- 1Department of Pulmonary and Critical Care Medicine, China-Japan Friendship Hospital, Beijing, China
- 2Clinical Trial Center, National Cancer Center/National Clinical Research Center for Cancer/Cancer Hospital Chinese Academy of Medical Sciences, Peking Union Medical College, Beijing, China
- 3School of Medicine, Tsinghua University, Beijing, China
- 4National Center for Respiratory Medicine, Institute of Respiratory Medicine, National Clinical Research Center for Respiratory Disease, Chinese Academy of Medical Sciences, Beijing, China
- 5Department of Pneumology, Center for Interstitial and Rare Lung Diseases, Ruhrlandklinik, University Hospital, Essen, Germany
- 6Tsinghua University-Peking University Joint Center for Life Sciences, Beijing, China
- 7Peking Union Medical College, Beijing, China
Sarcoidosis is a granulomatous disease of unknown etiology, immunologically characterized by a Th1 immune response. Transcriptome-wide expression studies in various types of sarcoid tissues contributed to better understanding of disease mechanisms. We performed a systematic database search on Gene Expression Omnibus (GEO) and utilized transcriptomic data from blood and sarcoidosis-affected tissues in a meta-analysis to identify a cross-tissue, cross-platform signature. Datasets were further separated into training and testing sets for development of a diagnostic classifier for sarcoidosis. A total of 690 differentially expressed genes were identified in the analysis among various tissues. 29 of the genes were robustly associated with sarcoidosis in the meta-analysis both in blood and in lung-associated tissues. Top genes included LINC01278 (P = 3.11 × 10–13), GBP5 (P = 5.56 × 10–07), and PSMB9 (P = 1.11 × 10–06). Pathway enrichment analysis revealed activated IFN-γ, IL-1, and IL-18, autophagy, and viral infection response. IL-17 was observed to be enriched in peripheral blood specific signature genes. A 16-gene classifier achieved excellent performance in the independent validation data (AUC 0.711–0.964). This study provides a cross-tissue meta-analysis for expression profiles of sarcoidosis and identifies a diagnostic classifier that potentially can complement more invasive procedures.
Introduction
Sarcoidosis is a systemic disorder featured by the presence of non-caseating granuloma. The incidence varies considerably depending on sex, age, ethnicity and geographic regions, indicating that both genetic predisposition, and environmental factors play essential roles in the pathogenesis. The etiology of sarcoidosis remains uncertain despite decades of effort. Multiple genome-wide expression studies have been performed on sarcoidosis in order to understand underlying molecular mechanisms, including directly affected tissues such as lung and skin, fluids in contact with granulomas like bronchoalveolar lavage (BAL), and peripheral blood.
Expression profiles of circulatory blood and sarcoid tissues are quite different, but can be implicated in pathways involved in innate and adaptive immunity, granuloma formation, and fibroproliferation (1–3). Th1 associated molecules, especially INF-γ response transcription factor STAT1 as well as STAT1 regulated chemokines (IL-5, IL-7, IL-15, CCR5, CXCL9, CXCL10, and CXCL11) have been recognized as key inflammatory factors in sarcoidosis in transcriptome-wide analysis of lung, lymph nodes and peripheral blood (4, 5).
Aside from tissue-independent common pathways, genes enriched in IFN signaling (type I and II) and the Th17 pathway, including IL-23, IL-23R, and IL-21, are dysregulated in skin tissue of active cutaneous sarcoidosis (6). Upregulated genes in orbital tissues further validated the role of IFN-γ and type I IFNs, including CXCL10, GBP5, STAT1, AIM2, ICAM1, and JAK2 (7). Enrichment analysis of transcription factor binding sites revealed that interferon-response factor 1 and 2 (IRF-1 and IRF-2), and nuclear factor κB, are involved in the transcriptional modulation in sarcoidosis.
In addition to pathways associated with adaptive immune system and T-cell signaling, differentially expressed genes in BAL identified a novel gene network linkage between immunoproteasome subunits (PSMB-8, −9, −10), and found TWIST1, a biomarker of M1-activation, to be up-regulated in sarcoidosis patients compared to controls (8, 9). Comparison of BAL cells from patients with severe and stable sarcoidosis demonstrated increased expression of protein kinase TYK2 and cell cycle inhibitor p21Waf1/Cip1, as well as reduced expression of Cathelicidin (CAMP), confirming the involvement of Th1 and INF-γ immune responses (10, 11). MMP12 and ADAMDEC1 were newly identified to be significantly associated with sarcoidosis severity in lung tissue and BAL (5).
Peripheral blood has also been extensively examined for sarcoidosis specific gene identification. Whole blood gene expression signature distinguished sarcoidosis from healthy controls with an error rate of 12.1% (12). The genes belonged to Th1-type inflammation, such as INF signaling pathway (IFN, STAT1), and to T-cell homeostasis and survival (IL-15 and IL-7R). A 20 gene signature was identified in peripheral blood mononuclear cells (PBMC) with an accuracy of 86.0% to distinguish healthy subjects from those with sarcoidosis, but performed less well when applied to replication datasets (13). Unlike the prior model, the cohort-specific 20 gene signature was not composed of genes belonging to T-cell, JAK/STAT, or cytokines pathways.
The diagnosis of sarcoidosis relies on histopathologic examination and compatible clinical presentation, with other causes of granulomatous inflammation excluded (14). A definitive diagnosis is challenging because several other diseases can show similar histopathologic changes (15). The prognosis is less favorable among patients with more advanced stage at diagnosis, emphasizing the significance to develop auxiliary approaches to help early diagnosis of such potentially hazardous disease. However, the clinical value of sarcoid tissue-based diagnostic gene markers remains unclear owing to the limitations of obtaining biopsy samples.
In this study, a full meta-analysis was performed utilizing all available genome-wide expression datasets for sarcoidosis vs. healthy subjects from public database to explore robust gene markers across different tissues and to propose an expression-oriented diagnostic panel. To our knowledge, this is to date the largest cross-tissue transcriptomic meta-analysis of sarcoidosis.
Materials and methods
Dataset identification
A systematic database search was performed on Gene Expression Omnibus (GEO). A total of 1,757 records were found with sarcoidosis as search term and organism confined to Homo sapiens (Feb 5 2021). We excluded 1,712 duplicated and 7 irrelevant results. Nine cell lines were excluded since they do not depict transcriptional features and functions in vivo. 3 methylation datasets, 1 single-cell dataset, 3 non-coding RNA datasets, 1 dataset with less than two sarcoidosis subjects, and 6 datasets without compatible control were subsequently excluded. Two array-based studies without transcriptome-wide data were removed, and 13 datasets were finally included in our meta-analysis (Figure 1). We checked definition of sarcoidosis and control in each of the included study. Diagnosis of sarcoidosis was made by a sarcoidosis specialist, biopsy evidence, compatible clinical, and radiological findings according to the WASOG guidelines (15) in GSE19314, GSE42834, GSE16538, and GSE37912. Similar diagnosis criteria were applied in GSE83456 and GSE75023. The other studies used pathology-confirmed biopsy displaying typical non-necrotizing epithelioid granuloma as definition. Controls were defined as recruited healthy volunteers or disease-uninvolved tissues.
