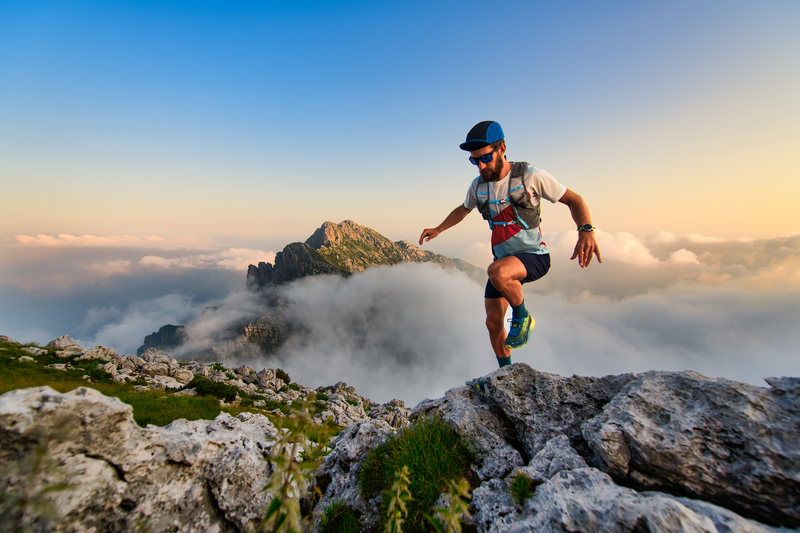
94% of researchers rate our articles as excellent or good
Learn more about the work of our research integrity team to safeguard the quality of each article we publish.
Find out more
MINI REVIEW article
Front. Med. , 30 June 2022
Sec. Rheumatology
Volume 9 - 2022 | https://doi.org/10.3389/fmed.2022.911977
This article is part of the Research Topic Global Excellence in Rheumatology: Asia and Australasia View all 12 articles
Systemic sclerosis (SSc), a complex multi-systemic disease characterized by immune dysregulation, vasculopathy and fibrosis, is associated with high mortality. Its pathogenesis is only partially understood. The heterogenous pathological processes that define SSc and its stages present a challenge to targeting appropriate treatment, with differing treatment outcomes of SSc patients despite similar initial clinical presentations. Timing of the appropriate treatments targeted at the underlying disease process is critical. For example, immunomodulatory treatments may be used for patients in a predominantly inflammatory phase, anti-fibrotic treatments for those in the fibrotic phase, or combination therapies for those in the fibro-inflammatory phase. In advancing personalized care through precision medicine, groups of patients with similar disease characteristics and shared pathological processes may be identified through molecular stratification. This would improve current clinical sub-setting systems and guide personalization of therapies. In this review, we will provide updates in SSc clinical and molecular stratification in relation to patient outcomes and treatment responses. Promises of molecular stratification through advances in high-dimensional tools, including omic-based stratification (transcriptomics, genomics, epigenomics, proteomics, cytomics, microbiomics) and machine learning will be discussed. Innovative and more granular stratification systems that integrate molecular characteristics to clinical phenotypes would potentially improve therapeutic approaches through personalized medicine and lead to better patient outcomes.
Systemic sclerosis (SSc) is a multi-system immune-mediated disease characterized by vasculopathy and fibrosis of skin and internal organs (1). Early clinical manifestations include Raynaud's phenomenon, puffy swollen fingers and gastroesophageal reflux (2, 3). Later manifestations include musculoskeletal involvement, severe vasculopathy such as digital ulcerations, gastrointestinal (GI) complications, interstitial lung disease (ILD), pulmonary arterial hypertension (PAH) and scleroderma renal crisis (4). Although uncommon, SSc has one of the highest morbidity and mortality among autoimmune diseases, with cumulative 10-year survival of 62% from diagnosis (1, 5). Unmet needs in the management of SSc include risk stratification to prognosticate severity of disease complications and to predict treatment responses.
The heterogenous pathological processes that define SSc is a challenge to targeting appropriate treatment. SSc subset classification into relatively homogenous subtypes would have prognostic value to stratify patients for disease complications and treatment responses. The classification of SSc subsets have relied mainly on clinical features. Incorporation of laboratory (e.g., autoantibodies) and molecular gene signatures could lead to a more granular classification system. This is a promising approach toward precision medicine.
The most commonly used SSc classification system is based on the extent of skin involvement, specifically limited cutaneous (lc) and diffuse cutaneous (dc) SSc (6). A minority (<5%) of patients have clinical features of SSc and SSc-specific antibody without any skin involvement, and this group is classified as sine-scleroderma (7). This SSc classification system is suitable for clinical care as it is mainly based on clinical examination of skin with fairly distinct clinical associations and specific serum auto-antibody profiles (8). Nevertheless, due to the heterogenous nature of SSc, patients may present with similar initial clinical manifestations, but have different clinical outcomes and responses to treatment. Thus, SSc classification system needs to be refined to incorporate laboratory tools, such as auto-antibody profiles and gene expression signatures.
Molecular stratification allows segregation of different groups of patients based on pathogenetically homogenous subsets in relation to organ manifestations, prognosis and treatment response. The pathogenesis of SSc involves a complex interplay between immune activation and vascular damage, which leads to activation of fibroblast and excessive collagen deposition in the skin and internal organ (9). Better understanding of variable contributions from each process during the course of the disease could help tailor treatment approaches for different patients.
Therapies used for different clinical manifestations of SSc were shown to have varied efficacy (10). Evidence-based treatment guidelines published in 2017 by EUSTAR adopt an organ-based approach, rather than one based on the patients' clinical, laboratory or molecular subsets (11). The diverse natural course of SSc disease makes it challenging to predict which patients will benefit the most from particular treatment based on clinical manifestations alone. Molecular stratification of SSc will help to personalize treatment based on distinct molecular signatures.
In this review article, we will provide updates in SSc clinical and molecular stratification in relation to patient outcomes and treatment responses. We will also discuss promises of molecular stratification through advances in high-dimensional tools, including deep phenotyping of tissues to single cell analysis, network medicine, omic-based stratification (transcriptomics, genomics, epigenomics, proteomics, cytomics, and microbiomics) and machine learning.
SSc classification system is a rapidly evolving field. Over the years, a combination of multi-system involvement, SSc-specific autoantibodies, and nail-fold capillaroscopy (NFC) patterns have emerged to supplement SSc classification.
