- 1School of Pharmaceutical Sciences, Guangzhou University of Chinese Medicine, Guangzhou, China
- 2Department of Pharmacy, Affiliated Dongguan Hospital, Southern Medical University, Dongguan, China
- 3The Research Centre of Chinese Herbal Resource, Shaoguan Institute of Danxia Dendrobium Officinale, Shaoguan, China
- 4Department of Systems Biomedical Sciences, School of Medicine, Jinan University, Guangzhou, China
- 5NHC Key Laboratory of Male Reproduction and Genetics, Guangzhou, China
- 6Department of Central Laboratory, Family Planning Research Institute of Guangdong Province, Guangzhou, China
Objective: The present study aimed to investigate the potential mechanism of Dendrobium officinale (D. officinale) on colorectal cancer and the relevant targets in the pathway using a network pharmacological approach.
Methods: (1) We identified the major bioactive components of D. officinale by UPLC-ESI-MS/MS and established the in-house library by using the literature mining method. (2) Target prediction was performed by SwissADME and SwissTargetPrediction. (3) A protein–protein interaction (PPI) network and component–target–pathway network (C-T-P network) were constructed. (4) The GO pathways and the KEGG pathway enrichment analysis were carried out by the Metascape database. (5) Molecular docking was performed by AutoDock software. (6) A series of experimental assays including cell proliferation, cell invasion and migration, and TUNEL staining in CRC were performed in CRC cell lines (HT-29, Lovo, SW-620, and HCT-116) to confirm the inhibitory effects of D. officinale.
Results: (1) In total, 396 candidate active components of D. officinale were identified by UPLC-ESI-MS/MS and selected from the database. (2) From OMIM, GeneCards, DrugBank, and TTD databases, 1,666 gene symbols related to CRC were gathered, and (3) 34 overlapping gene symbols related to CRC and drugs were obtained. (4) These results suggested that the anti-CRC components of D. officinale were mainly apigenin, naringenin, caffeic acid, γ-linolenic acid, α-linolenic acid, cis-10-heptadecenoic acid, etc., and the core targets of action were mainly ESR1, EGFR, PTGS2, MMP9, MMP2, PPARG, etc. (5) The proliferation of muscle cells, the regulation of inflammatory response, the response of cells to organic cyclic compounds, and the apoptotic signaling pathway might serve as principal pathways for CRC treatment. (6) The reliability of some important active components and targets was further validated by molecular docking. The molecular docking analysis suggested an important role of apigenin, naringenin, PTGS2, and MMP9 in delivering the pharmacological activity of D. officinale against CRC. (7) These results of the evaluation experiment in vitro suggested that D. officinale had a strong inhibitory effect on CRC cell lines, and it exerted anti-CRC activity by activating CRC cell apoptosis and inhibiting CRC cell migration and invasion.
Conclusion: This study may provide valuable insights into exploring the mechanism of action of D. officinale against CRC.
Introduction
Colorectal cancer (CRC) is the third most common cancer after lung and breast cancers, and it is also the second leading cause of cancer-related mortality (1). Dendrobium officinale Kimura & Migo (D. officinale), a widely known traditional food–medicine herb, has been commonly employed in China and other Asian countries for thousands of years (2). According to Shennong's Classic of Materia Medica, the famous classic book of Chinese materia medica, it could benefit the intestines and stomach, supplementing body fluids (3). Also, the functions of benefiting the intestines and stomach, increasing saliva, nourishing Yin, and clearing heat were recorded by Chinese pharmacopeia (2020 edition). D. officinale has also been widely used as a traditional Chinese medicinal herb for thousands of years to treat gastrointestinal sickness in China (4). More importantly, their significant anti-CRC benefits have also been validated (1, 5–10).
However, previous studies have shown that D. officinale possesses a variety of active components, including polysaccharides (1, 6, 11, 12), flavonoids (13), alkaloids (14), amino acids (15), and bibenzyl (16). The underlying mechanisms of anti-colon cancer effects of D. officinale remain unclear because of the complex compositions. Moreover, the action mechanism of D. officinale inhibits the colon cancer cell growth, and metastasis is not clear, indicating the need for an investigation.
Traditional Chinese herb medicine or formulations contain multiple active components, targets, and pathways, which are not able to be elucidated by traditional methods (17). Fortunately, the approach of network pharmacology is an effective method to analyze traditional Chinese medicine for both prescription and single herb (18, 19) and provides a new perspective for studying Chinese herbal formulas (20). As a new paradigm in drug studies, derived from systems biology and bioinformatics, network pharmacology can reveal the underlying molecular mechanisms of Chinese herb medicine, via the construction and visualization of “medicine–target–disease” interaction network (21, 22). Network pharmacology can reflect the “multiple components–multiple targets–multiple pathways” of anticancer effects of Chinese herb medicine (23), which is potential to solve the same problems of the D. officinale methanolic extract.
This study investigated the potential mechanism of D. officinale on CRC and the relevant targets in the pathway. First, we identified the major bioactive components of D. officinale by UPLC-ESI-MS/MS and established the in-house library. Second, a series of network pharmacological analyses, including target prediction, GO and KEGG enrichment analysis, and network construction, were conducted to identify the CRC-related targets and potential mechanisms of D. officinale. Third, the reliability of some key active components and targets was further validated by molecular docking. Finally, a series of experimental assays including cell proliferation, cell invasion and migration, and TUNEL staining were performed in CRC cell lines to confirm the inhibitory effects of D. officinale. The workflow of our network pharmacological and experimental studies of D. officinale in CRC is shown in Figure 1. By characterizing the D. officinale composition and its therapeutic mechanisms on CRC, we laid the foundation for the clinical application of D. officinale.
Materials and Methods
Chemicals and Reagents
D. officinale was collected from Shaoguan Hejiantang Ecological Agriculture Company Ltd. (latitude 24°80' N, longitude 113°59' E, Shaoguan, Guangdong, China) in June 2020 and was identified by Professor Yuechun Huang (Laboratory of the Department of Clinical Pharmacy, College of the First Clinical Medical, Guangzhou University of Chinese Medicine). The voucher specimen number was 57826, and a specimen was deposited in the School of Pharmaceutical Sciences, Guangzhou University of Chinese Medicine (Guangzhou, China).
The human colorectal cancer (CRC) cell lines HT-29, Lovo, SW-620, and HCT-116 were obtained from the Cell Line Bank of the Chinese Academy of Sciences (Shanghai, China). Reagents 5-fluorouracil (5-Fu), dimethyl sulfoxide (DMSO), and 3-[4,5-dimethylthiazol-2-yl]-2,5-diphenyltetrazolium bromide (MTT) were purchased from Sigma Aldrich Co. (St. Louis, MO, USA). HPLC-grade acetonitrile, methanol, and acetic acid were purchased from Merck Millipore (Billerica, MA, USA). Ultrapure water was prepared using a Millipore Alpha-Q water system (Merck Millipore). All other reagents were of analytical grade; 24-well chambers with 8 μm pore size (#353097) and Matrigel matrix (#354263) were purchased from BD Biosciences (San Jose, CA, USA). Cell culture dishes and plates were purchased from Wuxi NEST biotechnology Co. (Wuxi, Jiangsu, China); 4% paraformaldehyde was purchased from Biosharp Life Sciences (Hefei, Anhui, China); 0.1% crystal violet was purchased from Beijing Leagene Biotech Co., Ltd (Beijing, China). The One Step TUNEL Apoptosis Assay kit (#C1089), Enhanced Immunostaining Permeabilization Solution (#P0097), and DAPI Staining Solution (#C1006) were purchased from Beyotime Biotechnology (Shanghai, China).
Sample Preparation
Leaves from fresh stems of D. officinale were removed and cleaned with tap water. Then, the vacuum freeze-dried stems were ground to a powder (MM 400, Retsch, Haan, Germany) at 30 Hz for 1.5 min in a grinder. The powder (100 mg) was extracted for 24 h at 4°C with 0.6 ml 70% aqueous methanol and vortexed six times to increase the extraction rate. After centrifuging at 10,000 × g for 10 min, the supernatant was aspirated and filtered using a 0.22 μm microporous membrane for UPLC-ESI-MS/MS analysis.
Ultra-High-Performance Liquid Chromatography and Mass Spectrometry Conditions
The data acquisition instrument system included a UPLC-ESI-MS/MS system, which mainly includes UPLC (Shim-pack UFLC SHIMADZU CBM30A, SHIMADZU, Kyoto, Japan) coupled with an ESI-triple quadrupole linear ion trap MS/MS system (Applied Biosystems 4500 Q TRAP; AB SCIEX, Foster City, CA, USA).
The filtered supernatant was separated using an Acquity UHPLC HSS T3 C18 column (2.1 mm × 100 mm, 1.8 μm; Waters, Milford, MA, USA). The mobile phase consisted of 0.04% acetic acid in deionized water (mobile phase A) and 0.04% acetic acid in acetonitrile (mobile phase B). Separation was conducted using the following gradient elution: 0–10.00 min, 5–95% B; 10.00–11.00 min, 95% B; 11.0–11.10 min, 95–5% B; and 11.10–14.00 min, 5% B for equilibration of the column. The column temperature was maintained at 40°C. The flow rate was set at 0.35 ml/min, and the injection volume was 4 μL.
The mass spectrometry conditions were listed as follows: the electrospray ionization (ESI) temperature was 550°C, the ion spray voltage (IS) was 5,500 V (positive ion mode)/−4,500 V (negative ion mode), the curtain gas (CUR) was set to 30 psi, and the collision-induced dissociation (CAD) parameter was set high. In the triple quadrupole (QQQ), declustering potential (DP) and collision energy (CE) for individual multiple reaction monitoring (MRM) transitions were done with further DP and CE optimization (24, 25). Based on the self-built MetWare database (Wuhan Metware Biotechnology Co., Ltd., Wuhan, China), the material was characterized according to the secondary spectrum information.
