- 1Sanofi S.A., Framingham, MA, United States
- 2American Thrombosis and Hemostasis Network, Rochester, NY, United States
- 3Bioverativ, a Sanofi Company, Waltham, MA, United States
- 4Sanofi S.A., Cambridge, MA, United States
Introduction: Up to 30% of individuals with hemophilia A develop inhibitors to replacement factor VIII (FVIII), rendering the treatment ineffective. The underlying mechanism of inhibitor development remains poorly understood. The My Life, Our Future Research Repository (MLOF RR) has gathered F8 and F9 mutational information, phenotypic data, and biological material from over 11,000 participants with hemophilia A (HA) and B as well as carriers enrolled across US hemophilia treatment centers, including over 5,000 whole-genome sequences. Identifying genes associated with inhibitors may contribute to our understanding of why certain patients develop those neutralizing antibodies.
Aim and Methods: Here, we performed a genome-wide association study and gene-based analyses to identify genes associated with inhibitors in participants with HA from the MLOF RR.
Results: We identify a genome-wide significant association within the human leukocyte antigen (HLA) locus in participants with HA with F8 intronic inversions. HLA typing revealed independent associations with the HLA alleles major histocompatibility complex, class II, DR beta 1 (HLA DRB1*15:01) and major histocompatibility complex, class II, DQ beta 1 (DQB1*03:03). Variant aggregation tests further identified low-frequency variants within GRID2IP (glutamate receptor, ionotropic, delta 2 [GRID2] interacting protein 1) significantly associated with inhibitors.
Conclusion: Overall, our study confirms the association of DRB1*15:01 with FVIII inhibitors and identifies a novel association of DQB1*03:03 in individuals with HA carrying intronic inversions of F8. In addition, our results implicate GRID2IP, encoding GRID2-interacting protein, with the development of inhibitors, and suggest an unrecognized role of this gene in autoimmunity.
Introduction
Inhibitor development against factor VIII (FVIII) is the most serious complication of replacement factor therapy. Thirty percent of individuals with severe hemophilia A (HA) receiving factor replacement therapy develop inhibitors, rendering the treatment ineffective (1). The underlying basis for why some individuals develop inhibitors while others do not remains poorly understood, but risk factors include ethnic background, family history, and F8 variant, suggesting that genetics is an important contributor (2–6). Individuals with HA with intronic inversions, large structural variants, or nonsense variants are 7–10 times more likely to develop inhibitors compared with those with milder mutations (4). Individuals with missense variants have the lowest incidence of inhibitors (<10%) presumably because they synthesize FVIII polypeptides that can induce tolerance (5). The intron 22 inversion accounts for up to 50% of mutations among individuals with severe HA and results in a truncated F8 with inverted intron 1 through 22 (4, 7). Individuals with inhibitors are classified as low or high responders if their inhibitor titers are below or higher than 5 Bethesda units per mL (BU/mL), respectively. Individuals with high-titer inhibitors are less likely to respond to immune tolerance induction (ITI) (8). Therefore, the genetic risk factors contributing to low or high inhibitors may differ and may be dependent on the underlying pathogenic F8 variant.
Genetic studies have been performed to investigate mechanisms and biomarkers of inhibitor development in individuals with HA, and have implicated variants in genes including MAPK9, CD86, HMOX1, FCGR2A, IL2, IL10, TNF, LTA, and CTLA4 (9–22). The largest study included over 13,000 single-nucleotide polymorphisms from 1,081 genes in 833 subjects (22). In addition, human leukocyte antigen (HLA) alleles have been associated with inhibitor risk, including major histocompatibility complex, class II, DR beta 1 (HLA DRB1*15:01) and major histocompatibility complex, class II, DQ beta 1 (HLA DQB1*06:02) (17, 19, 23–25).
Genome-wide association studies (GWAS) have become more widely used as an approach to uncover the etiology of diseases. They have some limitations, however, as they require a large sample size and may miss rare variants. In cases where multiple rare variants with small effects are likely to contribute to the disease, whole exome sequencing and gene burden analysis have been used to provide insights into clinical phenotype (26).
The My Life, Our Future Research Repository (MLOF RR) has gathered F8 and F9 mutational information, phenotypic data, and biological material from over 11,000 participants with hemophilia A and B as well as carriers enrolled across US hemophilia treatment centers, and whole-genome sequencing of over 5,000 genomes (27, 28).
Here, GWAS and gene-based analyses were performed in participants with HA from the MLOF RR to identify genes associated with inhibitor development.
Materials and Methods
My Life, Our Future Study
The MLOF program consists of a collaboration between the American Thrombosis and Hemostasis Network (ATHN), National Hemophilia Foundation, and Bloodworks Northwest, with funding from Bioverativ, a Sanofi company (27). Informed consent was obtained for inclusion of data and biological samples from a subset of participants who were chosen for whole-genome sequencing (WGS). The MLOF study included participants with hemophilia of all types and severities, and whether a causative F8 or F9 variant was identified or not. In this study, only the subset of participants with HA were considered. Phenotypic and demographic data, including self-reported race and ethnicity, were provided by ATHN and were collected across hemophilia treatment centers around the United States.
Whole-Genome Sequencing
WGS of samples was conducted as part of TOPMed (Trans-Omics for Precision Medicine),1 and data collection, processing, and quality control are described elsewhere (27, 29). WGS data were obtained through the database of Genotypes and Phenotypes (dbGaP, phs001515.v1.p1) as a processed Variant Call Format file as well as mapped reads (compressed alignment files). Variants that did not pass quality control or those that were monomorphic were filtered out within the MLOF RR cohort, and 119,015,152 variants remained. Samples duplicated or with sex mismatch were removed (N = 10). We produced a genetic relationship matrix using the PLINK 2.0-make-king option (30) and estimated principal components (PCs) using PC-AiR (31). We generated a final genetic relationship matrix using PC-Relate and the PCs from PC-AiR to account for population structure (32).
Inhibitor Definition
We defined participants with HA as having a history of inhibitors (cases) if they had active inhibitors and currently prescribed bypassing agents or ITI, or if they had a previous history of inhibitors and were not currently prescribed bypassing agents or ITI. We defined controls (participants with HA without inhibitors) as having no history of usage of bypassing agents or ITI and status reported as “no history of inhibitors,” or if presence of inhibitors was ruled out. Participants with high-titer inhibitors had a peak inhibitor titer of >5 BU/mL as measured by the Bethesda inhibitor assay. The low-titer inhibitors group was not defined because we could not discriminate between low-titer participants or participants with missing or non-exhaustive BU measures. Participants with unknown inhibitor status were excluded.
Cohort Definitions
Given the difference in inhibitor prevalence between ethnic groups (3, 6), and to reduce confounding due to population structure, we separated the MLOF RR cohort by genetic ancestry. For each subgroup, we excluded participants if any of their first 2 genetic PCs were >3 standard deviations away from the group mean. The largest subgroup was individuals who reported “White” (European) ethnicity and not “Hispanic, Latino/a, or Spanish origin” (N = 2,246), and we performed discovery analyses on this subset of individuals. We attempted replication of significant associations in the 2 other largest subgroups from this dataset, namely “Black or African American” (N = 310) and “Hispanic, Latino/a, or Spanish origin” (N = 488), excluding participants with mild hemophilia A in the replication cohorts due to small sample size. We restricted all analyses to male participants with HA because only 1 female participant with genetic data had a history of inhibitors. Total numbers of cases and controls included are reported in Table 1.
