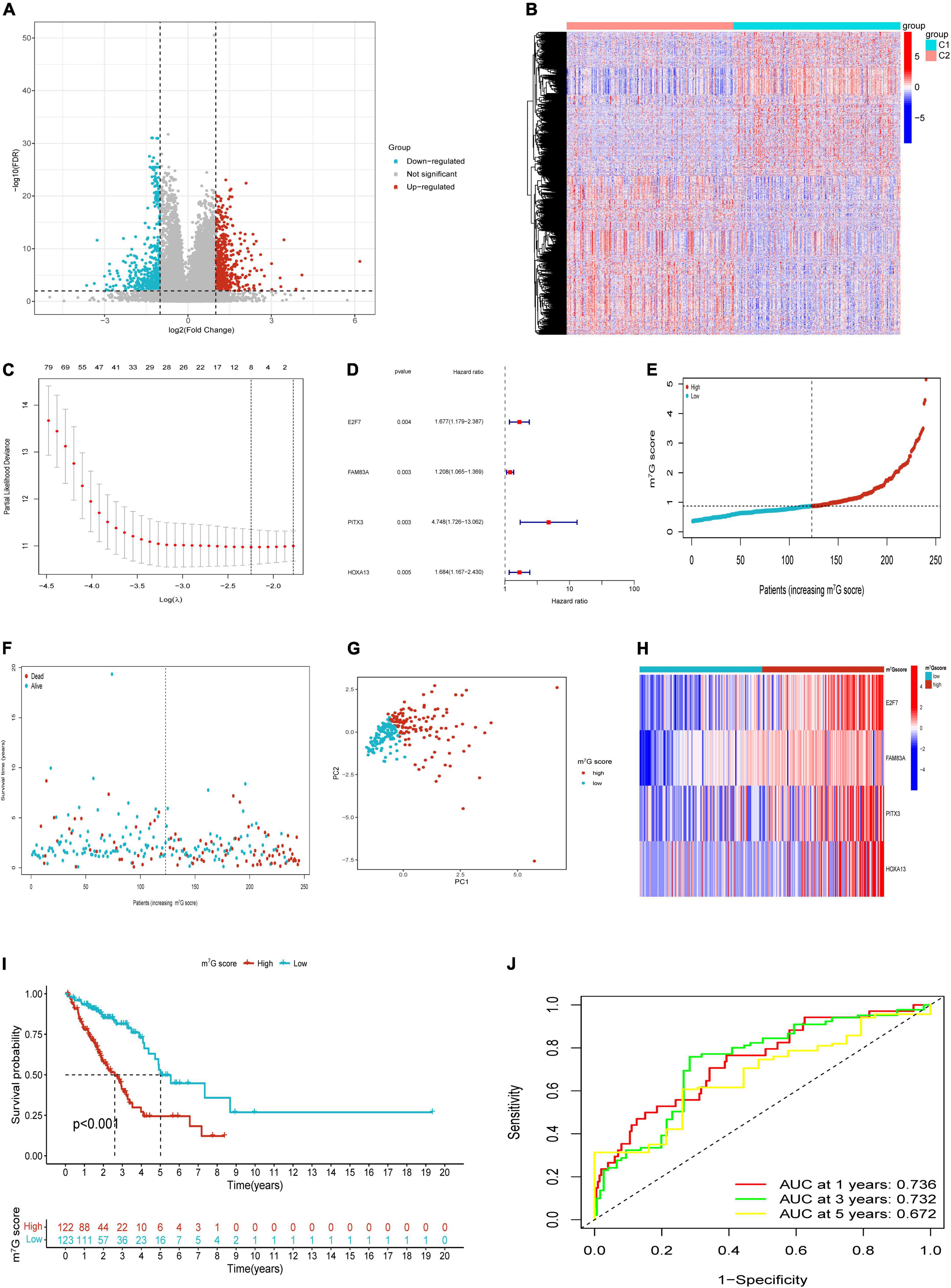
Figure 5. Construction of m7G related signature based on TCGA train cohort. (A) Volcano plot of DEGs. (B) Heatmap of DEGs. (C) Eight genes through Lasso regression analysis. (D) Four key genes through multivariate Cox regression. (E,F) Distribution of m7G score and survival state. (G) Distribution of patients according to m7G-related signature. (H) Heatmap of four genes expression between high and low m7G score groups. (I) Survival analysis in two groups. (J) AUC for predicting 1-, 3-, 5-years survival rates.
Verification of m7G related signature and analysis of survival in different clinical subgroups
Patients in the internal verification cohort (test and entire cohort) and the external verification cohort (GSE31210) were categorized into two groups on the basis of the same risk formula in train cohort. Patients of test cohort were classified into two groups (Figures 6A–C), which were consistent with the result of the train cohort. The heatmap showed the expression profile of four key gene were apparently different between two groups (Figure 6D). The Kaplan–Meier curve also indicated that two groups had distinct survival (Figure 6E). The area of AUC verified that the signature was a great indicator for assessing prognosis in LUAD (Figure 6F). The similar results were acquired in the entire cohort and GSE31210 cohort (Figures 6G–L, 7A–F). On the basis of Kaplan–Meier analysis of entire TCGA cohort, we also found that patients presented lower survival rate in high m7G score group among different clinical subgroups compared to low m7G score group (Supplementary Figure 1).
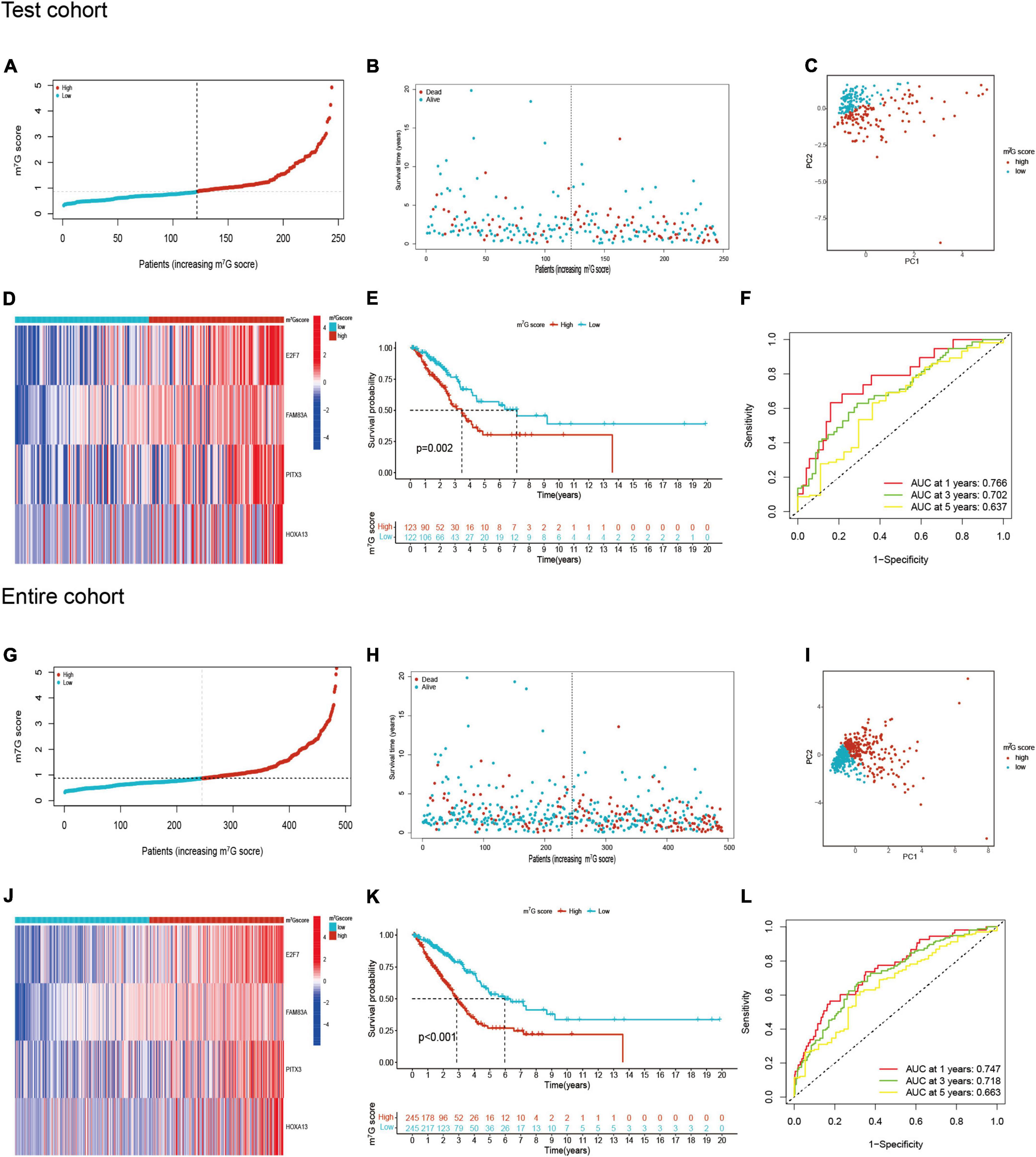
Figure 6. m7G related signature validation in TCGA test cohort and TCGA entire cohort. (A,B) Distribution of m7G score and survival state in TCGA test cohort. (C) Distribution of patients in TCGA test cohort. (D) Four genes expression between two groups in TCGA test cohort. (E) Survival analysis between two groups in TCGA test cohort. (F) AUC for predicting 1-, 3-, 5-years survival rates in TCGA test cohort. (G–L) The results of validation in TCGA entire cohort.