Active Component Preparation From D. officinale and Target Prediction
The chemical composition of D. officinale was mainly obtained from UPLC-ESI-MS/MS analysis. Furthermore, we used the literature mining method (PubMed, CNKI.net, Cqvip.com, and Web of Science) to search for those that had been reported as the key active components of D. officinale, while had not been identified in UPLC-ESI-MS/MS analysis. The in-house library that covers all phytochemical constituents obtained from UPLC-ESI-MS/MS analysis and previously reported compounds from the literature is established in Table 1 and Supplementary Table 1, including the compound name, molecular weight, molecular formula, ion mode, and related references. The in-house library totally acquired 396 active components after removing duplicates.
The molecular structures of the compounds were downloaded from the PubChem database (https://pubchem.ncbi.nlm.nih.gov/) or mapped with ChemDraw 18.0 software and were saved in the MDL sdf. format. All chemical structures were converted to the Mol2 format using ChemBio3D Ultra software and then were prepared and converted into SMILES files using Open Babel GUI software for subsequent analyses.
The SwissADME tool (http://www.swissadme.ch/) was used for analyzing active components with absorption, distribution, metabolism, and excretion (ADME) properties and druglikeness evaluation to screen for active components with potential therapeutic effects. The screening criterion we applied in this research were (1) pharmacokinetics “high” and (2) druglikeness (DL) with more than two “yes.”
To obtain the targets of active components in D. officinale, SwissTargetPrediction (http://www.swisstargetprediction.ch/) (26) was employed to identify the targets. Finally, we totally acquired 116 genes after removing duplicates.
The target prediction results were sorted from high to low according to “probability,” and a threshold (probability >0.5) was set to obtain more credible targets. The official names of drug targets were retrieved through the UniProtKB search function in the UniProt database (http://www.uniprot.org/).
Collection of Predicted Targets Related to Colon Cancer
“Colon cancer” was used as the keyword to obtain colon cancer-related targets. The known targets associated with colon cancer were derived from five databases, DisGeNet (https://www.disgenet.org/), Online Mendelian Inheritance in Man (OMIM, http://www.omim.org/), Therapeutic Target Database (TTD, http://database.idrb.cqu.edu.cn/TTD/), GeneCards (https://www.genecards.Org/), and DrugBank (http://www.drugbank.ca/). We searched these databases and totally acquired 1,666 genes after removing duplicates.
Protein–Protein Interaction (PPI) Network Construction and Analysis
To obtain overlapping targets, VENNY 2.1 (http://bioinfogp.cnb.csic.es/tools/venny/index.html) software was used to cross D. officinale active component targets with CRC-related targets. Then, network protein–protein interaction network was constructed by the Search Tool for the Retrieval of Interacting Genes/Proteins (STRING) online database (https://string-db.org/, version. 11.0), using the overlap targets between D. officinale active components targets and CRC-related targets (27), where the species was limited to “Homo sapiens,” medium confidence of protein interaction data with a score > 0.400, and other basic settings were the default value.
To clarify the signaling pathways and function enrichment of potential target genes, Gene Ontology (GO) biological process enrichment analysis and Kyoto Encyclopedia of Genes and Genomes (KEGG) pathway enrichments analysis were conducted based on the Metascape database (http://metascape.org/gp/index.html) (28), using P < 0.01, min overlap genes = 3, and min enrichment factor > 1.5 as the cutoff criterion.
The PPI network was visualized utilizing Cytoscape v3.7.2 (www.cytoscape.org/) for further analyses (29, 30). The parameters “betweenness centrality (BC),” “closeness centrality (CC),” and “degree” were calculated using Network Analyzer to assess the topological importance of the nodes in the PPI network (31) and visualized the “component–target–pathway” network with the Merge feature of Cytoscape v3.7.2.
Molecular Docking
To evaluate the predicted targets, AutoDock software (4.2 version) was used to dock the structures of six active components, including apigenin, naringenin, caffeic acid, γ-linolenic acid, α-linolenic acid, and cis-10-heptadecenoic acid. These active components were docked with six candidate proteins, including ESR1 (PDB ID: 1hcq), EGFR (PDB ID: 5wb7), PTGS2 (PDB ID: 5f19), MMP9 (PDB ID: 1l6j), MMP2 (PDB ID: 1eak), and PPARG (PDB ID: 3e00), respectively. The 3D structures of ligands were obtained from the ZINC database (http://zinc.docking.org/) and saved as a mol2 file. The 3D crystal structures of the candidate protein were downloaded from the RCSB Protein Data Bank (PDB) database (https://www.rcsb.org/) and saved in the pdb format.
PyMOL software separated the original ligand from the target protein and removed the water molecule, phosphate, and other inactive ligands of the target protein (32). The protein and active components were saved in the pdb format. The AutoDockTools 1.5.6 was used to convert the pdb format of the active components and proteins to the pdbqt format. The active pocket parameters were set, and AutoDock was administered for docking. When the binding energy was ≤ – 5.0 kJ/mol, the active component was considered to have good target binding activity (33).
Experimental Evaluation
Preparation of D. officinale Methanol Aqueous Extract
To prepare the DOME, the powdered samples (100 g) of dried stems of D. officinale were extracted with 2 L 70% methanol three times, each time for 2 h. The methanol extract was concentrated using a rotary evaporator, and the concentrate was freeze-dried under vacuum at −20°C by using a vacuum freeze dryer (SCIENTZ-10Z, Ningbo Scientz Biotechnology Co., Ltd., Ningbo, Zhejiang, China). Finally, the DOME was dissolved in DMSO to adjust the stock concentration (20%, w/v) and stored at −20°C for future study.
Cell Viability
The CRC cell lines (HT-29, Lovo, SW-620, and HCT-116) were cultured in McCoy's 5A medium supplemented with 10% FBS, 100 U/ml penicillin, and 100 μg/ml streptomycin. All cultures were incubated at 37°C in an incubator with 5% CO2.
The effect of the DOME on HT-29, Lovo, SW-620, and HCT-116 cell growth was assessed using an MTT assay. In brief, the cells in the logarithmic phase were seeded in 96-well plates at 1 × 104 cells/well. After incubation for 24 h, they were exposed to the DOME (0, 250, 500, and 1,000 μg/ml) for another 24, 48, and 72 h in triplicate. Then 5-Fu (50 μg/mL) was used as the positive control, 20 μL MTT solution (5 mg/ml) was added to each well, and the cells were incubated for 4 h at 37°C in an incubator with 5% CO2. After discarding the supernatants, 100 μL DMSO was added, and the absorbance at 488 nm was measured using a microplate reader (Multiskan GO Microplate Spectrophotometer, Thermo Fisher Scientific, Waltham, MA, USA).
Wound Healing Migration Assay
Cell migration was evaluated with a wound healing assay. About 1 × 106 Lovo cells were seeded into 6-well plates prepared with five straight lines on the back and allowed to grow to 100% confluency in 10% fetal bovine serum (FBS) medium. Then, a clear cell-free line was manually created by scratching a single layer using a sterile 10 μL pipette tip in each well. After scratching, the wounded monolayers were washed three times with PBS and photographed using an inverted microscope (TS100, Nikon Corp., Tokyo, Japan) at 100 × magnification as the picture for 0 h. Then, the cells were exposed to DOME (0, 250, 500, and 1,000 μg/mL) in a 2.5% FBS medium for another 24 and 48 h, and photographed at 24 and 48 h under the microscope. The width of the scratch was measured by ImageJ software (National Institutes of Health, Bethesda, MD, USA) in 10 fields. The relative migration rate was calculated as follows:
Transwell Migration and Invasion Assay
Migration and invasion were performed using 24-well chambers with 8 μm pore size. For the migration assay, Lovo cells were resuspended in 100 μL FBS-free medium (5 × 104 cells). Then, the cells were exposed to DOME (0, 250, 500, and 1,000 μg/ml) and added to the top chamber. After that, 800 μL medium supplemented with 20% FBS was added to the lower chamber. After incubation at 37°C with 5% CO2 for 48 h, non-migrative Lovo cells on the upper chamber were carefully removed with a cotton swab. Then, the migrated Lovo cells were fixed in 4% paraformaldehyde for 20 min and stained with 0.1% crystal violet for 20 min. Migrated cell images were observed and photographed using an inverted fluorescence microscope (BX51, Olympus Optical Co. Ltd., Tokyo, Japan), at 200 × magnification. The images were quantitated using ImageJ software in six random fields.
For the invasion assay, the invasion capacity of Lovo cells was evaluated with the Matrigel matrix gel invasion assay. Then, the invasion assay was similarly performed as the migration assay, except that the upper chamber was pre-coated with 100 μL Matrigel (300 μg/ml) overnight before seeding with Lovo cells. The relative migration or invasion rate was calculated as follows:
TUNEL Staining Assay
Apoptotic DNA fragmentation was examined using the One Step TUNEL Apoptosis Assay kit in accordance with the manufacturer's instructions. In brief, the Lovo cells were plated in the cell culture dish (35 mm) at a density of 1 × 105 cells/well. After culturing at 37°C in an incubator with 5% CO2 for 24 h, the cells were treated with the DOME (0, 250, 500, and 1,000 μg/mL) in triplicate for another 48 h. After treatment, the Lovo cells were washed with PBS three times, fixed with 4% paraformaldehyde for 30 min, and permeabilized with Enhanced Immunostaining Permeabilization Solution for 5 min. The cells were then washed with PBS and incubated with TUNEL assay in the dark for 1 h at 37°C. The cells were also stained with DAPI for 10 min after a rinse with PBS to visualize cell nuclei. Cyanine 3 (Cy3)-labeled TUNEL-positive cells were imaged at × 200 magnification using a confocal laser scanning fluorescence microscope (Nikon A1 confocal, Nikon, Tokyo, Japan) with 550 nm excitation and 570 nm emission wavelengths. The cells with red fluorescence were defined as apoptotic cells.