Single-Variant Analysis
We performed single-variant association tests on inhibitor status as a binary phenotype using logistic mixed-model score tests implemented in the R (3.6.1) package generalized linear mixed-model association tests (GMMAT) (v1.2.0) (33), adjusting for age, F8 variant, hemophilia A severity, sequencing center, and the first 3 PCs. Pathogenic F8 variant types were defined as large inversions, large structural variants [>50 base pairs (bp)], splice-site, nonsense, frameshift, and other [small (<50 bp) in-frame, synonymous, missense, and untranslated region variants]. Disease severity was defined as mild (>5 to <50% FVIII), moderate (1 to <5% FVIII), or severe (<1% FVIII) hemophilia A. We also performed association tests restricted to the subset of participants with intronic inversions using GMMAT, adjusting for age, sequencing center, and the first 3 PCs. The genetic relationship matrix estimated from PC-related was used as input for GMMAT. Analyses were restricted to variants with a minor allele frequency (MAF) >1% and ≥5 expected variant counts in cases. Variants with a P value <5 × 10–8 were considered statistically significant. We performed association tests for three different phenotype definitions: (1) all-titer inhibitors, (2) high-titer inhibitors (BU > 5), and (3) all-titer inhibitors restricted to participants with intronic inversions. All three case definitions were compared to non-inhibitor controls, restricting to participants with HA with intronic inversion in analysis 3. We generated locus plots using LocusZoom (34).
Replication and Meta-Analysis (Single Variant)
As the score test does not provide effect size estimates, we reanalyzed all variants With P < 1 × 10−7 in the European-ancestry discovery analysis using Wald tests implemented in GMMAT, which provides effect size estimates and standard errors. Logistic mixed models were adjusted for covariates. Then, we attempted to replicate those associations in the replication (i.e., non-European ancestry) cohorts using Wald tests, adjusting for F8 mutation type, sequencing center, age, and the first 3 PCs. Given the smaller sample sizes of non-European ancestry cohorts, mutation type was more broadly defined as presence or absence of large F8 structural variants or intronic inversions in those cohorts.
We used inverse variance meta-analysis as implemented in the R package meta to combine results for the discovery and replication analyses (35).
Variant Aggregation Analysis
We conducted gene-based analyses using variant-set mixed-model association tests (SMMAT) (36) and restricted the analysis to variants with an MAF < 5% and annotated as missense or predicted to have a high impact on protein-coding genes as defined using SnpEff 4.1 (37). We only considered genes with ≥ 3 polymorphic variants and an aggregated expected minor allele count in cases ≥ 5. Gene-based tests were adjusted for covariates as in the single-variant analysis.
Replication and Meta-Analysis (Variant Aggregation Analysis)
Replication tests of genes significantly associated with inhibitors in the discovery analysis were attempted in the replication cohorts using SMMAT and meta analyzed each cohort using smmat.meta included in the GMMAT package, including covariates, as described above.
Human Leukocyte Antigen Typing
We typed HLA alleles based on the WGS data using HLA*LA (bioconda v1.0) (38) and performed 4-digit HLA allele association analysis in R by logistic regression, adjusting for covariates as in the single-variant analysis. We performed HLA allele association for all participants with inhibitors and a subgroup of participants with intronic inversion. Each HLA allele was tested separately based on the presence or absence of the allele. HLA alleles with a Bonferroni-corrected P value < 0.05 were significant. We attempted to replicate significant association in the non-European cohorts. For participants with an intronic inversion, we further tested each HLA allele after conditioning on the presence or absence of DRB1*15:01.
FinnGen Phenome-Wide Association Study
We retrieved genetic associations for GRID2IP (glutamate receptor, ionotropic, delta 2 [GRID2] interacting protein 1) in the FinnGen dataset through the FinnGen PheWeb portal (http://r6.finngen.fi, release 6). We report all associations with P < 1 × 10–4.
Expression Quantitative Trait Loci (eQTL) and Gene Expression
We retrieved expression quantitative trait loci (eQTL) for IGLV (immunoglobulin lambda variable) cluster genes from the Genotype-Tissue Expression portal (version 8). Gene expression of GRID2IP was assessed in the European Bioinformatic Institute (EBI) Expression Atlas through the Open Targets portal (39). We also assessed colocalization between GWAS and eQTL studies through the Open Targets Genetics portal.2
Association With Clinical Phenotypes
We tested the association between significant variants identified in the association analyses and the following clinical phenotypes: age at inhibitor diagnosis, peak inhibitor titer, number of bleeds in the last 3 years, successful ITI, and length of ITI treatment. We tested the association between quantitative and binary phenotypes using linear and logistic regression respectively, adjusting for age, genetic ancestry, hemophilia A severity, and F8 variant type. Quantitative phenotypes were log-transformed prior to association testing.
Results
Genome-Wide Association Study of Inhibitors in Participants With HA
We first performed single-variant GWAS of participants with inhibitors in the MLOF RR using logistic mixed models implemented in GMMAT, focusing on participants of European ancestry, the largest subgroup (Supplementary Table 1). No variant reached genome-wide significance (Figure 1A and Supplementary Figure 1). The most significant variant was rs62158559 (P = 7.6 × 10–8), downstream of FOXI3. We tested the association of all variants with an association P value <1 × 10–7 with inhibitors in independent MLOF RR subgroups of Black or African American and Hispanic, Latino/a, or Spanish origin using Wald tests and performed inverse variance meta-analysis (Supplementary Table 2). No variant reached significance.
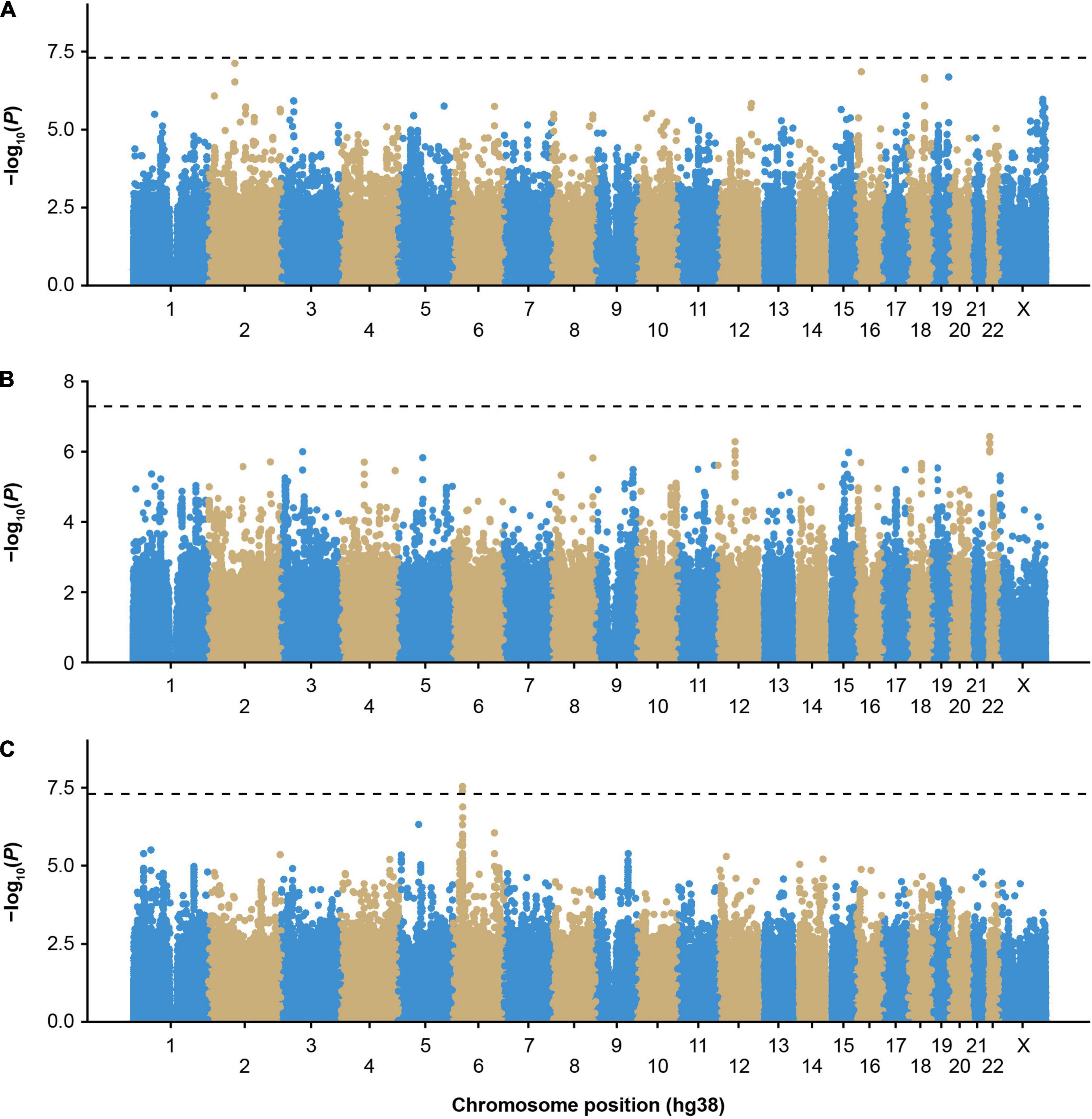
Figure 1. Genome-wide association study of inhibitors in European participants of the MLOF study. (A) Participants with all-titer inhibitor hemophilia A (N = 306 cases, N = 1,699 controls), (B) high-titer inhibitor participants (N = 110 cases, N = 1,699 controls), and (C) participants with all-titer inhibitor hemophilia A with F8 intronic inversions (N = 142 cases, N = 411 controls). Colors distinguish the different chromosomes. P-values were calculated by performing score tests using GMMAT after fitting logistic mixed models to account for population structure and relatedness and adjusting for age, sequencing center, F8 mutation type, severity, and the first 3 genetic principal components. P-values < 5 × 10–8 were considered significant. GMMAT, generalized linear mixed-model association tests; hg, human genome; MLOF, My Life, Our Future.