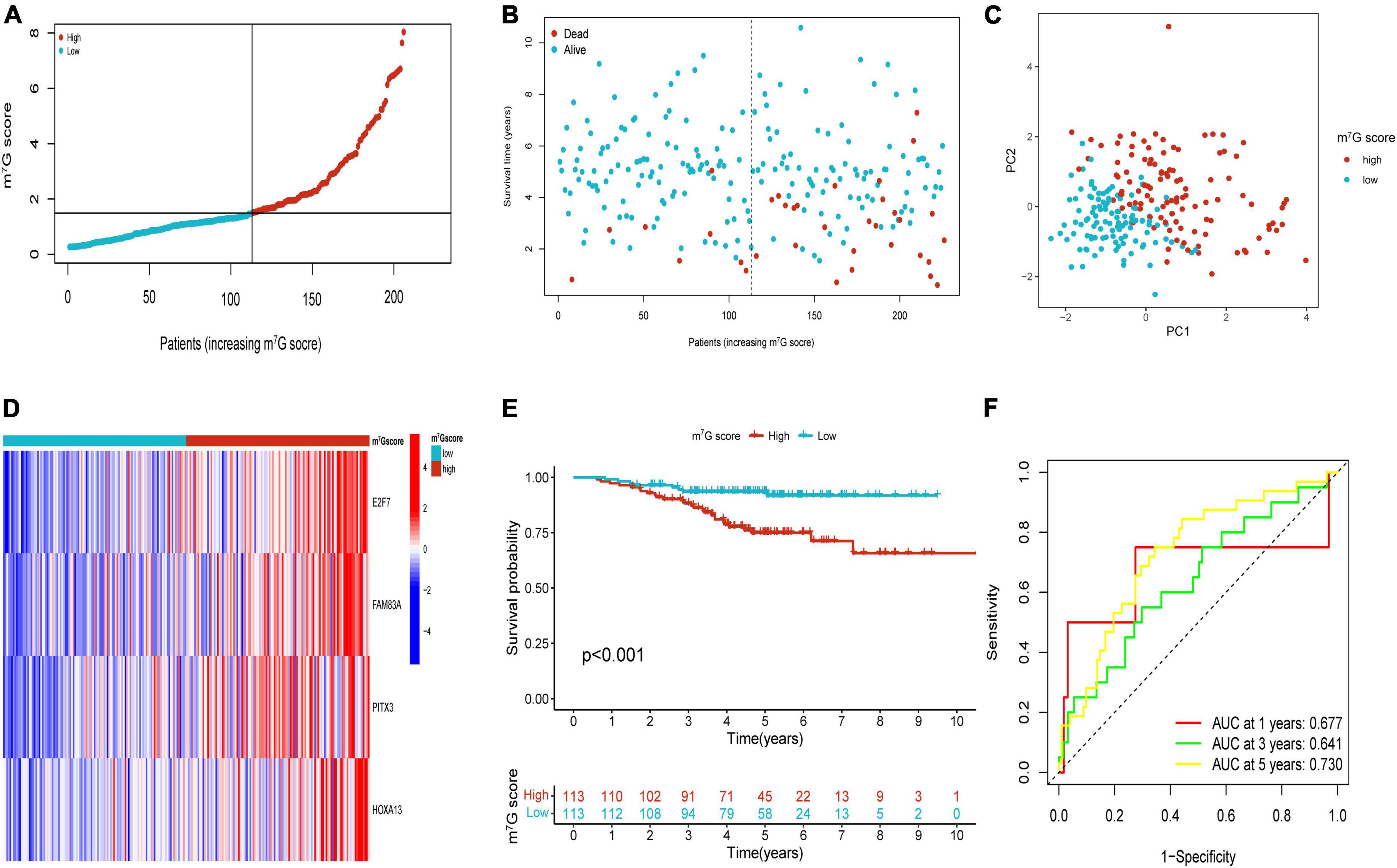
Figure 7. Validation of m7G related signature in GSE31210. (A,B) The distribution of m7G score and survival state. (C) Distribution of patients according to m7G-related signature. (D) Heatmap of four genes expression between two groups. (E) Survival analysis between two groups. (F) AUC for predicting 1-, 3-, 5-years survival rates.
Evaluation of association between m7G score, clinicopathological features, and molecular subtype
The results showed strikingly distinct of m7G score in age, gender, N-stage, M-stage, clinical stage and T-stage (Figures 8A–F) (P < 0.05). We also investigated the relation between m7G score, m7G subtype and survival state by using Sankey diagram (Figure 8G). We found that C2 subtype has a strong correlation with low m7G score, while C1 subtype has a strong correlation with high m7G score. Moreover, the majority of patients with low m7G score were alive, which was consistent with preceding survival analysis. Furthermore, stacked bar chart also presented C1 subtype has a strong correlation with high m7G score (Figure 8H). Similarly, the m7G score was higher in C1 subtype than that in C2 subtype (Figure 8I).
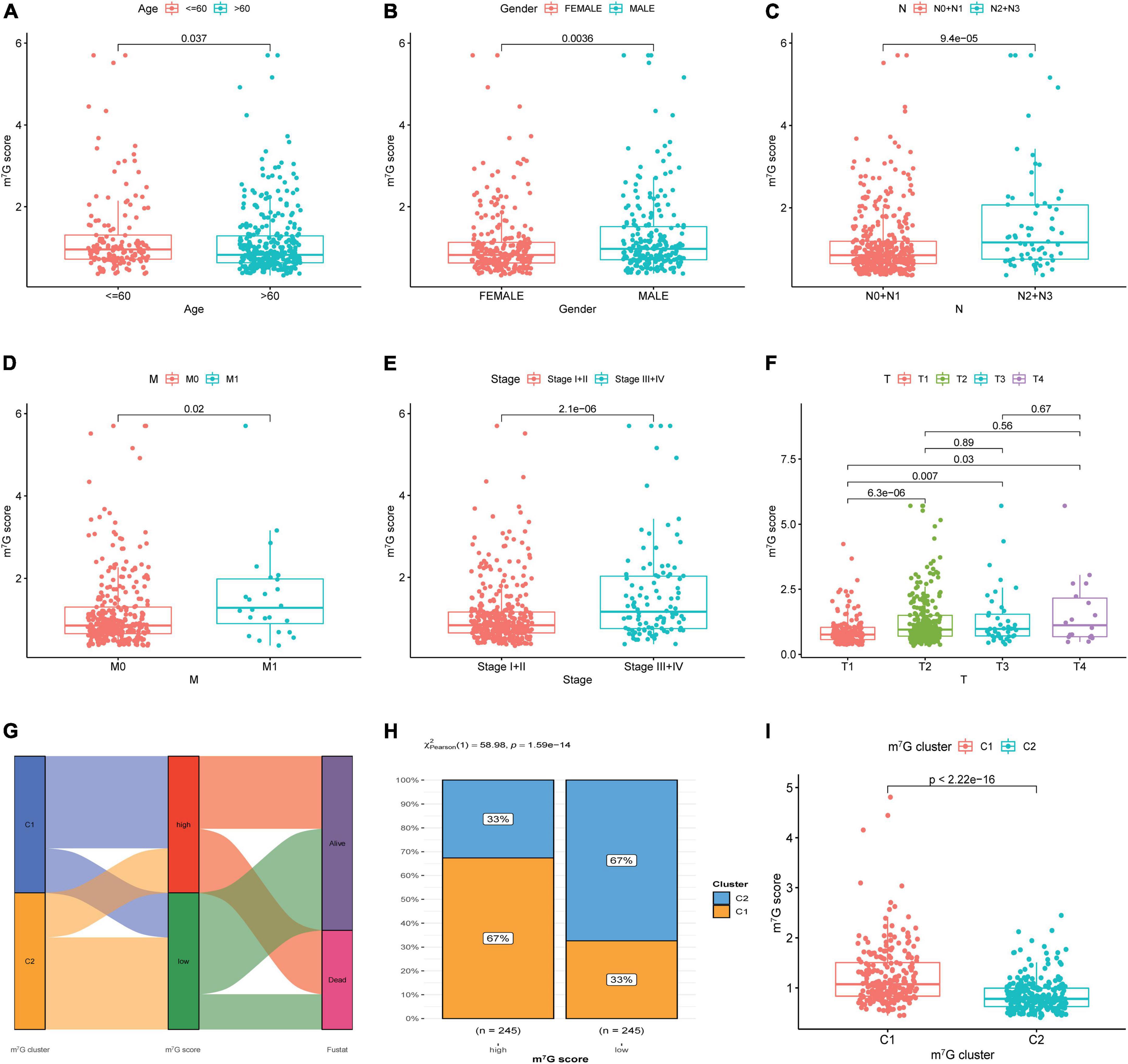
Figure 8. Correlation among clinical features, molecular subtype and m7G score. (A–F) Correlation between clinical features and m7G score. (G) Relation between m7G score, m7G subtype and survival state by using Sankey diagram. (H) Distribution of m7G subtype between high and low m7G score groups. (I) Comparison of m7G score between C1 and C2 subtypes.