Results
Thirty Four Potential Active Components Were Focused on by Network Pharmacology Analysis
For comprehensive identification of D. officinale chemical constituents, 70% aqueous methanol of D. officinale was analyzed using UPLC-ESI-MS/MS both in positive and negative ion scanning modes. The corresponding total ion current diagrams are shown in Supplementary Figures 1A,B. In total, 304 D. officinale constituents were finally identified (Table 1). According to the literature, additional 92 active components were collected (Supplementary Table 1). The in-house library totally acquired 396 candidate active components. After screening by SwissADME online tools, we explored the drug targets for the aforementioned candidate active components in D. officinale using SwissTargetPrediction databases, which resulted in a total of 116 putative targets (Supplementary Table 2). At the same time, 1,666 colon cancer-related targets were gathered after removing overlapping targets among different databases with 12 targets from the DisGeNet database, 495 targets from the OMIM database, 1,263 targets from the GeneCards database, 37 targets from the DrugBank database, and eight targets from the TTD database (Figure 2A).
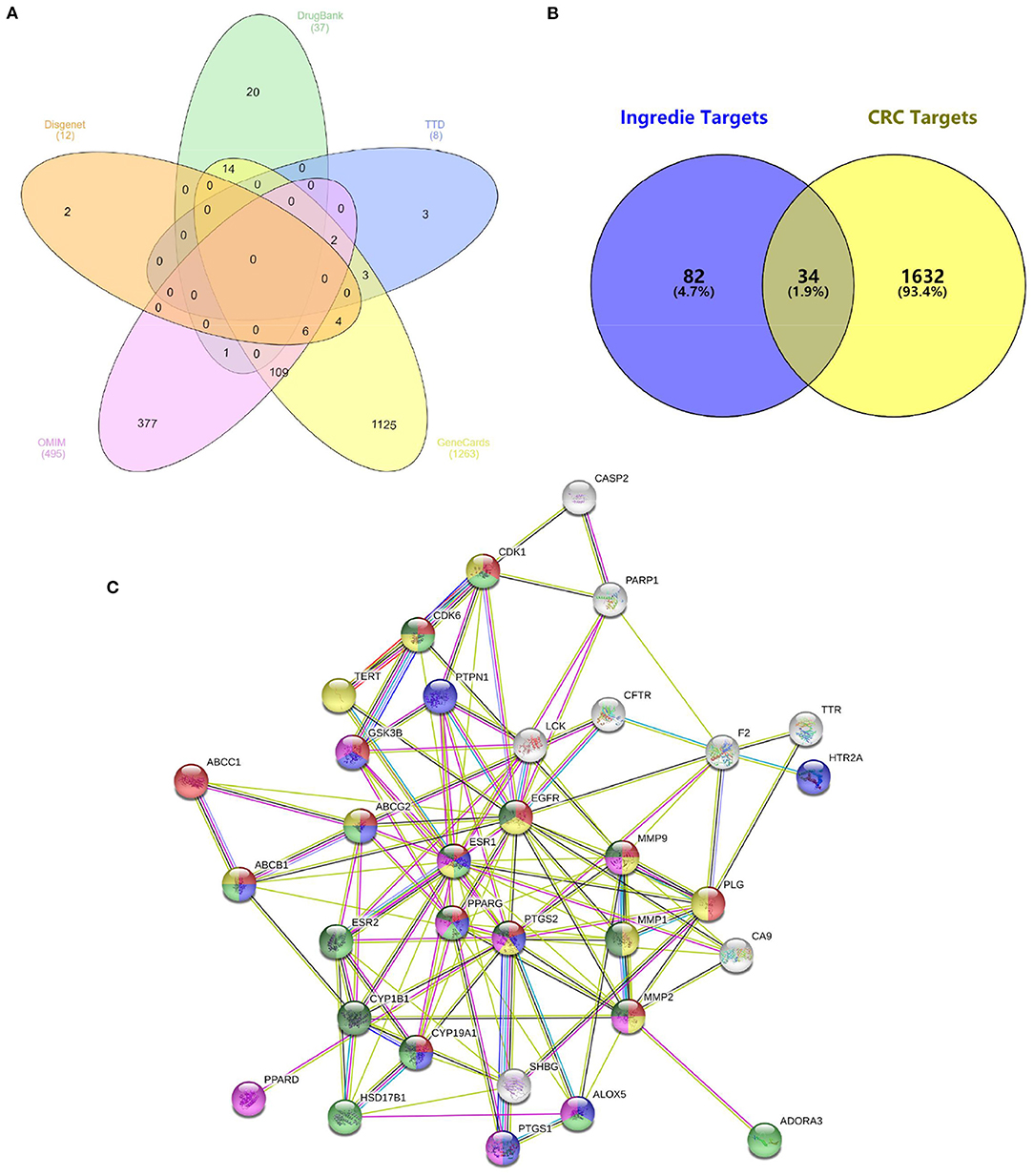
Figure 2. (A) 1,666 colon cancer-related targets. (B) Overlapping targets of active component targets and CRC targets. (C) Protein–protein interaction (PPI) network analysis.
Then, we crossed 116 D. officinale active component targets with 1,666 CRC-related targets using VENNY 2.1 software (Figure 2B). As a result, 34 common targets were focused on, namely, CA9, CYP1B1, ABCC1, PLG, CYP19A1, HSD17B1, SHBG, ESR1, ESR2, EGFR, FLT3, CDK1, PTGS2, CDK6, GSK3B, TTR, CFTR, ABCG2, ALOX5, PARP1, ABCB1, PPARG, PPARD, PTGS1, TERT, ADORA3, HTR2A, LCK, CASP2, MMP9, MMP1, MMP2, PTPN1, and F2. 36 active components (Supplementary Table 3). These 34 targets were linked by a network pharmacology approach.
Construction and Analysis of Target PPI Network
The 34 common targets were submitted to STRING version 11.0 for PPI network construction (Figure 2C). As shown in Figure 2C, the results included a total of 34 nodes and 119 edges, the nodes that represent the target proteins and the edges that represent the interactions between the proteins. In this network interaction, the larger the degree is, the stronger the interaction relationship between the targets will be. It also indicates that this key target protein plays a pivotal role in regulating the network as the hub target (34). Then, Cytoscape 3.7.2 software was used for visualization and calculation of the topological parameters, such as degree, BC, and CC. Moreover, the top six targets were obtained using the value of degree as the condition for screening core targets, mainly involving ESR1, EGFR, PTGS2, MMP9, MMP2, and PPARG.
GO and KEGG Analysis
The Metascape database was used to carry out the GO enrichment analysis related to biological processes (BP), cellular components (CC), and molecular functions (MF) and KEGG pathway analysis of 34 common target genes. In the BP group, the most enriched genes concerned “muscle cell proliferation (GO:0033002),” “regulation of inflammatory response (GO:0050727),” “cellular response to organic cyclic compound (GO:0071407),” “apoptotic signaling pathway (GO:0097190),” and “regulation of protein localization to nucleus (GO:1900180)” (Figure 3A). The most enriched genes in the CC group were in “membrane raft (GO:0045121),” “side of membrane (GO:0098552),” and “nuclear chromosome, telomeric region (GO:0000784)” (Figure 3B). The genes in the MF group that were most enriched comprised “xenobiotic transmembrane transporting ATPase activity (GO:0008559),” “nuclear receptor activity (GO:0004879),” and “serine-type endopeptidase activity (GO:0004252)” (Figure 3C). KEGG pathway analysis indicated that “pathways in cancer (hsa05200),” “Ovarian steroidogenesis (hsa04913),” and “MicroRNAs in cancer (hsa05206)” were involved in the regulation of common target genes (Figure 3D). Details are listed in Supplementary Table 4. Based on these results, we were interested in and focused on the apoptotic signaling pathway, which was highly enriched in the GO and KEGG databases.
