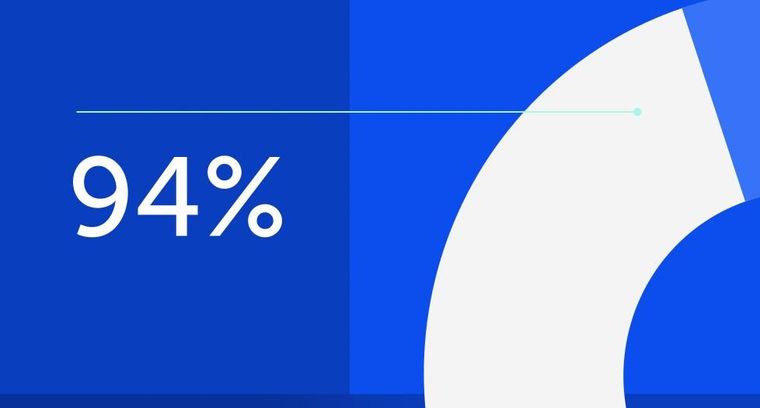
94% of researchers rate our articles as excellent or good
Learn more about the work of our research integrity team to safeguard the quality of each article we publish.
Find out more
REVIEW article
Front. Med., 06 June 2022
Sec. Pulmonary Medicine
Volume 9 - 2022 | https://doi.org/10.3389/fmed.2022.855250
This article is part of the Research TopicInflammatory Markers in Patients with Chronic Respiratory Failure: C-reactive Protein, Procalcitonin, Neutrophil/Lymphocyte ratio in Acute and Chronic State of Pulmonary DiseasesView all 4 articles
Background: Liquid biomarkers have shown increasing utility in the clinical management of airway diseases. Salivary and blood samples are particularly amenable to point-of-care (POC) testing due to simple specimen collection and processing. However, very few POC tests have successfully progressed to clinical application due to the uncertainty and unpredictability surrounding their diagnostic accuracy.
Objective: To review liquid biomarkers of airway diseases with well-established diagnostic accuracies and discuss their prospects for future POC applications.
Methodology: A literature review of publications indexed in Medline or Embase was performed to evaluate the diagnostic accuracy of liquid biomarkers for chronic obstructive pulmonary disease (COPD), asthma, laryngopharyngeal reflux (LPR), and COVID-19.
Results: Of 3,628 studies, 71 fulfilled the inclusion criteria. Sputum and blood eosinophils were the most frequently investigated biomarkers for the management of asthma and COPD. Salivary pepsin was the only biomarker with a well-documented accuracy for the diagnosis of LPR. Inflammatory blood biomarkers (e.g., CRP, D-dimers, ferritin) were found to be useful to predict the severity, complications, and mortality related to COVID-19 infection.
Conclusion: Multiple liquid biomarkers have well-established diagnostic accuracies and are thus amenable to POC testing in clinical settings.
Rapid advancements in genomics, transcriptomics, proteomics, metabolomics, and other “-omics” technologies have allowed for the identification of a vast array of new biomarkers that can be used as tools for improving prevention, diagnosis, prognosis, and management of both non-communicable and communicable airway diseases. It is postulated that this “biomarker revolution” has been paving the way for precision medicine (1). The FDA-NIH Biomarker Working Group defines biomarkers as “defined characteristics that [are] measured as indicator[s] of normal biological processes, pathogenic processes or responses to an exposure or intervention, including therapeutic interventions.” (2) Biomarkers can be useful at all stages of disease progression (Figure 1), including:
(1) For risk assessment of diseases (susceptibility biomarkers).
(2) For detection of diseases (screening and diagnostic biomarkers).
(3) For establishing prognosis (prognostic biomarkers).
(4) For planning treatment (predictive biomarkers).
(5) For monitoring therapeutic and adverse effects related to treatment (response or safety biomarkers).
(6) For assessment of disease status or detection of disease recurrence (monitoring biomarkers) (2).
Point-of-care (POC) technology provides a convenient alternative to centralized laboratory analysis of biological samples. POC technology allows certain molecular and antigen tests to be performed and analyzed rapidly anywhere in a near-patient setting, such as in patients’ homes or at their bedsides (3). Biofluids or liquid biomarkers are particularly amenable to POC testing. Not only are biofluids a rich source of molecular proteins and peptides, but their collection is also relatively simple and minimally invasive. For instance, salivary biomarkers have been proposed to detect and monitor inflammatory and infectious diseases including chronic inflammatory disorders (e.g., inflammatory bowel and periodontal conditions) and sexually transmitted infections (e.g., HIV and HPV) (4–7). The diagnostic accuracy of molecular biomarkers, however, need to be rigorously established before they transition to the convenience of POC testing.
A growing number of liquid biomarkers have been associated with airway diseases. The airway tract is comprised of the organs involved in respiration, speech, and deglutition, including the nose, oral cavity, pharynx, larynx, trachea, bronchi, and lungs (8). Airway and pulmonary diseases may include obstructive airway conditions such as asthma or chronic obstructive pulmonary disease (COPD), infectious diseases such as COVID-19, and chronic chemical irritation such as airway reflux, also known as laryngopharyngeal reflux (LPR). The human airway is lined with airway surface liquid, which plays an important role in the clearing of environmental toxins and defense against foreign particles (9). Local biofluids (e.g., saliva, sputum, mucus, etc.) thus naturally lend themselves as a convenient source of airway liquid biomarkers (10–13). Peripheral biofluids (e.g., blood, serum, urine, etc.) may also provide valuable information regarding systemic processes that contribute to airway diseases (14–16) (Figure 2).
Although biofluid samples can be easily obtained in physicians’ offices or outside of the healthcare setting (e.g., through sputum induction), POC testing has yet to be made widely available for the management of airway diseases. A long-standing and legitimate barrier to the implementation of POC testing is the uncertainty surrounding the diagnostic accuracy of liquid biomarker measurements (17). In this review, we aim to provide peer-reviewed evidence regarding the clinical utility of airway liquid biomarkers with well-established diagnostic accuracies that would be readily translated to POC testing. We first survey recent literature on liquid biomarkers in COPD, asthma, airway reflux, and COVID-19 and their diagnostic accuracies. Then, we discuss several emerging POC platforms under development and present prospects of future POC applications for liquid biomarkers in airway diseases.
Four common airway and pulmonary conditions, namely asthma, COPD, LPR, and COVID-19, were included in this review. The biomarkers of these four conditions were the most abundantly researched and could be tested through biomarker detection devices available on the market.
A review of the literature was performed to identify relevant studies reporting on the diagnostic accuracy of liquid biomarkers for airway diseases. The PRISMA (Preferred Reporting Items for Systematic reviews and Meta-analyses) framework was used to guide the search and the reporting of the review (18). Two databases, namely Medline and Embase, were searched for references published from 2000 to 2021, respectively. The following terms were searched: “biological marker”; “biomarker”; “biologic marker”; “diagnostic accuracy”; “sensitivity and specificity”; “sensitivity”; “specificity”; “laryngopharyngeal reflux”; “proximal reflux”; “hypopharyngeal reflux”; “extra-esophageal reflux”; “reflux laryngitis”; “COVID-19”; “coronavirus disease 2019”; “COPD”; “chronic obstructive lung disease”; “chronic obstructive pulmonary disease”; “emphysema”; “chronic bronchitis”, and “asthma” (Supplementary Table 1).
Studies were included if they reported the diagnostic accuracy of at least one liquid biomarker by providing measures of sensitivity, specificity, positive predictive value (PPV), negative predictive value (NPV), and area under the ROC curve (AUC). Studies were required to include human adult subjects with a diagnosis of COPD, asthma, laryngopharyngeal reflux, or COVID-19 infection. Only articles written in English were included due to limited resources for the translation of studies.
Studies that did not report a complete set of diagnostic accuracy measures (i.e., sensitivity, specificity, PPV, NPV, and AUC) for at least one liquid biomarker were excluded. Studies conducted in animal or pediatric populations were excluded. Articles written in languages other than English were also excluded.
Sources were assessed in a two steps-process to determine their relevance to this study’s objectives. The first step (screening) included a review of titles and abstracts to discard sources that did not match the inclusion criteria. The second step (eligibility assessment) consisted of a full-text assessment of all remaining articles against the eligibility criteria.
The literature search yielded 3,628 results that were imported into the Covidence systematic review software Version v2625 (Veritas Health Innovation, Melbourne, Australia) for screening and eligibility assessment (Figure 3). Nine references were identified by hand-search or by expert recommendations. A total of 1,459 duplicates were removed by the automated software. After the removal of duplicates, 2,178 different studies were screened. The first step of the screening process yielded 435 studies that were assessed for eligibility by full-text reading. After full-text eligibility assessment, 71 studies were included for this review.
Chronic obstructive pulmonary disease (COPD) refers to a group of poorly reversible and progressive respiratory conditions, mostly seen in patients with dyspnea, chronic cough, sputum production, and a history of exposure to risk factors of the disease (such as primary or secondary cigarette smoke exposure) (19, 20). The pathophysiology of COPD is associated with multiple factors such as genetic susceptibility, abnormal lung development, cellular senescence, and chronic environmental insults to the lungs, all of which can contribute to the development of chronic pulmonary inflammation (19). Clinical diagnosis of COPD is based on documented persistent airflow limitation on spirometry (19). Inflammatory biomarkers such as high sensitivity C-reactive protein (hs-CRP) have been studied for their ability to diagnose COPD, with moderate specificities (75–83%) but low sensitivities (42–50%) (21) (Table 1).
Table 1. Diagnostic accuracies of liquid biomarkers for chronic obstructive pulmonary disease (COPD).
Various COPD classification systems have been proposed over the years, moving away from the classical binary classification of COPD as emphysema and chronic bronchitis (22). Some of these phenotypes include the asthma-COPD overlap syndrome (ACOS), the non-exacerbator phenotype, the frequent exacerbator with emphysema phenotype, and the frequent exacerbator with chronic bronchitis phenotype (22–24). Blood eosinophils can be used to diagnose ACOS and eosinophilic COPD, with sensitivities of 29–55% and 77%, respectively, and specificities of 71–95% and 63–76%, respectively (25–27). Blood sRAGE has been studied for its ability to distinguish the frequent exacerbator COPD phenotype from non-frequent exacerbator COPD phenotype and was found to have a moderate sensitivity of 80%, with a high specificity of 93% (28).
The natural history of COPD is punctuated by periods of acute worsening of respiratory symptoms called acute exacerbations (AECOPD), and a gradual decline in lung function, all of which can eventually lead to death (29). In the US and Canada, the average cost of a severe COPD exacerbation was estimated to be over $18,000 and $9,500, respectively (30, 31). For this reason, there has been a growing interest in identifying biomarkers allowing for early diagnosis of COPD exacerbations. Blood biomarkers, such as neutrophil to lymphocyte ratio (NLR), C-reactive protein (CRP), leukocyte counts, and mean platelet volume, have all been identified as promising biomarkers of COPD exacerbations, with moderate sensitivities (71–80%) and specificities (71–82%) (32, 33).