To reduce heterogeneity that may be due to differing genetic risk factors between participants with low- (≤5 BU) or high-titer inhibitors (>5 BU), we performed a GWAS including only high-titer inhibitor participants (Figure 1B and Supplementary Figure 2), and no loci reached genome-wide significance (P < 5 × 10–8). However, we detected an association trend for a cluster of variants in linkage disequilibrium (cut-off: r2 > 0.8) near the chromosome 22 IGLV cluster (Supplementary Figures 3, 4 and Supplementary Table 2). The top variant, rs5756720 (P = 3.6 × 10–7), has low frequency in European ancestry participants (MAF = 2.3%) but reached a frequency of 8% in high-titer inhibitor participants.
The HLA region has previously been implicated in inhibitor formation (17–19, 23–25). We hypothesized that different HLA alleles, as well as other genetic risk factors, may be implicated in the formation of inhibitors depending on the underlying F8 variant. Inversions were one of the most frequent mutation types observed in MLOF (27%–40% of all participants), consistent with that of common hemophilia A variants (4, 7, 40). Therefore, we performed a GWAS of inhibitors (all titers) restricting to participants with intronic inversions (Figure 1C and Supplementary Figure 5) and observed a significant association for variant Chr6:32438468_CA/C (P = 4.1 × 10–8), located near HLA-DRA (Figure 2A), which we replicated in the Black or African American subgroup (P = 0.040) (Figure 2B). Participants with intronic inversions carrying the CA allele had an increased inhibitor risk (meta-analysis odds ratio [OR] = 2.3 [1.7–3.0]) compared to those with the C allele.
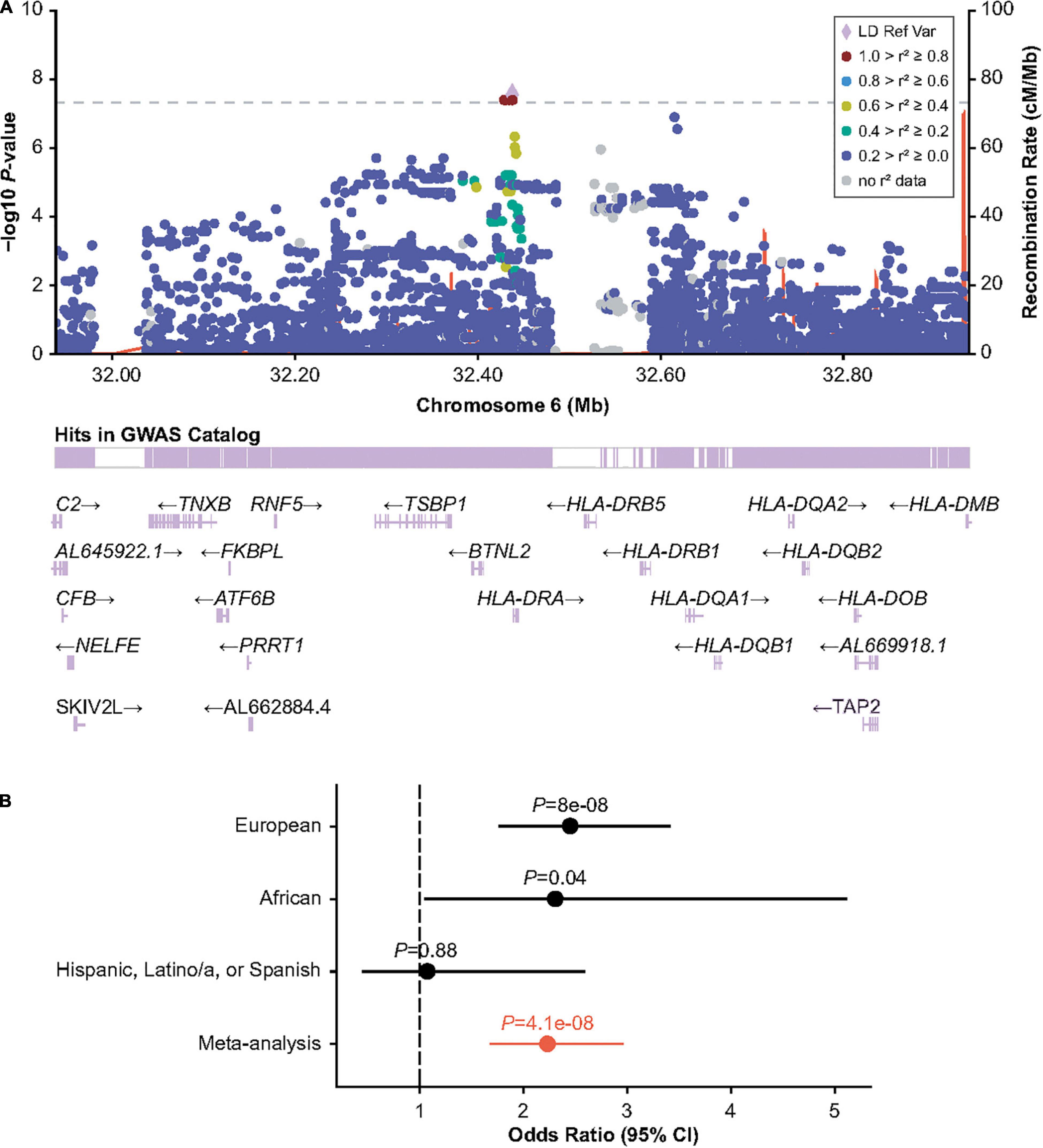
Figure 2. Association of human leukocyte antigen (HLA) region variant 6:32438468_CA/C with inhibitors in participants with HA with intronic F8 inversions. (A) LocusZoom plot showing GMMAT score test P-value within the HLA region. (B) Wald-test P-value and odds ratios for the discovery (European ancestry) analysis, and replication analyses in individuals of Black or African American and Hispanic, Latino/a, or Spanish origin. Odds ratios are calculated for the CA allele. Meta-analysis odds ratio and P values were calculated by inverse variance meta-analysis. CI, confidence interval; GMMAT, generalized linear mixed-model association tests; GWAS, genome-wide association study; LD Ref Var, linkage disequilibrium reference variant; HA, hemophilia A.
DRB1*15:01 and DQB1*03:03 Are Associated With Inhibitors in Participants With HA
To understand which HLA gene was implicated in inhibitor formation, we typed HLA alleles using HLA*LA (38). Presence of the DRB1*15:01 allele was significantly associated with increased inhibitor risk after multiple testing correction (European ancestry P = 1.7 × 10–4, meta-analysis P = 5.6 × 10–5) (Table 2; Supplementary Tables 4–6). The association with DRB1*15:01 was slightly stronger when stratifying for participants with F8 inversions (European P = 2.0 × 10–5, meta-analysis P = 2.8 × 10–5). DQB1*06:02 was also significantly associated with increased inhibitors within this subgroup (European ancestry P = 7.5 × 10–5, meta-analysis P = 8.3 × 10–5) but was not significant after conditioning for DRB1*15:01 in participants with intronic inversions (P > 0.05). Interestingly, we detected a secondary independent association with DQB1*03:03 after conditioning on DRB1*15:01 (P = 3.1 × 10–4) (Table 2).