Construction of nomogram and comparison of prognostic signatures
Univariate analysis identified that m7G score was related to poor prognosis in LUAD (Figure 9A). Moreover, m7Gs core was still an independent prognostic indicator after using multivariate analysis (Figure 9B) (P < 0.001). Subsequently, we used m7G score and other clinical factors to establish a nomogram (Figure 9C). Calibration curve demonstrated that 1, 3, 5-year predicted survival rates matched the veritable condition (Figure 9D). These evidences revealed that the m7G score could potentially assist clinical practice to evaluate the prognosis of LUAD patients.
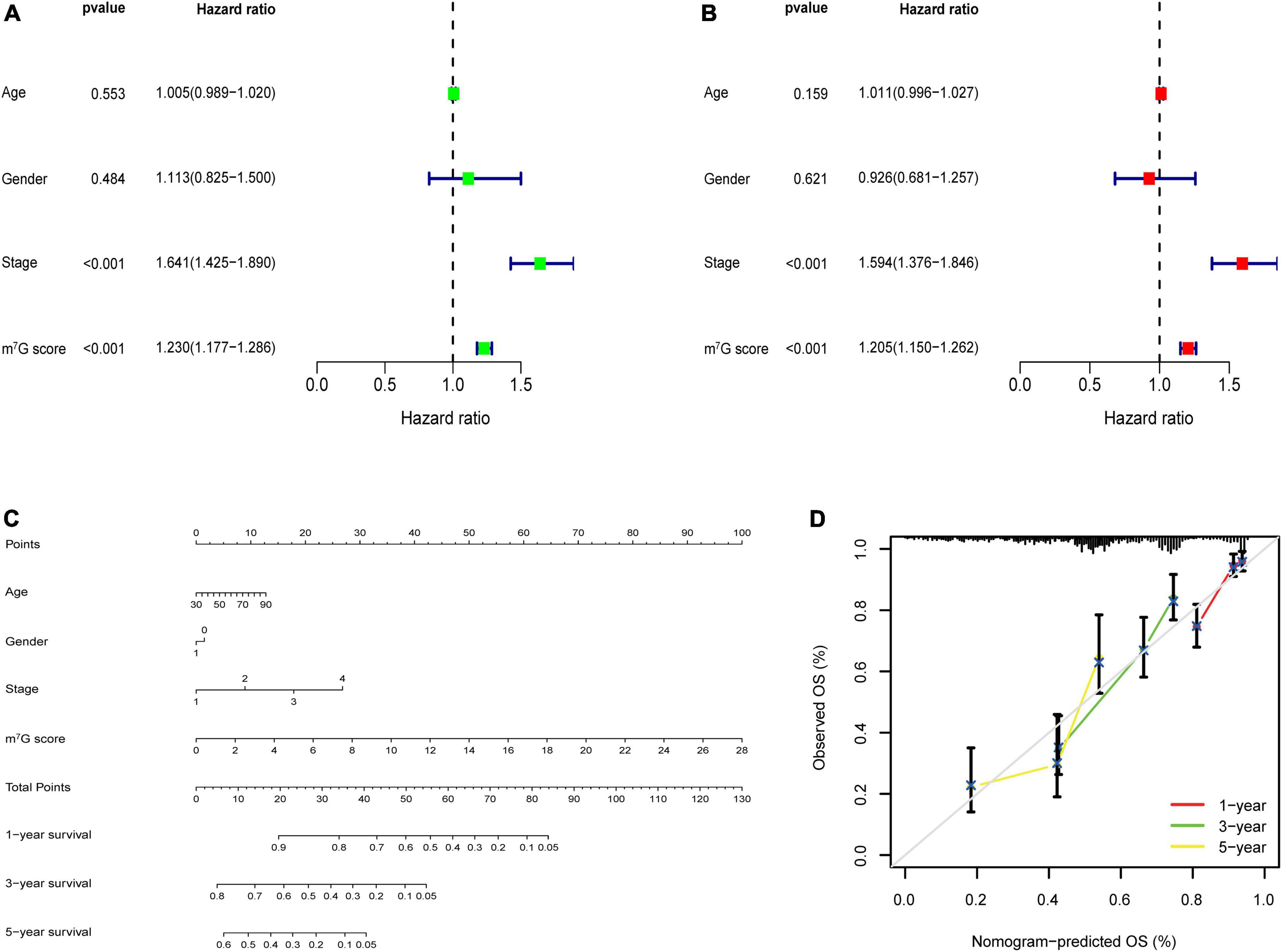
Figure 9. m7G related signature analysis and nomogram construction. (A) Univariate analysis of m7G score and clinical factors. (B) Multivariate analysis of m7G score and clinical factors. (C) Establishment of nomogram. (D) Calibration curve of the nomogram.
After reviewing previous researches, we further compared the m7G related signature with other prognostic models, including 5-gene signature (Wang) (33), 4-gene signature (Wu) (34), 3-gene signature (Yue) (35), and 5-gene signature (Zhai) (36). In order to ensure comparability among models, the risk score of each LUAD sample in entire TCGA cohort was calculated with the same formula according to corresponding genes in four signatures, and then patients were categorized into two groups based on same cut-off value (37). The results of survival analysis showed significant difference in four models (Figures 10A–C,G) (P < 0.05). However, all AUC at 1-, 3-, and 5-years in four models were lower than that corresponding AUC of our prognostic signature (Figures 10D–F,H). Furthermore, we conducted “survcomp” package to calculate the concordance index (C-index) of each signature. The C-index was highest in our prognostic signature (Figure 10I). Therefore, our signature was more efficient to estimate prognosis in LUAD.
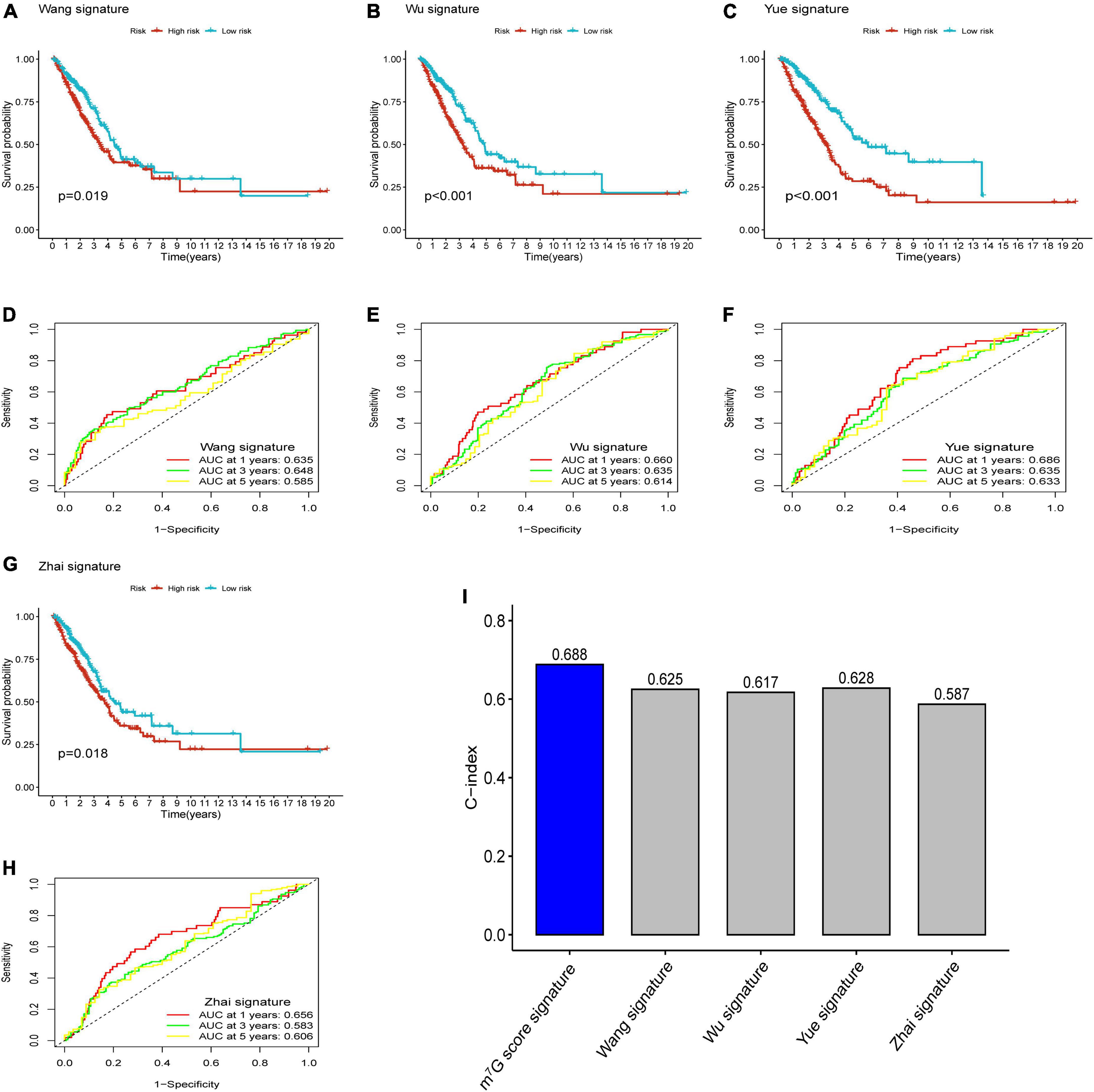
Figure 10. Comparison of prognostic signatures. (A–C,G) Survival analysis of 5-gene signature (Wang), 4-gene signature (Wu), 3-gene signature (Yue) and 5-gene signature (Zhai). (D–F,H) AUC of 5-gene signature (Wang), 4-gene signature (Wu), 3-gene signature (Yue), and 5-gene signature (Zhai) for predicting 1-, 3-, 5-years survival rates. (I) C-index of each signature.