Other blood biomarkers have been studied for their ability to diagnose complications among AECOPD patients, such as left and right ventricular failure and pulmonary hypertension (34–37). CA-125 was found to be highly accurate for the diagnosis of right ventricular failure, while NT-pro BNP was found to be moderately accurate for the diagnosis of left ventricular systolic failure, with sensitivities of 90 and 70%, and specificities of 86 and 83%, respectively (34, 35). Several other peripheral biomarkers such as procalcitonin, CRP, mean corpuscular hemoglobin concentration (MCHC), and blood cell count ratios have been identified as potential prognostic biomarkers for AECOPD patients (38–41). CRP, neutrophil to lymphocyte ratio (NLR), platelet to lymphocyte ratio (PLR), and MCHC can all be used to predict in-hospital mortality among AECOPD patients with sensitivities ranging from 59 to 89% and specificities ranging from 52 to 81% (38, 39, 41). Procalcitonin can be used to predict non-invasive mechanical ventilation (NIMV) failure in AECOPD patients with a sensitivity of 83% and a specificity of 84% (38). More multicentric research is needed in order to confirm the clinical utility of these biomarkers.
The measurement of COPD exacerbation biomarkers is not yet employed in routine clinical practice. Aside from lung function parameters, very few biomarkers are routinely used for the clinical management of COPD (19). The 2021 GOLD guidelines recommend the utilization of blood eosinophil counts to guide the prescription of inhaled corticosteroids (ICS) for COPD patients (19). Blood eosinophils are among the biomarkers with the best-established diagnostic accuracies in COPD: A blood eosinophil count <100 cells/μL can help to identify patients who are unlikely to respond to treatment with ICS, with a sensitivity of 100%, while a blood eosinophil count >300 cells/μL can help to identify patients with the greatest likelihood of benefiting from treatment with ICS (19, 42).
To date, the implementation of biomarkers in clinical practice for COPD has proven to be difficult, with available data on COPD biomarkers being complex to interpret, largely as a result of weak associations and lack of reproducibility between large patient cohorts (19). Studies varied greatly in terms of sample size, ranging from 5 to 101 COPD cases, biomarker studied (14 different biomarkers or scores evaluated across 18 studies), and biomarker utility (n = 2 studies assessing diagnostic biomarkers for COPD, n = 2 studies for AECOPD, n = 5 studies for AECOPD complications, n = 4 studies for COPD phenotype, and n = 5 studies for COPD prognostics). The collection of a plethora of detailed genetic and biochemical data from large cohorts (such as COPDGene and SPIROMICS cohorts) have led to an explosion of smaller studies investigating newer biomarkers (e.g., CC16, SP-D, IL-6, fibrinogen, etc.), which unfortunately often remain with uncertain diagnostic accuracies (43–45). Fibrinogen and IL-6, in particular, have been the subject of increasing research interest. However, no studies provided a complete set of diagnostic accuracy measures (i.e., sensitivity, specificity, PPV, NPV, and AUC) at the time of this review. Rigorous and exhaustive diagnostic accuracy studies are warranted to confirm the clinical utility of these biomarkers. In addition, the COPD Biomarker Qualification Consortium has identified several other promising biomarkers, such as desmosine and sRAGE, which can be prioritized for future COPD biomarker research (46). It is hoped that the discovery and implementation of appropriate COPD biomarkers will lead to improved diagnosis, risk stratification, management, and prognosis, thus enabling personalized medicine and leading to better outcomes in COPD patients.
Asthma is one of the most prevalent chronic diseases worldwide (47). Diagnosis is conventionally established based on suggestive clinical symptoms and the evidence of variable expiratory airflow limitation on pulmonary function testing (47).
Although the use of biomarkers for the diagnosis of asthma is rather limited in clinical practice, related studies have been flourishing over the past few years. For instance, atopy-related biomarkers, such as eosinophils, periostin, IgE, and thymus, and activation-regulated chemokine (TARC), and others were proposed for the diagnosis of asthma (12–14, 48–50) (Table 2). For the diagnosis of occupational asthma (OA), sputum eosinophilia following specific inhalation challenges demonstrated high specificities (86–97%) but low sensitivities (57–67%) (51, 52).
Asthma is now recognized as a heterogeneous disease characterized by distinctive endotypes and phenotypes. Asthma endotypes can be broadly categorized into T2-high and non-T2 asthma (53) Airway eosinophilia is typically associated with T2-high asthma, while airway neutrophilia is more commonly seen in non-T2 asthma (47). Asthma phenotypes often falling under the T2-high category include early-onset atopic asthma, late-onset eosinophilic asthma, and aspirin-exacerbated respiratory disease (AERD) (53). Asthma phenotypes often falling under the non-T2 category have been categorized according to associated clinical characteristics and include smoking-related asthma, obesity-related asthma, and elderly asthma (53). Of note, this asthma classification model is based on frequent associations between endotypes and clinical manifestations (phenotypes) with known exceptions. A better understanding of asthma biomarkers and the mechanism to which they are a testimony in the pathogenesis of the disease could eventually allow for a more precise classification of asthma and subsequent phenotypes.
Asthma biomarkers have been proposed to classify the disease according to endotypes and phenotypes. Being able to make such classification is notably useful in the health care setting due to their inherent therapeutic and prognostic implications and may provide the basis for personalized medicine (54). While peripheral neutrophil counts are not routinely used in clinical practice, the current international Global Initiative for Asthma (GINA) guidelines recommends the use of eosinophils in the diagnosis and management of moderate or severe asthma to:
(1) Confirm refractory type 2 inflammation (blood or sputum eosinophils).
(2) Assess severe asthma phenotypes (blood or sputum eosinophils).
(3) Adjust treatment for adults with persisting symptoms and/or exacerbations despite high dose ICS or ICS-LABA in the presence of sputum eosinophilia (>3%).
(4) Increase ICS dose independently of the level of symptom control in adults with sputum eosinophilia (>3%).
(5) Guide treatment for adults with moderate to severe asthma who are treated or can be referred to centers experienced with sputum induction testing.
(6) Confirm type-2 inflammatory phenotype in patients refractory to high dose ICS-LABA before prescribing type-2 biologic targeted therapy, such as anti-IL5, anti-IL5R, or anti-IL4R (must have blood eosinophilia but cut-off points may vary according to location) (47).
Sputum eosinophils are generally considered to be reliable biomarkers of Th2 airway inflammation in asthma (47). In order to perform sputum induction, the patient is required to inhale a hypertonic solution by nebulization, which helps to produce sputum that can then be expectorated (55). However, sputum induction testing has the disadvantage of not being readily available in primary care (47). Moreover, sputum induction’s success rate is only around 80%, due to difficulties that may arise during the sampling process (56). For instance, certain patients may experience bronchoconstriction due to the inhalation of hypertonic saline, and others may be unable to produce sufficient saliva for sputum analysis (55).
Blood eosinophils were thus proposed as peripheral surrogate biomarkers for sputum eosinophilia. Overall, studies evaluating the accuracy of blood eosinophils to detect sputum eosinophilia have found moderate to high sensitivities and specificities, ranging from 68 to 86% and 66 to 91%, respectively, for cut-off values between 0.19×109/L and 0.33×109/L (57–62). The use of higher cut-off values for blood eosinophils was associated with higher specificities (91–97%) but lower sensitivities (26–57%) (61, 63, 64). Some other diagnostic biomarkers that have been investigated for T2-high asthma include sputum basophil counts, serum periostin, blood IgE, type-2 innate lymphoid cell counts, and blood cell count ratios (58, 60–62, 64–66).
In addition to asthma endotyping, liquid biomarkers are also used for asthma phenotyping. For the diagnosis of AERD, a T2-high asthma phenotype, it was observed that different combinations of single-nucleotide polymorphism (SNPs) found in blood could differentiate AERD patients from aspirin-tolerant asthma patients with relatively low sensitivities of 34–65%, but high specificities of 85–93% (67, 68). Regarding non-T2 asthma phenotypes, peripheral neutrophil counts, and peripheral lymphocyte counts were found to predict airway neutrophilia with a 61.5 and 65.9% sensitivity, respectively, and a 63.2 and 48% specificity, respectively (62). Their combined ratio, i.e., neutrophil to lymphocyte ratio (NLR), presented a slightly better sensitivity (76.9%) but lower specificity (41.6%) for the prediction of airway neutrophilia (62).
Asthma biomarkers can also be used to predict response to pharmacological treatments (69, 70). Sputum and blood eosinophils have demonstrated sensitivities of 50–79% and specificities of 69–92% for the prediction of responsiveness to oral steroid treatment (70). Finally, asthma biomarkers, such as blood Il-13 and sputum eosinophils, were also proposed to monitor disease state, with highly variable sensitivities (63–94%) and specificities (55–95%) (15).
Included studies were highly heterogenous in terms of population studied, with sample sizes ranging from 6 to 562 cases, and studies including patients with various degrees of asthma control and subtypes of disease, e.g., occupational, and non-occupational, eosinophilic, neutrophilic, or AERD asthma phenotypes, etc. Moreover, few studies evaluated the same biomarkers for the exact same purposes. Overall, 23 different biomarkers or biomarker scores were evaluated across 29 studies. While eosinophils were some of the most frequently studied biomarkers (n = 17 studies), they were evaluated for a wide range of clinical purposes ranging from diagnosis of occupational asthma, non-occupational asthma, and asthma phenotype to the prediction of responsiveness to oral steroids, and the monitoring of disease control. This heterogeneity likely contributed to the high degree of variability in diagnostic accuracies reported across the literature.
In recent years, considerable progress has been made in our understanding of asthma with respect to its mechanistic pathways (endotypes) and clinical manifestations (phenotypes). However, these advances have predominantly been made in T2-high asthma, which is reflected in our literature search highlighting mainly type 2 inflammatory biomarkers such as eosinophils, IgE, periostin, T2-ILC, and IL-13. New serum biomarkers such as IL-17, chitinase-3-like protein 1, and ceramide to sphingosine-1-phosphate ratio are also proposed as diagnostic biomarkers for T2-low asthma (71). Large cohort studies have also investigated other T2-high biomarkers such as CCL26, eosinophil-derived neurotoxin, and IL-4, which unfortunately still have uncertain diagnostic accuracies (71). More comprehensive research is still needed to ascertain the diagnostic accuracy, and thus, the clinical utility of these novel biomarkers. Biomarkers in other forms, e.g., the fraction of nitric oxide (FeNO) from exhaled breaths, have well-researched diagnostic accuracies for T2-high asthma. However, gas biomarkers are out of the scope of this review.
Nevertheless, current research is increasingly shifting away from the evaluation of single diagnostic biomarkers toward the multidimensional assessment of a combination of biomarkers (72). New diagnostic algorithms and tools composed of multiple biomarkers can be developed to better classify asthma according to endotype and phenotype, which would considerably increase diagnostic accuracies and be possible via microfluidic POC platforms with multiplex capability (72).