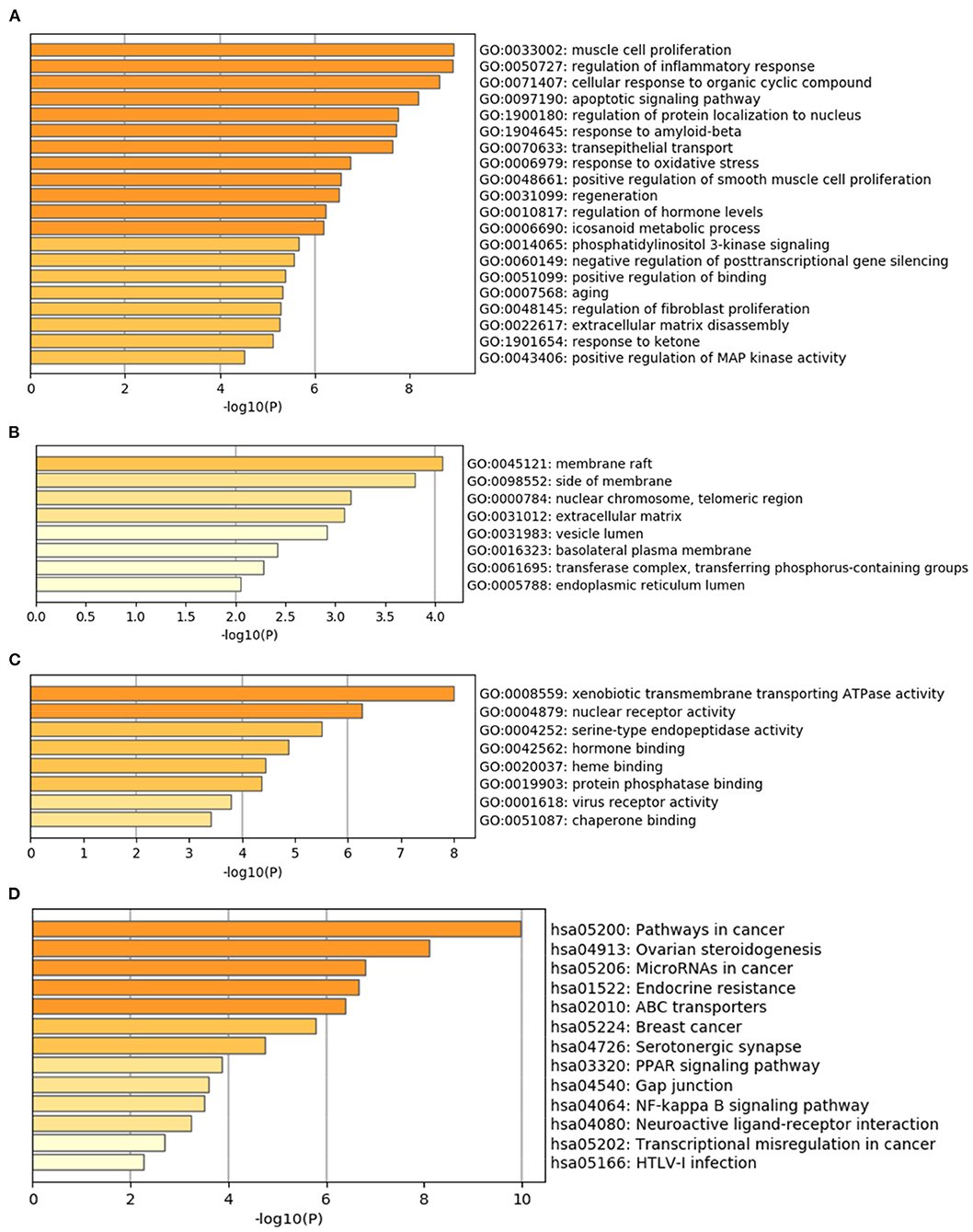
Figure 3. GO and KEGG pathway enrichment analyses of 34 common target genes. (A) Biological process analysis. (B) Cellular component analysis. (C) Molecular function analysis. (D) KEGG pathway analysis.
Analyses of the Component–Target–Pathway Network
According to the predicted results of the identified active components, key targets, and top 22 related pathways using the KEGG database (Supplementary Table 4), an integrated component–target–pathway network was constructed using Cytoscape.
As shown in Figure 4, 36 core active components were obtained, which were gallic acid, isorhamnetin, protocatechuic acid, homoeriodictyol, isosakuranetin (7'-methylnaringenin), tamarixetin, Di-O-methylquercetin, naringenin, naringenin chalcone, apigenin, scopoletin (7-hydroxy-5-methoxycoumarin), 13-hydroxy-9,11-octadecadienoic acid, 9-hydroxy-10,12-octadecadienoic acid, myristic acid, pentadecanoic acid, palmitoleic acid, γ-linolenic acid, α-linolenic acid, cis-10-heptadecenoic acid, L-glutamic acid, (-)-3-(3,4-dihydroxyphenyl)-2-methylalanine, 5-hydroxy-L-tryptophan, 3,4-dihydroxy-DL-phenylalanine, spermine, ferulic acid, syringic acid, trans-4-hydroxycinnamic acid methyl ester, trans-ferulic acid, caffeic acid, p-coumaric acid, gentisic acid, hexadecanoic acid, benzamidine, rutundic acid, p-hydroxy cinnamic acid, and 3',5, 5',7-tetrahydroxyflavanone (Supplementary Table 3). Apigenin, naringenin, caffeic acid, γ-linolenic acid, α-linolenic acid, cis-10-heptadecenoic acid, tamarixetin, palmitoleic acid, 3,4-dihydroxy-DL-phenylalanine, and p-coumaric acid were found to be the top 10 important active components in this network (Supplementary Table 5). Apigenin was the most important active component (degree = 17, BC = 0.6359, and CC = 0.4607), followed by naringenin (7, 0.3811, 0.3388). In addition, ESR1, EGFR, PTGS2, MMP9, MMP2, PPARG, PLG, CYP1B1, ESR2, and CYP19A1 were found to be the top 10 important targets in this network (Supplementary Table 6).
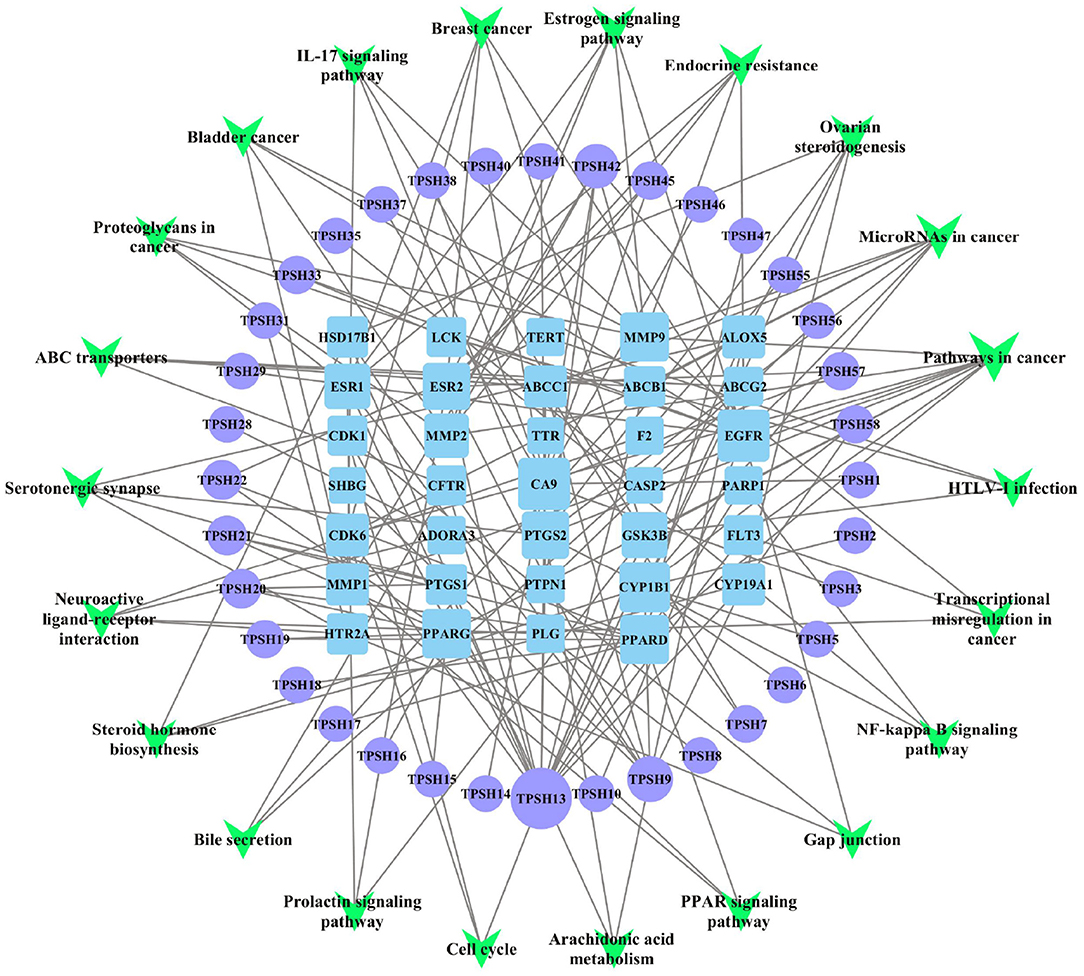
Figure 4. Component–target–pathway (C-T-P) network of D. officinale for CRC. The purple circles represent the active components of D. officinale, the blue squares represent the common targets, and the green inverted triangles represent the main pathways. Node size is proportional to its degree. The larger the area is, the more important it is in the network.
Molecular Docking
Molecular docking of the key active components of D. officinale (apigenin, naringenin, caffeic acid, γ-linolenic acid, α-linolenic acid, and cis-10-heptadecenoic acid) with the key target proteins (ESR1, EGFR, PTGS2, MMP9, MMP2, and PPARG) was performed by AutoDock 4.0.
The results showed that apigenin and naringenin are well-docked with PTGS2 and MMP9 and have a good affinity, suggesting that D. officinale has high accuracy in the treatment of CRC (Supplementary Table 7). Moreover, compared with other targets, the docking energy of apigenin with PTGS2 (−6.31 kJ/mol) was the lowest, indicating that the binding ability and stability of apigenin–PTGS2 complex were higher than those of the other targets. Apigenin was able to produce interaction with five amino acids within the binding pouch of PTGS2 such as ASN-34, GLN-327, ASP-157, GLN-461, and HIS-39 at bond lengths of 2.2, 2.1, 3.1, 2.0, 2.4, and 2.14 Å, respectively. The docking model is shown in Figure 5B. In addition, apigenin was docked with MMP9, and the binding energy was −5.20 kJ/mol. Also, apigenin was able to produce interaction with three amino acids within the binding pouch of MMP9 such as THR-246, ARG-332, and CYS-244 at bond lengths of 2.5, 1.7, and 2.4 Å, respectively. The docking model is shown in Figure 5A. The docking binding energies of naringenin with PTGS2 and MMP9 were −6.20 and −5.81 kJ/mol, respectively. Naringenin was able to produce interaction with four amino acids within the binding pouch of PTGS2 such as HIS-39, GLN-461, GLY-45, and GLN-327 at bond lengths of 1.8, 2.5, 2.1, 2.5, and 2.5 Å, respectively. Also, naringenin was able to produce interaction with one amino acid (PHE-107) within the binding pouch of MMP9 at a bond length of 2.1 Å. The docking model is shown in Figures 5C,D.