Investigation of immune microenvironment and anti-cancer therapy
Firstly, the results of bubble plot exhibited that CD8+ T cell, common lymphoid progenitor, plasmacytoid dendritic cell, macrophage M1/M0, CD4+ Th1/Th2 cell, neutrophil, cytotoxicity score, NK cell, cancer associated fibroblast, monocyte, Myeloid dendritic cell, and mast cell resting were positively correlated with m7G score (Figure 11A). The ssGSEA displayed that activated CD4 T cell, CD56dim natural killer cell, natural killer T cell, neutrophil, Type-2 T helper cell were more active in high m7G score group (Figure 11B) (P < 0.05). Subsequently, the level of CD274 (PD-L1) and PDCD1 (PD-1) were presented upregulated in high m7G score group (Figures 11C,D) (P < 0.05). Moreover, we compared somatic mutations in two risk groups. The results of mutation map showed remarkably high mutational rate in high m7G score group. TP53 was the highest mutational gene in both groups (Figures 12A,B). We also found that high m7G score group was related to higher TMB score (Figures 12C,D) (P < 0.05). Compared to low m7G score group, high m7G score group had strikingly lower TIDE score (Figure 12E) (P < 0.05).
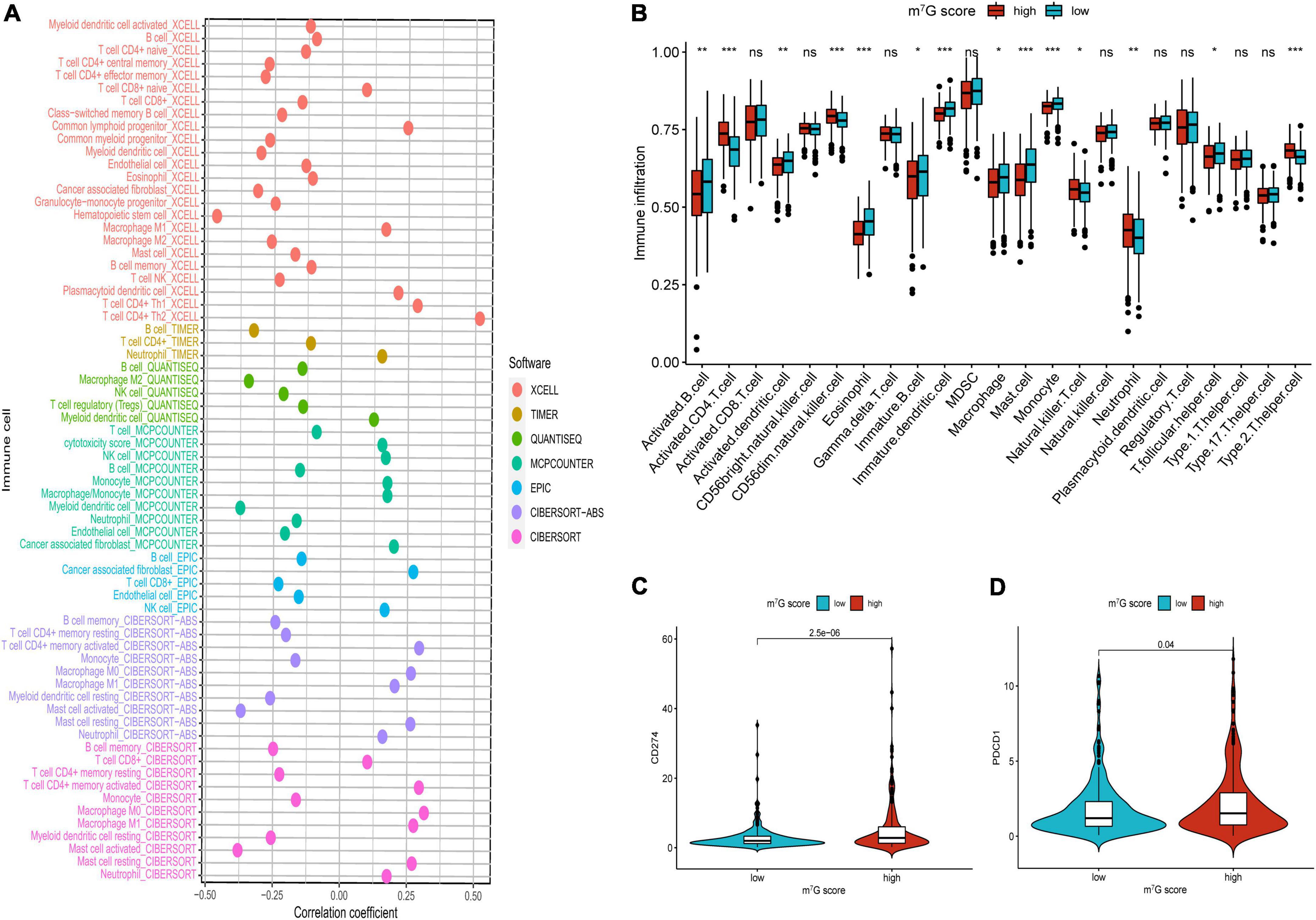
Figure 11. Comparison of immune infiltration and immune checkpoints between high and low m7G score groups. (A) Correlation between immune cell and m7G score. On the right side of the correlation coefficient = 0 indicates a positive correlation with m7G score. (B) Immune infiltration analysis by ssGSEA. (C) Comparison of CD274 (PD-L1). (D) Comparison of PDCD1 (PD-1). *P < 0.05; **P < 0.01; ***P < 0.001.