Laryngopharyngeal reflux refers to the retrograde flow of stomach contents into the laryngopharynx, where the refluxate meets the upper aerodigestive tract (73). LPR can be conceptualized as a supra-oesophageal manifestation of gastroesophageal reflux disease (GERD). However, LPR has recently been recognized as a distinct clinical entity from GERD, due to the numerous differences in the pathogenesis, clinical presentation, and outcomes between both diseases (73).
Three different constituents of refluxate are thought to mediate the majority of laryngeal symptoms seen in LPR: hydrochloric acid, pepsin, and bile salts (74). While intragastric pH can be as low as 1.5, the pH of the healthy laryngopharynx is neutral, and its epithelium can easily be damaged by gastric acidity (74). In contrast with the resistant epithelium of the oesophageal mucosa, laryngeal and pharyngeal epitheliums are significantly more vulnerable to damage from gastric acidity. Even a short exposure to gastric refluxate could lead to significant laryngopharyngeal damage (74).
The diagnosis of LPR is usually based on clinical symptoms, suggestive laryngoscopy findings, or 24-h pH monitoring, with or without multichannel intraluminal impedance (MII) (75). The 24-h combined hypopharyngeal-oesophageal multichannel intraluminal impedance with dual pH probe (24h-HEMII-pH) is often considered to be the diagnostic gold standard for LPR for its ability to detect both acid and nonacid reflux (74). It is generally preferred over stand-alone 24-h pH monitoring because approximately half of the patients with LPR may suffer from non-acidic reflux (74, 76). Moreover, when used independently, the proximal and distal oesophageal pH monitoring probes can be unreliable, with at best, a 75 and 50% sensitivity, respectively (76). The oesophageal pH monitoring test, with or without oesophageal impedance, is also not readily available in clinical practice, expensive, and not always tolerated by the patients due to its invasive nature (77, 78).
Laryngoscopic examination is more readily available than 24h-HEMII-pH and is considered to be an important screening tool for the diagnosis of LPR (79). However, laryngoscopic examination for the diagnosis of LPR has been associated with poor sensitivity and specificity of 40 and 50%, respectively (80). Indeed, the laryngoscopic signs associated with LPR are often nonspecific and can also be found in patients with other causes of laryngeal irritation such as phonotrauma, smoking, allergy, infection, and even in healthy individuals (75). Laryngoscopic findings of LPR can also be highly subjective with poor inter-rater reliability between examiners (75, 81). Belafsky’s reflux finding score (RFS), a standardized 8-item scoring system for laryngoscopy findings of LPR, was developed in an attempt to overcome this issue (82). Belafsky’s RFS has shown an increased sensitivity of 87.8% for detecting LPR in comparison with laryngoscopic examination, but its specificity was not improved (37.5%) (74, 83). As for the use of questionnaires, studies have shown variable RSI scores with a specificity ranging between 20 and 83% when used independently for the diagnosis of LPR (84, 85).
It has thus been argued that there is currently no ideal procedure for the diagnosis of LPR, with each existent diagnostic method involving its own limitations (74). For this reason, the use of biomarkers to diagnose LPR has gained considerable attention. Salivary pepsin, in particular, has been identified as a promising diagnostic biomarker for LPR.
Pepsin is a proteinase secreted by the chief cells of the gastric fundus and body (74). It is first secreted as an inactive zymogen called pepsinogen, which gets subsequently activated by gastric acidity (78). In patients with LPR, pepsin is often detectable in the saliva, which is an indicator of recent refluxate in the oropharynx or in the oral cavity where salivary samples are collected (83). However, the sensitivity and specificity of salivary pepsin for the diagnosis of LPR is highly variable and depends on which “gold standard” diagnostic methods were used to establish the corresponding diagnostic accuracy.
When 24-h pH monitoring is used as a gold-standard method to establish the diagnosis of LPR, the sensitivity and specificity of salivary pepsin vary between 42–85% and 28–86%, respectively (86). When establishing the diagnosis of LPR based on clinical symptoms only, the detection of salivary pepsin has a sensitivity of 40–48% and a specificity of 95–98% (11) (Table 3). The limited number of studies focusing on salivary pepsin, their variability in control groups (symptomatic patients vs. otolaryngology clinic patients) and reference tests employed may explain the wide range of reported diagnostic accuracies.
Due to the imperfect sensitivities and specificities of the gold-standard diagnostic methods used for comparison in these studies, the true diagnostic accuracy of salivary pepsin is difficult to determine. At the same time, the wide variability of salivary pepsin diagnostic accuracy values reported in the literature may complicate the interpretation of test results and may justify advocating for its use in combination with other biomarkers or diagnostic methods. Other emergent biomarkers, such as carbonic anhydrase type III, e-cadherins, mucins, and interleukins were also suggested as potential diagnostic biomarkers for LPR (74). However, to our best knowledge, the diagnostic accuracy of these new biomarkers has not yet been established in large patient cohorts. More research on the role of individual biomarkers and their multidimensional assessment in association with clinical signs, symptoms, and pH impedance is needed in order to develop a robust diagnostic algorithm for LPR (87).
Since the onset of the COVID-19 pandemic in March 2020, nearly 280 million people have contracted the disease and nearly 5.5 million COVID-19-related deaths have been recorded worldwide (as of January 10th, 2022) (88). In an effort to curb the spread of the disease, unprecedented scholarly efforts have been mobilized to investigate the pathophysiology of the viral infection and to develop rapid diagnostic methods for early detection of the disease. The diagnosis of COVID-19 infection is most commonly made on the basis of nucleic acid amplification testing (NAATs), antigen testing, or serology testing (89). To date, over 1,150 COVID-19 diagnostic tests have been commercialized, among which over a thousand have been approved by regulatory agencies in Europe or North America (90). Of these, 23% are serological tests, 45% are NAAT-based methods for RNA detection, and 31% are antigen detection tests (90). Most of these diagnostic tests have high diagnostic accuracies. A 2021 meta-analysis revealed that combined IgG and IgM serological testing yielded a sensitivity and specificity of 84.5 and 91.6%, respectively (89). Sputum PCR testing presented a sensitivity of 97.2%, with the specificities of most PCR tests being 100%, regardless of sample type (89). Interestingly, peripheral non-specific markers, such as CD169Mo ratio, CRP, and neutrophils have also shown high sensitivities (81–91%) for the diagnosis of COVID-19 infection, but highly variable specificities (55–90%) (91–93) (Table 4).
While the development of a vast array of diagnostic tests has allowed for increased rapidity and accuracy of diagnosis, significant progress remains to be made when it comes to the risk-stratification of infected patients. COVID-19 is not only a localized respiratory infection, but also a multisystem inflammatory disease involving complex immunological, inflammatory, and coagulative cascades (94). Of patients contracting COVID-19, a significant proportion will require hospitalization, intensive care unit (ICU) admission, and invasive mechanical ventilation (95). A significant proportion of COVID-19 patients will also develop severe and life-threatening complications such as acute kidney injury, coagulopathies, myocardial infarction, stroke, venous thrombosis, and acute respiratory distress syndrome (95). Our ability to predict which patient will develop severe COVID-19 disease remains limited. Several biomarkers of COVID-19 infection have thus been identified for their ability to predict disease progression and mortality.
Various peripheral blood biomarkers such as serum CRP, D-dimers, fibrinogen, procalcitonin, cytokines, electrolytes, blood cell counts, and blood cell count ratios have been studied for their ability to predict disease progression from non-severe COVID-19 to more severe forms of the disease (96–105). D-dimers have demonstrated moderate to high sensitivities of 82–91% and specificities of 66–88% to predict disease progression (100, 102). Similarly, CRP values between 3.69 and 22.41 mg/dl have been shown to predict disease severity with high sensitivities (83–93%), but inconsistent specificities (50–91%) (96, 98–100). Across different studies, CRP’s sensitivity for the prediction of mortality ranged from 42 to 97%, and its specificity ranged from 23 to 83% (104, 106–108).
Other candidate biomarkers for the prediction of mortality include ferritin, inflammatory cytokines (i.e., IL-2R, IL-6, IL-8, IL-10, TNF-a), troponin 1, mid-regional proadrenomedullin, blood cell counts, and blood cell count ratios (101, 102, 106–117). High levels of ferritin (1,288.5–1,799 ng/ml) have demonstrated a moderate diagnostic accuracy for the prediction of mortality with a sensitivity of approximately 70% and a specificity of 64–75% (101, 104). Lower ferritin cut-off values were less promising in terms of sensitivity (56–74%) and specificity (36–60%) (115). Increased serum D-dimers were also able to predict COVID-19 mortality with moderate sensitivities of 62–75% and specificities ranging from 72 to 84%.(106, 108) Additionally, D-dimer levels between 1,500 and 3,300 ng/ml have shown moderate to high sensitivities (70–96%) for the prediction of thrombotic events in COVID-19 patients. However, the associated specificities remained low to moderate, ranging from 22 to 69% (118–121).
To date, most risk-stratification clinical tools for the prediction of COVID-19 severity have used an imperfect combination of few clinical and biological variables. There is an urgent need for the development of accurate risk-stratification algorithms allowing for the early identification of patients at risk of severe COVID-19 disease, particularly in a context of limited human and material resources. More research on prognosis biomarkers of COVID-19 will allow for better integration of clinical and laboratory data and the creation of comprehensive and accurate risk-stratification algorithms. These risk-stratification systems could play a decisive role in the planning of patient management and future utilization of available resources.
As the field of liquid biomarkers continues to advance, novel biomarkers are discovered with uncertain diagnostic accuracies or cohort reproducibility (17). In the past, very few guidelines or protocols have been developed to oversee the direction of such research. Scientific data is still considered relatively insufficient to fully support the use of most biomarkers in clinical settings. In light of these challenges, health regulatory bodies (e.g., the U.S. Food and Drug Administration) and funding agencies (e.g., the Foundation for the National Institutes of Health) have developed a Biomarker Qualification Program and a Biomarkers Consortium, respectively, to facilitate biomarker development and standardization for clinical diagnostics and drug monitoring (122, 123). For translation into clinical practice, a biomarker needs to fulfill specific clinical and industrial standards through a process of discovery, analytical validation, clinical validation, and be reviewed by relevant health authorities for qualification (124). A rigorous validation and qualification process is necessary, but also leads to multiple scientific and institutional challenges for clinical translation (Figure 4). Nevertheless, relevant stakeholders (e.g., researchers, clinicians, patients) should be made aware of these limitations and ensure that practical steps (e.g., reporting both positive and negative findings, using robust analytical techniques) are taken in the early development phase of biomarkers (17, 125–127). For instance, analytical validation involves the assessment of the test platform used to measure the biomarker in terms of accuracy, reproducibility, dynamic range, and variability (124). Clinical validation is needed to ensure the consistency and accuracy of the test in predicting the clinical outcome that it is intended to reflect and further characterizes diagnostic accuracy (124). Qualification allows for the identification of biomarkers that can be relied on for a specific interpretation and application within a specific context of use by relevant health authorities (124, 128). As such, a prioritization of biomarkers to be used with defined purposes (e.g., disease phenotyping vs. risk stratification) in specific contexts is recommended at an early stage of the biomarker development process to facilitate validation and qualification.