Low-Frequency Variants in GRID2IP Are Associated With High-Titer Inhibitors
We performed variant aggregation tests using the efficient hybrid burden and Sequence Kernel Association Test (SKAT) implemented in SMMAT (36) to assess whether aggregating low-frequency and rare variants (MAF < 0.05) could reveal additional associations. We first tested whether we could capture the effect of F8 mutations on inhibitor status by gene burden tests by omitting F8 mutation type or hemophilia A severity in the regression model. F8 mutations were associated with inhibitors (burden P = 1.6 × 10–6, efficient test P = 1.0 × 10–5) (Supplementary Table 6); small F8 mutations were protective against inhibitors, reflecting that the participants carrying those variants are less likely to develop inhibitors compared with participants with large structural variants (Supplementary Table 7).
We next assessed whether other genes were associated with inhibitors after controlling for F8 mutation type and severity. We identified a significant association for ZPBP2 with all-titer inhibitors, and ITGB4, GRID2IP, and RGS16 with high-titer inhibitors (Table 3; Figure 3; and Supplementary Tables 3, 4, 8–10). Of these 4 genes, the association with GRID2IP was replicated in Black or African American participants (P = 0.0078, meta-analysis P = 1.6 × 10–8). The strongest single-variant association within GRID2IP was observed for rs61732374 (Chr7:6510953_G_A, Supplementary Table 11). The rs61732374-A allele was associated with increased inhibitor risk (MAF = 2%, single-variant, Wald P = 2.1 × 10–5, OR [95% confidence interval (CI)] = 5.2 [2.4–11.0]). The same variant was nominally associated with inhibitors in Black or African American participants (MAF = 1%, Wald P = 0.0092, OR [95% CI] = 32.2 [2.4–439.7]; meta-analysis P = 1.5 × 10–6) but was monomorphic in individuals of Hispanic, Latino/a, or Spanish origin. rs61732374 encodes a missense variant changing arginine 504 to a cysteine.
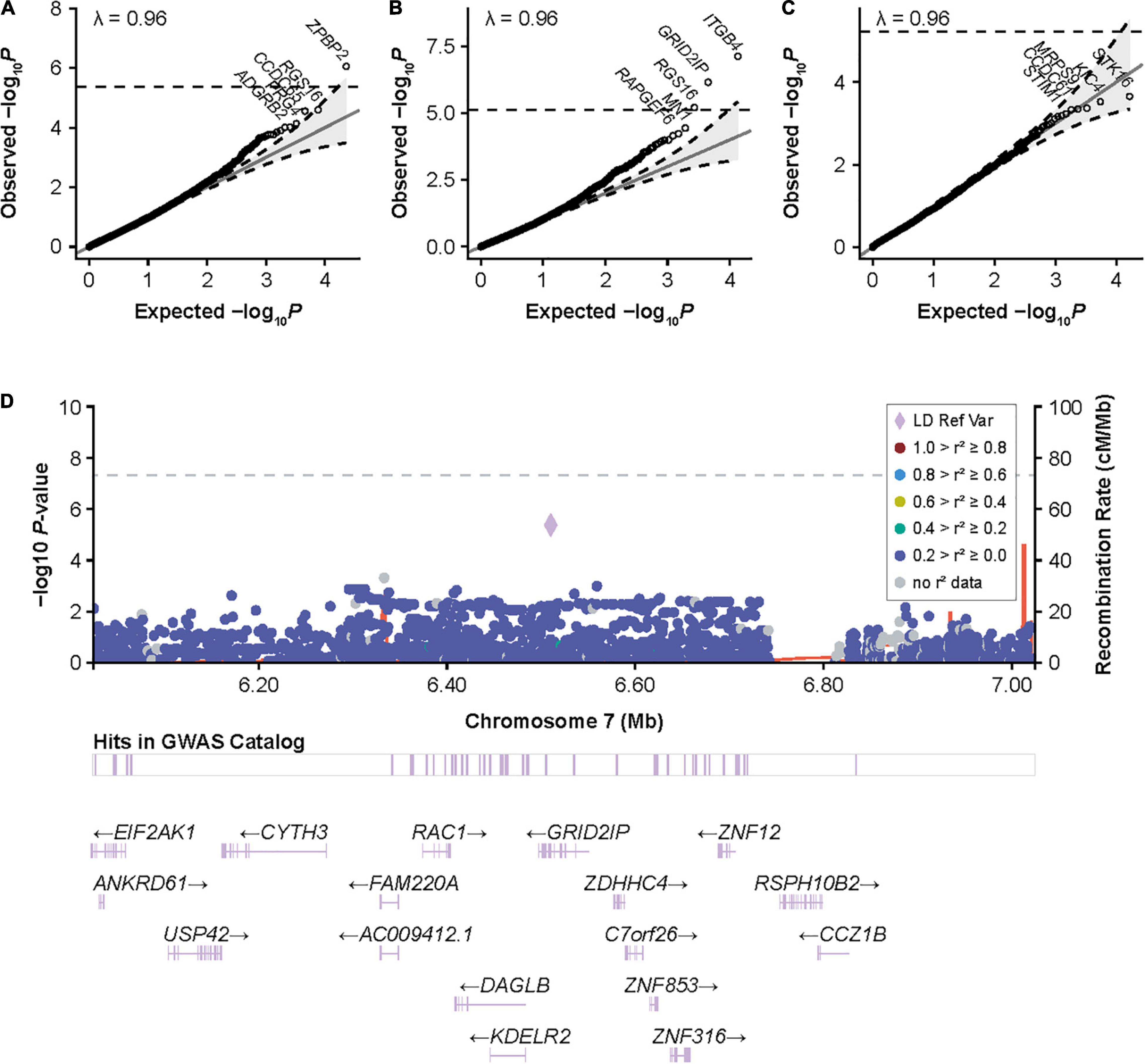
Figure 3. Gene-based associations with inhibitors and high-titer inhibitors in the European discovery cohort and around GRID2IP in participants with HA. (A) Quantile-quantile (QQ) plots for inhibitor status, (B) high-titer inhibitor status, and (C) inhibitor status in patients with intronic F8 inversions in the European discovery cohort for gene-based variant aggregation tests. Association P values were calculated using logistic mixed-model score tests using variant-set mixed-model association tests (SMMAT), adjusting for age, sequencing center, F8 mutation type, hemophilia A severity, and the first 3 principal components. (D) LocusZoom plot reporting single-variant generalized linear mixed-model association tests (GMMAT) score test P values of high-titer inhibitors in participants with hemophilia A around GRID2IP. Logistic mixed models were adjusted for age, sequencing center, F8 mutation type, hemophilia A severity, and the first three principal components. In contrast to the main single-variant analysis with high-titer inhibitor, all variants with an allele frequency >1% were included to show the association with the top coding GRID2IP variant included in variant aggregation tests (rs61732374, P = 4 × 10–6). GRID2IP, glutamate receptor, ionotropic, delta 2 [GRID2] interacting protein 1; GWAS, genome-wide association study; LD Ref Var, linkage disequilibrium reference variant; HA, hemophilia A.
Association With Clinical Phenotypes
We investigated whether DRB1*15:01, DQB1*03:03, and the GRID2IP rs61732374 missense variant were associated with additional clinical phenotypes in MLOF participants, including number of bleeds, peak inhibitor titers, and age at inhibitor diagnosis. We did not observe a significant association between the 2 HLA alleles and number of bleeds, inhibitor titers, or age at inhibitor diagnosis (P > 0.05). However, inhibitor-positive participants carrying the rs61732374-A allele displayed increased inhibitor titers (P = 0.017, Figure 4). In addition, 162 participants underwent ITI. We tested whether these genotypes could influence ITI success or length of treatment but did not identify significant associations (P > 0.05).