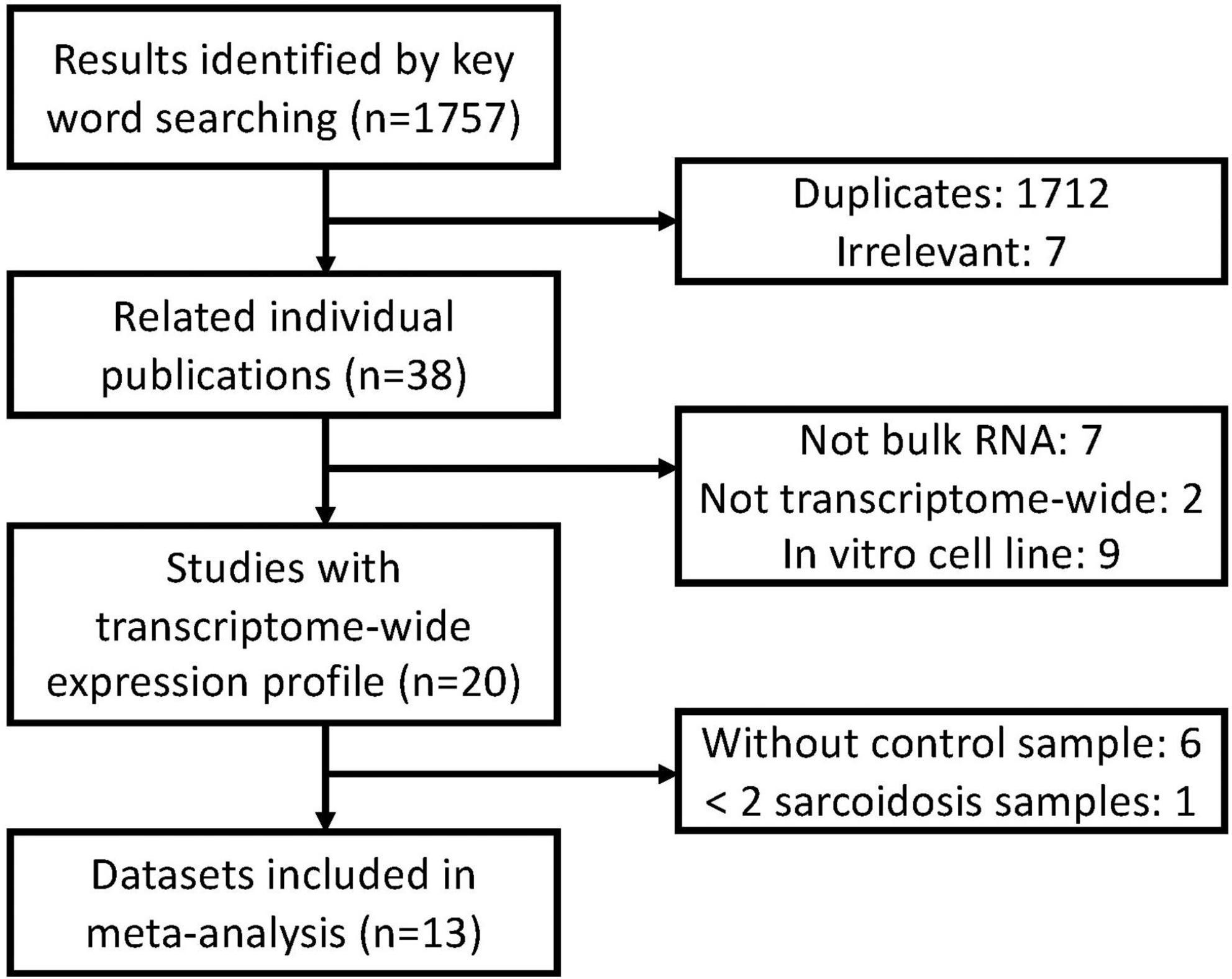
Figure 1. Overview of the meta-analysis approach for development and validation of sarcoidosis transcriptome panel.
Quality control and pre-processing
Selected datasets downloaded from the GEO repository1 consist of different forms of expression measurements and probe annotation files. R package limma and affy were utilized to concordantly process the datasets to enable comparison (16, 17). For datasets with available raw CEL files for download, expression intensities were extracted and normalized using robust multi-array average (RMA) based on the corresponding custom chip definition file (CDF). Only genes estimated to be present in more than 10% samples were included in subsequent analysis. Data generated from Affymetrix HGU133 Plus 2.0, Affymetrix Human Gene/Exon 1.0 ST Array, and Illumina HumanHT-12 V4.0 expression beadchip underwent procedures described above. For RNA sequencing data generated from Illumina HiSeq 3000 platform, raw counts were downloaded and normalization factors were calculated with edgeR (18). Probes of different arrays were subsequently replaced by official gene symbols, and multiple expression measurements were collapsed by maximum value when one gene has replicative measurements.
Differential gene expression analysis for generating meta-signature
We performed differential expression analysis on individual datasets by comparing sarcoidosis vs. normal samples using a linear model-based R package LIMMA (16). Considering limited and incomplete demographic and clinical variables available in public database, we used permutated unwanted variation estimation instead of including available but incomplete variables into the model to test difference between cases and controls. To identify, estimate and remove unwanted sources of variation to compensate for incomplete information of samples provided by public datasets, surrogate variable analysis was applied to each dataset using the “leek” method (19). The estimated surrogate variables were used as covariates in the formula of differential expression analysis. The probes with Benjamini-Hochberg corrected P-value < 0.05, with multiple-testing adjusted, were considered as significant (20). Since inconsistence in terms of study design, cohorts, measurements, etc., meta-analysis was performed with metafor package using residual maximum likelihood (REML) model (21). We performed the analysis in blood sets, lung-associated sets, and all available sets, respectively. Pathway enrichment analysis was further conducted with ClueGO based on GO biological process databases (08/05/2020) (22). For differentially expressed genes (DEGs) in the meta-analysis of blood and lung-associated tissues, significant genes in one meta-analysis but not in the other were identified as tissue-specific. Top genes of these tissue-specific genes were also enriched for biological processes to unveil potential involved pathways of tissue-relevance.