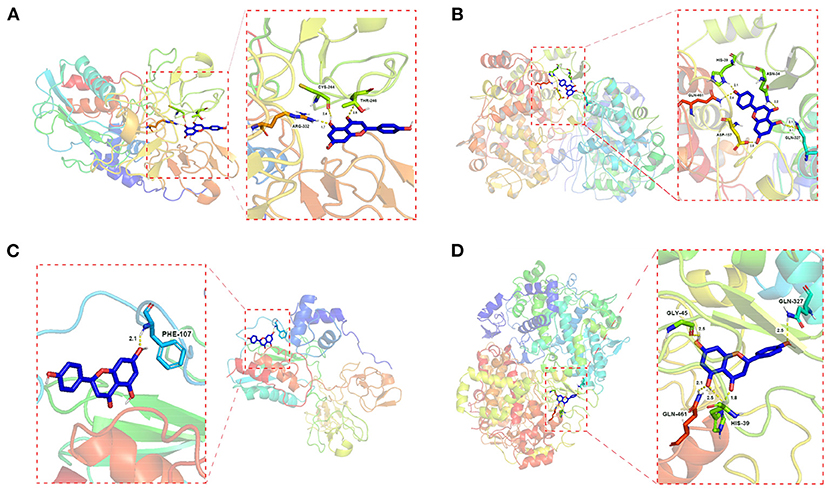
Figure 5. Schematic (3D) representation of the molecular model of the active component combined with the target proteins. Components: apigenin. Target proteins: (A) MMP9; (B) PTGS2; components: naringenin. Target proteins: (C) MMP9; (D) PTGS2.
Experimental Evaluation
MTT Assay
To investigate the antitumor effect of the DOME on CRC, the treated HT-29, Lovo, SW-620, and HCT-116 cells were analyzed using an MTT assay. As shown in Figure 6, the results showed that the DOME decreased the proliferation of the four CRC cell lines in a dose- and time-dependent manner. In addition, the inhibitory rate was 1,000 μg/ml DOME ≤ 20% on the four CRC cells at 24 h, except the DOME on HT-29 cells (44.83%). However, the inhibitory rate was 1,000 μg/ml DOME ≥40% on these CRC cells at 48 h, which is close to the effect at 72 h. Therefore, this study chose 48 h as the time of the DOME on CRC cells in the subsequent experiments.
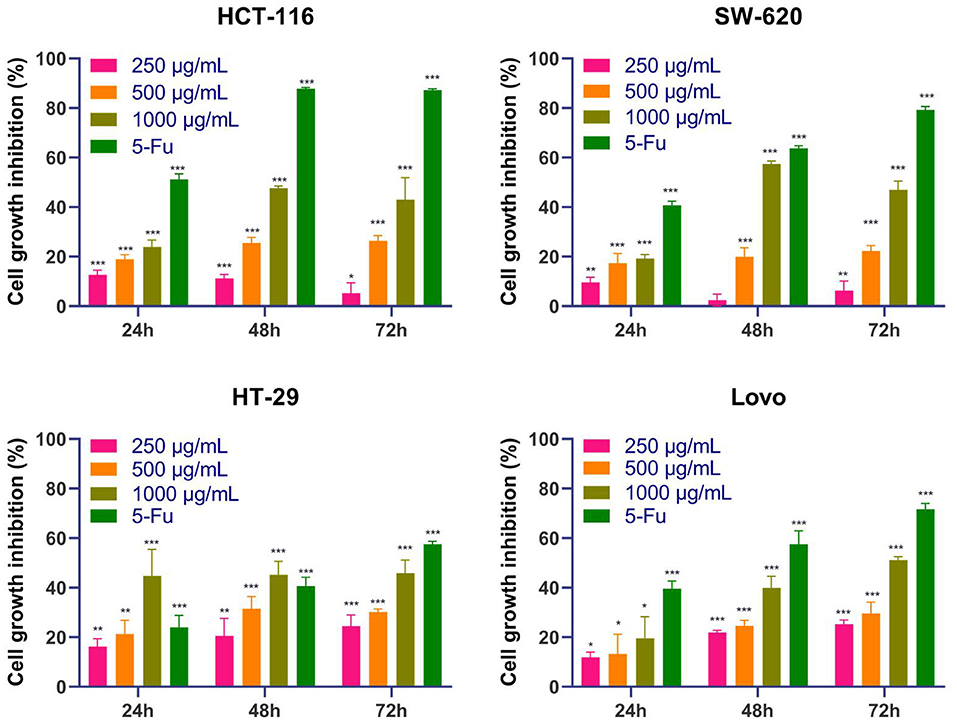
Figure 6. Effects of DOME on the proliferation of CRC cell lines. The cell viability of HT-29, Lovo, SW-620, and HCT-116 cells treated with DOME for 24, 48, and 72 h were determined by MTT assay. The error bars represent SEM (*P < 0.05, **P < 0.01, ***P < 0.001, when compared with blank group).
Wound Healing Migration Assay and Transwell Migration and Invasion Assay
Because MMP-2 and MMP-9 are important executors in cell migration and invasion, and based on the previous results in the component–target–pathway network analysis and molecular docking analysis, we further assessed the inhibitory effects of the DOME on the in vitro migration and invasion potential of Lovo cells using the wound healing migration assay and transwell assay, respectively. These results showed that the DOME significantly inhibited Lovo cell migration in a dose- and time-dependent manner (Figures 7A–C). To further assess the effects of the DOME on the migration of Lovo cells in vitro, the transwell migration assay was employed. The transwell migration assay also revealed that the DOME markedly weakened the migration ability of Lovo cells (P < 0.01, Figures 7D–F), further confirming the result of the wound healing assay.
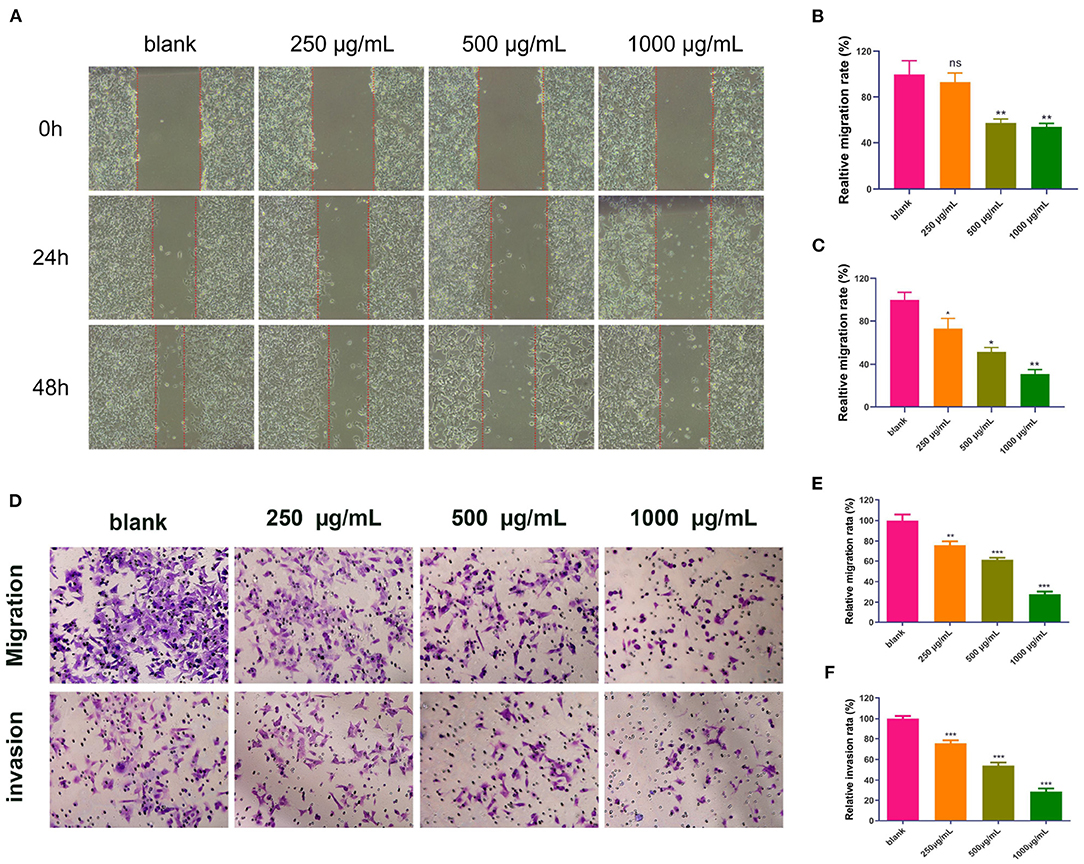
Figure 7. DOME suppresses migration and invasion of Lovo cells. (A) Images of wound healing assay; quantification of wound healing assay in Lovo cells at 24 h (B) and 48 h (C); (D) transwell assay in Lovo cells. (E) quantification of transwell migration assay in Lovo cells; (F) quantification of transwell invasion assay in Lovo cells. The error bars represent SEM (*P < 0.05, **P < 0.01, ***P < 0.001, when compared with the blank group).
Furthermore, cell invasion ability was determined by a transwell invasion assay. Compared to the blank group, the number of invaded cells in the DOME group was significantly reduced in a dose-dependent manner (Figures 7E,F). These results suggested that the DOME could suppress Lovo cell migration and invasion in vitro.
TUNEL Staining
According to the predicted results of the GO and KEGG pathway enrichment analyses and to further confirm the apoptosis-inducing activities of DOME in Lovo cells, TUNEL staining was also performed. The cell apoptosis in Lovo cells was determined with TUNEL assay after DOME treatments for 48 h. As shown in Figure 8, TUNEL-positive nuclei (representing apoptotic cells) were stained with red fluorescence, while total nuclei stained with DAPI and exhibited blue fluorescence. Figure 8 shows that the DOME remarkably increased TUNEL-positive (red) cell number. The result demonstrated that the DOME induces apoptosis in Lovo cells, and this effect was significantly enhanced in a dose-dependent manner.