The most commonly used system of SSc classification is a two-subset criteria by LeRoy et al., which dichotomizes patients into lcSSc and dcSSc based on extent and pattern of skin fibrosis (12). LcSSc, which includes patients with cutaneous involvement distal to the elbows or knees, is usually associated with anti-centromere antibody (ACA), telangiectasia and late onset of PAH (12). Whereas, dcSSc, which includes patients with cutaneous involvement proximal to the elbows or knees, is frequently associated with anti-topoisomerase I antibody (ATA), tendon friction rub, early internal organ involvement such as ILD, myocardial and diffuse GI tract involvement; hence, dcSSc is known to have poorer prognosis than lcSSc (12).
Although this classification system has a discriminatory value in the prognostication of patients, it has various limitations. There may be overlapping clinical features between the two subsets, e.g., ILD occurrence was 30% in lcSSc and 50% in dcSSc (p = 0.16) (13). A subgroup of patients may have serological, vascular and internal organ manifestations of SSc but without cutaneous involvement, and this subgroup has been classified as sine scleroderma (14). In addition, patients with very early diagnosis of systemic sclerosis (VEDOSS) may not have cutaneous involvement or internal organ involvement but have early SSc features such as Raynaud's phenomenon with vascular changes on NFC or SSc-specific autoantibody (2). A subset of patients may also display overlap syndromes with other connective tissue diseases (e.g., systemic lupus erythematosus and polymyositis), and be variably associated with anti-Ku, PM-Scl75 or anti-U1-ribonucleoprotein antibodies (15, 16).
Combining clinical data with laboratory tools may provide better prognostic value and be feasibly applied in routine clinical care. NFC patterns have been demonstrated to have prognostic value to inform disease activity and disease progression. The abnormal NFC patterns are classified as early, active and late (17, 18). In an international multi-center cohort study evaluating cross-sectional data in the EUSTAR registry, early/active NFC patterns were found in patients with mild/moderate skin involvement and low number of disease manifestations; whereas late NFC pattern was associated with more severe forms of SSc disease (17, 18). Moreover, the NFC pattern could also be an indicator of overall disease progression (18). Prospective cohort study of SSc patients (n = 140) over 3 years showed that reduced capillary density was associated with overall disease progression, progression of skin fibrosis, occurrence of new digital ulcers and new onset PAH (18). Furthermore, the severity of NFC patterns was shown to be predictive of future severe organ involvement with increasing risk from early to late pattern, after adjusting for disease duration, subset and vasoactive medications (19, 20).
SSc-specific autoantibodies are strong predictors of disease outcome and internal organ involvement (21). The 3 main SSc-specific autoantibodies are ACA, ATA and anti-RNA polymerase III antibody (anti-RNAP III), and they are usually mutually exclusive (21, 22). SSc patients with ACA have better prognosis and are more likely to have limited cutaneous involvement and PAH (21). Patients with ATA represent a distinct subgroup with extensive cutaneous involvement and increased risk of ILD (21), and anti-RNAP III represents a subgroup with higher risk of malignancies and development of scleroderma renal crisis (21, 23). Other SSc-specific autoantibodies identified to have prognostic values include anti-Th/To, which is associated with lcSSc and ILD but lower prevalence of PAH (21, 24).
Integration of autoantibody profiles with clinical phenotypes can provide a more robust and comprehensive classification system to risk stratify the patients better. Using cluster analysis of a combination of auto-antibodies and clinical features in 140 SSc patients, Boonstra et al. revealed 5 subgroups of patients with different prognosis and clinical outcomes (25). However, autoantibodies only partially contributed to risk stratification as not all ATA-positive patients had worse prognosis. Another cluster analysis of a large database using clinical and serologic variables (120 EUSTAR centers, n = 6927) showed that dichotomous classification of SSc patients were insufficient as significant proportion of patients with lcSSc (39%) and dcSSc (19%) clustered discordantly. By using data on the presence of organ damage to prognosticate risk of more organ damage/ death, the study identified 6 different clusters with more homogenous clinical phenotypes (Table 1) (26). Although cluster analyses have improved current classification system for better risk stratification, the analysis was driven by data readily available to physicians and none of the analyses included high-throughput molecular data (26). Moreover, the mean disease duration of patients in the EUSTAR study was 11 years (26). We believe that incorporation of high throughput molecular data could improve SSc stratification system.
Van der Kroef et al. showed that prior to the onset of skin fibrosis and other organ manifestations, patients with Raynaud's phenomenon, positivity for SSc-specific autoantibodies and/ or specific NFC patterns, were shown to have different immune cell subset frequencies (Table 1) (28). Hierarchical cluster analysis showed that circulating immune cell population could be used to distinguish different SSc subsets into 4 different clusters, namely cluster 1 (high CD16+ monocytes and low memory B cells), cluster 2 (increased classical monocytes), cluster 3 (increased memory B cells), and cluster 4 (lower classical monocytes) (28). The different clusters were associated with different clinical features, for example limited cutaneous involvement in cluster 1 and no skin involvement in cluster 3 and 4. In contrast, cluster 2 was enriched in patients with ILD and diffuse cutaneous involvement (28). Future studies should further investigate the value of cellular phenotyping in relation to disease progression and treatment response.
SSc classification based on gene expression phenotyping were previously described, namely the fibroproliferative, inflammatory, normal-like intrinsic gene subsets (Table 1) (27, 35). Serial biopsies of skin specimen showed that the intrinsic gene subsets were inherent and stable features of the disease, suggesting distinct pathogenic processes between patients (27, 35). More recent studies by Skaug et al. showed that immune cell and fibroblast signatures changed over time in early dcSSc (within 3 years of disease onset) with a tendency toward normalization as the immune cell and fibroblast signatures declined at follow up (36). This could inform future clinical trials to stratify patients in early disease.
Within an individual with SSc, the intrinsic gene subsets were shown to be consistent across the different skin biopsy sites regardless of the clinical involvement (thickened skin or morphologically normal skin) (27, 35). In addition, the intrinsic gene subsets were demonstrated to be conserved across tissues such as the esophagus and skin (37). This highlights common pathogenic processes in SSc across different tissues. Nevertheless, the tissue microenvironment plays an important role in the immune-fibrotic axes (38). By using functional genomic network analysis, Taroni et al. identified a distinct lung specific innate immune process which suggests certain gene pairs are more likely to interact in a particular tissue than the others (38).