Figure 4. Challenges in biomarker discovery, validation, and qualification for clinical translation.
Current clinical biomarker detection methods vary based on the health condition tested for and the specific markers of interest. For example, LPR detection commonly utilizes enzyme-linked immunosorbent assays (ELISAs) and lateral flow assays (LFAs) such as the Peptest ® to monitor pH levels and pepsin concentration (129). Similarly, COVID-19 diagnosis relies primarily on various NAATs such as real-time reverse-transcription PCR or loop-mediated isothermal amplification (LAMP), but also leveraging antigen/antibody testing via ELISAs, LFAs, neutralization bioassays, and chemiluminescent immunoassays (130, 131). Each format has associated advantages (e.g., speed, multiplexing, automation) and disadvantages (e.g., trained personnel and dedicated laboratories). For instance, traditional ELISAs take several hours to complete and require dedicated equipment and trained personnel, which limits same-day diagnosis capacity for clinics lacking such resources. In contrast, LFAs offer rapid testing but suffer from reduced sensitivity and accuracy that may necessitate further validation for a reliable diagnosis (132, 133). Additionally, LFAs results are generally qualitative or semi-quantitative, which can be problematic for diseases that require accurate, quantitative results to provide a correct diagnosis and treatment plan (134–136). Irrespective of the test format, the sample requirements (acquisition/preparation/volume) can vary significantly. In some cases, sample acquisition can cause notable patient discomfort such as the mid-turbinate swab required for certain COVID-19 tests (131). The physical properties of a sample may also dictate the speed of the assay with, for example, viscous samples prolonging analysis (137).
To address the limitations of current methods, novel microfluidic detection devices are being actively explored to develop POC testing capable of delivering accurate, rapid, inexpensive diagnoses (138–142). Microfluidic devices include microfabricated fluid channels capable of efficiently manipulating small amounts of fluids for biochemical reactions (143). It should be acknowledged that although LFAs do not fit this conventional definition, they do have a root in microfluidics (144). These microfluidic platforms possess several critical advantages for in-vitro diagnostics over conventional clinical practices (including LFAs). Firstly, they can handle small sample volumes with significant spatial-temporal accuracy in a high-throughput manner—notable criteria of major diagnostic companies (130, 145). The improved sensing parameters those microfluidic devices provide are partly attributable to the precise control of fluid quantities and sample/reagent flow rates, which enable the separation and detection of analytes with high accuracy and sensitivity (146). Furthermore, these systems can deliver diagnostic results within minutes and are generally fabricated from inexpensive polymers (e.g., PDMS or polycarbonate) or cellulose (e.g., paper or thread) substrates (147–150). Finally, microfluidic platforms offer the distinct benefit of having multiplexing capacity. For example, multiple biomarkers of a given disease or infection can be detected simultaneously through several channels of a single device, which could significantly enhance the accuracy of diagnosis and reduce the risk of false-positive and false-negative results (151). An alternative multiplexing method developed combined bead capture and sonic mixing technology into a single device to detect cancer biomarkers within 20 min at a sensitivity level up to 0.028 ng/mL (147). Notably, this device had the capacity to analyze up to 500 biomarkers simultaneously (147, 152). A further multiplex microfluidic detection system used microfluidic cassettes, in combination with microarray technology, to detect tumor markers and demonstrated comparable results with standard, slower clinical instruments (148). As no liquid exchange occurs between the disposable cassette and handheld device, cross-contamination between samples is minimized. Fundamentally, these examples demonstrate the significant scope and flexibility of different microfluidic technologies that can be harnessed to develop multiplexed detection systems.
Microfluidic devices have been proposed to analyze biomarkers present in various biofluids including saliva, sputum, and blood (153–156). Blood has traditionally been the predominantly studied fluid in biomarker research due to the simplicity of sample collection and analysis. Most biomarkers with well-established diagnostic accuracy for COPD, asthma, LPR, and COVID-19 are thus still from blood, rather than from saliva, sputum, or other local surface fluids, with the exception of a few sputum biomarkers in asthma, and saliva biomarkers in LPR. In fact, saliva- and sputum-based biomarkers have been increasingly recognized as “a window on health status” in molecular diagnostics (157). For instance, laryngeal surface secretions have been evaluated for injury-induced inflammation of vocal folds in humans (158). Further efforts to establish the reliability and accuracy of saliva and sputum-based biomarkers of airway diseases will fulfill a true promise of point-of-care diagnosis for patients and clinicians.
By analyzing specific biomarkers, several devices have been applied for diagnosing asthma, COPD, and COVID-19 (156, 159, 160). For instance, a recently developed COVID-19 diagnostic tool utilized a microfluidic chip and Raman spectroscopy to provide an integrated platform that can trap viruses from biological fluids and identify patient infection status within minutes (161). A notable advantage of this system is that, as the detection is based upon the physical properties of the virus, accurate COVID-19 detection is possible across multiple variants. Equally, the platform could be readily adjusted to capture other viruses or bacteria relevant for other infections. Comprehensive reviews of the growing number of microfluidic devices applied for biomarker analysis are available elsewhere with an understanding that very limited products reached commercialization standards (139, 141, 145, 162–165).
The simplicity of POC technology is critical to promoting its accessibility and devolving testing away from laboratories to help streamline the future of diagnostic technology (166). As these devices are intended to be operable by both patients and clinicians alike, it is critical that no convoluted training and equipment is required for their use (167). Research efforts continue to target the development of devices capable of delivering accurate results in a compressed timescale. For instance, the SARS-CoV-2 lateral flow rapid tests that have become widely available enable users to perform a simple self-test at home and achieve a result within 15 min. However, it should be noted that whilst huge advances have been made in rapid, self-testing since the onset of the SARS-CoV-2 pandemic, RT-PCR analysis remains the gold standard for diagnosis. In the case of SARS-CoV-2 testing, one study found that four different kits reported sensitivity values only in the range of 44.6–54.9% (168). In comparison, RT-PCR has previously been found to have a sensitivity of 97.2% when testing sputum samples (89). As such, the sensitivity of rapid testing currently limits it to functioning as an initial diagnosis that requires further confirmation via conventional laboratory testing.
Point-of-care research also aims to diversify the output that a single sample can provide by expanding the multiplex capacity of testing platforms (169). If multiplex devices with high fidelity become widely available, POC technology can help minimize diagnostic wait times and enable patients to receive treatment far sooner than sequential, lengthy testing procedures would otherwise allow (170). The access of multiplex POC technology will be a gamechanger for chronic and complex disease diagnosis and monitoring. Unfortunately, a drawback of multiplex devices is that they are much more susceptible to cross-contamination, which could severely impair the accuracy of the result (171). To address this, device architecture can be modified with, for example, hydrophobic barriers that ensure reagents and analytes are restricted to flow along a defined path only that prevents mixing (172, 173).
Aside from the technical challenges that remain for POC testing, administrative obstacles must be realized and overcome to deploy POC devices to the general public. A top-down approach driven by a limited number of health professionals has proven ineffective in practice (174). Establishing an effective POC testing network requires a well-structured, multi-disciplinary governance encompassing both clinical and managerial support. For instance, allocating human resources of staff training on POC devices is needed to keep the POC testing network sustainable. Communicating the availability of POC testing to patients is also critical in ensuring a high uptake of community use. This is notably true for promoting its use in isolated rural areas where the introduction of POC testing is predicted to have the greatest impact (175).
The landscape of biomarkers for airway diseases remains extremely vast and highly heterogeneous. Nonetheless, research efforts and financial investments have facilitated the discovery of a variety of promising biomarkers, among which a few have well-circumscribed diagnostic accuracies and can reliably be used clinically. For communicable airway diseases like COVID-19, diagnosis is largely based on direct detection of the viral load whilst supplementary biomarkers are needed for risk stratification and severity prediction. For non-communicable airway diseases, liquid biomarkers have various clinical applications. For instance, sputum and blood eosinophils can be used for the phenotyping and endotyping of asthma, blood eosinophils to guide management of COPD, and salivary pepsin to diagnose LPR.
Despite considerable progress, the majority of microfluidic POC devices remain at a pre-clinical, developmental stage. The lack of standardization across device fabrication and the difficulty in incorporating multiple testing components into a single device present obstacle for manufacturing and scaling-up production. From a testing standpoint, the technical complexity and validation challenges remain limiting factors for device translation to clinical settings. That said, the field of diagnostics continues to transition toward detection devices based on ease of use, low sample volume, and rapid results. Given the ongoing drive to develop microfluidic systems that are simple to use and sensitive, microfluidic systems will continue to trend toward becoming an indispensable part of the healthcare industry as a critical element of POC-based disease diagnosis and pathogen detection in airway diseases.
VL and NL-J conceived the manuscript. VL, PC, and NL-J wrote the manuscript. VL designed the figures and tables. All authors read and approved the manuscript.
The study was funded by the Canada Research Chair (NL-J) and grants CIHR PJT-159475 (XL) and CIHR PJT-156412 (NL-J) from the Canadian Institutes of Health Research.
The presented content is solely the responsibility of the authors and does not necessarily represent the official views of the above funding agencies.
The authors declare that the research was conducted in the absence of any commercial or financial relationships that could be construed as a potential conflict of interest.
All claims expressed in this article are solely those of the authors and do not necessarily represent those of their affiliated organizations, or those of the publisher, the editors and the reviewers. Any product that may be evaluated in this article, or claim that may be made by its manufacturer, is not guaranteed or endorsed by the publisher.
The Supplementary Material for this article can be found online at: https://www.frontiersin.org/articles/10.3389/fmed.2022.855250/full#supplementary-material
1. Schisterman EF, Albert PS. The biomarker revolution. Stat Med. (2012) 31:2513–5. doi: 10.1002/sim.5499
2. NIH. BEST (Biomarkers, EndpointS, and other Tools) Resource. FDA-NIH Biomarker Working Group. Silver Spring, MD: Food and Drug Administration (2016).
3. St John A, Price CP. Existing and emerging technologies for point-of-care testing. Clin Biochem Rev. (2014) 35:155–67.
4. Nijakowski K, Surdacka A. Salivary biomarkers for diagnosis of inflammatory bowel diseases: a systematic review. Int J Mol Sci. (2020) 21:7477. doi: 10.3390/ijms21207477
5. Kim JJ, Kim CJ, Camargo PM. Salivary biomarkers in the diagnosis of periodontal diseases. J Calif Dent Assoc. (2013) 41:119–24.
6. Prasad P, D’Souza V, Mithra P, Radhakrishnan R. Salivary biomarkers associated with the progression of disease in people living with HIV: a scoping review [version 1; peer review: 1 approved, 1 not approved]. F1000Research. (2021) 10:130. doi: 10.12688/f1000research.50813.1
7. Smith DH, Raslan S, Samuels MA, Iglesias T, Buitron I, Deo S, et al. Current salivary biomarkers for detection of human papilloma virus-induced oropharyngeal squamous cell carcinoma. Head Neck. (2021) 43:3618–30. doi: 10.1002/hed.26830
8. Goldenberg D. Anatomy and physiology of the upper aerodigestive tract. In: T Verlagsgruppe , editor. Handbook of Otolaryngology. Stuttgart: Thieme Verlagsgruppe (2011).