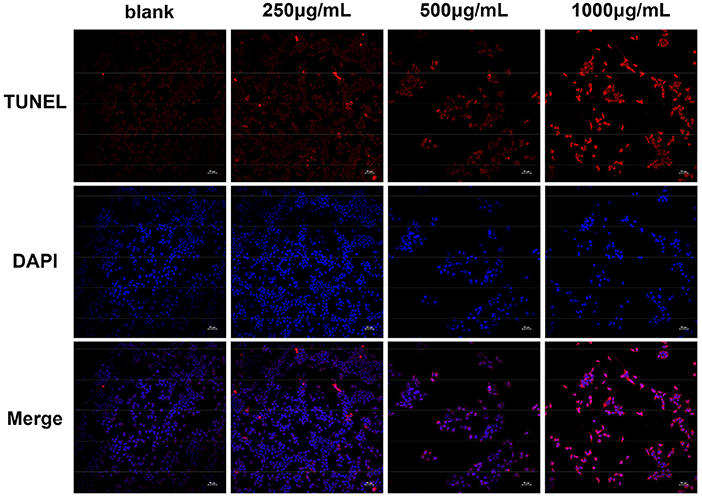
Figure 8. Effect of DOME on cell apoptosis in Lovo cells. The cell apoptosis was determined using TUNEL assay, TUNEL-positive nuclei in red fluorescent color, and total nuclei staining with DAPI. Scale bar: 50 μm.
Discussion
Currently, chemotherapy is the conventional treatment method for CRC, such as 5-Fu. However, chemotherapy has many adverse effects (35). A number of prior studies have shown that D. officinale exhibits excellent bioactivity against CRC (9, 10). Used as a medicine and food dual-use product for more than a thousand years, D. officinale has fewer side effects (1). However, the underlying mechanisms of anti-colon cancer effects of D. officinale remain unclear because of the complex compositions. The core active components and core target of D. officinale, which play the key role against the CRC, are unclear. We applied metabolomics combined with network pharmacology to screen the active components, drug targets, and singling pathway. Moreover, we performed further experimental validation using a series of cellular functional and molecular biological assays in vitro. Overall, our results did not only clarify the anti-CRC effective components and their potential targets of D. officinale but also offer in-depth knowledge of the molecular mechanisms of D. officinale.
In this study, 304 metabolites of active components in D. officinale 70% methanol extracts were determined by UPLC-ESI-MS/MS metabonomic analysis, while 178 compounds were identified by UPLC-MS/MS from the D. officinale anhydrous ethanol extracts as reported in the literature (36). It may be due to the different origins of D. officinale or the different extraction solvents used. Furthermore, in order to fully establish the component library of D. officinale and make the network pharmacological analysis more accurate (37), we used the literature mining method (PubMed, CNKI.net, Cqvip.com, and Web of Science) to search for those that had been reported as the key active components of D. officinale, while had not been identified in UPLC-ESI-MS/MS analysis. Overall, 92 active components of D. officinale were obtained. So, the in-house library totally acquired 396 active components. Subsequently, 116 putative targets were obtained by screening using the SwissADME online tools and SwissTargetPrediction databases. Then, 1,666 colon cancer-related targets were gathered from the DisGeNet database, OMIM database, GeneCards database, DrugBank database, and the TTD database (Figure 2A); 34 common targets were acquired after crossing 116 D. officinale active component targets with 1,666 CRC-related targets using VENNY 2.1 software.
Network pharmacology analysis results showed that the key active components mainly involved apigenin, naringenin, caffeic acid, γ-linolenic acid, α-linolenic acid, and cis-10-heptadecenoic acid, and the top six targets mainly involved ESR1, EGFR, PTGS2, MMP9, MMP2, and PPARG. These results involving key active components naringenin and caffeic acid were consistent with the literature report (36). The results of molecular docking suggested that the aforementioned key molecules are well-docked with important target proteins and have a good affinity, suggesting that D. officinale has high accuracy in the treatment of CRC (Supplementary Table 7). Moreover, the docking energy of apigenin with PTGS2 (−6.31 kJ/mol) was the lowest, followed by naringenin with PTGS2 (−6.20 kJ/mol), naringenin with MMP9 (−5.81 kJ/mol), and apigenin with MMP9 (−5.20 kJ/mol) (Figure 5), indicating that apigenin and naringenin in D. officinale were the most important active components, and PTGS2 and MMP9 were the most important targets that play the role of against CRC.
The finding that apigenin and naringenin were the most important active components is consistent with previous studies which have demonstrated that apigenin and naringenin have inhibitory effects against CRC (38–41). However, it is worth noting that the content of naringenin in D. officinale is much more than apigenin, consistent with a previous study (42). The study showed that the content of naringenin in D. officinale was generally higher, and the average mass fraction of the components reached 26.87 μg·g−1. Correspondingly, the component mass fraction of apigenin in D. officinale is ≤ 0.5 μg·g−1, it mainly exists in the form of flavonoid carbon glycosides, with apigenin as the core, rather than its free state (43–45). Therefore, naringenin might play a more important role than apigenin. However, there is also a possibility that the flavonoid carbon glycosides with apigenin as the nucleus degrade into free-state apigenin to exert anti-CRC effects.
MMP-2 and MMP-9 are important executors in cell migration and invasion (46, 47), and PTGS2 has been recognized to play an important role in regulating cell migration, invasion, and proliferation (48). In addition, in accordance with the results of molecular docking, a previous study has demonstrated that apigenin and naringenin can inhibit the invasion and migration of colon cancer cells by inhibiting the epithelial-to-mesenchymal transition (EMT) of CRC (49, 50). Hence, it could conceivably be hypothesized that the mechanism of D. officinale against CRC is closely related to the inhibition of tumor invasion and migration. The experiments of wound healing migration assay and transwell migration and invasion assay in this study further verified the conjecture. Despite these promising results, there were also some limitations to this research. We have not proven that apigenin and naringenin could exert anti-colon cancer effects via regulating PTGS2 and MMP9 with additional experiments in this study. The experimental evaluation was conducted only in vitro. Further in vivo animal experiments still need to be performed to confirm and validate these findings.
The GO and KEGG analysis results showed that the target proteins involved in the main pathways were “muscle cell proliferation (GO:0033002),” “regulation of inflammatory response (GO:0050727),” “cellular response to organic cyclic compound (GO:0071407),” “apoptotic signaling pathway (GO:0097190),” “regulation of protein localization to nucleus (GO:1900180),” “pathways in cancer” (hsa05200), “ovarian steroidogenesis” (has04913), and “microRNAs in cancer” (hsa05206) (Figures 3A,D). As shown in Supplementary Figure 2, the cancer-related KEGG pathways were determined as pathways related to the “pathways in cancer”: PPAR signaling pathway, MAPK signaling pathway, calcium signaling pathway, cAMP signaling pathway, cytokine–cytokine receptor interaction, HIF-1 signaling pathway, cell cycle, p53 signaling pathway, mTOR signaling pathway, PI3K-Akt signaling pathway, apoptosis, Wnt signaling pathway, Notch signaling pathway, Hedgehog signaling pathway, TGF-beta signaling pathway, VEGF signaling pathway, focal adhesion, ECM–receptor interaction, adherens junction, JAK-STAT signaling pathway, and estrogen signaling pathway (51). Combined with the core protein targets analyzed in the previous network pharmacology (mainly ESR1, EGFR, PTGS2, MMP9, MMP2, PPARG, etc.), we speculate that the subcellular pathways that may play a role in inhibiting colon cancer proliferation, invasion, and migration are EGFR-associated signaling pathways (EGFR/Raf/MEK/ERK cascades) and PPAR signaling pathway. As shown in Figure 4D, “PPAR signaling pathway (hsa03320)” was also the eighth most enriched pathway in the KEGG pathway analysis. As shown in Supplementary Figures 3, 4, combined with the protein targets screened in the previous network pharmacology, we suppose miR-145 suppresses tumorigenesis and metastasis of the colorectal cancer cells by inhibiting the expression of EGFR. Dysregulation of specific miRNAs has been associated with certain types of cancer, where they may act as either oncogenes or tumor suppressors, depending on their target genes (52). For example, in situ hybridization detected accumulation of miR-145 in normal colon epithelia with no signal from adenocarcinomas cells. Loss of miR-145 in various tumors suggests its role as a tumor suppressor. miR-145 is downregulated in colorectal cancer (53, 54). In fact, miR-145 has been well-documented as a tumor suppressor gene in multiple tumor types because of its anti-proliferative and pro-apoptotic effects. Several reports suggest that miR-145 is a suppressor of metastasis. For example, miR-145 negatively regulates MUC1 and suppresses invasion and metastasis of breast cancer cells (55), and miR-145 suppresses proliferation, metastasis, and EMT of colorectal cancer via suppressing the EGFR-associated signaling pathway (56–58). In this study, other mechanisms in the aforementioned signal pathways might also play an important role. Further research is required to be performed to better understand the underlying mechanisms.
Conclusion
In conclusion, the present results demonstrated that the core active components are apigenin and naringenin, and the core targets are PTGS2 and MMP-9 when D. officinale exerts antitumor effects on CRC through a combination of network pharmacology, metabolomics, and molecular docking. Moreover, the apoptotic signaling pathway and inhibition of tumor invasion and migration signaling pathway had been validated to play a vital role in D. officinale against CRC. Our findings provided valuable insights into exploring the mechanism of action of D. officinale against CRC.
Data Availability Statement
The datasets presented in this study can be found in online repositories. The names of the repository/repositories and accession number(s) can be found at: MetaboLights; MTBLS4678.
Author Contributions
ST: conceptualization, data curation, writing—original draft preparation, writing—review and editing, and visualization. ST, JL, and HW: methodology and validation. ST, RH, and ZR: software. ST and HW: formal analysis. ST, SD, and WH: investigation. GW: resources, supervision, project administration, and funding acquisition. All authors contributed to the article and approved the submitted version.