Training and validation of sarcoidosis classifier
To build a diagnostic model for sarcoidosis across tissues, the datasets were further divided into training and testing sets, each containing expression profiles from both blood and lung-associated tissue. Datasets GSE19314, GSE18781, GSE42834, GSE83456, GSE16538, GSE73394, and GSE148036 were used as training set to build the prediction model, while datasets GSE37912, GSE34608, GSE75023, GSE105149, GSE32887, and GSE119136 were included as testing sets. Candidate predictors were filtered by the criteria that the gene should be significantly differentially expressed in more than 3 of the 4 blood sets and in more than 2 of the 3 lung-tissue-associated sets. And those candidates with consistent regulatory directions across the discovery sets will be selected as predictors. The gene with log-transformed fold change (logFC) > 0 is regarded as a positive regulatory factor, and that less than 0 is a negative regulator. Classifier was generated by random forest (RF), Lasso and Elastic-Net Regularized Generalized Linear Models (GLMNET), and Gradient Boosting Machine (GBM) implemented in R package caret. The models were tuned using 10-fold cross-validation. Predictor selection and model training were performed only in training sets, and thus the other test sets could be used as external validation sets. The performance of classifiers was measured using threshold-dependent (sensitivity, specificity, accuracy) and threshold-independent ROC analysis (AUC). The prediction model with the highest performance in the training sets was chosen for assessment of predictive power in six independent testing datasets. To address the problem of systemic difference between cases and controls, randomly selected genes of identical size were compared to ensure the prediction power of model.
Results
Differential expression analysis and meta-signature identification
In total 317 sarcoidosis patients and 339 healthy controls from the 13 GEO datasets were included in the final meta-analysis (Table 1 and Supplementary Table 1). Sarcoidosis patients included cutaneous, pulmonary, and lacrimal gland involvement. Random-effect models were applied to the 6 blood sets, the 4 lung-associated sets, and all 13 sets combined, respectively, to identify genes associated with sarcoidosis within blood, within lung, and between various tissues. Of the 12,968 genes available in at least 3 blood datasets and 3 lung tissue associated sets, 856 were significant at FDR < 0.05 in meta-analysis of peripheral blood, and 690 were significant in lung associated tissues, while 290 genes were differentially expressed when all 13 datasets from various tissues were combined in the meta-analysis (Figure 2A). Despite elevated significance and robustness of the meta-analysis, only 69 DEGs are commonly observed to be differentially expressed in blood and lung tissue, and 29 of them remain significant when more heterogenous sarcoid tissue are included (Table 2).
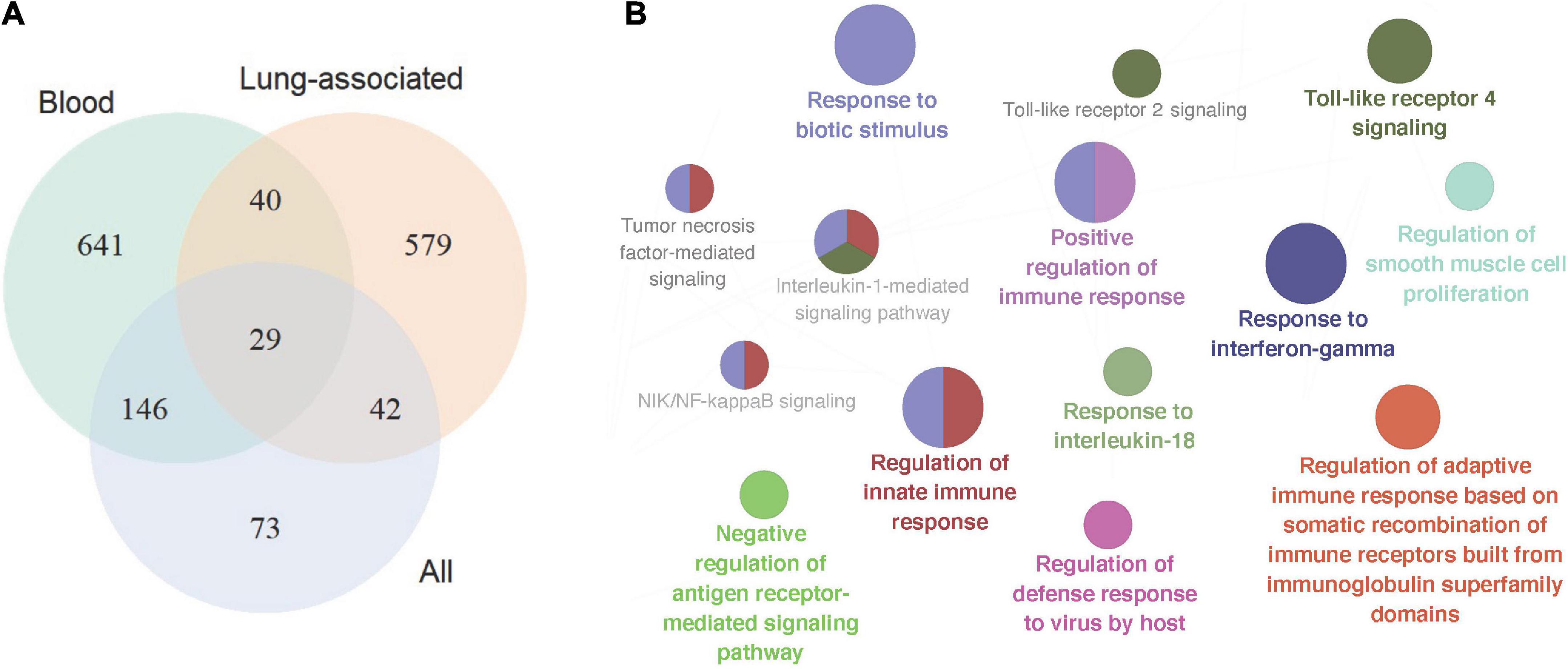
Figure 2. Signature gene identification in blood, lung-associated tissues, and all available tissue types. (A) Venn plot depicted differentially expressed genes in the three sets of meta-analyses, in peripheral blood samples, lung-associated tissues, and in all 13 cross-tissue samples combined, respectively. (B) Pathway enrichment analysis of the top signature genes in the meta-analysis across various tissues. The size of the nodes reflects the enrichment significance of the terms, and the colors denote groups of biological process.