Molecular phenotyping of SSc patients has the potential to guide therapeutic approaches, specifically by selecting treatments that individual patients are most likely to respond based on their unique intrinsic gene subsets. Intrinsic gene subsets at baseline have been shown to potentially be predictive of therapeutic responses. Several studies have investigated the relationship between intrinsic gene subsets and specific treatment responses (Table 2).
SSc patients in the fibroproliferative, but not the inflammatory gene subsets were shown to respond to tyrosine kinase inhibitors (TKI). TKI were explored as therapies for SSc because of the central role of tyrosine kinases in the pathogenesis of fibrosis (46). Imatinib is a small molecule TKI that antagonize c-Abl, a downstream mediator of PDGF and TGFβ receptors (R) (46). Use of Imatinib as experimental drug in SSc was previously reported in patients with dcSSc and clinical response to imatinib showed reduced expressions of genes typically found in the fibroproliferative subset (39). Similarly, responses to nilotinib, another TKI, were seen in patients with higher baseline expression of genes associated with TGFβR and PDGFR signaling, which significantly decreased in the improvers (n = 4, out of 6 patients) (40). In a more recent trial analyzing response to dasatinib, improvers (n = 3, out of total of 12 subjects) mapped to the fibroproliferative or normal-like subsets, whereas most of the non-improvers (n = 7, out of 9 non-improvers) were in the inflammatory subsets (41).
Janus kinase (JAK), which is a non-receptor tyrosine kinase that transduce cytokine signals via phosphorylation of STATs, has been suggested in pre-clinical studies to play a role in the pathogenesis of SSc through either pro-inflammatory or pro-fibrotic signals to the target cells (47). Gene expression profiling analysis confirmed elevated IL6/JAK/STAT and tofacitinib gene signatures in skin biopsies from the previously defined inflammatory subset of dcSSc patients, as compared to healthy controls (48). A pilot, single-center study of patients (n = 10, case series) evaluated the use tofacitinib, which inhibits primary JAK1/3 signaling in dcSSc with refractory skin thickness (49). The results demonstrated significant modified Rodnan skin score (mRSS) improvement in the first month suggesting its role as an effective immunosuppressant in refractory dcSSc with progressive skin thickness. PhaseI/II randomized controlled trial (NCT03274076) by Khanna et al. is ongoing, with initial results showing safety and a trend toward mRSS improvement (50). Further studies are needed to confirm the efficacy of tofacitinib and to evaluate its response in relation to inflammatory and fibrotic gene signatures.
In contrast to improvers to TKI, improvers to immunosuppressive medications were likely to be in the inflammatory gene subsets. Responders to mycophenolate mofetil (MMF) which targets lymphocyte proliferation (51), mapped to the inflammatory gene subset (n = 4, out of 7 improvers), whereas the non-improvers mapped to the fibroproliferative gene subset (n=2 subjects) (43). Likewise, responders to abatacept, which inhibits T cell activation by blocking CD80/CD86 interaction with CD28 (52), were in the inflammatory gene subset (n = 4 out of 5 improvers) and had higher baseline levels of CD28 signaling. The non-improver (n = 1) was in the normal-like gene subset with lower baseline levels of CD28 signaling (44).
Fresolimumab targets TGFβ signaling, with high baseline levels of TGFβ-regulated gene thrombospondin-1 (THBS1) that declined in patients with improved skin scores (42). Taroni et al. performed functional genomic meta-analysis, specifically functional genomic networks and machine learning of publicly available gene expression data from clinical trials of different therapeutics, including MMF and fresolimumab (53). While improvers to fresolimumab had high baseline TGFβ-related genes, non-improvers had elevated baseline levels of immune-related genes (53). Conversely, MMF improvers had high baseline immune-related genes that decreased post treatment (53). This study highlights the significance of genome-wide gene expression data gathered in clinical trials, which provides insight into the functional consequences of treatment and may be used to tailor treatment approaches.
The intrinsic complexity of SSc with heterogeneous manifestations necessitates a more strategic approach to thoroughly understand the underlying molecular mechanisms and to guide therapeutic approaches. Multiple-omics approaches from individual patients should be the direction of future work. Integration of high dimensional data encompassing information from transcriptomics, as well as genomics, epigenomics, proteomics, cytomics and microbiomics could lead to a more granular stratification system.
Through transcriptomic analysis, molecular signatures of SSc patients have been identified as described above. In addition, transcriptomic analysis has also revealed potential biomarkers with cross-sectional relationship with mRSS and may shed light into the disease pathogenesis (54). Two of the genes, cartilage oligomeric protein (COMP) and thrombospondin-1 (THBS1), are known to be regulated by transforming growth factor-β (TGFβ), whereas the other two genes, interferon-induced protein 44 (IFI44), and sialoadhesin (SIGLEC1) are known to be regulated by interferon (IFN) (54). More recently, systemic gene expression profiling through high throughput unsupervised clustering analysis has identified multiple genes as potential pharmacodynamic biomarkers in SSc skin (55). The identified genes were not limited to TGF? and IFN-regulated genes, but also MHC class I, proteasome, antigen processing, macrophage and vascular marker genes (55). These results highlight the roles of macrophage driven and vascular injury pathways in driving the disease process leading to fibrosis (55).
Technological advances in genomics, such as genome-wide association study (GWAS) and candidate gene approach (CGA) have highlighted important SSc susceptibility genes and non-HLA susceptibility genes (56). The majority of SSc susceptibility loci were found to be involved in innate or adaptive immune system (56). In addition, meta-analysis of GWAS revealed molecular pathways potentially involved in vasculopathy and fibrosis, both of which are central in the pathogenesis of SSc (57).
Although genetics play an important role in SSc pathogenesis, genetic factors alone are not sufficient to explain the disease occurrence, as there is low concordance rate of SSc among monozygotic twins (58). It is believed that environmental factors play an important role in the disease pathogenesis possibly through epigenetic regulation mediated through modifications in DNA, histone and non-coding RNAs (ncRNAs) (58, 59). However, the underlying pathophysiology linking genetic factors, epigenetic and environmental factors are still not fully understood.