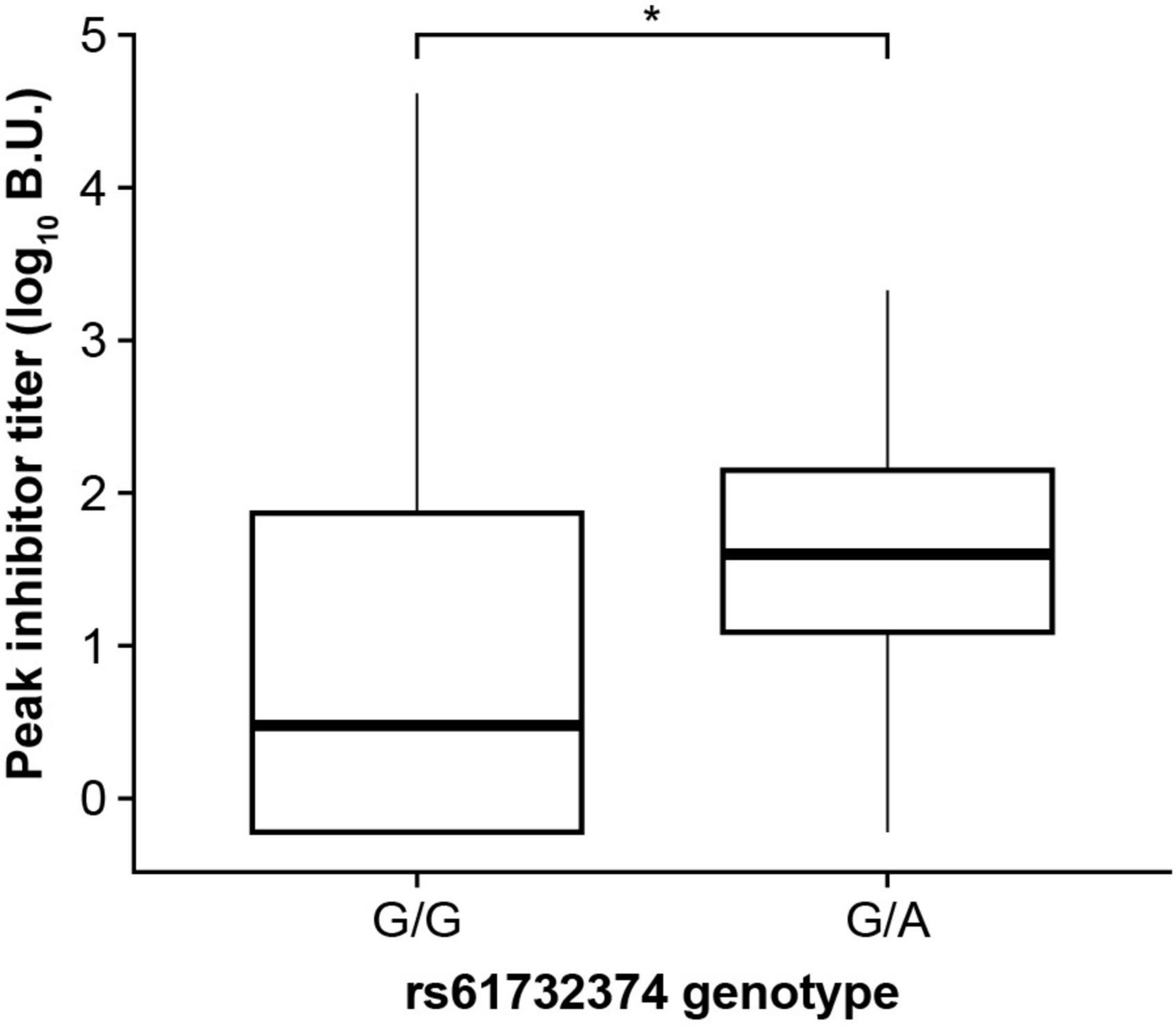
Figure 4. The GRID2IP rs61732374-A allele is associated with increased peak inhibitor titer. Significance was evaluated using a linear regression model adjusting for age, hemophilia A severity, F8 mutation type, and genetic ancestry group. Inhibitor titers were log transformed prior to association testing. The analysis included black or African American as well as European ancestry participants with history of inhibitors (current or past) and available peak inhibitor titer information (N = 310). The analysis could not be performed in the Hispanic, Latino/a, or Spanish group as the A-allele was not observed in this population. BU, Bethesda Units. * P < 0.05.
Discussion
We performed a large-scale GWAS of FVIII inhibitors in the MLOF RR cohort in participants who underwent WGS. Despite comprehensive genotyping, no variant reached genome-wide significance when including all participants with HA, which suggests that our study may be underpowered to detect associations due to the relatively low number of inhibitor cases and/or disease heterogeneity. The latter is supported by the detection of significant association of a variant within the HLA region with inhibitors in participants carrying intronic inversions.
To understand which HLA alleles may contribute to inhibitor risk, we typed and performed an association analysis of HLA alleles at 4-digit resolution. We identified an association between the presence of DRB1*15:01 and increased risk of inhibitors. This association was stronger in participants with intronic inversions, in whom we identified a secondary independent association with DQB1*03:03. DRB1*15:01 has been previously identified as associated with inhibitors, particularly in participants with intron 22 inversions (23). To our knowledge, we report for the first time an association with DQB1*03:03, which was only detectable after conditioning on DRB1*15:01. In addition to increased sample size, this may partly explain why association with this allele was not detected in previous studies (23, 24, 41, 42). Nonetheless, this result will require replication in independent studies.
HLA genes identified in this study encode major histocompatibility complex class II molecules, which may contribute to inhibitor formation through presentation of F8 peptides to CD4 T-helper cells by antigen-presenting cells. In addition, our results suggest that HLA alleles may modulate inhibitor risk (5) differently depending on the underlying F8 mutation. Different F8 missense variants, such as p.Arg612Cys and p.Arg2169His, are more likely to lead to inhibitor development in part because they lead to higher levels of novel binding between treatment FVIII peptides and HLA-DRB1 (43). Similar mechanisms may be at play for FVIII inversions, although individuals with HA with the intron-22 inversion may be partly tolerized to FVIII, as the full FVIII sequence remains expressed intracellularly as 2 non-secreted polypeptides (44).
The HLA variant DPB1*02:02 has been previously identified as a risk factor for inhibitor development in the MLOF study (45). The variant was not included in our analysis as its frequency was under our inclusion threshold (<1%). Outside the HLA region, we also detected a potential association with variants within the chromosome 22 IGLV cluster. The IGLV genes encode the variable region of immunoglobulins, which defines the antigen-binding site. Therefore, it is plausible that variants that modulate IGLV genes outside their coding sequence may influence the risk of developing neutralizing autoantibodies, although we only detected colocalizing eQTLs for IGLV pseudogenes (Supplementary Figure 4).
Finally, variant aggregation tests identified an association of rare variants in ZPBP2 with inhibitors, and ITGB4, GRID2IP, and RGS16 with high-titer inhibitors. Both ZPBP2 and GRID2IP are part of loci that have been previously associated with autoimmune disease, including asthma and inflammatory bowel diseases (IBDs) (46–52). This suggests that inhibitor risk may be modulated by common loci associated with inhibitors. It is possible that the associations detected in this study are capturing the effect of common nearby variants. However, the strongest association for inhibitors around GRID2IP was with the low-frequency coding variant rs61732374, in weak linkage disequilibrium with nearby variants (r2 < 0.2) (Supplementary Figure 3). In addition, all variants included in ZPBP2 were rare (MAF < 1%).