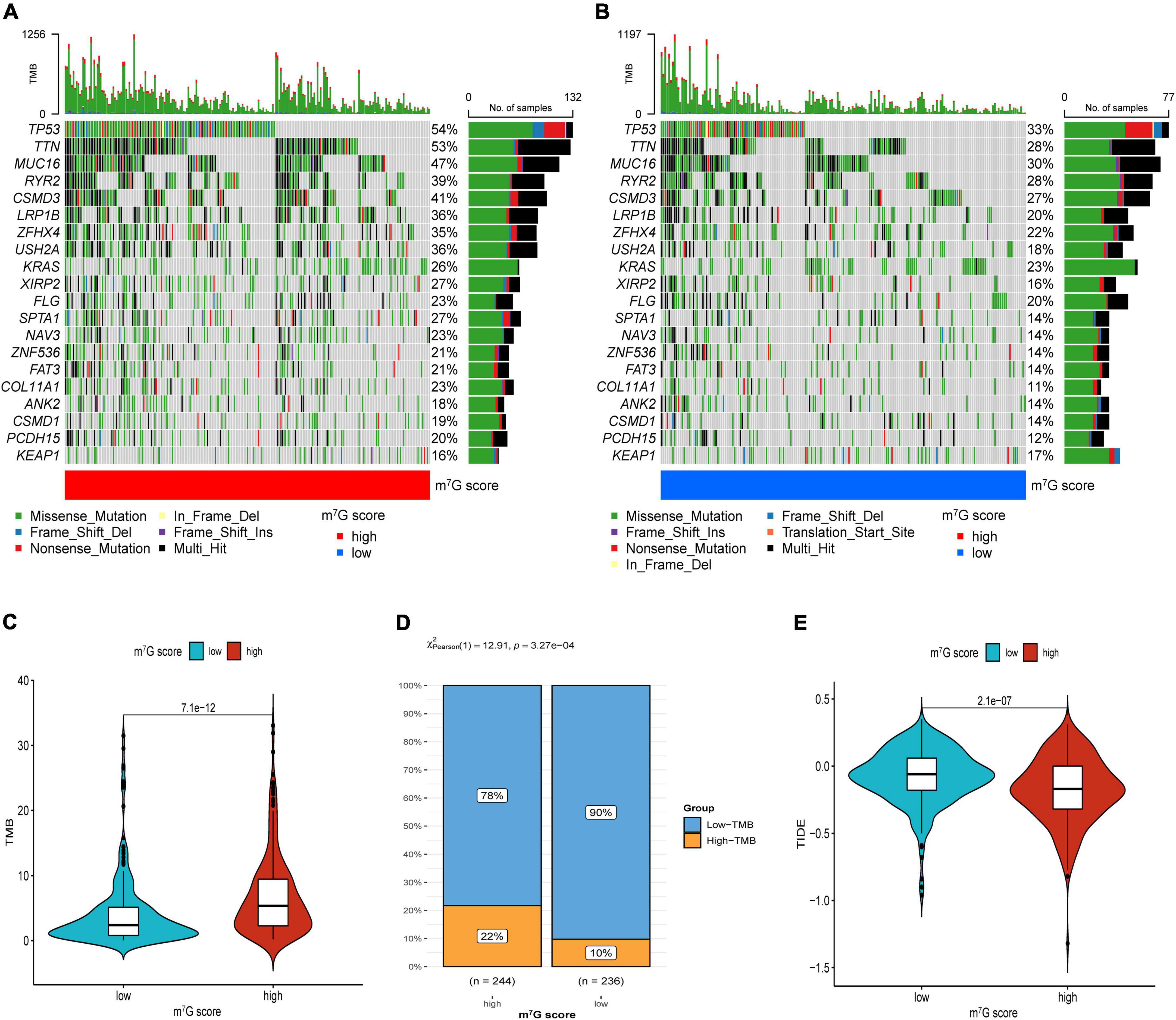
Figure 12. Immunotherapeutic response evaluation in high and low m7G score groups. (A) Mutation plot in high m7G score group. (B) Mutation plot in low m7G score group. (C) Results of TMB between two groups. (D) Distribution of low and high TMB between two subtypes. (E) Results of TIDE between two groups.
We further investigated common drug sensitivity in two groups (Figure 13). Patients presented lower IC50 of Cisplatin, Docetaxel, Doxorubicin, Etoposide, Gemcitabine, Paclitaxel, and Rapamycin in high m7G score group, representing these drugs were more effective for high m7G score group. Meanwhile, IC50 of Bicalutamide, Erlotinib, Axitinib, Imatinib, Metformin, Methotrexate, Bexarotene, Sorafenib, and Temsirolimus were lower in low m7G score group, representing these drugs were more effective for low m7G score group.
Discussion
Recently, the role of m7G in tumors has begun to receive increasing attention. However, there are no reports on studying the molecular subtype correlated with m7G and the implications of m7G related signature on the prognosis and immunotherapy in LUAD. Therefore, we expect to discover more tumor phenotypes through this classification, which could be used to evaluate the prognosis of LUAD patients. Here, we first extracted twenty-nine m7G related regulators expression profiles from TCGA, four of which were demonstrated to have prognostic value. Then, two novel molecular subtypes were identified according to theses prognostic genes. Results showed that patients in C1 subtype had more poor survival outcomes and advanced tumor stages compared to C2 subtype through survival analysis and clinicopathological features comparison, indicating m7G regulators correlated with prognosis and progression of LUAD. And the two subtypes presented markedly different molecular features. Compared with C2 subtype, m7G regulators were more activated in C1 subtype, including LARP1, WDR4, and NCBP1, while only EIF4E3 was activated in C2 subtype. Xu et al. (38) demonstrated that the expression level of LARP1 was upregulated in NSCLC, which positively related to poor prognosis and progression of cancer. A study of WDR4 uncovered that knockdown of WDR4 could restrain the aggressiveness of NSCLC cells, demonstrating that WDR4 may have tumorigenic function in lung cancer (18). NCBP1 was significantly overexpressed in LUAD, combined with CUL4B, which promoted the proliferation, migration and invasion of tumor cells (39). It was reported that compared with patients with lower expression of EIF4E3, patients with high expression of EIF4E3 had markedly better survival rates in various cancers, including LUAD (40). These results are consistent with our study, indicating LUAD patients with C1 subtype have poor prognosis compared to C2 subtype. Furthermore, we investigated the possible functional mechanisms in both two subtypes by using GSEA analysis. Interestingly, the functional pathways enriched in C1 subtype were mainly cell proliferation-related pathways, which may indicate advanced clinicopathological staging, adverse survival outcomes and aggressive tumor subtypes. This evidence also suggested the worse prognosis of C1 subtype. Immune cells as an important part of the tumor microenvironment intensively relate to the response to immunotherapy (41). Recently studies showed that RNA modifications were correlated with the differentiation of immune cells in the tumor microenvironment (42). As one of the RNA modifications, m7G also influenced immune cells in the tumor microenvironment. Chen et al. (43) quantified the tumor-infiltrating lymphocytes and found that CD4+ T exhaustion and Tregs decline after knockout of m7G regulators. Besides, Devarkar et al. (44) presented that m7G was involved in innate immunity mediated by RIG-I. Therefore, the immune score was applied to characterize immune microenvironmental landscapes in two subtypes. Then we found that follicular helper T cells, resting NK cells and activated mast cells were increased in C1 subtype. It was reported that follicular helper T cells could recruit CD8+ T cells to enhance antitumor immune response (45). The resting NK cells could secrete various cytokines to kill target cells, for example tumor cells (46). The TLR4 activation by mast cells resulted in the secretion of CXCL10, which could recruit effector T cells to influence antitumor immune response (47). These evidences indicated that C1 subtype with activated m7G regulators may more sensitive to immunotherapy compared to C2 subtype. Increasing investigations suggested that TMB is a biomarker of response to immunotherapy and is positively relate to the effectiveness of ICB in various cancers, including NSCLC (48). In this study, the TMB score in patients with C1 subtype was distinctly higher compared with C2 subtype. After dividing TMB into high and low groups, more percentage of high TMB was observed in patients with C1 subtype compared with C2 subtype. Consequently, patients with C1 subtype may present better immunotherapeutic response than C2 subtype. Besides, by using an immunotherapy cohort of lung cancer, we also demonstrated that patients with C1 subtype had better immunotherapy efficacy than C2 subtype.
Considering the individual heterogeneity of m7G modification, we utilized a novel m7G score to quantify the m7G modification patterns in LUAD. In the TCGA train cohort, we identified four key genes (E2F7, FAM83A, HOXA13, and PITX3), and then calculated the m7G score through the previously mentioned algorithm. After separating patients into high and low m7G score groups, we found that the four genes were overexpressed in the high m7G score group. It was reported that overexpression of E2F7 correlated with poor prognosis and microRNA-935 could inhibit tumor metastasis and invasion by targeted suppression the level of E2F7 in NSCLC (49). Wang et al. (50) found that activating the expression of E2F7 expression by targeting microRNA-140-3p could promote the progression of LUAD. Studies presented that FAM83A was significantly related to TMB and DNA damage response pathways in NCSLC (51, 52), indicating that it may play an important part in tumor progression and immunotherapy. Hu et al. (53) demonstrated that the expression of FAM83A regulated the proliferation and invasiveness of NSCLC through PI3K/Akt/mTOR pathway. Investigations showed HOXA13, as a nuclear transcription factor, was related to tumor cells proliferation and differentiation, which could accelerate tumor aggressive characteristics through disturbing P53 and Wnt/β-catenin signaling pathways in NSCLC (54). One research reported that HOXA13 was markedly upregulated and strongly correlated with tumorigenesis and progression in LUAD (55). Some studies demonstrated that PITX3 as a transcription factor was involved in many tumors (56, 57). Zhang et al. (58) presented that high expression of PITX3 was strongly associated with the poor prognosis in LUAD. According to these evidences, we indicated that patients with high m7G score in which these four genes were activated, had poor survival. Also, survival analysis demonstrated that the high m7G score group presented worse survival outcomes. ROC curves further showed the great efficacy of m7G score to predict survival rate. And, the TCGA test cohort, entire cohort and GSE31210 cohort were applied to validate the accuracy and reliability of the m7G related signature. Similar results were acquired from all validation cohorts, demonstrating that the prognostic signature may be a robust biomarker to evaluate the prognosis in LUAD. Patients with high m7G score also presented remarkably poor survival condition among different clinical subgroups. After analyzing the association between m7G score and clinicopathological parameters, we observed that m7G score was significantly high in N2 + N3, M1, Stage III + IV and T2-T4, suggesting high m7G score is associate with cancer progression. The characterization of m7G modification patterns showed C2 subtype had lower m7G score compared with C1 subtype. And, the high m7G score correlated with poor survival and cancer progression was consistent with characteristics of C1 subtype. Univariate and multivariate analysis presented that the m7G score was an independent prognosis predictor of LUAD patients. Subsequently, the nomogram also presented high accuracy in predicting survival rate of 1, 3, 5-years. In recent years, many signatures were built to predict the prognosis of LUAD patients. Furthermore, we presented that the AUC area and C-index of our signature were higher than the other four public prognostic signatures, suggesting our signature have better performance in predicting clinical prognosis in LUAD patients.