The cellular responses to genetic, epigenetic and environment factors are reflected in the proteomic profiles. Accumulating data in high throughput proteomics have pointed to number of proteins and pathways associated with SSc progression and pathogenesis (60). Through progress in aptamer-based proteomic technology, a large array of serum protein was identified and could potentially be used as biomarkers in SSc to assess clinical progress as a number of differentially expressed proteins were found to correlate with mRSS (33). Differential expressions of proteins (midkine and FSTL3) were found in SSc patients with PAH and could potentially serve as a PAH biomarker and promising drug target (34). Type I interferons (IFNs), which are key regulators of innate immunity, play a role in the pathophysiology of SSc (61). Type I IFN signature was found in patients with very early SSc (before overt skin fibrosis), ATA and anti-U1 RNP antibodies (62, 63). High IFN-inducible chemokine levels were correlated with more severe skin, lung and muscle involvement in SSc (64). IFN-inducible proteins were demonstrated to have promising prognostic value in predicting treatment response (45, 61, 65).
Phase I trial of anifrolumab for SSc showed suppressed IFN signature in whole blood and skin, and this finding corresponded to suppression of T cell activation and collagen accumulation (66, 67). These shed light to the promising potential of using peripheral markers (e.g. high or low IFN signatures) to stratify patients for targeted treatment. More recently, serum proteins were shown to potentially be useful to guide therapeutic approaches in SSc-ILD patients (45). SSc-ILD patients with higher score of serum interferon-inducible proteins (IFNγ-inducible10-kd protein, monokine induced by IFNγ, monocyte chemotactic protein 2, β2-microglobulin, tumor necrosis factor receptor type II, and macrophage inflammatory protein 3β) responded better to immunosuppression (45). Future prospective longitudinal clinical studies are needed to evaluate the prognostic values of various candidate proteomic biomarkers in clinical practice.
In comparison to above techniques, cytomics allows simultaneous analysis of a number of parameters. It could be potentially used to shed light into the pathophysiology of SSc. High dimensional cytometry has proven to be a powerful tool to quantify large number of immune cell subsets and analyze their correlation with clinical markers (28, 68, 69). The frequency of monocyte subsets was found to be correlated with disease severity in SSc and changes in monocyte frequencies were already noted in the early phase of SSc disease in the pre-fibrotic stage. (28) Our group investigated blood mononuclear cells from SSc patients using mass cytometry and transcriptomic analysis (68). Unsupervised clustering analysis were performed to identify nodes composed of similar cells, and the results revealed significant differences in the frequencies of T and B cell subsets in SSc subsets, as well as compared to healthy controls (68). In patients with ILD compared to those without ILD, we found increased nodes representing CD4+ T cells expressing CCR4 and ICOS, but decreased nodes representing mucosal associated invariant T cells (68). In addition, based on peripheral blood immune cell phenotypes and organ involvement, Kubo S et al. stratified SSc patients into 3 groups: Treg-dominant group, Tfh-dominant group and fewer abnormalities group (Table 1) (29). Future studies could evaluate the potential role of immune cell phenotypes to prognosticate therapeutic response, e.g., role of targeted therapy for B cells by rituximab in Tfh-plasmablast dominant group. Despite these advances, identification of high dimensional biomarkers to clearly stratify patients with SSc remains an unmet need. The Extended Polydimensional Immunome Characterization (EPIC), a web-based discovery tool could be deployed for comprehensive analyses of single cell dataset to identify high dimensional biomarkers in SSc patients in comparison to healthy datasets (69).
Dysbiosis of the GI microbiome is known to have systemic effect on the immune system in SSc (70). Two culture-independent metagenomic sequencing technologies have been used to characterize the GI microbiome. Most commonly reported technology is the 16S RNA sequencing, which enables bacterial identification (answers the question “who are they?”). Whole-genome shotgun sequencing enables the identification of the gene and their metabolic and enzymatic pathways (answers the question “what are they doing?”). In SSc compared to healthy controls, consistent observations using both approaches have shown reduced abundance of Bacteroides species (which protects host from mucosal inflammation), Clostridium and Faecalibacterium species (butyrate-producing organisms that enhance epithelial barrier function), and increased abundance of Lactobacillus (implicated in SSc GI dysmotility) and Bifidobacterium species (30, 70). Alpha diversity, which is the complexity of microbiome composition within individuals of a group, was suggested to be decreased with SSc patients with more severe disease, longer disease duration and dcSSc (Table 1) (30–32, 71–73). There have been a few small clinical trials on GI-microbiome therapeutic interventions in SSc (74–76). In a placebo-controlled trial of probiotics, Low et al. found that baseline microbiome composition and probiotics were independently associated with GI symptom improvement. (75) These suggest a potential role of GI microbiome modulation to improve GI symptoms. Longitudinal studies of GI microbiome in SSc are needed to understand the contribution of microbiome alteration to the development of GI and extra-intestinal manifestations of SSc.
With the tremendous amount of multi-omics data in SSc, advances in machine learning has made it possible to integrate high dimensional data with cutting-edge computational tools. Systems biology based approach has the potential to condense multiple-omics data to derive meaningful molecular interaction network and facilitate better capture of SSc complex pathogenesis (77). Although it is challenging to delineate the modular organization at the molecular level, multiple integration strategies have been developed to analyze regulatory relationships between each omic layer (78). Future integration of multi-omics data may improve our understanding of complex SSc pathogenesis and distinguish distinct patient subtypes.
SSc is a complex multisystemic disease with heterogenous clinical manifestations and characteristics. Advances in transcriptomics have led to identification of distinct SSc molecular gene signatures. Tremendous amount of multi-omics data has emerged in SSc field and machine learning could be deployed to integrate and analyze multi-omic data in SSc to develop a more granular classification system.
Challenges in multi-omic data analysis include the rarity of SSc disease and hence its limited sample size. Furthermore, lack of clinical information, e.g., disease duration or treatment history, further hinders clinical phenotyping of the subjects in publicly available datasets. In the future, a more comprehensive longitudinal study of SSc patients and collaborative effort to integrate high dimensional data would be pivotal to create an SSc atlas at global level to gain more insights into disease etiology, prognosis and progression. This may help the characterization of heterogenous SSc patients and personalization of therapeutic approaches toward precision medicine.