We observed some inflation at the tails of gene-based test quantile-quantile plots, particularly for the high-titer inhibitor analysis, although this was not reflected in the genomic control (λ = 0.96). This may be due to the imbalance between the number of case and control participants (6% high-titer inhibitor cases). Nevertheless, we were able to replicate the GRID2IP association in Black or African American participants. The association between inhibitors and GRID2IP variants was only observed in the high-titer inhibitor group. Consistently, we observed a significant association between the low-frequency GRID2IP missense variant, rs61732374 (Arg504Cys), and increased inhibitor titers at peak in participants with a history of inhibitors. Although our results suggest that variants in GRID2IP may increase the risk of developing high levels of inhibitors, we did not identify a significant impact on ITI outcomes in the subset of participants who underwent this treatment. GRID2IP encodes the glutamate receptor ionotropic delta 2 (GRID2) interacting protein, which is predominantly expressed in the brain and functions as a linker between GRID2 and actin at the parallel fiber-Purkinje cell synapse (53). GRID2IP expression is strongly upregulated in peripheral blood mononuclear cells of patients with vitiligo (54). Interestingly, another GRID2IP missense variant, rs184043502, was strongly associated with IBDs in the FinnGen biobank (P = 2 × 10–10) (Supplementary Table 12). In addition, significant association at the GRID2IP locus for irritable bowel disease and white blood cell counts strongly colocalized with GRID2IP blood eQTLs (posterior colocalization probability > 0.97) (39, 49, 55). Together, this suggests that GRID2IP may have an unrecognized role in autoimmunity.
Conclusion
In conclusion, GWAS and HLA typing revealed a significant association of variants in the HLA region, in particular HLA DRB1*15:01 and DQB1*03:03, with inhibitors in participants with F8 intronic inversions. Our results suggest that the underlying cause of FVIII inhibitors is likely heterogeneous and dependent on the underlying F8 pathogenic variant. Finally, we identified and replicated an association between low-frequency coding variants in GRID2IP and FVIII inhibitors, highlighting a potential role of the gene in anti-drug antibody development.
Data Availability Statement
The original contributions presented in this study are included in the article/supplementary material, further inquiries can be directed to the corresponding author.
Ethics Statement
The Coordinating Ethics Committee of the Hospital District of Helsinki and Uusimaa (HUS) approved the FinnGen study protocol Nr HUS/990/2017. MLOF phenotypic data were provided by ATHN under the WIRB Protocol #20122010 on a de-identified basis. The patients/participants provided their written informed consent to participate in this study.
Author Contributions
SL designed the study, carried out analyses, interpreted data, performed statistical analyses, and wrote the manuscript. CH analyzed data and performed statistical analyses. KK, DR, and JD designed the study, collected and interpreted data, and wrote the manuscript. JD, TH, and CL designed the original research plan. All authors provided critical revision of the manuscript and had final approval of the manuscript for publication.
Author Disclaimer
The content of this manuscript is solely the responsibility of the authors and does not necessarily represent the official views of the MLOF program or its partners.
Conflict of Interest
SL, DR, KK, and JD were employed by Sanofi, DR, KK, and JD hold shares and/or stock options in the company. CL was employed by Nimbus Therapeutics. TH was employed by Repertoire Immune Medicines.
The remaining author declares that the research was conducted in the absence of any commercial or financial relationships that could be construed as a potential conflict of interest.
This study was funded by Sanofi (Cambridge, MA, United States). The funder had the following involvement with the study: Sanofi (and Sobi) reviewed the manuscript and Sanofi funded editorial support, provided by Ashleigh Pulkoski-Gross, Ph.D., CMPP, and Sheila Longo, Ph.D., of JK Associates Inc., part of Fishawack Health. All authors declare no other competing interests. CL and TH are former employees of Sanofi.
Publisher’s Note
All claims expressed in this article are solely those of the authors and do not necessarily represent those of their affiliated organizations, or those of the publisher, the editors and the reviewers. Any product that may be evaluated in this article, or claim that may be made by its manufacturer, is not guaranteed or endorsed by the publisher.
Acknowledgments
We would like to thank Till Andlauer, Glenn Perce, Joe Salas, Robert Peters, Alexandra Hicks, Clément Chatelain, and Len Valentino for the productive discussions and feedback. We also thank Graham Neill for providing feedback and helping with the preparation of the manuscript. We would also like to thank all participants in the MLOF study, their families, and the hemophilia treatment center staff. The MLOF Research Repository is a collection of genetic samples and data developed through the collaboration of the American Thrombosis and Hemostasis Network, Bloodworks Northwest, and the National Hemophilia Foundation with support from Bioverativ (Sanofi) and under the leadership of the project’s Principal Investigator, Barbara Konkle. Phenotypic data is provided through the ATHNdataset, under the stewardship of the American Thrombosis and Hemostasis Network. The partners acknowledge the dedicated efforts of the hemophilia treatment centers and their patients for contributing to this Research Repository. Molecular data for the TOPMed program were supported by the National Heart, Lung, and Blood Institute (NHLBI). Genome Sequencing for “NHLBI TOPMed: MLOF” (phs001515) was performed at Baylor (HHSN268201600033I) and New York Genome Center (NYGC) Genomics (HHSN268201500016C). Core support including centralized genomic read mapping and genotype calling, along with variant quality metrics and filtering, was provided by the TOPMed Informatics Research Center (3R01HL-117626-02S1; contract HHSN268201800002I). Core support including phenotype harmonization, data management, sample-identity quality control, and general program coordination were provided by the TOPMed Data Coordinating Center (R01HL-120393; U01HL-120393; contract HHSN268201800001I). We gratefully acknowledge the studies and participants who provided biological samples and data for TOPMed. We acknowledge the FinnGen consortium for access to release 6 datasets used to perform phenome-wide association analyses. The FinnGen project is funded by 2 grants from Business Finland (HUS 4685/31/2016 and UH 4386/31/2016) and the following industry partners: AbbVie Inc., AstraZeneca UK Ltd, Biogen MA Inc., Celgene Corporation, Celgene International II Sàrl, Genentech Inc., Merck Sharp & Dohme Corp, Pfizer Inc., GlaxoSmithKline Intellectual Property Development Ltd., Sanofi US Services Inc., Maze Therapeutics Inc., Janssen Biotech Inc, and Novartis AG. The following biobanks are acknowledged for delivering biobank samples to FinnGen: Auria Biobank (www.auria.fi/biopankki), THL Biobank (www.thl.fi/biobank), Helsinki Biobank (www.helsinginbiopankki.fi), Biobank Borealis of Northern Finland (https://www.ppshp.fi/Tutkimus-ja-opetus/Biopankki/Pages/Biobank-Borealis-briefly-in-English.aspx), Finnish Clinical Biobank Tampere (www.tays.fi/en-US/Research_and_development/Finnish_Clinical_Biobank_Tampere), Biobank of Eastern Finland (www.ita-suomenbiopankki.fi/en), Central Finland Biobank (www.ksshp.fi/fi-FI/Potilaalle/Biopankki), Finnish Red Cross Blood Service Biobank (www.veripalvelu.fi/verenluovutus/biopankkitoiminta), and Terveystalo Biobank (www.terveystalo.com/fi/Yritystietoa/Terveystalo-Biopankki/Biopankki/). All Finnish Biobanks are members of BBMRI.fiinfrastructure(www.bbmri.fi). Finnish Biobank Cooperative-FINBB (https://finbb.fi/) is the coordinator of BBMRI-ERIC operations in Finland.
Supplementary Material
The Supplementary Material for this article can be found online at: https://www.frontiersin.org/articles/10.3389/fmed.2022.903838/full#supplementary-material
Footnotes
References
1. Astermark J. Inhibitor development: patient-determined risk factors. Haemophilia. (2010) 16:66–70. doi: 10.1111/j.1365-2516.2008.01923.x
2. Astermark J, Berntorp E, White GC, Kroner BL, Group MS. The Malmo international brother study (MIBS): further support for genetic predisposition to inhibitor development in hemophilia patients. Haemophilia. (2001) 7:267–72. doi: 10.1046/j.1365-2516.2001.00510.x
3. Viel KR, Ameri A, Abshire TC, Iyer RV, Watts RG, Lutcher C, et al. Inhibitors of factor VIII in black patients with hemophilia. N Engl J Med. (2009) 360:1618–27. doi: 10.1056/NEJMoa075760
4. Oldenburg J, El-Maarri O, Schwaab R. Inhibitor development in correlation to factor VIII genotypes. Haemophilia. (2002) 8(Suppl. 2):23–9. doi: 10.1046/j.1351-8216.2001.00134.x
5. Sauna ZE, Lozier JN, Kasper CK, Yanover C, Nichols T, Howard TE. The intron-22-inverted F8 locus permits factor VIII synthesis: explanation for low inhibitor risk and a role for pharmacogenomics. Blood. (2015) 125:223–8. doi: 10.1182/blood-2013-12-530113
6. Pratt KP. Higher incidence of neutralizing anti-FVIII antibodies (inhibitors) in black and hispanic American hemophilia A patients enrolled in the my life, our future programme. Blood. (2019) 134(Suppl. 1):901.