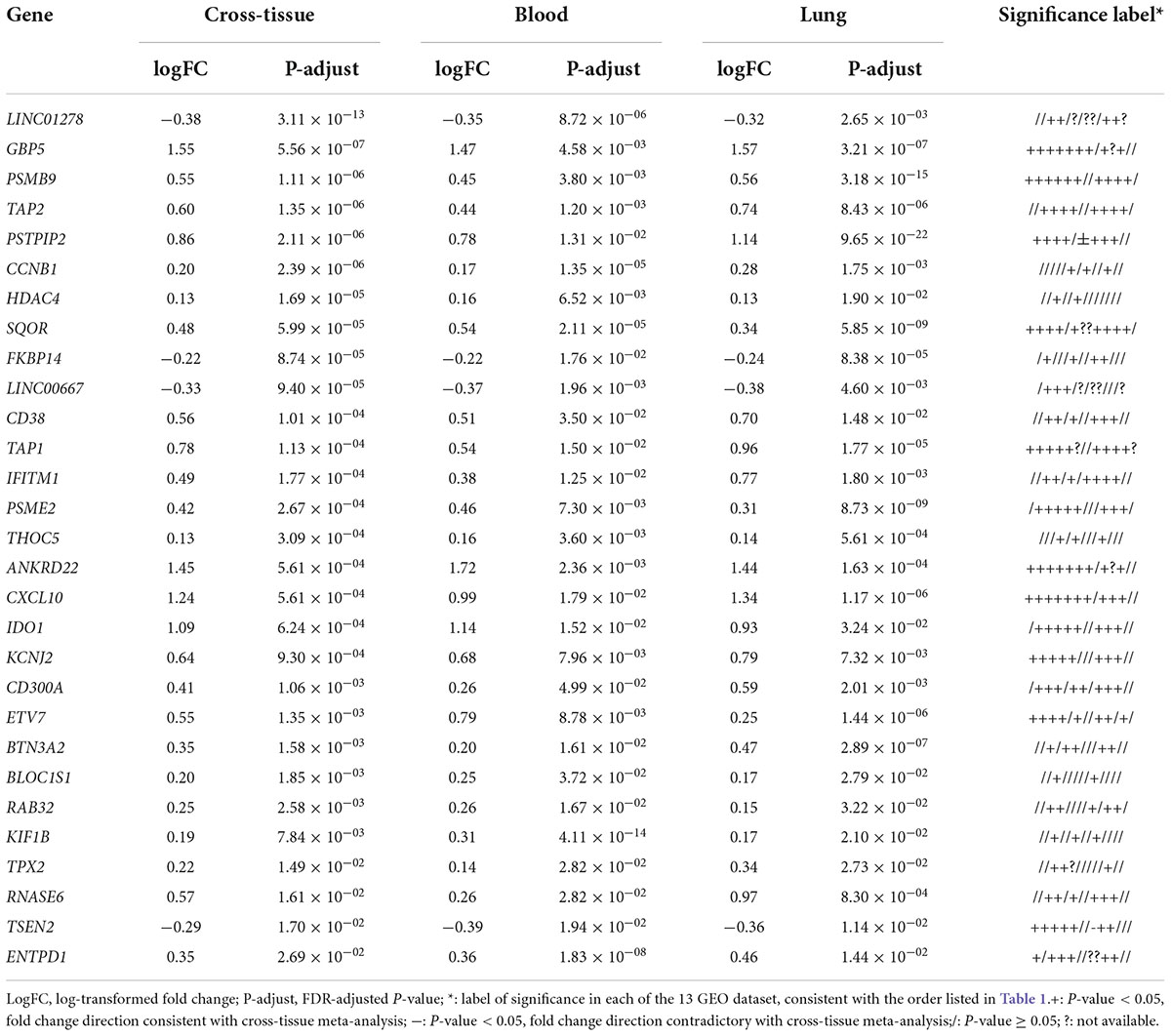
Table 2. Triple-significant differentially expressed genes (DEGs) in cross-tissue, blood, and lung-associated tissue meta-analyses.
Top up-regulated genes in sarcoidosis include interferon signature genes such as GBP5 and IFITM1, indicating active regulation of the interferon signaling. Chemokine genes induced by interferon such as CXCL10 are also upregulated. Intriguingly, we identified genes significantly associated with sarcoidosis that caught limited attention in previous studies. These genes include the long non-coding gene LINC01278 and some other genes known to be involved in the interferon network but lacking understanding of their role in pulmonary diseases, such as IDO1 and BTN3A2.
Down-regulated genes that achieved significance in the meta-analysis of blood but failed in lung-associated tissues include TRABD2A and NLRC3. The former encodes for a metalloprotease and negative regulator or Wnt signaling, while the latter is characterized as a negative regulator of the type I IFN pathway. Up-regulated blood-specific genes include MYD88. The gene ranking top in lung-specific DEGs is the lncRNA gene HCP5 and IL10RA. Most of the genes lack sufficient exploration as to their roles in pulmonary sarcoidosis, but more or less are associated with inflammatory pathways potentially of influence in the pathogenesis of the disease.
Pathway enrichment analysis of sarcoidosis signature genes
Pathway enrichment analysis of the top 200 significant genes in meta-analysis revealed particular pathways that may be associated with sarcoidosis in blood and sarcoid tissues. 55 significant biological processes are functionally grouped into 10 critical pathways as shown in Figure 2B, In addition to the well-known IFN-γ response, we found activation of the cytokines IL-1 and IL-18. Host defense response to biotic stimuli, especially virus infection, is also significantly involved.
Top DEGs in meta-analysis of lung-associated tissue unveiled 16 groups of 36 pathways, most of them also observed in cross-tissue analysis (Supplementary Table 2). Although sarcoidosis is widely recognized as a Th1 disease, NK cells may also play a role in the pathogenesis. The biological process of cell-cell adhesion mediated by integrin is also significantly enriched in lung-associated tissues. With deepened understanding of integrin functions such as roles in cell survival, migration, and proliferation, potentials as therapeutic targets especially in respiratory disease may be exhibited. Pathway enrichment analysis in top genes of peripheral blood showed limited results with 18 biological processes divided into 4 groups, including mitosis, mRNA stabilization, IFN-γ signaling, and viral infection response (Supplementary Table 3). Unsurprisingly, interferon-gamma-mediated signaling pathways are consistently involved in all three meta-analyses.
Biological processes enriched in tissue-specific differentially expressed genes in blood and lung
To identify tissue-specific sarcoidosis signature in peripheral blood and sarcoid lung tissue, we further performed pathway enrichment based on the top 200 genes ranked by P-value selected from those DEGs that achieved significance in blood but not in lung-associated tissues or vice versa (Figure 3). Positive regulation of interleukin-17 (IL-17) production was significantly involved in blood-specific DEGs, concordant with previous understanding of this critical signaling pathway. Activation of autophagy was significantly enriched in both blood and lung-specific genes. The gene AIM2, functioning as a key factor of pyroptosis, was among the identified signatures for sarcoidosis, further emphasizing the role of cell death in sarcoidosis. NK cell mediated immune response to tumor cells was evident in DEGs in lung-associated tissues.