AL and SA contributed to conception and design of the study. MN wrote the first draft of the manuscript. VC contributed to manuscript revision and editing. All authors contributed to manuscript revision, read, and approved the submitted version.
MN holds Seah Cheng Siang Memorial Research Award; AL holds a National Medical Research Council (NMRC) Clinical Scientist Award (grant number: MOH-CSAINV19may-0005); SA holds grant support from NMRC (NMRC/OFLCG/002/2018, CIRG19may-0052, MOH-STaR19nov-0002, COVID19TUG21-0120, NMRC/CG1/006/2021-KKH/MOH-000988-00), and A*STAR (H22P0M0003).
The authors declare that the research was conducted in the absence of any commercial or financial relationships that could be construed as a potential conflict of interest.
All claims expressed in this article are solely those of the authors and do not necessarily represent those of their affiliated organizations, or those of the publisher, the editors and the reviewers. Any product that may be evaluated in this article, or claim that may be made by its manufacturer, is not guaranteed or endorsed by the publisher.
1. Denton CP, Khanna D. Systemic sclerosis. Lancet. (2017) 390:1685–99. doi: 10.1016/S0140-6736(17)30933-9
2. Avouac J, Fransen J, Walker UA, Riccieri V, Smith V, Muller C, et al. Preliminary criteria for the very early diagnosis of systemic sclerosis: results of a Delphi Consensus Study from EULAR Scleroderma Trials and Research Group. Ann Rheum Dis. (2011) 70:476–81. doi: 10.1136/ard.2010.136929
3. Van den Hombergh WM, Carreira PE, Knaapen-Hans HK, van den Hoogen FH, Fransen J, Vonk MC. An easy prediction rule for diffuse cutaneous systemic sclerosis using only the timing and type of first symptoms and auto-antibodies: derivation and validation. Rheumatology (Oxford). (2016) 55:2023–32. doi: 10.1093/rheumatology/kew305
4. Allanore Y, Simms R, Distler O, Trojanowska M, Pope J, Denton CP, et al. Systemic sclerosis. Nat Rev Dis Primers. (2015) 23:15002. doi: 10.1038/nrdp.2015.2
5. Rubio-Rivas M, Royo C, Simeón CP, Corbella X, Fonollosa V. Mortality and survival in systemic sclerosis: systematic review and meta-analysis. Semin Arthritis Rheum. (2014) 44:208–19. doi: 10.1016/j.semarthrit.2014.05.010
6. Wollheim FA. Classification of systemic sclerosis. Visions Reality Rheumato (Oxford). (2005) 44:1212–16. doi: 10.1093/rheumatology/keh671
7. Diab S, Dostrovsky N, Hudson M, Tatibouet S, Fritzler MJ, Baron M, et al. Systemic sclerosis sine scleroderma: a multicenter study of 1417 subjects. J Rheumatol. (2014) 41:2179–85. doi: 10.3899/jrheum.140236
8. Liaskos C, Marou E, Simopoulou T, Barmakoudi M, Efthymiou G, Scheper T, et al. Disease-related autoantibody profile in patients with systemic sclerosis. Autoimmunity. (2017) 50:414–21. doi: 10.1080/08916934.2017.1357699
9. Varga J, Trojanowska M, Kuwana M. Pathogenesis of systemic sclerosis: recent insights of molecular and cellular mechanisms and therapeutic opportunities. J Scleroderma Relat Disord. (2017) 2:137–52. doi: 10.5301/jsrd.5000249
10. Campochiaro C, Allanore Y. An update on targeted therapies in systemic sclerosis based on a systematic review from the last 3 years. Arthritis Res Ther. (2021) 23:155. doi: 10.1186/s13075-021-02536-5
11. Kowal-Bielecka O, Fransen J, Avouac J, Becker M, Kulak A, Allanore Y, et al. Update of EULAR recommendations for the treatment of systemic sclerosis. Ann Rheum Dis. (2017) 76:1327–39. doi: 10.1136/annrheumdis-2016-209909
12. LeRoy EC, Black C, Fleischmajer R, Jablonska S, Krieg T, Medsger TA Jr, et al. Scleroderma (systemic sclerosis): classification, subsets and pathogenesis. J Rheum. (1988) 15:202–5.
13. Kane GC, Varga J, Conant EF, Spirn PW, Jimenez S, Fish JE. Lung involvement in systemic sclerosis (scleroderma): relation to classification based on extent of skin involvement or autoantibody status. Respir Med. (1996) 90:223–30. doi: 10.1016/S0954-6111(96)90291-7
14. Poormoghim H, Lucas M, Fertig N, Medsger TA Jr. Systemic sclerosis sine scleroderma: demographic, clinical, and serologic features and survival in forty-eight patients. Arthritis Rheum. (2000) 43:444–51. doi: 10.1002/1529-0131(200002)43:2<444::AID-ANR27>3.0.CO;2-G
15. Hoa S, Hudson M, Troyanov Y, Proudman S, Walker J, Stevens W, et al. Single-specificity anti-Ku antibodies in an international cohort of 2140 systemic sclerosis subjects: clinical associations. Medicine (Baltimore). (2016) 95:e4713. doi: 10.1097/MD.0000000000004713
16. Chaigne B, Scirè CA, Talarico R, Alexander T, Amoura Z, Avcin T, et al. Mixed connective tissue disease: state of the art on clinical practice guidelines. RMD Open. (2019) 4:e000783. doi: 10.1136/rmdopen-2018-000783
17. Ingegnoli F, Ardoino I, Boracchi P, Cutolo M. EUSTAR co-authors. Nailfold capillaroscopy in systemic sclerosis: data from the EULAR scleroderma trials and research (EUSTAR) database. Microvasc Res. (2013) 89:122–8. doi: 10.1016/j.mvr.2013.06.003
18. Avouac J, Lepri G, Smith V, Toniolo E, Hurabielle C, Vallet A, et al. Sequential nailfold videocapillaroscopy examinations have responsiveness to detect organ progression in systemic sclerosis. Semin Arthritis Rheum. (2017) 47:86–94. doi: 10.1016/j.semarthrit.2017.02.006
19. Smith V, Riccieri V, Pizzorni C, Decuman S, Deschepper E, Bonroy C, et al. Nailfold capillaroscopy for prediction of novel future severe organ involvement in systemic sclerosis. J Rheumatol. (2013) 40:2023–8. doi: 10.3899/jrheum.130528
20. Smith V, Decuman S, Sulli A, Bonroy C, Piettte Y, Deschepper E, et al. Do worsening scleroderma capillaroscopic patterns predict future severe organ involvement? a pilot study. Ann Rheum Dis. (2012) 71:1636–9. doi: 10.1136/annrheumdis-2011-200780
21. Nihtyanova SI, Denton CP. Autoantibodies as predictive tools in systemic sclerosis. Nat Rev Rheumatol. (2010) 6:112–6. doi: 10.1038/nrrheum.2009.238
22. Ho KT, Reveille JD. The clinical relevance of autoantibodies in scleroderma. Arthritis Res Ther. (2003) 5:80–93. doi: 10.1186/ar628
23. Iniesta Arandia N, Simeón-Aznar CP, Guillén Del Castillo A, Colunga Argüelles D, Rubio-Rivas M, Trapiella Martínez L, et al. Influence of antibody profile in clinical features and prognosis in a cohort of Spanish patients with systemic sclerosis. Clin Exp Rheumatol. (2017) 35 Suppl 106:98–105.