9. Lillehoj EP, Kim KC. Airway mucus: its components and function. Arch Pharmacal Res. (2002) 25:770. doi: 10.1007/BF02976990
10. Tiwari M. Science behind human saliva. J Nat Sci Biol Med. (2011) 2:53–8. doi: 10.4103/0976-9668.82322
11. Barona-Lleo L, Barona-De Guzman R, Krstulovic C. The diagnostic usefullness of the salivary pepsin test in symptomatic laryngopharyngeal reflux. J Voice. (2019) 33:923–8. doi: 10.1016/j.jvoice.2018.07.008
12. Smith AD, Cowan JO, Filsell S, McLachlan C, Monti-Sheehan G, Jackson P, et al. Diagnosing asthma: comparisons between exhaled nitric oxide measurements and conventional tests. Am J Respir Crit Care Med. (2004) 169:473–8. doi: 10.1164/rccm.200310-1376OC
13. Fortuna AM, Feixas T, Gonzalez M, Casan P. Diagnostic utility of inflammatory biomarkers in asthma: exhaled nitric oxide and induced sputum eosinophil count. Respir Med. (2007) 101:2416–21. doi: 10.1016/j.rmed.2007.05.019
14. Ahmad Al Obaidi AH, Mohamed Al Samarai AG, Yahya Al Samarai AK, Al Janabi JM. The predictive value of IgE as biomarker in asthma. J Asthma. (2008) 45:654–63. doi: 10.1080/02770900802126958
15. Tsilogianni Z, Hillas G, Bakakos P, Aggelakis L, Konstantellou E, Papaioannou AI, et al. Sputum interleukin-13 as a biomarker for the evaluation of asthma control. Clin Exp Allergy. (2016) 46:923–31. doi: 10.1111/cea.12729
16. Zuo H, Xie X, Peng J, Wang L, Zhu R. Predictive value of novel inflammation-based biomarkers for pulmonary hypertension in the acute exacerbation of chronic obstructive pulmonary disease. Anal Cell Pathol. (2019) 2019:5189165. doi: 10.1155/2019/5189165
17. Drucker E, Krapfenbauer K. Pitfalls and limitations in translation from biomarker discovery to clinical utility in predictive and personalised medicine. EPMA J. (2013) 4:7. doi: 10.1186/1878-5085-4-7
18. Moher D, Liberati A, Tetzlaff J, Altman DG, Group P. Preferred reporting items for systematic reviews and meta-analyses: the PRISMA statement. J Clin Epidemiol. (2009) 62:1006–12.
19. Global Initiative for Chronic Obstructive Lung Disease. Global Strategy for the Diagnosis, Management, and Prevention of Chronic Obstructive Pulmonary Disease (2021 Report). (2021).
20. Qureshi H, Sharafkhaneh A, Hanania NA. Chronic obstructive pulmonary disease exacerbations: latest evidence and clinical implications. Ther Adv Chron Dis. (2014) 5:212–27. doi: 10.1177/2040622314532862
21. Tilemann L, Gindner L, Meyer F, Szecsenyi J, Schneider A. Differences in local and systemic inflammatory markers in patients with obstructive airways disease. Prim Care Respir J. (2011) 20:407–14. doi: 10.4104/pcrj.2011.00069
22. Miravitlles M, Soler-Cataluña JJ, Calle M, Soriano JB. Treatment of COPD by clinical phenotypes: putting old evidence into clinical practice. Eur Respir J. (2013) 41:1252. doi: 10.1183/09031936.00118912
23. Fragoso E, André S, Boleo-Tomé JP, Areias V, Munhá J, Cardoso J. Understanding COPD: a vision on phenotypes, comorbidities and treatment approach. Rev Portuguesa Pneumol. (2016) 22:101–11. doi: 10.1016/j.rppnen.2015.12.001
24. Corlateanu A, Mendez Y, Wang Y, Garnica RDJA, Botnaru V, Siafakas N. Chronic obstructive pulmonary disease and phenotypes: a state-of-the-art. Pulmonology. (2020) 26:95–100. doi: 10.1016/j.pulmoe.2019.10.006
25. Antus B, Paska C, Barta I. Predictive value of exhaled nitric oxide and blood eosinophil count in the assessment of airway eosinophilia in COPD. Int J Chron Obstruct Pulmon Dis. (2020) 15:2025–35. doi: 10.2147/COPD.S257965
26. Takayama Y, Ohnishi H, Ogasawara F, Oyama K, Kubota T, Yokoyama A. Clinical utility of fractional exhaled nitric oxide and blood eosinophils counts in the diagnosis of asthma-COPD overlap. Int J Chron Obstruct Pulmon Dis. (2018) 13:2525–32. doi: 10.2147/COPD.S167600
27. Li M, Yang T, He R, Li A, Dang W, Liu X, et al. The value of inflammatory biomarkers in differentiating asthma-COPD overlap from COPD. Int J Chron Obstruct Pulmon Dis. (2020) 15:3025–37. doi: 10.2147/COPD.S273422
28. Milkowska-Dymanowska J, Bialas AJ, Szewczyk K, Kurmanowska Z, Gorski P, Piotrowski WJ. The usefulness of soluble receptor for advanced glycation end-products in the identification of COPD frequent exacerbator phenotype. Int J Chron Obstruct Pulmon Dis. (2018) 13:3879–84. doi: 10.2147/COPD.S186170
29. Suissa S, Dell’Aniello S, Ernst P. Long-term natural history of chronic obstructive pulmonary disease: severe exacerbations and mortality. Thorax. (2012) 67:957–63. doi: 10.1136/thoraxjnl-2011-201518
30. Mittmann N, Kuramoto L, Seung SJ, Haddon JM, Bradley-Kennedy C, FitzGerald JM. The cost of moderate and severe COPD exacerbations to the Canadian healthcare system. Respir Med. (2008) 102:413–21. doi: 10.1016/j.rmed.2007.10.010
31. Abudagga A, Sun SX, Tan H, Solem CT. Exacerbations among chronic bronchitis patients treated with maintenance medications from a US managed care population: an administrative claims data analysis. Int J Chron Obstruct Pulmon Dis. (2013) 8:175–85. doi: 10.2147/COPD.S40437
32. Taylan M, Demir M, Kaya H, Selimoglu Sen H, Abakay O, Carkanat AI, et al. Alterations of the neutrophil-lymphocyte ratio during the period of stable and acute exacerbation of chronic obstructive pulmonary disease patients. Clin Respir J. (2017) 11:311–7. doi: 10.1111/crj.12336
33. Agapakis DI, Massa EV, Hantzis I, Maraslis S, Alexiou E, Imprialos KP, et al. The role of mean platelet volume in chronic obstructive pulmonary disease exacerbation. Respir Care. (2016) 61:44–9. doi: 10.4187/respcare.04132
34. Andrijevic I, Milutinov S, Lozanov Crvenkovic Z, Matijasevic J, Andrijevic A, Kovacevic T, et al. N-terminal prohormone of brain natriuretic peptide (NT-proBNP) as a diagnostic biomarker of left ventricular systolic dysfunction in patients with acute exacerbation of chronic obstructive pulmonary disease (AECOPD). Lung. (2018) 196:583–90. doi: 10.1007/s00408-018-0137-3
35. Yilmaz MB, Zorlu A, Dogan OT, Karahan O, Tandogan I, Akkurt I. Role of CA-125 in identification of right ventricular failure in chronic obstructive pulmonary disease. Clin Cardiol. (2011) 34:244–8. doi: 10.1002/clc.20868
36. Titova E, Christensen A, Henriksen AH, Steinshamn S, Asberg A. Comparison of procalcitonin, C-reactive protein, white blood cell count and clinical status in diagnosing pneumonia in patients hospitalized with acute exacerbations of COPD: a prospective observational study. Chron Respir Dis. (2019) 16:1479972318769762. doi: 10.1177/1479972318769762
37. Mouronte-Roibas C, Leiro-Fernandez V, Ruano-Ravina A, Ramos-Hernandez C, Casado-Rey P, Botana-Rial M, et al. Predictive value of a series of inflammatory markers in COPD for lung cancer diagnosis: a case-control study. Respir Res. (2019) 20:198. doi: 10.1186/s12931-019-1155-2
38. Wang J, Shang H, Yang X, Guo S, Cui Z. Procalcitonin, C-reactive protein, PaCO2, and noninvasive mechanical ventilation failure in chronic obstructive pulmonary disease exacerbation. Medicine. (2019) 98:e15171. doi: 10.1097/MD.0000000000015171
39. Yao C, Liu X, Tang Z. Prognostic role of neutrophil-lymphocyte ratio and platelet-lymphocyte ratio for hospital mortality in patients with AECOPD. Int J Chron Obstruct Pulmon Dis. (2017) 12:2285–90. doi: 10.2147/COPD.S141760
40. Ergan B, Sahin AA, Topeli A. Serum procalcitonin as a biomarker for the prediction of bacterial exacerbation and mortality in severe COPD exacerbations requiring mechanical ventilation. Respir Int Rev Thorac Dis. (2016) 91:316–24. doi: 10.1159/000445440
41. Sato K, Inoue S, Ishibashi Y, Ota T, Murano H, Furuyama K, et al. Association between low mean corpuscular hemoglobin and prognosis in patients with exacerbation of chronic obstructive pulmonary disease. Respir Investig. (2021) 59:498–504. doi: 10.1016/j.resinv.2021.01.006
42. Yamaji Y, Oishi K, Hamada K, Ohteru Y, Chikumoto A, Murakawa K, et al. Detection of type2 biomarkers for response in COPD. J Breath Res. (2020) 14:026007. doi: 10.1088/1752-7163/ab71a4
43. Stockley RA, Halpin DMG, Celli BR, Singh D. Chronic obstructive pulmonary disease biomarkers and their interpretation. Am J Respir Crit Care Med. (2019) 199:1195–204. doi: 10.1164/rccm.201810-1860SO
44. Keene JD, Jacobson S, Kechris K, Kinney GL, Foreman MG, Doerschuk CM, et al. Biomarkers predictive of exacerbations in the SPIROMICS and COPDGene cohorts. Am J Respir Crit Care Med. (2017) 195:473–81. doi: 10.1164/rccm.201607-1330OC
45. Akiki Z, Fakih D, Jounblat R, Chamat S, Waked M, Holmskov U, et al. Surfactant protein D, a clinical biomarker for chronic obstructive pulmonary disease with excellent discriminant values. Exp Therap Med. (2016) 11:723–30. doi: 10.3892/etm.2016.2986
46. Rennard SI, Merrill D, Tal-Singer R, Sciurba FC. The St. George’s respiratory questionnaire appendix to the food and drug administration draft guidance on COPD: why a small step forward is so important. Chest. (2017) 152:914–6. doi: 10.1016/j.chest.2017.07.040