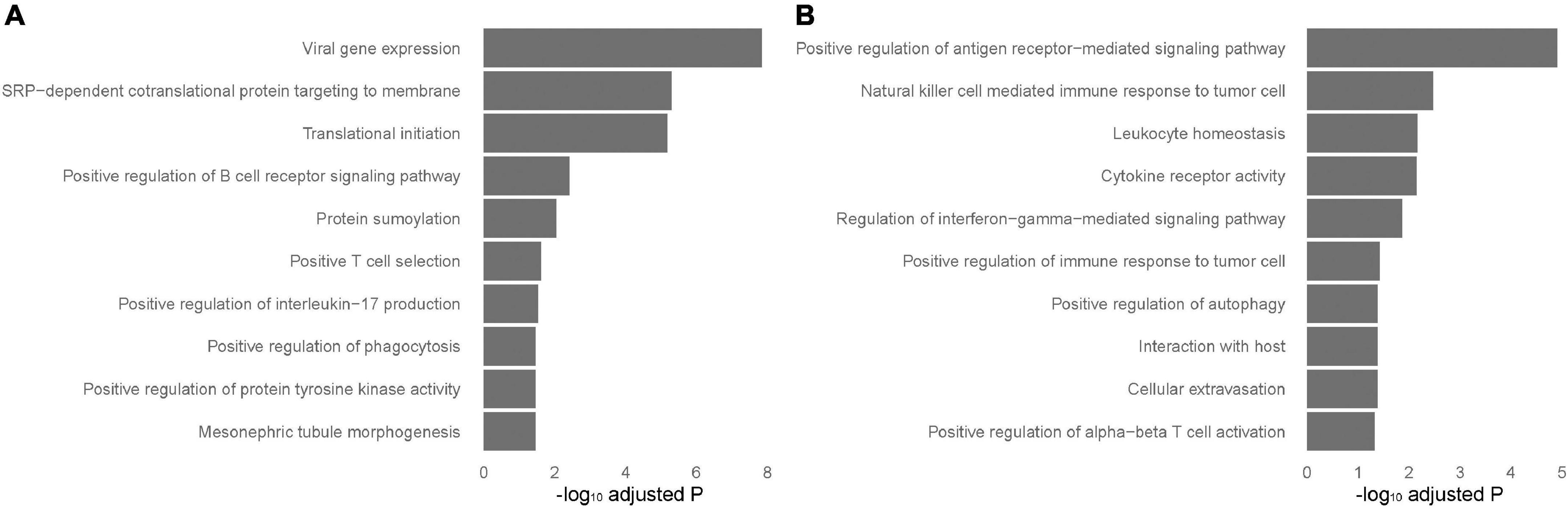
Figure 3. Tissue-specific pathways enriched in blood and lung-associated tissues. Enriched pathways specific to peripheral blood and lung-associated tissues. The analysis was performed based on the top differentially expressed genes specific to peripheral blood (A) and lung-associated tissues (B) identified in the results of meta-analyses.
Predictor selection and classifier establishment
Sixteen genes met the requirement in the training sets based on the variable selection criteria with consistent directions of fold change across all discovery sets (n = 447), indicating robust dysregulation across datasets and thus were used for training of classifier (Figure 4A). All of these 16 genes function as protein-coding genes, and 8 were up-regulated while the other half were down-regulated. GBM and RF outperformed GLMNET in respect of both AUC and accuracy (GBM: AUC = 0.985, Accuracy = 0.937; RF: AUC = 0.998, Accuracy = 0.978; GLMNET: AUC = 0.949, Accuracy = 0.895) (Figure 4B and Supplementary Figure 1). The importance of each gene as a predictor variable was evaluated in the model (Figure 4C). STAT1 provides the heaviest weight in all three models amongst the 16 predictors. Other predictors making major contributions include TMEM140, AQP3, and SOD2.
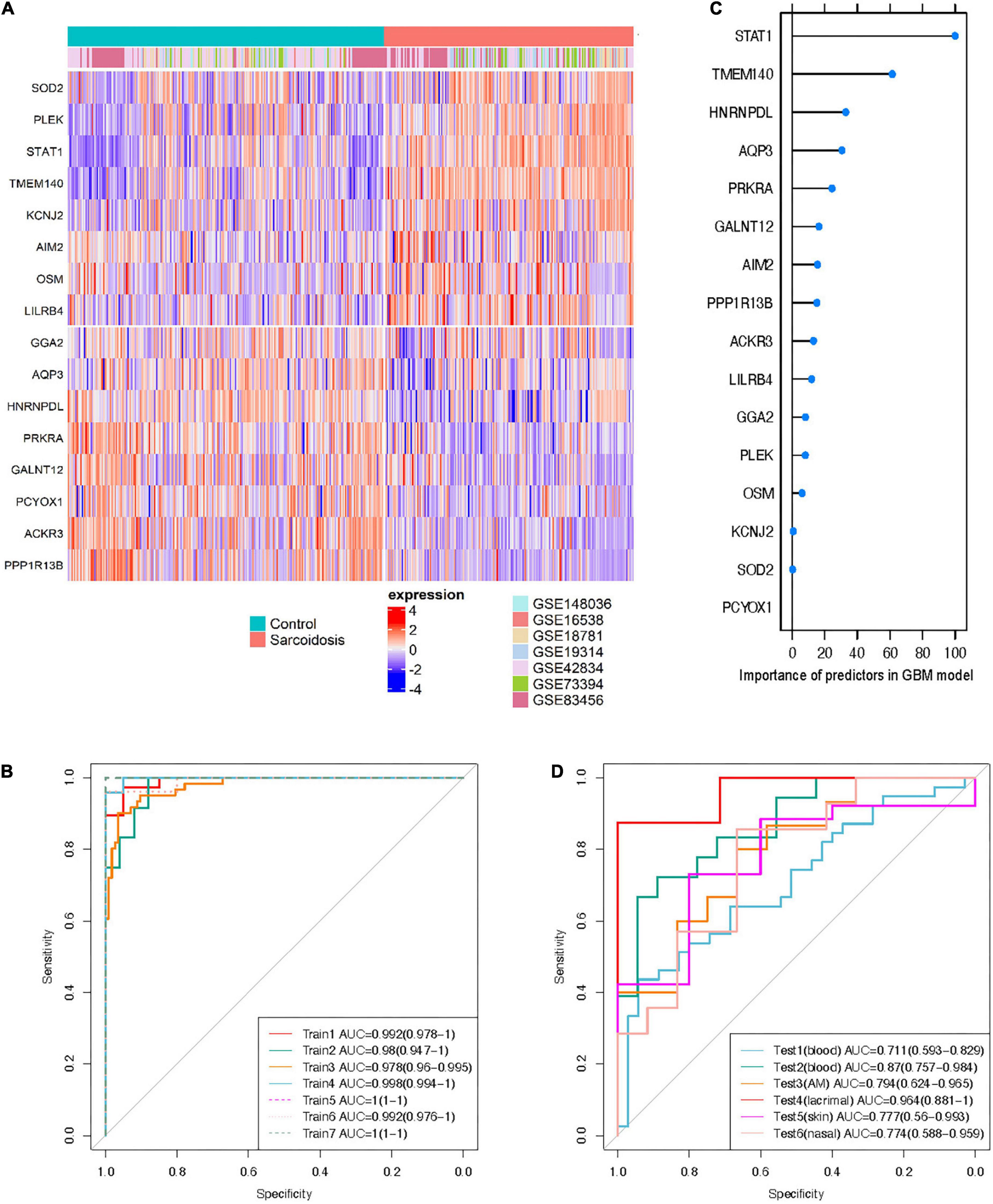
Figure 4. Cross-tissue classification model for sarcoidosis. (A) Heatmap shows expression profile of the 16 predictors in training sets. Half of the predictors were up-regulated while the others were down-regulated. (B) Importance of the predictors in GBM model. ROC curve of the classifier was shown in training sets (C), and test sets (D).