24. Ceribelli A, Cavazzana I, Franceschini F, Airò P, Tincani A, Cattaneo R, et al. Anti-Th/To are common antinucleolar autoantibodies in Italian patients with scleroderma. J Rheumatol. (2010) 37:2071–5. doi: 10.3899/jrheum.100316
25. Boonstra M, Mertens BJA, Bakker JA, Ninaber MK, Ajmone Marsan N. van der Helm-van Mil AHM, et al. To what extent do autoantibodies help to identify high-risk patients in systemic sclerosis? Clin Exp Rheumatol. (2018) 36:109–17.
26. Sobanski V, Giovannelli J, Allanore Y, Riemekasten G, Airò P, Vettori S, et al. Phenotypes determined by cluster analysis and their survival in the prospective european scleroderma trials and research cohort of patients with systemic sclerosis. Arthritis Rheumatol. (2019) 71:1553–70. doi: 10.1002/art.40906
27. Pendergrass SA, Lemaire R, Francis IP, Mahoney JM, Lafyatis R, Whitfield ML. Intrinsic gene expression subsets of diffuse cutaneous systemic sclerosis are stable in serial skin biopsies. J Invest Dermatol. (2012) 132:1363–73. doi: 10.1038/jid.2011.472
28. van der Kroef M, van den Hoogen LL, Mertens JS, Blokland SLM, Haskett S, Devaprasad A, et al. Cytometry by time of flight identifies distinct signatures in patients with systemic sclerosis, systemic lupus erythematosus and Sjogrens syndrome. Eur J Immunol. (2020) 50:119–29. doi: 10.1002/eji.201948129
29. Kubo S, Nakayamada S, Miyazaki Y, Yoshikawa M, Yoshinari H, Satoh Y, et al. Distinctive association of peripheral immune cell phenotypes with capillaroscopic microvascular patterns in systemic sclerosis. Rheumatology. (2019) 58:2273–83. doi: 10.1093/rheumatology/kez244
30. Plichta DR, Somani J, Pichaud M, Wallace ZS, Fernandes AD, Perugino CA, et al. Congruent microbiome signatures in fibrosis-prone autoimmune diseases: IgG4-related disease and systemic sclerosis. Genome Med. (2021) 13:35. doi: 10.1186/s13073-021-00853-7
31. Volkmann ER, Hoffmann-Vold AM, Chang YL, Jacobs JP, Tillisch K, Mayer EA, et al. Systemic sclerosis is associated with specific alterations in gastrointestinal microbiota in two independent cohorts. BMJ Open Gastroenterol. (2017) 14:e000134. doi: 10.1136/bmjgast-2017-000134
32. Natalello G, Bosello SL, Paroni Sterbini F, Posteraro B, De Lorenzis E, Canestrari GB, et al. Gut microbiota analysis in systemic sclerosis according to disease characteristics and nutritional status. Clin Exp Rheumatol. (2020) 38:73–84.
33. Rice LM, Mantero JC, Stifano G, Ziemek J, Simms RW, Gordon J, et al. A proteome-derived longitudinal pharmacodynamic biomarker for diffuse systemic sclerosis skin. J Invest Dermatol. (2017) 137:62–70. doi: 10.1016/j.jid.2016.08.027
34. Rice LM, Mantero JC, Stratton EA, Warburton R, Roberts K, Hill N, et al. Serum biomarker for diagnostic evaluation of pulmonary arterial hypertension in systemic sclerosis. Arthritis Res Ther. (2018) 20:185. doi: 10.1186/s13075-018-1679-8
35. Milano A, Pendergrass SA, Sargent JL, George LK, McCalmont TH, Connolly MK, Whitfield ML. Molecular subsets in the gene expression signatures of scleroderma skin. PLoS ONE. (2008) 3: 1371. doi: 10.1371/annotation/05bed72c-c6f6-4685-a732-02c78e5f66c2
36. Skaug B, Lyons MA, Swindell WR, Salazar GA, Wu M, Tran TM, et al. Large-scale analysis of longitudinal skin gene expression in systemic sclerosis reveals relationships of immune cell and fibroblast activity with skin thickness and a trend towards normalisation over time. Ann Rheum Dis. (2022) 81:516–23. doi: 10.1136/annrheumdis-2021-221352
37. Taroni JN, Martyanov V, Huang CC, Mahoney JM, Hirano I, Shetuni B, et al. Molecular characterization of systemic sclerosis esophageal pathology identifies inflammatory and proliferative signatures. Arthritis Res Ther. (2015) 17:194. doi: 10.1186/s13075-015-0695-1
38. Taroni JN, Greene CS, Martyanov V, Wood TA, Christmann RB, Farber HW, et al. A novel multi-network approach reveals tissue-specific cellular modulators of fibrosis in systemic sclerosis. Genome Med. (2017) 9:27. doi: 10.1186/s13073-017-0417-1
39. Chung L, Fiorentino DF, Benbarak MJ, Adler AS, Mariano MM, Paniagua RT, et al. Molecular framework for response to imatinib mesylate in systemic sclerosis. Arthritis Rheum. (2009) 60:584–91. doi: 10.1002/art.24221
40. Gordon JK, Martyanov V, Magro C, Wildman HF, Wood TA, Huang WT, et al. Nilotinib (Tasigna) in the treatment of early diffuse systemic sclerosis: An open-label, pilot clinical trial. Arthritis Res Ther. (2015) 17:213. doi: 10.1186/s13075-015-0721-3
41. Martyanov V, Kim GJ, Hayes W, Du S, Ganguly BJ, Sy O, et al. Novel lung imaging biomarkers and skin gene expression subsetting in dasatinib treatment of systemic sclerosis-associated interstitial lung disease. PLoS ONE. (2017) 12:e0187580. doi: 10.1371/journal.pone.0187580
42. Rice LM, Padilla CM, McLaughlin SR, Mathes A, Ziemek J, Goummih S, et al. Fresolimumab treatment decreases biomarkers and improves clinical symptoms in systemic sclerosis patients. J Clin Invest. (2015) 125:2795–807. doi: 10.1172/JCI77958