47. GINA. Global Strategy for Asthma Management and Prevention (2020 Update). Walworth, WI: GINA (2020).
48. Yormaz B, Menevse E, Cetin N, Esin Celik Z, Bakir H, Tulek B, et al. Diagnostic value of thymus and activation-regulated chemokine and of periostin in eosinophilic asthma: a prospective study. Allergy Asthma Proc. (2021) 42:e30–9. doi: 10.2500/aap.2021.42.200102
49. Vandenplas O, Froidure A, Meurer U, Rihs HP, Rifflart C, Soetaert S, et al. The role of allergen components for the diagnosis of latex-induced occupational asthma. Allergy. (2016) 71:840–9. doi: 10.1111/all.12872
50. Kim SH, Choi GS, Nam YH, Kim JH, Hur GY, Kim SH, et al. Role of vitamin D-binding protein in isocyanate-induced occupational asthma. Exp Mol Med. (2012) 44:319–29. doi: 10.3858/emm.2012.44.5.036
51. Racine G, Castano R, Cartier A, Lemiere C. Diagnostic accuracy of inflammatory markers for diagnosing occupational asthma. J Allergy Clin Immunol Pract. (2017) 5:1371–7.e1. doi: 10.1016/j.jaip.2017.02.001
52. Vandenplas O, D’Alpaos V, Heymans J, Jamart J, Thimpont J, Huaux F, et al. Sputum eosinophilia: an early marker of bronchial response to occupational agents. Allergy. (2009) 64:754–61. doi: 10.1111/j.1398-9995.2008.01896.x
53. Kuruvilla ME, Lee FE-H, Lee GB. Understanding asthma phenotypes, endotypes, and mechanisms of disease. Clin Rev Allergy Immunol. (2019) 56:219–33. doi: 10.1007/s12016-018-8712-1
54. Roth M, Stolz D. Biomarkers and personalised medicine for asthma. Eur Respir J. (2019) 53:1802094. doi: 10.1183/13993003.02094-2018
55. Sadeghi MH, Morice AH. The emerging role of the eosinophil and its measurement in chronic cough. Open Respir Med J. (2017) 11:17–30. doi: 10.2174/1874306401711010017
56. Guiot J, Demarche S, Henket M, Paulus V, Graff S, Schleich F, et al. Methodology for sputum induction and laboratory processing. J Vis Exp. (2017) 130:56612. doi: 10.3791/56612
57. Soma T, Iemura H, Naito E, Miyauchi S, Uchida Y, Nakagome K, et al. Implication of fraction of exhaled nitric oxide and blood eosinophil count in severe asthma. Allergol Int. (2018) 67S:S3–11. doi: 10.1016/j.alit.2018.04.003
58. Wagener AH, de Nijs SB, Lutter R, Sousa AR, Weersink EJM, Bel EH, et al. External validation of blood eosinophils, FE(NO) and serum periostin as surrogates for sputum eosinophils in asthma. Thorax. (2015) 70:115–20. doi: 10.1136/thoraxjnl-2014-205634
59. Liang Z, Zhao H, Lv Y, Li R, Dong H, Liu L, et al. Moderate accuracy of peripheral eosinophil count for predicting eosinophilic phenotype in steroid-naïve non-atopic adult asthmatics. Intern Med. (2012) 51:717–22. doi: 10.2169/internalmedicine.51.6834
60. Liu T, Wu J, Zhao J, Wang J, Zhang Y, Liu L, et al. Type 2 innate lymphoid cells: a novel biomarker of eosinophilic airway inflammation in patients with mild to moderate asthma. Respir Med. (2015) 109:1391–6. doi: 10.1016/j.rmed.2015.09.016
61. Suzuki Y, Wakahara K, Nishio T, Ito S, Hasegawa Y. Airway basophils are increased and activated in eosinophilic asthma. Allergy. (2017) 72:1532–9. doi: 10.1111/all.13197
62. Zhang XY, Simpson JL, Powell H, Yang IA, Upham JW, Reynolds PN, et al. Full blood count parameters for the detection of asthma inflammatory phenotypes. Clin Exp Allergy. (2014) 44:1137–45. doi: 10.1111/cea.12345
63. Coumou H, Westerhof GA, de Nijs SB, Amelink M, Bel EH. Diagnosing persistent blood eosinophilia in asthma with single blood eosinophil or exhaled nitric oxide level. Respir Med. (2018) 141:81–6. doi: 10.1016/j.rmed.2018.06.025
64. Westerhof GA, Korevaar DA, Amelink M, de Nijs SB, de Groot JC, Wang J, et al. Biomarkers to identify sputum eosinophilia in different adult asthma phenotypes. Eur Respir J. (2015) 46:688–96. doi: 10.1183/09031936.00012415
65. Jia G, Erickson RW, Choy DF, Mosesova S, Wu LC, Solberg OD, et al. Periostin is a systemic biomarker of eosinophilic airway inflammation in asthmatic patients. J Allergy Clin Immunol. (2012) 130:647–54.e10. doi: 10.1016/j.jaci.2012.06.025
66. Hilvering B, Vijverberg SJH, Jansen J, Houben L, Schweizer RC, Go S, et al. Diagnosing eosinophilic asthma using a multivariate prediction model based on blood granulocyte responsiveness. Allergy. (2017) 72:1202–11. doi: 10.1111/all.13117
67. Chang HS, Shin SW, Lee TH, Bae DJ, Park JS, Kim YH, et al. Development of a genetic marker set to diagnose aspirin-exacerbated respiratory disease in a genome-wide association study. Pharmacogenom J. (2015) 15:316–21. doi: 10.1038/tpj.2014.78
68. Shin S-W, Park BL, Chang H, Park JS, Bae D-J, Song H-J, et al. Exonic variants associated with development of aspirin exacerbated respiratory diseases. PLoS One. (2014) 9:e111887. doi: 10.1371/journal.pone.0111887
69. Shabana MA, Esawy MM, Ismail NA, Said AM. Predictive role of IL-17A/IL-10 ratio in persistent asthmatic patients on vitamin D supplement. Immunobiology. (2019) 224:721–7. doi: 10.1016/j.imbio.2019.09.005
70. Berthon BS, Gibson PG, Wood LG, MacDonald-Wicks LK, Baines KJ. A sputum gene expression signature predicts oral corticosteroid response in asthma. Eur Respir J. (2017) 49:1700180. doi: 10.1183/13993003.00180-2017
71. Lee Y, Quoc QL, Park HS. Biomarkers for severe asthma: lessons from longitudinal cohort studies. Allergy Asthma Immunol Res. (2021) 13:375–89. doi: 10.4168/aair.2021.13.3.375
72. Vijverberg SJ, Hilvering B, Raaijmakers JA, Lammers J-WJ, Maitland-van der Zee A-H, Koenderman L. Clinical utility of asthma biomarkers: from bench to bedside. Biol Targets Ther. (2013) 7:199–210. doi: 10.2147/BTT.S29976
73. Groome M, Cotton JP, Borland M, McLeod S, Johnston DA, Dillon JF. Prevalence of laryngopharyngeal reflux in a population with gastroesophageal reflux. Laryngoscope. (2007) 117:1424–8. doi: 10.1097/MLG.0b013e31806865cf
74. Asaoka D, Nagahara A, Matsumoto K, Hojo M, Watanabe S. Current perspectives on reflux laryngitis. Clin J Gastroenterol. (2014) 7:471–5. doi: 10.1007/s12328-014-0535-x
75. Campagnolo AM, Priston J, Thoen RH, Medeiros T, Assunção AR. Laryngopharyngeal reflux: diagnosis, treatment, and latest research. Int Arch Otorhinolaryngol. (2014) 18:184–91. doi: 10.1055/s-0033-1352504
76. Vaezi MF, Hicks DM, Abelson TI, Richter JE. Laryngeal signs and symptoms and gastroesophageal reflux disease (GERD): a critical assessment of cause and effect association. Clin Gastroenterol Hepatol. (2003) 1:333–44. doi: 10.1053/s1542-3565(03)00177-0
77. Yılmaz T, Bajin MD, Günaydın RÖ, Ozer S, Sözen T. Laryngopharyngeal reflux and Helicobacter pylori. World J Gastroenterol. (2014) 20:8964–70.
78. Printza A, Speletas M, Triaridis S, Wilson J. Is pepsin detected in the saliva of patients who experience pharyngeal reflux? Hippokratia. (2007) 11:145–9.
79. Adhami T, Goldblum JR, Richter JE, Vaezi MF. The role of gastric and duodenal agents in laryngeal injury: an experimental canine model. Am J Gastroenterol. (2004) 99:2098–106. doi: 10.1111/j.1572-0241.2004.40170.x
80. Ozmen S, Demirceken F, Barut Y, Dibek Misirlioglu E. Role of laryngoscopy in children with respiratory complaints and suspected reflux. Allergol Immunopathol. (2012) 40:204–9. doi: 10.1016/j.aller.2011.06.006
81. Branski RC, Bhattacharyya N, Shapiro J. The reliability of the assessment of endoscopic laryngeal findings associated with laryngopharyngeal reflux disease. Laryngoscope. (2002) 112:1019–24. doi: 10.1097/00005537-200206000-00016
82. Belafsky PC, Postma GN, Koufman JA. The validity and reliability of the reflux finding score (RFS). Laryngoscope. (2001) 111:1313–7. doi: 10.1097/00005537-200108000-00001
83. Park KH, Choi SM, Kwon SU, Yoon SW, Kim SU. Diagnosis of laryngopharyngeal reflux among globus patients. Otolaryngol Head Neck Surg. (2006) 134:81–5. doi: 10.1016/j.otohns.2005.08.025
84. Vázquez de la Iglesia F, Fernández González S, de la Cámara Gómez M. Laryngopharyngeal reflux: correlation between symptoms and signs by means of clinical assessment questionnaires and fibroendoscopy. Is this sufficient for diagnosis? Acta Otorrinolaringol. (2007) 58:421–5. doi: 10.1016/s2173-5735(07)70381-2
85. Mallikarjunappa AM, Deshpande GA. Comparison of reflux symptom index (RSI) with reflux finding score (RFS) and its effectiveness in diagnosis of laryngopharyngeal reflux disease (LPRD). Indian J Otolaryngol Head Neck Surg. (2020) 1–5.
86. Weitzendorfer M, Antoniou SA, Schredl P, Witzel K, Weitzendorfer IC, Majerus A, et al. Pepsin and oropharyngeal pH monitoring to diagnose patients with laryngopharyngeal reflux. Laryngoscope. (2020) 130:1780–6. doi: 10.1002/lary.28320
87. Lechien JR, Schindler A, Hamdan AL, Bobin F, Barillari MR, Harmegnies B, et al. The development of new clinical instruments in laryngopharyngeal reflux disease: the international project of young otolaryngologists of the international federation of Oto-rhino-laryngological societies. Eur Ann Otorhinolaryngol Head Neck Dis. (2018) 135(5 Suppl.):S85–91. doi: 10.1016/j.anorl.2018.05.013
89. Böger B, Fachi MM, Vilhena RO, Cobre AF, Tonin FS, Pontarolo R. Systematic review with meta-analysis of the accuracy of diagnostic tests for COVID-19. Am J Infect Control. (2021) 49:21–9.