The independent performance of GBM and RF classifiers was evaluated in the 6 external testing sets (n = 209). The GBM model performed more reliable than the RF model in both blood and sarcoid tissues (AUC 0.711–0.964), possibly owing to problem of over-fitting in RF model despite cross-validation (Figure 4D and Supplementary Table 4). The lacrimal tissue set achieved the best AUC with 0.964 (accuracy = 0.933). We also randomly selected gene sets of identical size from the candidates that were available across all 13 datasets. With identical training and testing parameters, the model built from 16 randomly selected genes achieved an AUC of 0.464, 0.596, and 0.528 using the algorithms of GLMNET, GBM, and RF, respectively (Supplementary Figure 1), further emphasizing that the performance of the 16-gene set can be attributed to the biological significance of these consistently differentiated genes.
Discussion
A meta-analysis of transcriptome-wide association studies was conducted between sarcoidosis and normal subjects across tissues by means of mining public datasets. We identified robust and novel genes potentially associated with sarcoidosis. A disease prediction classifier was subsequently built using machine learning and validated in independent datasets to discover candidate cross-tissue sarcoidosis biomarkers.
The expression profile of 29 genes was significantly associated with sarcoidosis the in meta-analysis of blood, lung, and cross-tissue datasets. Intriguingly, the long intergenic non-protein coding RNA LINC01278 was found to be down-regulated in sarcoid tissues. LINC01278 has been proposed to negatively regulate accumulation of β-catenin and ultimately inhibit the transcription of downstream target genes activated by Wnt/β-catenin signaling (23). Evidence of increased pulmonary Wnt-activation has been reported in sarcoidosis, potentially regulating myofibroblast differentiation of lung resident mesenchymal stem cells (24). Although LINC01278 had never been observed to play an explicit role in sarcoidosis, it might contribute to the disease in critical biological pathways.
The role of the MHC genes in presenting antigen and triggering activation of T cells makes them good candidates for involvement in sarcoidosis. PSMB9, a gene downstream of STAT1, which is known to integrate with IFNG and to play a proteolytic role in MHC1 antigen presentation, has been reported to be upregulated in sarcoidosis (25). Non-MHC genes, TAP1, and TAP2, encoding the transporter associated with antigen processing, which participate in the antigen processing pathways prior to its presentation, are also interesting candidates and have been observed to be upregulated in sarcoidosis. A polymorphism of TAP2 detected in patients with sarcoidosis further validated this point (26).
Multifunctional membrane surface glycoprotein (CD38) is considered as a marker of immune activation and involved in the regulation of lymphocyte adhesion to endothelial cells. Both CD3+CD4+CD38+ and CD38+ B cell subsets were found to be elevated in BAL as markers of an acute immune response in sarcoidosis patients (27, 28). In addition to lymphocytes, PSTPIP2, a gene supposed to be associated with autoinflammatory processes of macrophages in a mouse model, was found to be upregulated in progressive fibrotic pulmonary sarcoidosis (12).
Aberrant HDAC enzyme activities are evident in fibrotic diseases, of which HDAC4 is important in lung fibrosis by modulating the production of ECM in lung myofibroblasts (29). Although widely accepted as a key factor in IPF, no HDAC inhibitors (HDACIs) have been investigated in sarcoidosis. Some dysregulated genes discovered in our meta-analysis have not been found to be associated with sarcoidosis previously, but variations of these genes such as CCNB1, BLOC1S1, and KIF1B are associated to some extent with fibrotic diseases, including complication of sarcoidosis and tuberculosis.
Enrichment of biological processes was performed in the top 200 genes ranked by P-value in the meta-analysis of blood, lung, and all datasets, respectively. Biological regulation of NK cells and myeloid cells seem to play a role in sarcoidosis. Increased cells of NK lineage were observed in our single-cell dataset of BAL. It is known that a subpopulation of CD56+ NK cells is activated and produces IFN-γ and TNF-α in sarcoidosis patients, implying involvement of these cells in granuloma formation (30). A strong Th2-M2 polarization was identified in both pulmonary and muscular sarcoidosis (31). Biological processes enriched from blood-specific DEGs revealed positive regulation of IL-17 and tyrosine kinase activity, while both blood and lung-specific genes showed activation of autophagy. Lung-specific genes otherwise were enriched for response of tumor, especially mediated by NK cells. The occurrence of a sarcoid-like localized or distant granulomatous reaction in cancer has been widely realized. In fact, sarcoidosis can occur before, during, or after the onset of solid or hematological malignancies.
To ensure the independence between training and testing sets, predictors of the cross-tissue classifier were selected based on the 7 training sets, and thus moderately different from DEGs in our meta-analysis. Interestingly, KCNJ2, one of the identified cross-tissue signature genes, is included as a predictor, which is activated in IPF but lacks exploration in sarcoidosis (32).
In the past few years, multiple prediction models based on transcriptomic signature have been developed in order to assist in the diagnosis of sarcoidosis, but none of them is currently used in clinical management (13, 33, 34). Intriguingly, microRNAs are frequently used in such models and perform well in diagnosis. Current models are built based on gene signature in peripheral blood or PBMC. The linear signature score is the most commonly used method to build classification models by assigning weights to selected gene markers. Two 8-microRNA diagnostic models achieved accuracy of 0.86 and 1, respectively in development datasets, suggesting that microRNAs might act as a crucial regulator in the pathogenesis (33, 34). The other two models were built using 20 and 17 genes, while long non-coding RNA genes were repeatedly identified as predictors (13, 34). STAT4 and other factors of interferon signaling, as well as cytokine-related genes like IL6ST are major gene markers. These two models also performed well with an accuracy of 0.86 and AUC of 0.87, respectively. Compared with these diagnostic models, our classifier is the first cross-tissue model to predict diagnosis of sarcoidosis, indicating a scheme of systematic transcriptomic alteration over the body. However, whether the diagnostic model based on transcriptome can get universally applied assisting clinical decision still need further studies to validate.