43. Hinchcliff M, Huang CC, Wood TA, Matthew Mahoney J, Martyanov V, Bhattacharyya S, et al. Molecular signatures in skin associated with clinical improvement during mycophenolate treatment in systemic sclerosis. J Invest Dermatol. (2013) 133:1979–89. doi: 10.1038/jid.2013.130
44. Chakravarty EF, Martyanov V, Fiorentino D, Wood TA, Haddon DJ, Jarrell JA, et al. Gene expression changes reflect clinical response in a placebo-controlled randomized trial of abatacept in patients with diffuse cutaneous systemic sclerosis. Arthritis Res Ther. (2015) 17:159. doi: 10.1186/s13075-015-0669-3
45. Assassi S, Li N, Volkmann ER, Mayes MD, Rünger D, Ying J, et al. Predictive significance of serum interferon-inducible protein score for response to treatment in systemic sclerosis–related interstitial lung disease. Arthritis Rheum. (2021) 73:1005–13. doi: 10.1002/art.41627
46. Beyer C, Distler JHW. Tyrosine kinase signaling in fibrotic disorders: translation of basic research to human disease. Biochim Biophys Acta. (2013) 1832:897–904. doi: 10.1016/j.bbadis.2012.06.008
47. Talotta R. The rationale for targeting the JAK/STAT pathway in scleroderma-associated interstitial lung disease. Immunotherapy. (2021) 13:241–56. doi: 10.2217/imt-2020-0270
48. Wang W, Bhattacharyya S, Marangoni RG, Carns M, Dennis-Aren K, Yeldandi A, et al. The JAK/STAT pathway is activated in systemic sclerosis and is effectively targeted by tofacitinib. J Scleroderma Relat Disord. (2020) 5:40–50. doi: 10.1177/2397198319865367
49. You H, Xu D, Hou Y, Zhou J, Wang Q, Li M, et al. Tofacitinib as a possible treatment for skin thickening in diffuse cutaneous systemic sclerosis. Rheumatology (Oxford). (2021) 60:2472–7. doi: 10.1093/rheumatology/keaa613
50. Khanna D, Bush E, Nagaraja V, Koenig A, Khanna P, Young A, et al. Tofacitinib in early diffuse cutaneous systemic sclerosis— results of Phase I/II investigator-initiated, double-blind randomized placebo-controlled trial [abstract]. Arthritis Rheumatol. (2019) 71.
51. Omair MA, Alahmadi A, Johnson SR. Safety and effectiveness of mycophenolate in systemic sclerosis. a systematic review. PLoS ONE. (2015) 10:e0124205. doi: 10.1371/journal.pone.0124205
52. Boleto G, Allanore Y, Avouac J. Targeting costimulatory pathways in systemic sclerosis. Front Immunol. (2018) 9:2998. doi: 10.3389/fimmu.2018.02998
53. Taroni JN, Martyanov V, Mahoney JM, Whitfield ML. A Functional genomic meta-analysis of clinical trials in systemic sclerosis: toward precision medicine and combination therapy. J Invest Dermatol. (2017) 137:1033–41. doi: 10.1016/j.jid.2016.12.007
54. Farina G, Lafyatis D, Lemaire R, Lafyatis R. A four-gene biomarker predicts skin disease in patients with diffuse cutaneous systemic sclerosis. Arthritis Rheum. (2010) 62:580–8. doi: 10.1002/art.27220
55. Rice LM, Ziemek J, Stratton EA, McLaughlin SR, Padilla CM, Mathes AL, et al. A longitudinal biomarker for the extent of skin disease in patients with diffuse cutaneous systemic sclerosis. Arthritis Rheumatol. (2015) 67:3004–15. doi: 10.1002/art.39287
56. Ota Y, Kuwana M. Updates on genetics in systemic sclerosis. Inflamm Regener. (2021) 41:1. doi: 10.1186/s41232-021-00167-6
57. López-Isac E, Acosta-Herrera M, Kerick M, Assassi S, Satpathy AT, Granja J, et al. GWAS for systemic sclerosis identifies multiple risk loci and highlights fibrotic and vasculopathy pathways. Nat Commun. (2019) 10:4955. doi: 10.1038/s41467-019-12760-y
58. Ramahi A, Altorok N, Kahaleh B. Epigenetics and systemic sclerosis: an answer to disease onset and evolution? Eur J Rheumatol. (2020) 7:S147–S56. doi: 10.5152/eurjrheum.2020.19112
59. Rusek M, Krasowska D. Non-Coding RNA in systemic sclerosis: a valuable tool for translational and personalized medicine. Genes (Basel). (2021) 12:1296. doi: 10.3390/genes12091296
60. Chairta P, Nicolaou P, Sokratous K, Galant C, Houssiau F, Oulas A, et al. Comparative analysis of affected and unaffected areas of systemic sclerosis skin biopsies by high-throughput proteomic approaches. Arthritis Res Ther. (2020) 22:107. doi: 10.1186/s13075-020-02196-x
61. Skaug B, Assassi S. Type I interferon dysregulation in systemic sclerosis. Cytokine. (2020) 132:154635. doi: 10.1016/j.cyto.2018.12.018
62. Brkic Z, van Bon L, Cossu M, van Helden-Meeuwsen CG, Vonk MC, Knaapen H, et al. The interferon type I signature is present in systemic sclerosis before overt fibrosis and might contribute to its pathogenesis through high BAFF gene expression and high collagen synthesis. Ann Rheum Dis. (2016) 75:1567–73. doi: 10.1136/annrheumdis-2015-207392
63. Assassi S, Mayes MD, Arnett FC, et al. Systemic sclerosis and lupus: points in an interferon-mediated continuum. Arthritis Rheum. (2010) 62:589–98. doi: 10.1002/art.27224
64. Liu X, Mayes MD, Tan FK, Wu M, Reveille JD, Harper BE, et al. Correlation of interferon-inducible chemokine plasma levels with disease severity in systemic sclerosis. Arthritis Rheum. (2013) 65:226–35. doi: 10.1002/art.37742