90. SARS-CoV-2 Diagnostic Pipeline. Foundation for Innovative New Diagnostics (FIND). (2022). Available online at: https://www.finddx.org/test-directory/ (accessed January 10, 2022).
91. Comins-Boo A, Gutierrez-Larranaga M, Roa-Bautista A, Guiral Foz S, Renuncio Garcia M, Gonzalez Lopez E, et al. Validation of a quick flow cytometry-based assay for acute infection based on CD64 and CD169 expression. new tools for early diagnosis in COVID-19 pandemic. Front Med. (2021) 8:655785. doi: 10.3389/fmed.2021.655785
92. Outh R, Boutin C, Gueudet P, Suzuki M, Saada M, Aumaitre H. Eosinopenia <100/muL as a marker of active COVID-19: An observational prospective study. J Microbiol Immunol Infect. (2021) 54:61–8. doi: 10.1016/j.jmii.2020.12.005
93. Li Q, Ding X, Xia G, Chen H-G, Chen F, Geng Z, et al. Eosinopenia and elevated C-reactive protein facilitate triage of COVID-19 patients in fever clinic: a retrospective case-control study. EClinicalMedicine. (2020) 23:100375. doi: 10.1016/j.eclinm.2020.100375
94. Samprathi M, Jayashree M. Biomarkers in COVID-19: an up-to-date review. Front Pediatr. (2021) 8:607647. doi: 10.3389/fped.2020.607647
95. Chang R, Elhusseiny KM, Yeh Y-C, Sun W-Z. COVID-19 ICU and mechanical ventilation patient characteristics and outcomes—a systematic review and meta-analysis. PLoS One. (2021) 16:e0246318. doi: 10.1371/journal.pone.0246318
96. Ahnach M, Nejjari S, Zbiri S, Ousti F, Elkettani C. C-reactive protein as an early predictor of COVID-19 severity. J Med Biochem. (2020) 39:500–7. doi: 10.5937/jomb0-27554
97. Bi X, Su Z, Yan H, Du J, Wang J, Chen L, et al. Prediction of severe illness due to COVID-19 based on an analysis of initial fibrinogen to albumin ratio and platelet count. Platelets. (2020) 31:674–9. doi: 10.1080/09537104.2020.1760230
98. Ding R, Yang Z, Huang D, Wang Y, Li X, Zhou X, et al. Identification of parameters in routine blood and coagulation tests related to the severity of COVID-19. Int J Med Sci. (2021) 18:1207–15. doi: 10.7150/ijms.47494
99. Tan C, Huang Y, Shi F, Tan K, Ma Q, Chen Y, et al. C-reactive protein correlates with computed tomographic findings and predicts severe COVID-19 early. J Med Virol. (2020) 92:856–62. doi: 10.1002/jmv.25871
100. Tang Y, Li Y, Sun J, Pan H, Yao F, Jiao X. Selection of an optimal combination panel to better triage COVID-19 hospitalized patients. J Inflamm Res. (2020) 13:773–87. doi: 10.2147/JIR.S273193
101. Rasyid H, Sangkereng A, Harjianti T, Soetjipto AS. Impact of age to ferritin and neutrophil-lymphocyte ratio as biomarkers for intensive care requirement and mortality risk in COVID-19 patients in Makassar, Indonesia. Physiol Rep. (2021) 9:e14876. doi: 10.14814/phy2.14876
102. Chen X, Wang Q, Xu M, Li C. A retrospective analysis of the coagulation dysfunction in COVID-19 patients. Clin Appl Thromb Hemostas. (2020) 26:1076029620964868. doi: 10.1177/1076029620964868
103. Doğan HO, Bolat S, Büyüktuna SA, Sarıismailoğlu R, Çetinkaya N, Doğan K, et al. The use of laboratory parameters and computed tomography score to determine intensive care unit requirement in COVID-19. Turk J Biochem. (2021) 46:157–66. doi: 10.1515/tjb-2020-0359
104. Luo X, Zhou W, Yan X, Guo T, Wang B, Xia H, et al. Prognostic value of C-reactive protein in patients with coronavirus 2019. Clin Infect Dis. (2020) 71:2174–9. doi: 10.1093/cid/ciaa641
105. Yang C, Ma X, Wu J, Han J, Zheng Z, Duan H, et al. Low serum calcium and phosphorus and their clinical performance in detecting COVID-19 patients. J Med Virol. (2021) 93:1639–51. doi: 10.1002/jmv.26515
106. Laguna-Goya R, Utrero-Rico A, Talayero P, Lasa-Lazaro M, Ramirez-Fernandez A, Naranjo L, et al. IL-6-based mortality risk model for hospitalized patients with COVID-19. J Allergy Clin Immunol. (2020) 146:799–807.e9. doi: 10.1016/j.jaci.2020.07.009
107. Sharifpour M, Rangaraju S, Liu M, Alabyad D, Nahab FB, Creel-Bulos CM, et al. C-reactive protein as a prognostic indicator in hospitalized patients with COVID-19. PLoS One. (2020) 15:e0242400. doi: 10.1371/journal.pone.0242400
108. Qin J-J, Cheng X, Zhou F, Lei F, Akolkar G, Cai J, et al. Redefining cardiac biomarkers in predicting mortality of inpatients with COVID-19. Hypertension. (2020) 76:1104–12. doi: 10.1161/HYPERTENSIONAHA.120.15528
109. Albarran-Sanchez A, Gonzalez-Rios RD, Alberti-Minutti P, Noyola-Garcia ME, Contreras-Garcia CE, Anda-Garay JC, et al. Association of neutrophil-to-lymphocyte and lymphocyte-to-C-reactive protein ratios with COVID-19-related mortality. Gac Med Mex. (2020) 156:553–8. doi: 10.24875/GMM.M21000481
110. Gregoriano C, Koch D, Kutz A, Haubitz S, Conen A, Bernasconi L, et al. The vasoactive peptide MR-pro-adrenomedullin in COVID-19 patients: an observational study. Clin Chem Lab Med. (2021) 59:995–1004. doi: 10.1515/cclm-2020-1295
111. Luo Y, Mao L, Yuan X, Xue Y, Lin Q, Tang G, et al. Prediction model based on the combination of cytokines and lymphocyte subsets for prognosis of SARS-CoV-2 infection. J Clin Immunol. (2020) 40:960–9. doi: 10.1007/s10875-020-00821-7
112. Wang J, Zhang H, Qiao R, Ge Q, Zhang S, Zhao Z, et al. Thrombo-inflammatory features predicting mortality in patients with COVID-19: The FAD-85 score. J Int Med Res. (2020) 48:300060520955037. doi: 10.1177/0300060520955037
113. Booth AL, Abels E, McCaffrey P. Development of a prognostic model for mortality in COVID-19 infection using machine learning. Modern Pathol. (2021) 34:522–31. doi: 10.1038/s41379-020-00700-x
114. De Michieli L, Babuin L, Vigolo S, Berti De Marinis G, Lunardon A, Favretto F, et al. Using high sensitivity cardiac troponin values in patients with SARS-CoV-2 infection (COVID-19): the Padova experience. Clin Biochem. (2021) 90:8–14. doi: 10.1016/j.clinbiochem.2021.01.006
115. Feld J, Tremblay D, Thibaud S, Kessler A, Naymagon L. Ferritin levels in patients with COVID-19: a poor predictor of mortality and hemophagocytic lymphohistiocytosis. Int J Lab Hematol. (2020) 42:773–9. doi: 10.1111/ijlh.13309
116. Ozyilmaz S, Ergun Alis E, Ermis E, Allahverdiyev S, Ucar H. Assessment of the relationship between mortality and troponin I levels in hospitalized patients with the novel coronavirus (COVID-19). Medicina. (2020) 56:693. doi: 10.3390/medicina56120693
117. Chen F-F, Zhong M, Liu Y, Zhang Y, Zhang K, Su D-Z, et al. The characteristics and outcomes of 681 severe cases with COVID-19 in China. J Crit Care. (2020) 60:32–7. doi: 10.1016/j.jcrc.2020.07.003
118. Voicu S, Delrue M, Chousterman BG, Stepanian A, Bonnin P, Malissin I, et al. Imbalance between procoagulant factors and natural coagulation inhibitors contributes to hypercoagulability in the critically ill COVID-19 patient: clinical implications. Eur Rev Med Pharmacol Sci. (2020) 24:9161–8. doi: 10.26355/eurrev_202009_22866
119. Demelo-Rodriguez P, Cervilla-Munoz E, Ordieres-Ortega L, Parra-Virto A, Toledano-Macias M, Toledo-Samaniego N, et al. Incidence of asymptomatic deep vein thrombosis in patients with COVID-19 pneumonia and elevated D-dimer levels. Thrombos Res. (2020) 192:23–6. doi: 10.1016/j.thromres.2020.05.018
120. Creel-Bulos C, Liu M, Auld SC, Gaddh M, Kempton CL, Sharifpour M, et al. Trends and diagnostic value of D-dimer levels in patients hospitalized with coronavirus disease 2019. Medicine. (2020) 99:e23186. doi: 10.1097/MD.0000000000023186
121. Dujardin RWG, Hilderink BN, Haksteen WE, Middeldorp S, Vlaar APJ, Thachil J, et al. Biomarkers for the prediction of venous thromboembolism in critically ill COVID-19 patients. Thromb Res. (2020) 196:308–12. doi: 10.1016/j.thromres.2020.09.017
122. FNIH.Biomarkers Consortium. (2021). Available online at: https://fnih.org/our-programs/biomarkers-consortium (accessed April 27, 2022).
123. FDA.Biomarker Qualification Program: U.S. Food and Drug Administration. (2021). Available online at: https://www.fda.gov/drugs/drug-development-tool-ddt-qualification-programs/biomarker-qualification-program (accessed April 27, 2022).
124. NINDS.Focus On Biomarkers Research. (2022). Available online at: https://www.ninds.nih.gov/Current-Research/Focus-Tools-Topics/Biomarkers (accessed April 27, 2022).
125. Mattsson-Carlgren N, Palmqvist S, Blennow K, Hansson O. Increasing the reproducibility of fluid biomarker studies in neurodegenerative studies. Nat Commun. (2020) 11:6252.
126. Lavezzari G, Womack AW. Industry perspectives on biomarker qualification. Clin Pharmacol Ther. (2016) 99:208–13. doi: 10.1002/cpt.264
127. Davis KD, Aghaeepour N, Ahn AH, Angst MS, Borsook D, Brenton A, et al. Discovery and validation of biomarkers to aid the development of safe and effective pain therapeutics: challenges and opportunities. Nat Rev Neurol. (2020) 16:381–400. doi: 10.1038/s41582-020-0362-2
128. Amur SG, Sanyal S, Chakravarty AG, Noone MH, Kaiser J, McCune S, et al. Building a roadmap to biomarker qualification: challenges and opportunities. Biomark Med. (2015) 9:1095–105. doi: 10.2217/bmm.15.90
130. Johns Hopkins Center for Health Security.Serology Testing for COVID-19. Baltimore, MD: Johns Hopkins Center for Health Security (2021).