Currently, although the immunotherapy of lung cancer has got great progress, how to choose the appropriate therapeutic regime for patients is still a clinical challenge. Besides, a part of patients did not obtain effective benefits from immunotherapy (59), and even some patients will undergo obvious side effects during therapy (60). Therefore, it is critical to explore a novel method to guide individualized and precise treatment in LUAD patients. The results of various evaluation methods of immune cell infiltration showed distinct activation of immune cells in both groups, which were similar to molecular subtypes. We speculated there was different immunotherapeutic response in two groups, so we further investigated the association between m7G score groups, immune checkpoint, TMB and TIDE. The immune checkpoints are also an integral part of the immune system and participate in regulating immune escape (61). In recent years, immunotherapy targeting immune checkpoints has obtained huge clinical therapeutic results, especially anti-PD-1/PD-L1 antibody (62). In the study, we observed that patients with high m7G score had upregulated PD-1/PD-L1, indicating that these patients may be more sensitive to ICB than low m7G score. Subsequently, compared with the low m7G score group, the high m7G score group had markedly higher TMB which was consistent with the C1 subtype. In addition, we found that compared with the patients with low m7G score, patients with high m7G score had more percentage of TP53 mutation. Studies showed that TP53 mutation was remarkably related to high PD-L1 expression and patients with TP53 mutation could acquire benefits from ICB therapy in LUAD (63, 64). Investigations presented TIDE was an accurate biomarker used to predict the immunotherapeutic effects of NSCLC, which was negatively associated with the efficacy of ICB (31). Meanwhile, recent studies have reported the clinical application of TIDE in predicting and evaluating immunotherapeutic response (65, 66). In our study, compared with patients with low m7G score patients with high m7G score had lower TIDE score, suggesting patients in the high m7G score group may obtain clinical benefits from immunotherapy. Integration analysis of the m7G score, immune cell infiltration, immune checkpoint, TMB, and TIDE indicated that the signature is a potential biomarker to assess immunotherapeutic response and tailor individualized treatment for LUAD patients.
Chemotherapy is a classic treatment for lung cancer, but patients have different response rates to chemotherapy drugs. Selecting an appropriate chemotherapy regimen is helpful to improve the prognosis and reduce the economic burden of patients. Our study revealed that common drugs including Cisplatin, Docetaxel, Doxorubicin, Etoposide, Gemcitabine, Paclitaxel, and Rapamycin were suitable for patients with high m7G score, while Axitinib, Bexarotene, Bicalutamide, Erlotinib, Imatinib, Metformin, Methotrexate, Sorafenib, and Temsirolimus were more appropriate for patients with low m7G score.
Our research may assist in judging the prognosis in LUAD, but there are also some limitations. First, the research is a retrospective study according to the data from TCGA and GEO datasets, so it’s crucial to collect prospective clinical data to further verify the signature. Second, the potential functional mechanisms of m7G score are not fully verified, so these need to be further verified by experiments at the molecular level in vivo and in vitro. Finally, the drug response in patients is based on methodological prediction, so clinical trials need to be implemented in the future.
Conclusion
In summary, we identified two novel molecular subtypes of LUAD according to m7G regulators. The survival, immune infiltration, and TMB are significantly different in two subtypes. The m7G related signature to quantify the heterogeneity of the two subtypes was constructed. The signature can be employed to predict prognosis in LUAD, then the internal and external cohort were applied to verify the prognostic value. And the signature was elucidated be helpful to guide immunotherapy and chemotherapy. Therefore, this research provides a new direction for improving prognosis and current anti-cancer strategies in LUAD.
Data availability statement
The datasets presented in this study can be found in online repositories. The names of the repository/repositories and accession number(s) can be found in the article/Supplementary material.
Ethics statement
Ethical review and approval was not required for the study on human participants in accordance with the local legislation and institutional requirements. Written informed consent for participation was not required for this study in accordance with the national legislation and the institutional requirements.
Author contributions
ZL and XY conceived and designed the study. ZL and WW performed the data analysis. ZL wrote the manuscript. WW and JW participated in collecting the data and helped draft the manuscript. XY and WW prepared and edited the manuscript. All authors reviewed and approved the manuscript.
Funding
This study was supported by the National Natural Science Foundation of China (Grant No. 81660493) and the Natural Science Foundation of Jiangxi Province (Grant No. 20202ACBL206019).
Conflict of interest
The authors declare that the research was conducted in the absence of any commercial or financial relationships that could be construed as a potential conflict of interest.
Publisher’s note
All claims expressed in this article are solely those of the authors and do not necessarily represent those of their affiliated organizations, or those of the publisher, the editors and the reviewers. Any product that may be evaluated in this article, or claim that may be made by its manufacturer, is not guaranteed or endorsed by the publisher.
Supplementary material
The Supplementary Material for this article can be found online at: https://www.frontiersin.org/articles/10.3389/fmed.2022.962972/full#supplementary-material
Supplementary Figure 1 | Survival analysis among different clinical subgroups.
Supplementary Table 1 | Three m7G-related gene sets.
Supplementary Table 2 | 201 prognostic genes through univariate Cox analysis.
Abbreviations
LUAD, lung adenocarcinoma; m7G, N7-methylguanosine; TCGA, The Cancer Genome Atlas; TMB, tumor mutational burden; DEGs, differentially expressed genes; NSCLC, non-small cell lung cancer; ICB, immune checkpoint blockade; GEO, Gene Expression Omnibus; PCA, principal component analysis; GSEA, Gene Set Enrichment Analysis; FDR, false discovery rate; LASSO, least absolute shrinkage and selection operator; ROC, receiver operating characteristics; ssGSEA, single-sample gene set enrichment analysis; TIDE, tumor immune dysfunction and exclusion; IC50, half-maximal inhibitory concentration; CDF, cumulative distribution function; AUC, areas under the curves; C-index, concordance index.