We have to acknowledge some limitations of this study. First, meta-analysis suffers from inherent statistical limitations since the datasets lack concordance being derived from different batches, techniques, and platforms, although surrogate variables were estimated in our study. Also, the definitions of sarcoidosis and controls are slightly different among the datasets, but largely consistent and broadly acceptable. Second, whereas sarcoidosis is a heterogenous and complicated disease, clinical characteristics and disease status were not provided in most public datasets, limiting the clinical interpretation of significant genes. Third, particularly differentially expressed genes were not validated biologically in our study. Further experiments are needed to identify exact role they play in sarcoidosis. Lastly, our classifier tested only sarcoidosis vs. “healthy” controls but not vs. other granulomatous or interstitial lung diseases. The diagnostic power of this classifier to discriminate sarcoidosis from other diseases remains to be investigated.
This transcriptomics-based meta-analysis identified gene expression profiles and shared pathways associated with sarcoidosis across various tissues. This allowed to construct a 16-gene diagnostic classifier for sarcoidosis that potentially can complement more invasive procedures. Its precise diagnostic power needs to be validated in more abundant datasets of various tissues also from patients with other diseases.
Data availability statement
The datasets presented in this study can be found in online repositories. The names of the repository/repositories and accession number(s) can be found in the article/Supplementary material.
Ethics statement
This was a meta-analysis using previously publicated data on public database. The used data were approved previously individually. The patients/participants provided their written informed consent to participate in this study.
Author contributions
HD and CW contributed to conception and design of the study. YJ conducted the analysis. YJ, DJ, and HD drafted the manuscript. UC contributed to the revision of the manuscripts. All authors contributed to manuscript revision and approved the submitted version.
Funding
This work was supported by the National Natural Science Foundation of China (Grant Nos. 82170080 and 81870056) and the Elite Medical Professionals Project of China-Japan Friendship Hospital (No. ZRJY2021-GG11).
Conflict of interest
The authors declare that the research was conducted in the absence of any commercial or financial relationships that could be construed as a potential conflict of interest.
Publisher’s note
All claims expressed in this article are solely those of the authors and do not necessarily represent those of their affiliated organizations, or those of the publisher, the editors and the reviewers. Any product that may be evaluated in this article, or claim that may be made by its manufacturer, is not guaranteed or endorsed by the publisher.
Supplementary material
The Supplementary Material for this article can be found online at: https://www.frontiersin.org/articles/10.3389/fnagi.2022.977191/full#supplementary-material
Footnotes
References
1. Bonham CA, Strek ME, Patterson KC. From granuloma to fibrosis: sarcoidosis associated pulmonary fibrosis. Curr Opin Pulm Med. (2016) 22:484–91. doi: 10.1097/MCP.0000000000000301
2. Broos CE, van Nimwegen M, Hoogsteden HC, Hendriks RW, Kool M, van den Blink B. Granuloma formation in pulmonary sarcoidosis. Front Immunol. (2013) 4:437. doi: 10.3389/fimmu.2013.00437
3. Chen ES. Innate immunity in sarcoidosis pathobiology. Curr Opin Pulm Med. (2016) 22:469–75. doi: 10.1097/MCP.0000000000000305
4. Rosenbaum JT, Pasadhika S, Crouser ED, Choi D, Harrington CA, Lewis JA, et al. Hypothesis: sarcoidosis is a STAT1-mediated disease. Clin Immunol. (2009) 132:174–83. doi: 10.1016/j.clim.2009.04.010
5. Crouser ED, Culver DA, Knox KS, Julian MW, Shao G, Abraham S, et al. Gene expression profiling identifies MMP-12 and ADAMDEC1 as potential pathogenic mediators of pulmonary sarcoidosis. Am J Respir Crit Care Med. (2009) 179:929–38. doi: 10.1164/rccm.200803-490OC
6. Judson MA, Marchell RM, Mascelli M, Piantone A, Barnathan ES, Petty KJ, et al. Molecular profiling and gene expression analysis in cutaneous sarcoidosis: the role of interleukin-12, interleukin-23, and the T-helper 17 pathway. J Am Acad Dermatol. (2012) 66:901–10. doi: 10.1016/j.jaad.2011.06.017
7. Rosenbaum JT, Choi D, Wilson DJ, Grossniklaus HE, Harrington CA, Sibley CH, et al. Parallel gene expression changes in sarcoidosis involving the lacrimal gland, orbital tissue, or blood. JAMA Ophthalmol. (2015) 133:770–7. doi: 10.1001/jamaophthalmol.2015.0726
8. Gharib SA, Malur A, Huizar I, Barna BP, Kavuru MS, Schnapp LM, et al. Sarcoidosis activates diverse transcriptional programs in bronchoalveolar lavage cells. Respir Res. (2016) 17:93. doi: 10.1186/s12931-016-0411-y
9. Barna BP, Huizar I, Malur A, McPeek M, Marshall I, Jacob M, et al. Carbon nanotube-induced pulmonary granulomatous disease: twist1 and alveolar macrophage M1 activation. Int J Mol Sci. (2013) 14:23858–71. doi: 10.3390/ijms141223858
10. Barna BP, Culver DA, Kanchwala A, Singh RJ, Huizar I, Abraham S, et al. Alveolar macrophage cathelicidin deficiency in severe sarcoidosis. J Innate Immun. (2012) 4:569–78. doi: 10.1159/000339149
11. Schischmanoff PO, Naccache JM, Carrere A, Richardson S, Kambouchner M, Raphael M, et al. Progressive pulmonary sarcoidosis is associated with over-expression of TYK2 and p21Waf1/Cip1. Sarcoidosis Vasc Diffuse Lung Dis. (2006) 23:101–7.