Funding
This work was supported by the Innovation project of Dendrobium truth-seeking in Guangzhou University of Traditional Chinese Medicine (No. 2019shqz0209801), the 2018 Shaoguan City Science and Technology Plan Project: Special Project of Industry-University-Research Cooperation (No. 2018CS11919), and the 2019 Guangdong Province Special Fund for Science and Technology (Big project + task list) Project: Ecological Cultivation and Sustainable Utilization of Danxia Dendrobium officinale, a rare Southern Medicine in Guangdong Province (Nos. 2019gdskjzxzj-zt3-2 and 210316166270419).
Conflict of Interest
The authors declare that the research was conducted in the absence of any commercial or financial relationships that could be construed as a potential conflict of interest.
Publisher's Note
All claims expressed in this article are solely those of the authors and do not necessarily represent those of their affiliated organizations, or those of the publisher, the editors and the reviewers. Any product that may be evaluated in this article, or claim that may be made by its manufacturer, is not guaranteed or endorsed by the publisher.
Supplementary Material
The Supplementary Material for this article can be found online at: https://www.frontiersin.org/articles/10.3389/fmed.2022.879986/full#supplementary-material
Abbreviations
ADME, absorption, distribution, metabolism, and excretion; BC, betweenness centrality; CC, closeness centrality; CRC, colorectal cancer; D. officinale, Dendrobium officinale; DOME, D. officinale methanolic extract; DMSO, dimethyl sulfoxide; FBS, fetal bovine serum; GO, Gene Ontology; 5-Fu, 5-fluorouracil; C-T-P network, component–target–pathway network; KEGG, Kyoto Encyclopedia of Genes and Genomes; MF, molecular functions; MTT, 3-[4,5-dimethylthiazol-2-yl]-2,5-diphenyltetrazolium bromide; OMIM, Online Mendelian Inheritance in Man; PDB, Protein Data Bank; PPI, protein–protein interaction; STRING, Search Tool for the Retrieval of Interacting Genes/Proteins; TTD, Therapeutic Target Database.
References
1. Tao S, Huang C, Tan Z, Duan S, Zhang X, Ren Z, et al. Effect of the polysaccharides derived from Dendrobium officinale stems on human HT-29 colorectal cancer cells and a zebrafish model. Food Biosci. (2021) 41:100995. doi: 10.1016/j.fbio.2021.100995
2. Ng TB, Liu J, Wong JH, Ye X, Wing Sze SC, Tong Y, et al. Review of research on Dendrobium, a prized folk medicine. Appl Microbiol Biotechnol. (2012) 93:1795–803. doi: 10.1007/s00253-011-3829-7
3. Liang J, Chen S, Chen J, Lin J, Xiong Q, Yang Y, et al. Therapeutic roles of polysaccharides from Dendrobium officinaleon colitis and its underlying mechanisms. Carbohydr Polymers. (2018) 185:159–68. doi: 10.1016/j.carbpol.2018.01.013
4. Luo D, Qu C, Zhang Z, Xie J, Xu L, Yang H, et al. Granularity and laxative effect of ultrafine powder of Dendrobium officinale. J Med Food. (2017) 20:180–8. doi: 10.1089/jmf.2016.3827
5. Zhang K, Zhou X, Wang J, Zhou Y, Qi W, Chen H, et al. Dendrobium officinale polysaccharide triggers mitochondrial disorder to induce colon cancer cell death via ROS-AMPK-autophagy pathway. Carbohydr Polymers. (2021) 264:118018. doi: 10.1016/j.carbpol.2021.118018
6. Tao S, Ren Z, Yang Z, Duan S, Wan Z, Huang J, et al. Effects of different molecular weight polysaccharides from Dendrobium officinale Kimura & Migo on human colorectal cancer and transcriptome analysis of differentially expressed genes. Front Pharmacol. (2021) 12:704486. doi: 10.3389/fphar.2021.704486
7. Liang J, Li H, Chen J, He L, Du X, Zhou L, et al. Dendrobium officinale polysaccharides alleviate colon tumorigenesis via restoring intestinal barrier function and enhancing anti-tumor immune response. Pharmacol Res. (2019) 148:104417. doi: 10.1016/j.phrs.2019.104417
8. Li G, Sun P, Zhou Y, Zhao X, Chen F. Preventive effects of Dendrobium candidum Wall ex Lindl. on the formation of lung metastases in BALB/c mice injected with 26-M3.1 colon carcinoma cells. Oncol Lett. (2014) 8:1879–85. doi: 10.3892/ol.2014.2383
9. Wang Q, Sun P, Li G, Zhu K, Wang C, Zhao X. Inhibitory effects of Dendrobium candidum Wall ex Lindl. on azoxymethane- and dextran sulfate sodium-induced colon carcinogenesis in C57BL/6 mice. Oncol Lett. (2014) 7:493–8. doi: 10.3892/ol.2013.1728
10. Zhao X, Sun P, Qian Y, Suo H. D. candidum has in vitro anticancer effects in HCT-116 cancer cells and exerts in vivo anti-metastatic effects in mice. Nutr Res Pract. (2014) 8:487–93. doi: 10.4162/nrp.2014.8.5.487
11. Tao S, Lei Z, Huang K, Li Y, Ren Z, Zhang X, et al. Structural characterization and immunomodulatory activity of two novel polysaccharides derived from the stem of Dendrobium officinale Kimura et Migo. J Funct Foods. (2019) 57:121–34. doi: 10.1016/j.jff.2019.04.013
12. Yue H, Zeng H, Ding K. A review of isolation methods, structure features and bioactivities of polysaccharides from Dendrobium species. Chin J Natural Med. (2020) 18:1–27. doi: 10.1016/S1875-5364(20)30001-7
13. Zhiyao Ren, Xiaoyu Ji, Zhenbin Jiao, Yingyi Luo, Guo-Qiang Zhang, ShengchangTao, et al. Functional analysis of a novel C-glycosyltransferase in the orchid Dendrobium catenatum. Horticult Res. (2020) 7:1–18. doi: 10.1038/s41438-020-0330-4
14. Chen Y, Wang Y, Lyu P, Chen L, Shen C, Sun C. Comparative transcriptomic analysis reveal the regulation mechanism underlying MeJA-induced accumulation of alkaloids in Dendrobium officinale. J Plant Res. (2019) 132:419–29. doi: 10.1007/s10265-019-01099-6
15. Nie SP, Cui SW, Xie MY. Glucomannans From Dendrobium officinale and Aloe. Bioactive Polysaccharides. Elsevier Inc. (2018). p. 295–347.
16. Tang H, Zhao T, Sheng Y, Zheng T, Fu L, Zhang Y. Dendrobium officinale Kimura et Migo: a review on its ethnopharmacology, phytochemistry, pharmacology, and industrialization. Evid Based Complement Altern Med. (2017) 2017:7436259. doi: 10.1155/2017/7436259
17. Ma Y, Zhou K, Fan J, Sun S. Traditional Chinese medicine: potential approaches from modern dynamical complexity theories. Front Med. (2016) 10:28–32. doi: 10.1007/s11684-016-0434-2
18. Zhao J, Tian S, Lu D, Yang J, Zeng H, Zhang F, et al. Systems pharmacological study illustrates the immune regulation, anti-infection, anti-inflammation, and multi-organ protection mechanism of Qing-Fei-Pai-Du decoction in the treatment of COVID-19. Phytomedicine. (2021) 85:153315. doi: 10.1016/j.phymed.2020.153315
19. Ren L, Zheng X, Liu J, Li W, Fu W, Tang Q, et al. Network pharmacology study of traditional Chinese medicines for stroke treatment and effective constituents screening. J Ethnopharmacol. (2019) 242:112044. doi: 10.1016/j.jep.2019.112044
20. Zineh I. Quantitative systems pharmacology: a regulatory perspective on translation. CPT Pharmacometr Syst Pharmacol. (2019) 8:336–9. doi: 10.1002/psp4.12403
21. Ma YM, Zhang XZ, Su ZZ, Li N, Cao L, Ding G, et al. Insight into the molecular mechanism of a herbal injection by integrating network pharmacology and in vitro. J Ethnopharmacol. (2015) 173:91–9. doi: 10.1016/j.jep.2015.07.016
22. Li S, Zhang B. Traditional Chinese medicine network pharmacology: theory, methodology and application. Chin J Natural Med. (2013) 11:110–20. doi: 10.1016/S1875-5364(13)60037-0
23. Cao S, Han Y, Li Q, Chen Y, Zhu D, Su Z, et al. Mapping pharmacological network of multi-targeting Litchi ingredients in cancer therapeutics. Front Pharmacol. (2020) 11:451. doi: 10.3389/fphar.2020.00451
24. Chen W, Gong L, Guo Z, Wang W, Zhang H, Liu X, et al. A novel integrated method for large-scale detection, identification, and quantification of widely targeted metabolites: application in the study of rice metabolomics. Mol Plant. (2013) 6:1769–80. doi: 10.1093/mp/sst080
25. Chen L, Wu Q, He W, He T, Wu Q, Miao Y. Combined de novo transcriptome and metabolome analysis of common bean response to Fusarium oxysporum f. sp. phaseoli infection. Int J Mol Sci. (2019) 20:6278. doi: 10.3390/ijms20246278
26. Gfeller D, Grosdidier A, Wirth M, Daina A, Michielin O, Zoete V. SwissTargetPrediction: a web server for target prediction of bioactive small molecules. Nucleic Acids Res. (2014) 42:W32–8. doi: 10.1093/nar/gku293