7. Eckhardt CL, van Velzen AS, Peters M, Astermark J, Brons PP, Castaman G, et al. Factor VIII gene (F8) mutation and risk of inhibitor development in nonsevere hemophilia A. Blood. (2013) 122:1954–62. doi: 10.1182/blood-2013-02-483263
8. Hay CR, DiMichele DM, International Immune Tolerance Study. The principal results of the international immune tolerance study: a randomized dose comparison. Blood. (2012) 119:1335–44. doi: 10.1182/blood-2011-08-369132
9. Eckhardt CL, Astermark J, Nagelkerke SQ, Geissler J, Tanck MW, Peters M, et al. The Fc gamma receptor IIa R131H polymorphism is associated with inhibitor development in severe hemophilia A. J Thromb Haemost. (2014) 12:1294–301. doi: 10.1111/jth.12631
10. Chaves D, Belisario A, Castro G, Santoro M, Rodrigues C. Analysis of cytokine genes polymorphism as markers for inhibitor development in haemophilia A. Int J Immunogenet. (2010) 37:79–82. doi: 10.1111/j.1744-313X.2009.00893.x
11. Gaitonde P, Peng A, Straubinger RM, Bankert RB, Balu-Iyer SV. Downregulation of CD40 signal and induction of TGF-beta by phosphatidylinositol mediates reduction in immunogenicity against recombinant human factor VIII. J Pharm Sci. (2012) 101:48–55. doi: 10.1002/jps.22746
12. Lozier JN, Rosenberg PS, Goedert JJ, Menashe I. A case-control study reveals immunoregulatory gene haplotypes that influence inhibitor risk in severe haemophilia A. Haemophilia. (2011) 17:641–9. doi: 10.1111/j.1365-2516.2010.02473.x
13. Lu Y, Ding Q, Dai J, Wang H, Wang X. Impact of polymorphisms in genes involved in autoimmune disease on inhibitor development in Chinese patients with haemophilia A. Thromb Haemost. (2012) 107:30–6. doi: 10.1160/TH11-06-0425
14. Astermark J, Oldenburg J, Carlson J, Pavlova A, Kavakli K, Berntorp E, et al. Polymorphisms in the TNFA gene and the risk of inhibitor development in patients with hemophilia A. Blood. (2006) 108:3739–45. doi: 10.1182/blood-2006-05-024711
15. Astermark J, Oldenburg J, Pavlova A, Berntorp E, Lefvert AK, Group MS. Polymorphisms in the IL10 but not in the IL1beta and IL4 genes are associated with inhibitor development in patients with hemophilia A. Blood. (2006) 107:3167–72. doi: 10.1182/blood-2005-09-3918
16. Astermark J, Wang X, Oldenburg J, Berntorp E, Lefvert AK, Group MS. Polymorphisms in the CTLA-4 gene and inhibitor development in patients with severe hemophilia A. J Thromb Haemost. (2007) 5:263–5. doi: 10.1111/j.1538-7836.2007.02290.x
17. Pavlova A, Delev D, Lacroix-Desmazes S, Schwaab R, Mende M, Fimmers R, et al. Impact of polymorphisms of the major histocompatibility complex class II, interleukin-10, tumor necrosis factor-alpha and cytotoxic T-lymphocyte antigen-4 genes on inhibitor development in severe hemophilia A. J Thromb Haemost. (2009) 7:2006–15. doi: 10.1111/j.1538-7836.2009.03636.x
18. Bachelet D, Albert T, Mbogning C, Hassler S, Zhang Y, Schultze-Strasser S, et al. Risk stratification integrating genetic data for factor VIII inhibitor development in patients with severe hemophilia A. PLoS One. (2019) 14:e0218258. doi: 10.1371/journal.pone.0218258
19. Pergantou H, Varela I, Moraloglou O, Economou M, Spanou K, Kapsimali Z, et al. Impact of HLA alleles and cytokine polymorphisms on inhibitors development in children with severe haemophilia A. Haemophilia. (2013) 19:706–10. doi: 10.1111/hae.12168
20. Pinto P, Ghosh K, Shetty S. Immune regulatory gene polymorphisms as predisposing risk factors for the development of factor VIII inhibitors in Indian severe haemophilia A patients. Haemophilia. (2012) 18:794–7. doi: 10.1111/j.1365-2516.2012.02845.x
21. Repesse Y, Peyron I, Dimitrov JD, Dasgupta S, Moshai EF, Costa C, et al. Development of inhibitory antibodies to therapeutic factor VIII in severe hemophilia A is associated with microsatellite polymorphisms in the HMOX1 promoter. Haematologica. (2013) 98:1650–5. doi: 10.3324/haematol.2013.084665
22. Astermark J, Donfield SM, Gomperts ED, Schwarz J, Menius ED, Pavlova A, et al. The polygenic nature of inhibitors in hemophilia A: results from the hemophilia inhibitor genetics study (HIGS) combined cohort. Blood. (2013) 121:1446–54. doi: 10.1182/blood-2012-06-434803
23. Oldenburg J, Picard JK, Schwaab R, Brackmann HH, Tuddenham EG, Simpson E. HLA genotype of patients with severe haemophilia A due to intron 22 inversion with and without inhibitors of factor VIII. Thromb Haemost. (1997) 77:238–42.
24. Hay CR, Ollier W, Pepper L, Cumming A, Keeney S, Goodeve AC, et al. HLA class II profile: a weak determinant of factor VIII inhibitor development in severe haemophilia A. UKHCDO inhibitor working party. Thromb Haemost. (1997) 77:234–7.
25. De Barros MF, Herrero JC, Sell AM, De Melo FC, Braga MA, Pelissari CB, et al. Influence of class I and II HLA alleles on inhibitor development in severe haemophilia A patients from the south of Brazil. Haemophilia. (2012) 18:e236–40. doi: 10.1111/j.1365-2516.2011.02604.x
26. Gonzaga-Jauregui C, Harel T, Gambin T, Kousi M, Griffin LB, Francescatto L, et al. Exome sequence analysis suggests that genetic burden contributes to phenotypic variability and complex neuropathy. Cell Rep. (2015) 12:1169–83. doi: 10.1016/j.celrep.2015.07.023
27. Konkle BA, Johnsen JM, Wheeler M, Watson C, Skinner M, Pierce GF, et al. Genotypes, phenotypes and whole genome sequence: approaches from the my life our future haemophilia project. Haemophilia. (2018) 24(Suppl. 6):87–94. doi: 10.1111/hae.13506
28. Johnsen JM, Fletcher SN, Huston H, Roberge S, Martin BK, Kircher M, et al. Novel approach to genetic analysis and results in 3000 hemophilia patients enrolled in the my life, our future initiative. Blood Adv. (2017) 1:824–34. doi: 10.1182/bloodadvances.2016002923
29. NHLBI Trans-Omics for Precision Medicine. TOPMed Whole Genome Sequencing Methods: Freeze 8. (2020). Available online at:https://www.nhlbiwgs.org/topmed-whole-genome-sequencing-methods-freeze-8 (accessed July 2, 2021).