12. Koth LL, Solberg OD, Peng JC, Bhakta NR, Nguyen CP, Woodruff PG. Sarcoidosis blood transcriptome reflects lung inflammation and overlaps with tuberculosis. Am J Respir Crit Care Med. (2011) 184:1153–63. doi: 10.1164/rccm.201106-1143OC
13. Zhou T, Zhang W, Sweiss NJ, Chen ES, Moller DR, Knox KS, et al. Peripheral blood gene expression as a novel genomic biomarker in complicated sarcoidosis. PLoS One. (2012) 7:e44818. doi: 10.1371/journal.pone.0044818
14. Costabel U, Hunninghake GW. ATS/ERS/WASOG statement on sarcoidosis. Sarcoidosis statement committee. American thoracic society. European respiratory society. World association for sarcoidosis and other granulomatous disorders. Eur Respir J. (1999) 14:735–7. doi: 10.1034/j.1399-3003.1999.14d02.x
15. Prasse A. The diagnosis, differential diagnosis, and treatment of sarcoidosis. Dtsch Arztebl Int. (2016) 113:565–74. doi: 10.3238/arztebl.2016.0565
16. Ritchie ME, Phipson B, Wu D, Hu Y, Law CW, Shi W, et al. limma powers differential expression analyses for RNA-sequencing and microarray studies. Nucleic Acids Res. (2015) 43:e47. doi: 10.1093/nar/gkv007
17. Gautier L, Cope L, Bolstad BM, Irizarry RA. affy–analysis of affymetrix genechip data at the probe level. Bioinformatics. (2004) 20:307–15. doi: 10.1093/bioinformatics/btg405
18. Robinson MD, McCarthy DJ, Smyth GK. edgeR: a bioconductor package for differential expression analysis of digital gene expression data. Bioinformatics. (2010) 26:139–40. doi: 10.1093/bioinformatics/btp616
19. Leek JT, Johnson WE, Parker HS, Jaffe AE, Storey JD. The sva package for removing batch effects and other unwanted variation in high-throughput experiments. Bioinformatics. (2012) 28:882–3. doi: 10.1093/bioinformatics/bts034
20. Benjamini Y, Hochberg YJ, JotRsss B. Controlling the false discovery rate: a practical and powerful approach to multiple testing. J R Statist Soc. (1995) 57:289–300. doi: 10.1111/j.2517-6161.1995.tb02031.x
21. Viechtbauer W. Conducting meta-analyses in R with the metafor package. J Statist Software. (2010) 36:1–48. doi: 10.18637/jss.v036.i03
22. Bindea G, Mlecnik B, Hackl H, Charoentong P, Tosolini M, Kirilovsky A, et al. ClueGO: a cytoscape plug-in to decipher functionally grouped gene ontology and pathway annotation networks. Bioinformatics. (2009) 25:1091–3. doi: 10.1093/bioinformatics/btp101
23. Lin S, Zhang W, Shi Z, Tan L, Zhu Y, Li H, et al. β-Catenin/LEF-1 transcription complex is responsible for the transcriptional activation of LINC01278. Cancer Cell Int. (2021) 21:380. doi: 10.1186/s12935-021-02082-9
24. Cao H, Wang C, Chen X, Hou J, Xiang Z, Shen Y, et al. Inhibition of Wnt/beta-catenin signaling suppresses myofibroblast differentiation of lung resident mesenchymal stem cells and pulmonary fibrosis. Sci Rep. (2018) 8:13644.
25. Guillaume B, Chapiro J, Stroobant V, Colau D, Van Holle B, Parvizi G, et al. Two abundant proteasome subtypes that uniquely process some antigens presented by HLA class I molecules. Proc Natl Acad Sci USA. (2010) 107:18599–604. doi: 10.1073/pnas.1009778107
26. Foley PJ, Lympany PA, Puscinska E, Zielinski J, Welsh KI, du Bois RM. Analysis of MHC encoded antigen-processing genes TAP1 and TAP2 polymorphisms in sarcoidosis. Am J Respir Crit Care Med. (1999) 160:1009–14. doi: 10.1164/ajrccm.160.3.9810032
27. Aleksoniene R, Besusparis J, Gruslys V, Jurgauskiene L, Laurinaviciene A, Laurinavicius A, et al. CD31(+), CD38(+), CD44(+), and CD103(+) lymphocytes in peripheral blood, bronchoalveolar lavage fluid and lung biopsy tissue in sarcoid patients and controls. J Thorac Dis. (2021) 13:2300–18. doi: 10.21037/jtd-20-2396
28. Kudryavtsev I, Serebriakova M, Starshinova A, Zinchenko Y, Basantsova N, Malkova A, et al. Imbalance in B cell and T follicular helper cell subsets in pulmonary sarcoidosis. Sci Rep. (2020) 10:1059. doi: 10.1038/s41598-020-57741-0
29. Lyu X, Hu M, Peng J, Zhang X, Sanders YY. HDAC inhibitors as antifibrotic drugs in cardiac and pulmonary fibrosis. Ther Adv Chronic Dis. (2019) 10:2040622319862697.
30. Zhang H, Costabel U, Dai H. The role of diverse immune cells in sarcoidosis. Front Immunol. (2021) 12:788502. doi: 10.3389/fimmu.2021.788502
31. Preusse C, Goebel HH, Pehl D, Rinnenthal JL, Kley RA, Allenbach Y, et al. Th2-M2 immunity in lesions of muscular sarcoidosis and macrophagic myofasciitis. Neuropathol Appl Neurobiol. (2015) 41:952–63. doi: 10.1111/nan.12231
32. Lee JU, Chang HS, Jung CA, Kim RH, Park CS, Park JS. Upregulation of potassium voltage-gated channel subfamily J member 2 levels in the lungs of patients with idiopathic pulmonary fibrosis. Can Respir J. (2020) 2020:3406530. doi: 10.1155/2020/3406530
33. Ascoli C, Huang Y, Schott C, Turturice BA, Metwally A, Perkins DL, et al. A circulating microRNA signature serves as a diagnostic and prognostic indicator in sarcoidosis. Am J Respir Cell Mol Biol. (2018) 58:40–54. doi: 10.1165/rcmb.2017-0207OC
Keywords: sarcoidosis, transcriptome, interferon, IL-17, machine learning
Citation: Jiang Y, Jiang D, Costabel U, Dai H and Wang C (2022) A transcriptomics-based meta-analysis identifies a cross-tissue signature for sarcoidosis. Front. Med. 9:960266. doi: 10.3389/fmed.2022.960266
Received: 02 June 2022; Accepted: 30 August 2022;
Published: 20 September 2022.
Edited by:
Ying Zhou, Tongji University, ChinaReviewed by:
Ilias C. Papanikolaou, General Hospital of Corfu, GreeceNancy Casanova, University of Arizona, United States
Claudio Tana, SS Annunziata Polyclinic Hospital, Chieti, Italy
Copyright © 2022 Jiang, Jiang, Costabel, Dai and Wang. This is an open-access article distributed under the terms of the Creative Commons Attribution License (CC BY). The use, distribution or reproduction in other forums is permitted, provided the original author(s) and the copyright owner(s) are credited and that the original publication in this journal is cited, in accordance with accepted academic practice. No use, distribution or reproduction is permitted which does not comply with these terms.
*Correspondence: Huaping Dai, daihuaping@ccmu.edu.cn; Chen Wang, cyh-birm@263.net
†These authors have contributed equally to this work