Footnotes
- ^ https://portal.gdc.cancer.gov/
- ^ https://www.ncbi.nlm.nih.gov/geo/
- ^ http://www.gsea-msigdb.org/gsea/index.jsp
- ^ http://tide.dfci.harvard.edu/
References
1. Sharma A, Shepard JO. Lung cancer biopsies. Radiol Clin North Am. (2018) 56:377–90. doi: 10.1016/j.rcl.2018.01.001
2. Song Q, Shang J, Zhang C, Zhang L, Wu X. Impact of the homogeneous and heterogeneous risk factors on the incidence and survival outcome of bone metastasis in NSCLC patients. J Cancer Res Clin Oncol. (2019) 145:737–46. doi: 10.1007/s00432-018-02826-7
3. Lin JJ, Cardarella S, Lydon CA, Dahlberg SE, Jackman DM, Janne PA, et al. Five-year survival in Egfr-mutant metastatic lung adenocarcinoma treated with Egfr-Tkis. J Thorac Oncol. (2016) 11:556–65. doi: 10.1016/j.jtho.2015.12.103
4. Duma N, Santana-Davila R, Molina JR. Non-small cell lung cancer: Epidemiology, screening, diagnosis, and treatment. Mayo Clin Proc. (2019) 94:1623–40. doi: 10.1016/j.mayocp.2019.01.013
5. Zou W, Wolchok JD, Chen L. Pd-L1 (B7-H1) and Pd-1 pathway blockade for cancer therapy: Mechanisms, response biomarkers, and combinations. Sci Transl Med. (2016) 8:328rv4. doi: 10.1126/scitranslmed.aad7118
6. Syn NL, Teng MWL, Mok TSK, Soo RA. De-novo and acquired resistance to immune checkpoint targeting. Lancet Oncol. (2017) 18:e731–41. doi: 10.1016/s1470-2045(17)30607-1
7. Mehta A, Dobersch S, Romero-Olmedo AJ, Barreto G. Epigenetics in lung cancer diagnosis and therapy. Cancer Metastasis Rev. (2015) 34:229–41. doi: 10.1007/s10555-015-9563-3
8. Sun X, Yi J, Yang J, Han Y, Qian X, Liu Y, et al. An integrated epigenomic-transcriptomic landscape of lung cancer reveals novel methylation driver genes of diagnostic and therapeutic relevance. Theranostics. (2021) 11:5346–64. doi: 10.7150/thno.58385
9. Boccaletto P, Machnicka MA, Purta E, Piątkowski P, Bagiński B, Wirecki TK, et al. Modomics: A database of rna modification pathways. 2017 Update. Nucleic Acids Res. (2018) 46:D303–7. doi: 10.1093/nar/gkx1030
10. Han X, Wang M, Zhao YL, Yang Y, Yang YG. Rna methylations in human cancers. Semin Cancer Biol. (2021) 75:97–115. doi: 10.1016/j.semcancer.2020.11.007
11. Cheng Y, Wang M, Zhou J, Dong H, Wang S, Xu H. The important role of N6-methyladenosine Rna modification in non-small cell lung cancer. Genes (Basel). (2021) 12:440. doi: 10.3390/genes12030440
12. Zhao LY, Song J, Liu Y, Song CX, Yi C. Mapping the epigenetic modifications of DNA and Rna. Protein Cell. (2020) 11:792–808. doi: 10.1007/s13238-020-00733-7
13. Cowling VH. Regulation of Mrna Cap Methylation. Biochem J. (2009) 425:295–302. doi: 10.1042/BJ20091352
14. Zhang LS, Liu C, Ma H, Dai Q, Sun HL, Luo G, et al. Transcriptome-wide mapping of internal N(7)-methylguanosine methylome in mammalian Mrna. Mol Cell. (2019) 74:1304–16.e8. doi: 10.1016/j.molcel.2019.03.036
15. Malbec L, Zhang T, Chen YS, Zhang Y, Sun BF, Shi BY, et al. Dynamic methylome of internal Mrna N(7)-Methylguanosine and its regulatory role in translation. Cell Res. (2019) 29:927–41. doi: 10.1038/s41422-019-0230-z
16. Han H, Yang C, Ma J, Zhang S, Zheng S, Ling R, et al. N(7)-Methylguanosine Trna modification promotes esophageal squamous cell carcinoma tumorigenesis via the Rptor/Ulk1/Autophagy axis. Nat Commun. (2022) 13:1478. doi: 10.1038/s41467-022-29125-7
17. D’Abronzo LS, Ghosh PM. Eif4e phosphorylation in prostate cancer. Neoplasia. (2018) 20:563–73. doi: 10.1016/j.neo.2018.04.003
18. Ma J, Han H, Huang Y, Yang C, Zheng S, Cai T, et al. Mettl1/Wdr4-Mediated M(7)G Trna modifications and M(7)G codon usage promote Mrna translation and lung cancer progression. Mol Ther. (2021) 29:3422–35. doi: 10.1016/j.ymthe.2021.08.005
19. Zhang M, Song J, Yuan W, Zhang W, Sun Z. Roles of Rna methylation on tumor immunity and clinical implications. Front Immunol. (2021) 12:641507. doi: 10.3389/fimmu.2021.641507
20. Yu P, Tong L, Song Y, Qu H, Chen Y. Systematic profiling of invasion-related gene signature predicts prognostic features of lung adenocarcinoma. J Cell Mol Med. (2021) 25:6388–402. doi: 10.1111/jcmm.16619
21. Li B, Huang Z, Yu W, Liu S, Zhang J, Wang Q, et al. Molecular subtypes based on Cnvs related gene signatures identify candidate prognostic biomarkers in lung adenocarcinoma. Neoplasia. (2021) 23:704–17. doi: 10.1016/j.neo.2021.05.006
22. Xu D, Li C, Zhang Y, Zhang J. DNA methylation molecular subtypes for prognosis prediction in lung adenocarcinoma. BMC Pulm Med. (2022) 22:133. doi: 10.1186/s12890-022-01924-0
23. Tomikawa C. 7-Methylguanosine modifications in transfer Rna (Trna). Int J Mol Sci. (2018) 19:4080. doi: 10.3390/ijms19124080
24. Wilkerson MD, Hayes DN. Consensusclusterplus: A class discovery tool with confidence assessments and item tracking. Bioinformatics. (2010) 26:1572–3. doi: 10.1093/bioinformatics/btq170
25. Newman AM, Liu CL, Green MR, Gentles AJ, Feng W, Xu Y, et al. Robust enumeration of cell subsets from tissue expression profiles. Nat Methods. (2015) 12:453–7. doi: 10.1038/nmeth.3337
26. Chan TA, Yarchoan M, Jaffee E, Swanton C, Quezada SA, Stenzinger A, et al. Development of tumor mutation burden as an immunotherapy biomarker: Utility for the oncology clinic. Ann Oncol Off J Eur Soc Med Oncol. (2019) 30:44–56. doi: 10.1093/annonc/mdy495
27. Reck M, Schenker M, Lee KH, Provencio M, Nishio M, Lesniewski-Kmak K, et al. Nivolumab plus ipilimumab versus chemotherapy as first-line treatment in advanced non-small-cell lung cancer with high tumour mutational burden: Patient-reported outcomes results from the randomised, open-label, Phase III checkmate 227 trial. Eur J Cancer. (2019) 116:137–47. doi: 10.1016/j.ejca.2019.05.008
28. Mo Z, Cao Z, Luo S, Chen Y, Zhang S. Novel molecular subtypes associated with 5mc methylation and their role in hepatocellular carcinoma immunotherapy. Front Mol Biosci. (2020) 7:562441. doi: 10.3389/fmolb.2020.562441
29. Sturm G, Finotello F, Petitprez F, Zhang JD, Baumbach J, Fridman WH, et al. Comprehensive evaluation of transcriptome-based cell-type quantification methods for immuno-oncology. Bioinformatics. (2019) 35:i436–45. doi: 10.1093/bioinformatics/btz363
30. Charoentong P, Finotello F, Angelova M, Mayer C, Efremova M, Rieder D, et al. Pan-cancer immunogenomic analyses reveal genotype-immunophenotype relationships and predictors of response to checkpoint blockade. Cell Rep. (2017) 18:248–62. doi: 10.1016/j.celrep.2016.12.019
31. Jiang P, Gu S, Pan D, Fu J, Sahu A, Hu X, et al. Signatures of T cell dysfunction and exclusion predict cancer immunotherapy response. Nat Med. (2018) 24:1550–8. doi: 10.1038/s41591-018-0136-1
32. Geeleher P, Cox N, Huang RS. Prrophetic: An R package for prediction of clinical chemotherapeutic response from tumor gene expression levels. PLoS One. (2014) 9:e107468. doi: 10.1371/journal.pone.0107468
33. Wang Z, Embaye KS, Yang Q, Qin L, Zhang C, Liu L, et al. Development and validation of a novel epigenetic-related prognostic signature and candidate drugs for patients with lung adenocarcinoma. Aging. (2021) 13:18701–17. doi: 10.18632/aging.203315
34. Wu Y, Yang L, Zhang L, Zheng X, Xu H, Wang K, et al. Identification of a four-gene signature associated with the prognosis prediction of lung adenocarcinoma based on integrated bioinformatics analysis. Genes (Basel). (2022) 13:238. doi: 10.3390/genes13020238
35. Yue C, Ma H, Zhou Y. Identification of prognostic gene signature associated with microenvironment of lung adenocarcinoma. PeerJ. (2019) 7:e8128. doi: 10.7717/peerj.8128
36. Zhai WY, Duan FF, Chen S, Wang JY, Lin YB, Wang YZ, et al. A novel inflammatory-related gene signature based model for risk stratification and prognosis prediction in lung adenocarcinoma. Front Genet. (2021) 12:798131. doi: 10.3389/fgene.2021.798131
37. Liang Y, Su Q, Wu X. Identification and validation of a novel six-gene prognostic signature of stem cell characteristic in colon cancer. Front Oncol. (2020) 10:571655. doi: 10.3389/fonc.2020.571655