30. Chang CC, Chow CC, Tellier LC, Vattikuti S, Purcell SM, Lee JJ. Second-generation PLINK: rising to the challenge of larger and richer datasets. Gigascience. (2015) 4:7. doi: 10.1186/s13742-015-0047-8
31. Conomos MP, Miller MB, Thornton TA. Robust inference of population structure for ancestry prediction and correction of stratification in the presence of relatedness. Genet Epidemiol. (2015) 39:276–93. doi: 10.1002/gepi.21896
32. Conomos MP, Reiner AP, Weir BS, Thornton TA. Model-free estimation of recent genetic relatedness. Am J Hum Genet. (2016) 98:127–48. doi: 10.1016/j.ajhg.2015.11.022
33. Chen H, Wang C, Conomos MP, Stilp AM, Li Z, Sofer T, et al. Control for population structure and relatedness for binary traits in genetic association studies via logistic mixed models. Am J Hum Genet. (2016) 98:653–66. doi: 10.1016/j.ajhg.2016.02.012
34. Pruim RJ, Welch RP, Sanna S, Teslovich TM, Chines PS, Gliedt TP, et al. LocusZoom: regional visualization of genome-wide association scan results. Bioinformatics. (2010) 26:2336–7. doi: 10.1093/bioinformatics/btq419
35. Balduzzi S, Rücker G, Schwarzer G. How to perform a meta-analysis with R: a practical tutorial. Evid Based Ment Health. (2019) 22:153–60. doi: 10.1136/ebmental-2019-300117
36. Chen H, Huffman JE, Brody JA, Wang C, Lee S, Li Z, et al. Efficient variant set mixed model association tests for continuous and binary traits in large-scale whole-genome sequencing studies. Am J Hum Genet. (2019) 104:260–74. doi: 10.1016/j.ajhg.2018.12.012
37. Cingolani P, Platts A, Wang le L, Coon M, Nguyen T, Wang L, et al. A program for annotating and predicting the effects of single nucleotide polymorphisms, SnpEff: SNPs in the genome of Drosophila melanogaster strain w1118; iso-2; iso-3. Fly (Austin). (2012) 6:80–92. doi: 10.4161/fly.19695
38. Dilthey AT, Mentzer AJ, Carapito R, Cutland C, Cereb N, Madhi SA, et al. HLA*LA-HLA typing from linearly projected graph alignments. Bioinformatics. (2019) 35:4394–6. doi: 10.1093/bioinformatics/btz235
39. Carvalho-Silva D, Pierleoni A, Pignatelli M, Ong C, Fumis L, Karamanis N, et al. Open targets platform: new developments and updates two years on. Nucleic Acids Res. (2019) 47:D1056–65. doi: 10.1093/nar/gky1133
40. Oldenburg J, Pavlova A. Genetic risk factors for inhibitors to factors VIII and IX. Haemophilia. (2006) 12(Suppl. 6):15–22. doi: 10.1111/j.1365-2516.2006.01361.x
41. Ohta H, Takahashi I, Kojima T, Takamatsu J, Shima M, Yoshioka A, et al. Histocompatibility antigens and alleles in Japanese haemophilia A patients with or without factor VIII antibodies. Tissue Antigens. (1999) 54:91–7. doi: 10.1034/j.1399-0039.1999.540111.x
42. Nathalang O, Sriwanitchrak P, Sasanakul W, Chuansumrit A. The association between HLA class II alleles and the occurrence of factor VIII inhibitor in Thai patients with hemophilia A. Turk J Haematol. (2012) 29:34–9. doi: 10.5505/tjh.2012.29795
43. Kempton CL, Payne AB. HLA-DRB1-factor VIII binding is a risk factor for inhibitor development in nonsevere hemophilia: a case-control study. Blood Adv. (2018) 2:1750–5. doi: 10.1182/bloodadvances.2018019323
44. Pandey GS, Yanover C, Miller-Jenkins LM, Garfield S, Cole SA, Curran JE, et al. Endogenous factor VIII synthesis from the intron 22-inverted F8 locus may modulate the immunogenicity of replacement therapy for hemophilia A. Nat Med. (2013) 19:1318–24. doi: 10.1038/nm.3270
45. McGill JR, Simhadri VL, Sauna ZE. HLA variants and inhibitor development in hemophilia A: a retrospective case-controlled study using the ATHNdataset. Front Med (Lausanne). (2021) 8:663396. doi: 10.3389/fmed.2021.663396
46. Miller M, Vuong C, Garcia MF, Rosenthal P, Das S, Weng N, et al. Does reduced zona pellucida binding protein 2 (ZPBP2) expression on chromosome 17q21 protect against asthma? J Allergy Clin Immunol. (2018) 142:706–9.e4. doi: 10.1016/j.jaci.2018.04.011
47. Ellinghaus D, Jostins L, Spain SL, Cortes A, Bethune J, Han B, et al. Analysis of five chronic inflammatory diseases identifies 27 new associations and highlights disease-specific patterns at shared loci. Nat Genet. (2016) 48:510–8. doi: 10.1038/ng.3528
48. Andreoletti G, Ashton JJ, Coelho T, Willis C, Haggarty R, Gibson J, et al. Exome analysis of patients with concurrent pediatric inflammatory bowel disease and autoimmune disease. Inflamm Bowel Dis. (2015) 21:1229–36. doi: 10.1097/MIB.0000000000000381
49. de Lange KM, Moutsianas L, Lee JC, Lamb CA, Luo Y, Kennedy NA, et al. Genome-wide association study implicates immune activation of multiple integrin genes in inflammatory bowel disease. Nat Genet. (2017) 49:256–61. doi: 10.1038/ng.3760
50. Jostins L, Ripke S, Weersma RK, Duerr RH, McGovern DP, Hui KY, et al. Host-microbe interactions have shaped the genetic architecture of inflammatory bowel disease. Nature. (2012) 491:119–24. doi: 10.1038/nature11582
51. Liu JZ, van Sommeren S, Huang H, Ng SC, Alberts R, Takahashi A, et al. Association analyses identify 38 susceptibility loci for inflammatory bowel disease and highlight shared genetic risk across populations. Nat Genet. (2015) 47:979–86. doi: 10.1038/ng.3359
52. Ek WE, Reznichenko A, Ripke S, Niesler B, Zucchelli M, Rivera NV, et al. Exploring the genetics of irritable bowel syndrome: a GWA study in the general population and replication in multinational case-control cohorts. Gut. (2015) 64:1774–82. doi: 10.1136/gutjnl-2014-307997
53. Matsuda K, Matsuda S, Gladding CM, Yuzaki M. Characterization of the delta2 glutamate receptor-binding protein delphilin: splicing variants with differential palmitoylation and an additional PDZ domain. J Biol Chem. (2006) 281:25577–87. doi: 10.1074/jbc.M602044200
54. Reimann E, Kingo K, Karelson M, Reemann P, Vasar E, Silm H, et al. Whole transcriptome analysis (RNA sequencing) of peripheral blood mononuclear cells of vitiligo patients. Dermatopathology (Basel). (2014) 1:11–23. doi: 10.1159/000357402
Keywords: genome-wide association study, inhibitors, humans, whole-genome sequencing, major histocompatibility complex, hemophilia A
Citation: Lessard S, He C, Rajpal DK, Klinger K, Loh C, Harris T and Dumont J (2022) Genome-Wide Association Study and Gene-Based Analysis of Participants With Hemophilia A and Inhibitors in the My Life, Our Future Research Repository. Front. Med. 9:903838. doi: 10.3389/fmed.2022.903838
Received: 24 March 2022; Accepted: 17 May 2022;
Published: 23 June 2022.
Edited by:
Jing He, Guangzhou Medical University, ChinaReviewed by:
Runhui Wu, Capital Medical University, ChinaBen J. Samelson-Jones, University of Pennsylvania, United States
Qizhen Shi, Medical College of Wisconsin, United States
Copyright © 2022 Lessard, He, Rajpal, Klinger, Loh, Harris and Dumont. This is an open-access article distributed under the terms of the Creative Commons Attribution License (CC BY). The use, distribution or reproduction in other forums is permitted, provided the original author(s) and the copyright owner(s) are credited and that the original publication in this journal is cited, in accordance with accepted academic practice. No use, distribution or reproduction is permitted which does not comply with these terms.
*Correspondence: Samuel Lessard, c2FtdWVsLmxlc3NhcmRAc2Fub2ZpLmNvbQ==