65. Wu M, Assassi S. The role of type 1 interferon in systemic sclerosis. Front Immunol. (2013) 4:266. doi: 10.3389/fimmu.2013.00266
66. Guo X, Higgs BW, Bay-Jensen AC, Karsdal MA, Yao Y, Roskos LK, et al. Suppression of T cell activation and collagen accumulation by an anti-IFNAR1 mAb, anifrolumab, in adult patients with systemic sclerosis. J Invest Dermatol. (2015) 135:2402–9. doi: 10.1038/jid.2015.188
67. Goldberg A, Geppert T, Schiopu E, Frech T, Hsu V, Simms RW, et al. Dose-escalation of human anti-interferon-α receptor monoclonal antibody MEDI-546 in subjects with systemic sclerosis: a phase 1, multicenter, open label study. Arthritis Res Ther. (2014) 16:R57. doi: 10.1186/ar4492
68. Paleja B, Low AHL, Kumar P, Saidin S, Lajam A, Nur Hazirah S, et al. Systemic sclerosis perturbs the architecture of the immunome. Front Immunol. (2020) 11:1602. doi: 10.3389/fimmu.2020.01602
69. Yeo JG, Wasser M, Kumar P, Pan L, Poh SL, Ally F. et al. The Extended Polydimensional Immunome Characterization (EPIC) web-based reference and discovery tool for cytometry data. Nat Biotechnol. (2020) 38:679–84. doi: 10.1038/s41587-020-0532-1
70. Tan TC, Noviani M, Leung YY, Low AHL. The microbiome and systemic sclerosis: a review of current evidence. Best Pract Res Clin Rheumatol. (2021) 35:101687. doi: 10.1016/j.berh.2021.101687
71. Bellocchi C, Fernández-Ochoa Á, Montanelli G, Vigone B, Santaniello A, Milani C, et al. Microbial and metabolic multi-omic correlations in systemic sclerosis patients. Ann N Y Acad Sci. (2018) 1421:97–109. doi: 10.1111/nyas.13736
72. Braun-Moscovici Y, Ben Simon S, Dolnikov K, Giryes S, Markovits D, Tavor Y, et al. THU0340 duration and systemic sclerosis subtype are associated with different gut microbiome profiles. Ann Rheum Dis. (2020) 79:401. doi: 10.1136/annrheumdis-2020-eular.4865
73. Andréasson K, Alrawi Z, Persson A, Jönsson G, Marsal J. Intestinal dysbiosis is common in systemic sclerosis and associated with gastrointestinal and extraintestinal features of disease. Arthritis Res Ther. (2016) 18:278. doi: 10.1186/s13075-016-1182-z
74. Marighela TF, Arismendi MI, Marvulle V, Brunialti MKC, Salomão R, Kayser C. Effect of probiotics on gastrointestinal symptoms and immune parameters in systemic sclerosis: a randomized placebo-controlled trial. Rheumatology (Oxford). (2019) 58:1985–90. doi: 10.1093/rheumatology/kez160
75. Low AHL, Teng GG, Pettersson S, de Sessions PF, Ho EXP, Fan Q, et al. A double-blind randomized placebo-controlled trial of probiotics in systemic sclerosis associated gastrointestinal disease. Semin Arthritis Rheum. (2019) 49:411–9. doi: 10.1016/j.semarthrit.2019.05.006
76. Fretheim H, Chung BK, Didriksen H, Bækkevold ES, Midtvedt Ø, Brunborg C, et al. Fecal microbiota transplantation in systemic sclerosis: A double-blind, placebo-controlled randomized pilot trial. PLoS ONE. (2020) 15:e0232739. doi: 10.1371/journal.pone.0232739
77. Mahoney JM, Taroni J, Martyanov V, Wood TA, Greene CS, Pioli PA, et al. Systems level analysis of systemic sclerosis shows a network of immune and profibrotic pathways connected with genetic polymorphisms. PLoS Comput Biol. (2015) 11:e1004005. doi: 10.1371/journal.pcbi.1004005
Keywords: systemic sclerosis, stratification, precision medicine, molecular, multi-omic analyses
Citation: Noviani M, Chellamuthu VR, Albani S and Low AHL (2022) Toward Molecular Stratification and Precision Medicine in Systemic Sclerosis. Front. Med. 9:911977. doi: 10.3389/fmed.2022.911977
Received: 03 April 2022; Accepted: 06 June 2022;
Published: 30 June 2022.
Edited by:
Ashish Jacob Mathew, Christian Medical College and Hospital, IndiaReviewed by:
Ramya Janardana, SJNAHS, IndiaCopyright © 2022 Noviani, Chellamuthu, Albani and Low. This is an open-access article distributed under the terms of the Creative Commons Attribution License (CC BY). The use, distribution or reproduction in other forums is permitted, provided the original author(s) and the copyright owner(s) are credited and that the original publication in this journal is cited, in accordance with accepted academic practice. No use, distribution or reproduction is permitted which does not comply with these terms.
*Correspondence: Andrea Hsiu Ling Low, YW5kcmVhLmxvdy5oLmxAc2luZ2hlYWx0aC5jb20uc2c=
†These authors have contributed equally to this work and share senior authorship
Disclaimer: All claims expressed in this article are solely those of the authors and do not necessarily represent those of their affiliated organizations, or those of the publisher, the editors and the reviewers. Any product that may be evaluated in this article or claim that may be made by its manufacturer is not guaranteed or endorsed by the publisher.
Research integrity at Frontiers
Learn more about the work of our research integrity team to safeguard the quality of each article we publish.