38. Xu Z, Xu J, Lu H, Lin B, Cai S, Guo J, et al. Larp1 is regulated by the Xist/Mir-374a axis and functions as an oncogene in non-small cell lung carcinoma. Oncol Rep. (2017) 38:3659–67. doi: 10.3892/or.2017.6040
39. Zhang H, Wang A, Tan Y, Wang S, Ma Q, Chen X, et al. Ncbp1 promotes the development of lung adenocarcinoma through up-regulation of Cul4b. J Cell Mol Med. (2019) 23:6965–77. doi: 10.1111/jcmm.14581
40. Wu S, Wagner G. Deep computational analysis details dysregulation of eukaryotic translation initiation complex Eif4f in human cancers. Cell Syst. (2021) 12:907–23.e6. doi: 10.1016/j.cels.2021.07.002
41. Zhang Y, Zhang Z. The history and advances in cancer immunotherapy: Understanding the characteristics of tumor-infiltrating immune cells and their therapeutic implications. Cell Mol Immunol. (2020) 17:807–21. doi: 10.1038/s41423-020-0488-6
42. Mehdi A, Rabbani SA. Role of methylation in pro- and anti-cancer immunity. Cancers (Basel). (2021) 13:545. doi: 10.3390/cancers13030545
43. Chen J, Li K, Chen J, Wang X, Ling R, Cheng M, et al. Aberrant translation regulated by Mettl1/Wdr4-Mediated Trna N7-methylguanosine modification drives head and neck squamous cell carcinoma progression. Cancer Commun (Lond). (2022) 42:223–44. doi: 10.1002/cac2.12273
44. Devarkar SC, Wang C, Miller MT, Ramanathan A, Jiang F, Khan AG, et al. Structural basis for M7g recognition and 2’-O-Methyl discrimination in capped Rnas by the innate immune receptor Rig-I. Proc Natl Acad Sci U S A. (2016) 113:596–601. doi: 10.1073/pnas.1515152113
45. Cui C, Wang J, Fagerberg E, Chen PM, Connolly KA, Damo M, et al. Neoantigen-driven B cell and Cd4 t follicular helper cell collaboration promotes anti-tumor Cd8 T cell responses. Cell. (2021) 184:6101–18.e13. doi: 10.1016/j.cell.2021.11.007
46. Bryceson YT, March ME, Ljunggren HG, Long EO. Synergy among receptors on resting Nk cells for the activation of natural cytotoxicity and cytokine secretion. Blood. (2006) 107:159–66. doi: 10.1182/blood-2005-04-1351
47. Kaesler S, Wolbing F, Kempf WE, Skabytska Y, Koberle M, Volz T, et al. Targeting Tumor-resident mast cells for effective anti-melanoma immune responses. JCI Insight. (2019) 4:e125057. doi: 10.1172/jci.insight.125057
48. Goodman AM, Kato S, Bazhenova L, Patel SP, Frampton GM, Miller V, et al. Tumor mutational burden as an independent predictor of response to immunotherapy in diverse cancers. Mol Cancer Ther. (2017) 16:2598–608. doi: 10.1158/1535-7163.MCT-17-0386
49. Wang C, Li S, Xu J, Niu W, Li S. Microrna-935 is reduced in non-small cell lung cancer tissue, is linked to poor outcome, and acts on signal transduction mediator E2f7 and the Akt pathway. Br J Biomed Sci. (2019) 76:17–23. doi: 10.1080/09674845.2018.1520066
50. Wang Y, Wo Y, Lu T, Sun X, Liu A, Dong Y, et al. Circ-aasdh functions as the progression of early stage lung adenocarcinoma by targeting Mir-140-3p to Activate E2f7 expression. Transl Lung Cancer Res. (2021) 10:57–70. doi: 10.21037/tlcr-20-1062
51. Ren J, Yang Y, Li C, Xie L, Hu R, Qin X, et al. A novel prognostic model of early-stage lung adenocarcinoma integrating methylation and immune biomarkers. Front Genet. (2020) 11:634634. doi: 10.3389/fgene.2020.634634
52. Luo R, Song J, Xiao X, Xie Z, Zhao Z, Zhang W, et al. Identifying Cpg methylation signature as a promising biomarker for recurrence and immunotherapy in non-small-cell lung carcinoma. Aging. (2020) 12:14649–76. doi: 10.18632/aging.103517
53. Hu H, Wang F, Wang M, Liu Y, Wu H, Chen X, et al. Fam83a is amplified and promotes tumorigenicity in non-small cell lung cancer via Erk and Pi3k/Akt/Mtor pathways. Int J Med Sci. (2020) 17:807–14. doi: 10.7150/ijms.33992
54. Wang Y, He B, Dong Y, He GJ, Qi XW, Li Y, et al. Homeobox-A13 acts as a functional prognostic and diagnostic biomarker via regulating P53 and Wnt signaling pathways in lung cancer. Cancer Biomark. (2021) 31:239–54. doi: 10.3233/CBM-200540
55. Deng Y, He R, Zhang R, Gan B, Zhang Y, Chen G, et al. The expression of Hoxa13 in lung adenocarcinoma and its clinical significance: A study based on the cancer genome atlas, oncomine and reverse transcription-quantitative polymerase chain reaction. Oncol Lett. (2018) 15:8556–72. doi: 10.3892/ol.2018.8381
56. Holmes EE, Goltz D, Sailer V, Jung M, Meller S, Uhl B, et al. Pitx3 promoter methylation is a prognostic biomarker for biochemical recurrence-free survival in prostate cancer patients after radical prostatectomy. Clin Epigenet. (2016) 8:104. doi: 10.1186/s13148-016-0270-x
57. Sailer V, Holmes EE, Gevensleben H, Goltz D, Droge F, Franzen A, et al. Pitx3 DNA methylation is an independent predictor of overall survival in patients with head and neck squamous cell carcinoma. Clin Epigenet. (2017) 9:12. doi: 10.1186/s13148-017-0317-7
58. Zhang C, Chen X, Chen Y, Cao M, Tang J, Zhong B, et al. The pitx gene family as potential biomarkers and therapeutic targets in lung adenocarcinoma. Medicine (Baltimore). (2021) 100:e23936. doi: 10.1097/MD.0000000000023936
59. Kurtulus S, Madi A, Escobar G, Klapholz M, Nyman J, Christian E, et al. Checkpoint blockade immunotherapy induces dynamic changes in Pd-1(-)Cd8(+) tumor-infiltrating T cells. Immunity. (2019) 50:181–94.e6. doi: 10.1016/j.immuni.2018.11.014
60. Pauken KE, Dougan M, Rose NR, Lichtman AH, Sharpe AH. Adverse events following cancer immunotherapy: Obstacles and opportunities. Trends Immunol. (2019) 40:511–23. doi: 10.1016/j.it.2019.04.002
61. Ramsay AG. Immune checkpoint blockade immunotherapy to activate anti-tumour T-cell immunity. Br J Haematol. (2013) 162:313–25. doi: 10.1111/bjh.12380
62. Sharpe AH. Introduction to checkpoint inhibitors and cancer immunotherapy. Immunol Rev. (2017) 276:5–8. doi: 10.1111/imr.12531
63. Schoenfeld AJ, Rizvi H, Bandlamudi C, Sauter JL, Travis WD, Rekhtman N, et al. Clinical and molecular correlates of Pd-L1 expression in patients with lung adenocarcinomas. Ann Oncol Off J Eur Soc Med Oncol. (2020) 31:599–608. doi: 10.1016/j.annonc.2020.01.065
64. Dong Z-Y, Zhong W-Z, Liu S-Y, Xie Z, Wu S-P, Wu YL. Ma15.10 potential predictive value of Tp53 and Kras mutation status for response to Pd-1 blockade immunotherapy in lung adenocarcinoma. J Thoracic Oncol. (2017) 12:S432–3. doi: 10.1016/j.jtho.2016.11.504
65. Bretz AC, Parnitzke U, Kronthaler K, Dreker T, Bartz R, Hermann F, et al. Domatinostat favors the immunotherapy response by modulating the tumor immune microenvironment (Time). J Immunother Cancer. (2019) 7:294. doi: 10.1186/s40425-019-0745-3
66. Jin R, Liu C, Zheng S, Wang X, Feng X, Li H, et al. Molecular heterogeneity of Anti-Pd-1/Pd-L1 immunotherapy efficacy is correlated with tumor immune microenvironment in East Asian patients with non-small cell lung cancer. Cancer Biol Med. (2020) 17:768–81. doi: 10.20892/j.issn.2095-3941.2020.0121
Keywords: N7-methylguanosine, lung adenocarcinoma, molecular subtype, prognosis, immunotherapy efficacy
Citation: Li ZH, Wang WJ, Wu J and Ye XQ (2022) Identification of N7-methylguanosine related signature for prognosis and immunotherapy efficacy prediction in lung adenocarcinoma. Front. Med. 9:962972. doi: 10.3389/fmed.2022.962972
Received: 14 June 2022; Accepted: 08 August 2022;
Published: 24 August 2022.
Edited by:
Somchai Chutipongtanate, University of Cincinnati, United StatesReviewed by:
Yongjun Zhang, University of Chinese Academy of Sciences, ChinaBrinda Balasubramanian, Mahidol University, Thailand
Copyright © 2022 Li, Wang, Wu and Ye. This is an open-access article distributed under the terms of the Creative Commons Attribution License (CC BY). The use, distribution or reproduction in other forums is permitted, provided the original author(s) and the copyright owner(s) are credited and that the original publication in this journal is cited, in accordance with accepted academic practice. No use, distribution or reproduction is permitted which does not comply with these terms.
*Correspondence: Xiaoqun Ye, NTExMjAxNjYzQHFxLmNvbQ==
†These authors have contributed equally to this work and share first authorship