27. Szklarczyk D, Gable AL, Lyon D, Junge A, Wyder S, Huerta-Cepas J, et al. STRING v11: protein-protein association networks with increased coverage, supporting functional discovery in genome-wide experimental datasets. Nucleic Acids Res. (2019) 47:D607–13. doi: 10.1093/nar/gky1131
28. Zhou Y, Zhou B, Pache L, Chang M, Khodabakhshi AH, Tanaseichuk O, et al. Metascape provides a biologist-oriented resource for the analysis of systems-level datasets. Nat Commun. (2019) 10:1523. doi: 10.1038/s41467-019-09234-6
29. Smoot ME, Ono K, Ruscheinski J, Wang PL, Ideker T. Cytoscape 2.8: new features for data integration and network visualization. Bioinformatics. (2011) 27:431–2. doi: 10.1093/bioinformatics/btq675
30. Shannon P, Markiel A, Ozier O, Baliga NS, Wang JT, Ramage D, et al. Cytoscape: a software environment for integrated models of biomolecular interaction networks. Genome Res. (2003) 13:2498–504. doi: 10.1101/gr.1239303
31. Assenov Y, Ramirez F, Schelhorn SE, Lengauer T, Albrecht M. Computing topological parameters of biological networks. Bioinformatics. (2008) 24:282–4. doi: 10.1093/bioinformatics/btm554
32. Warnecke A, Sandalova T, Achour A, Harris RA. PyTMs: a useful PyMOL plugin for modeling common post-translational modifications. BMC Bioinformatics. (2014) 15:370. doi: 10.1186/s12859-014-0370-6
33. Zhang ZJ, Wu WY, Hou JJ, Zhang LL, Li FF, Gao L, et al. Active constituents and mechanisms of Respiratory Detox Shot, a traditional Chinese medicine prescription, for COVID-19 control and prevention: network-molecular docking-LC-MS(E) analysis. J Integr Med. (2020) 18:229–41. doi: 10.1016/j.joim.2020.03.004
34. Ge Q, Chen L, Yuan Y, Liu L, Feng F, Lv P, et al. Network pharmacology-based dissection of the anti-diabetic mechanism of Lobelia chinensis. Front Pharmacol. (2020) 11:347. doi: 10.3389/fphar.2020.00347
35. Jin KT, Chen B, Liu YY, Lan HU, Yan JP. Monoclonal antibodies and chimeric antigen receptor (CAR) T cells in the treatment of colorectal cancer. Cancer Cell Int. (2021) 21:83. doi: 10.1186/s12935-021-01763-9
36. Wei L, Dong W, Han Z, Chen C, Jin Q, He J, et al. Network pharmacologic analysis of Dendrobium officinale extract inhibiting the proliferation of gastric cancer cells. Front Pharmacol. (2022) 13:832134. doi: 10.3389/fphar.2022.832134
37. Pan B, Wang Y, Wu C, Jia J, Huang C, Fang S, et al. A mechanism of action study on Danggui Sini decoction to discover its therapeutic effect on gastric cancer. Front Pharmacol. (2020) 11:592903. doi: 10.3389/fphar.2020.592903
38. Memariani Z, Abbas SQ, Ul Hassan SS, Ahmadi A, Chabra A. Naringin and naringenin as anticancer agents and adjuvants in cancer combination therapy: efficacy and molecular mechanisms of action, a comprehensive narrative review. Pharmacol Res. (2021) 171:105264. doi: 10.1016/j.phrs.2020.105264
39. Wang L, Zeng W, Wang L, Wang Z, Yin X, Qin Y, et al. Naringenin enhances the antitumor effect of therapeutic vaccines by promoting antigen cross-presentation. J Immunol. (2020) 204:622–31. doi: 10.4049/jimmunol.1900278
40. Li C, Lin D, Fu X, Zhang L, Fan Q, Liu Y, et al. Apigenin up-regulates transgelin and inhibits invasion and migration of colorectal cancer through decreased phosphorylation of AKT. J Nutr Biochem. (2013) 24:1766–75. doi: 10.1016/j.jnutbio.2013.03.006
41. Yang C, Song J, Hwang S, Choi J, Song G, Lim W. Apigenin enhances apoptosis induction by 5-fluorouracil through regulation of thymidylate synthase in colorectal cancer cells. Redox Biol. (2021) 47:102144. doi: 10.1016/j.redox.2021.102144
42. Lv C, Yang J, Kang C, Li Z, Guo L, Wang Y. Determination of 10 flavonoids by UPLC-MS /MS and analysis of polysaccharide contents and compositions in Dendrobii Officinalis Caulis from different habitats. Chin J Exp Trad Med Formulae. (2017) 23:47–52.
43. Zhou C, Xie Z, Lei Z, Huang Y, Wei G. Simultaneous identification and determination of flavonoids in Dendrobium officinale. Chem Cent J. (2018) 12:40. doi: 10.1186/s13065-018-0403-8
44. Ye Z, Dai JR, Zhang CG, Lu Y, Wu LL, Gong AGW, et al. Chemical differentiation of Dendrobium officinale and Dendrobium devonianum by using HPLC fingerprints, HPLC-ESI-MS, and HPTLC analyses. Evid Based Complement Altern Med. (2017) 2017:8647212. doi: 10.1155/2017/8647212
45. Zhang Y, Zhang L, Liu J, Liang J, Si J, Wu S. Dendrobium officinale leaves as a new antioxidant source. J Funct Foods. (2017) 37:400–15. doi: 10.1016/j.jff.2017.08.006
46. Muscella A, Vetrugno C, Cossa LG, Marsigliante S. TGF-beta1 activates RSC96 Schwann cells migration and invasion through MMP-2 and MMP-9 activities. J Neurochem. (2020) 153:525–38. doi: 10.1111/jnc.14913
47. Kalhori V, Tornquist K. MMP2 and MMP9 participate in S1P-induced invasion of follicular ML-1 thyroid cancer cells. Mol Cell Endocrinol. (2015) 404:113–22. doi: 10.1016/j.mce.2015.01.037
48. Cui HY, Wang SJ, Song F, Cheng X, Nan G, Zhao Y, et al. CD147 receptor is essential for TFF3-mediated signaling regulating colorectal cancer progression. Signal Transduct Target Ther. (2021) 6:268. doi: 10.1038/s41392-021-00677-2
49. Tong J, Shen Y, Zhang Z, Hu Y, Zhang X, Han L. Apigenin inhibits epithelial-mesenchymal transition of human colon cancer cells through NF-kappaB/Snail signaling pathway. Biosci Rep. (2019) 39:BSR20190452. doi: 10.1042/BSR20190452
50. Shi X, Luo X, Chen T, Guo W, Liang C, Tang S, et al. Naringenin inhibits migration, invasion, induces apoptosis in human lung cancer cells and arrests tumour progression in vitro. J Cell Mol Med. (2021) 25:2563–71. doi: 10.1111/jcmm.16226
51. Ulgen E, Sezerman OU. driveR: a novel method for prioritizing cancer driver genes using somatic genomics data. BMC Bioinformatics. (2021) 22:263. doi: 10.1186/s12859-021-04203-7
52. Matthias Eder, Scherr M. MicroRNA and lung cancer. N Engl J Med. (2005) 352:2446–8. doi: 10.1056/NEJMcibr051201
53. Bandres E, Cubedo E, Agirre X, Malumbres R, Zarate R, Ramirez N, et al. Identification by Real-time PCR of 13 mature microRNAs differentially expressed in colorectal cancer and non-tumoral tissues. Mol Cancer. (2006) 5:29. doi: 10.1186/1476-4598-5-29
54. Kitade Y, Akao Y. MicroRNAs and their therapeutic potential for human diseases: microRNAs, miR-143 and−145, function as anti-oncomirs and the application of chemically modified miR-143 as an anti-cancer drug. J Pharmacol Sci. (2010) 114:276–80. doi: 10.1254/jphs.10R12FM
55. Sachdeva M, Mo YY. MicroRNA-145 suppresses cell invasion and metastasis by directly targeting mucin 1. Cancer Res. (2010) 70:378–87. doi: 10.1158/0008-5472.CAN-09-2021
56. Chen Q, Zhou L, Ye X, Tao M, Wu J. miR-145-5p suppresses proliferation, metastasis and EMT of colorectal cancer by targeting CDCA3. Pathol Res Pract. (2020) 216:152872. doi: 10.1016/j.prp.2020.152872
57. Guocheng Huang, Benlin Wei, Zebo Chen, Jingyao Wang, Liwen Zhao, Xiqi Peng, et al. Identification of a four-microRNA panel in serum as promising biomarker for colorectal carcinoma detection. Biomark Med. (2020) 14:749–60. doi: 10.2217/bmm-2019-0605
Keywords: Dendrobium officinale, UPLC-ESI-MS/MS, network pharmacology, colorectal cancer, molecular docking
Citation: Tao S, Li J, Wang H, Ding S, Han W, He R, Ren Z and Wei G (2022) Anti-colon Cancer Effects of Dendrobium officinale Kimura & Migo Revealed by Network Pharmacology Integrated With Molecular Docking and Metabolomics Studies. Front. Med. 9:879986. doi: 10.3389/fmed.2022.879986
Received: 20 February 2022; Accepted: 23 May 2022;
Published: 30 June 2022.
Edited by:
Qi Liu, Fudan University, ChinaReviewed by:
Gédéon Ngiala Bongo, University of Kinshasa, Democratic Republic of CongoMaria Eugenia Jaramillo-Flores, Instituto Politécnico Nacional, Ciudad de México, Mexico
Copyright © 2022 Tao, Li, Wang, Ding, Han, He, Ren and Wei. This is an open-access article distributed under the terms of the Creative Commons Attribution License (CC BY). The use, distribution or reproduction in other forums is permitted, provided the original author(s) and the copyright owner(s) are credited and that the original publication in this journal is cited, in accordance with accepted academic practice. No use, distribution or reproduction is permitted which does not comply with these terms.
*Correspondence: Gang Wei, d2VpZ2FuZzAyMUBvdXRsb29rLmNvbQ==