131. Carter LJ, Garner LV, Smoot JW, Li Y, Zhou Q, Saveson CJ, et al. Assay techniques and test development for COVID-19 diagnosis. ACS Cent Sci. (2020) 6:591–605. doi: 10.1021/acscentsci.0c00501
132. Ocak E, Kubat G, Yorulmaz İ. Immunoserologic pepsin detection in the saliva as a non-invasive rapid diagnostic test for laryngopharyngeal reflux. Balkan Med J. (2015) 32:46–50. doi: 10.5152/balkanmedj.2015.15824
133. Knight J, Lively MO, Johnston N, Dettmar PW, Koufman JA. Sensitive pepsin immunoassay for detection of laryngopharyngeal reflux. Laryngoscope. (2005) 115:1473–8. doi: 10.1097/01.mlg.0000172043.51871.d9
134. Li J, Macdonald J. Multiplexed lateral flow biosensors: technological advances for radically improving point-of-care diagnoses. Biosens Bioelectron. (2016) 83:177–92. doi: 10.1016/j.bios.2016.04.021
135. Hu J, Choi JR, Wang S, Gong Y, Feng S, Pingguan-Murphy B, et al. Multiple test zones for improved detection performance in lateral flow assays. Sens Actuat B Chem. (2017) 243:484–8. doi: 10.1016/j.snb.2016.12.008
136. Yew CHT, Azari P, Choi JR, Li F, Pingguan-Murphy B. Electrospin-coating of nitrocellulose membrane enhances sensitivity in nucleic acid-based lateral flow assay. Anal Chim Acta. (2018) 1009:81–8. doi: 10.1016/j.aca.2018.01.016
138. Mahto SK. Revisiting pulmonary diseases using microfluidic technology. Int J Biosens Bioelectron. (2016) 1:1–5.
139. Iliescu FS, Poenar DP, Yu F, Ni M, Chan KH, Cima I, et al. Recent advances in microfluidic methods in cancer liquid biopsy. Biomicrofluidics. (2019) 13:1–44. doi: 10.1063/1.5087690
140. Ruzycka M, Cimpan MR, Rios-Mondragon I, Grudzinski IP. Microfluidics for studying metastatic patterns of lung cancer. J Nanobiotechnol. (2019) 17:1–30. doi: 10.1186/s12951-019-0492-0
141. Hung LY, Wu HW, Hsieh K, Lee GB. Microfluidic platforms for discovery and detection of molecular biomarkers. Microfluid Nanofluid. (2014) 16:941–63. doi: 10.1007/s10404-014-1354-6
142. Lu J, Pang J, Chen Y, Dong Q, Sheng J, Luo Y, et al. Application of microfluidic chips in separation and analysis of extracellular vesicles in liquid biopsy for cancer. Micromachines. (2019) 10:390. doi: 10.3390/mi10060390
143. Perkel JM. Microfluidics, macro-impacts. Biotechniques. (2012) 52:131–4. doi: 10.2144/000113816
144. Silva MLS. Microfluidic devices for glycobiomarker detection in cancer. Clin Chim Acta. (2021) 521:229–43. doi: 10.1016/j.cca.2021.07.013
145. Jiang K, Jokhun DS, Lim CT. Microfluidic detection of human diseases: from liquid biopsy to COVID-19 diagnosis. J Biomech. (2021) 117:110235. doi: 10.1016/j.jbiomech.2021.110235
146. Whitesides GM. The origins and the future of microfluidics. Nature. (2006) 442:368–73. doi: 10.1038/nature05058
147. Chen H, Chen C, Bai S, Gao Y, Metcalfe G, Cheng W, et al. Multiplexed detection of cancer biomarkers using a microfluidic platform integrating single bead trapping and acoustic mixing techniques. Nanoscale. (2018) 10:20196–206. doi: 10.1039/c8nr06367b
148. Li N, Shen M, Xu Y. A portable microfluidic system for point-of-care detection of multiple protein biomarkers. Micromachines. (2021) 12:347. doi: 10.3390/mi12040347
149. Martinez AW, Phillips ST, Whitesides GM, Carrilho E. Diagnostics for the developing world: microfluidic paper-based analytical devices. Anal Chem. (2010) 82:3–10. doi: 10.1021/ac9013989
150. Yetisen AK, Akram MS, Lowe CR. Paper-based microfluidic point-of-care diagnostic devices. Lab Chip. (2013) 13:2210–51. doi: 10.1039/c3lc50169h
151. Mitchell KR, Esene JE, Woolley AT. Advances in multiplex electrical and optical detection of biomarkers using microfluidic devices. Anal Bioanal Chem. (2021) 414: 167–80.
152. Wang L, Dai C, Jiang L, Tong G, Xiong Y, Khan K, et al. Advanced devices for tumor diagnosis and therapy. Small. (2021) 17:1–20. doi: 10.1002/smll.202100003
153. Zilberman Y, Sonkusale SR. Microfluidic optoelectronic sensor for salivary diagnostics of stomach cancer. Biosens Bioelectron. (2015) 67:465–71. doi: 10.1016/j.bios.2014.09.006
154. Chen Z, Mauk MG, Wang J, Abrams WR, Corstjens PLAM, Niedbala RS, et al. A microfluidic system for saliva-based detection of infectious diseases. Ann N Y Acad Sci. (2007) 1098:429–36. doi: 10.1196/annals.1384.024
155. Hou HW, Warkiani ME, Khoo BL, Li ZR, Soo RA, Tan DSW, et al. Isolation and retrieval of circulating tumor cells using centrifugal forces. Sci Rep. (2013) 3:1–8. doi: 10.1038/srep01259
156. Wu J, Hillier C, Komenda P, Lobato De Faria R, Levin D, Zhang M, et al. A microfluidic platform for evaluating neutrophil chemotaxis induced by sputum from COPD patients. PLoS One. (2015) 10:e0126523. doi: 10.1371/journal.pone.0126523
157. Yoshizawa JM, Schafer CA, Schafer JJ, Farrell JJ, Paster BJ, Wong DTW. Salivary biomarkers: toward future clinical and diagnostic utilities. Clin Microbiol Rev. (2013) 26:781–91. doi: 10.1128/CMR.00021-13
158. Verdolini Abbott K, Li NY, Branski RC, Rosen CA, Grillo E, Steinhauer K, et al. Vocal exercise may attenuate acute vocal fold inflammation. J Voice. (2012) 26:814.e1–13. doi: 10.1016/j.jvoice.2012.03.008
159. Sackmann EKH, Berthier E, Schwantes EA, Fichtinger PS, Evans MD, Dziadzio LL, et al. Characterizing asthma from a drop of blood using neutrophil chemotaxis. Proc Natl Acad Sci USA. (2014) 111:5813–8. doi: 10.1073/pnas.1324043111
160. Ganguli A, Mostafa A, Berger J, Aydin MY, Sun F, Stewart de Ramirez SA, et al. Rapid isothermal amplification and portable detection system for SARS-CoV-2. Proc Natl Acad Sci USA. (2020) 117: 22727–35.
161. Jadhav SA, Biji P, Panthalingal MK, Murali Krishna C, Rajkumar S, Joshi DS, et al. Development of integrated microfluidic platform coupled with surface-enhanced Raman spectroscopy for diagnosis of COVID-19. Med Hypothes. (2021) 146:110356. doi: 10.1016/j.mehy.2020.110356
162. Sanjay ST, Fu G, Dou M, Xu F, Liu R, Qi H, et al. Biomarker detection for disease diagnosis using cost-effective microfluidic platforms. Analyst. (2015) 140:7062–81.
163. Mejía-Salazar JR, Cruz KR, Vásques EMM, de Oliveira ON. Microfluidic point-of-care devices: new trends and future prospects for ehealth diagnostics. Sensors. (2020) 20:1–19. doi: 10.3390/s20071951
164. Li H, Steckl AJ. Paper microfluidics for point-of-care blood-based analysis and diagnostics. Anal Chem. (2019) 91:352–71. doi: 10.1021/acs.analchem.8b03636
165. Gong MM, Sinton D. Turning the page: advancing paper-based microfluidics for broad diagnostic application. Chem Rev. (2017) 117:8447–80. doi: 10.1021/acs.chemrev.7b00024
167. Korte BJ, Rompalo A, Manabe YC, Gaydos CA. Overcoming challenges with the adoption of point-of-care testing: from technology push and clinical needs to value propositions. Point Care. (2020) 19:77–83. doi: 10.1097/POC.0000000000000209
168. Olearo F, Nörz D, Heinrich F, Sutter JP, Roedl K, Schultze A, et al. Handling and accuracy of four rapid antigen tests for the diagnosis of SARS-CoV-2 compared to RT-qPCR. J Clin Virol. (2021) 137:104782. doi: 10.1016/j.jcv.2021.104782
169. Mitchell KR, Esene JE, Woolley AT. Advances in multiplex electrical and optical detection of biomarkers using microfluidic devices. Anal Bioanal Chem. (2022) 414:167–80.
170. Sharma Timilsina S, Fu G, Dou M, Xu F, Liu R, Qi H, et al. Biomarker detection for disease diagnosis using cost-effective microfluidic platforms. Analyst. (2015) 140: 7062–81.
171. Chu H, Liu C, Liu J, Yang J, Li Y, Zhang X. Recent advances and challenges of biosensing in point-of-care molecular diagnosis. Sens Actuators B Chem. (2021) 348:130708. doi: 10.1016/j.snb.2021.130708
172. Lu Y, Shi W, Jiang L, Qin J, Lin B. Rapid prototyping of paper-based microfluidics with wax for low-cost, portable bioassay. Electrophoresis. (2009) 30:1497–500. doi: 10.1002/elps.200800563
173. Li X, Tian J, Garnier G, Shen W. Fabrication of paper-based microfluidic sensors by printing. Colloids Surf B Biointerfaces. (2010) 76:564–70. doi: 10.1016/j.colsurfb.2009.12.023
174. Shephard M, Shephard A, Matthews S, Andrewartha K. The benefits and challenges of point-of-care testing in rural and remote primary care settings in Australia. Arch Pathol Lab Med. (2020) 144:1372–80. doi: 10.5858/arpa.2020-0105-RA
Keywords: biomarkers, airway diseases, point-of-care, diagnostic accuracy, COVID-19
Citation: Landry V, Coburn P, Kost K, Liu X and Li-Jessen NYK (2022) Diagnostic Accuracy of Liquid Biomarkers in Airway Diseases: Toward Point-of-Care Applications. Front. Med. 9:855250. doi: 10.3389/fmed.2022.855250
Received: 14 January 2022; Accepted: 04 May 2022;
Published: 06 June 2022.
Edited by:
Mats W. Johansson, Morgridge Institute for Research, United StatesReviewed by:
Corrado Pelaia, Magna Græcia University, ItalyCopyright © 2022 Landry, Coburn, Kost, Liu and Li-Jessen. This is an open-access article distributed under the terms of the Creative Commons Attribution License (CC BY). The use, distribution or reproduction in other forums is permitted, provided the original author(s) and the copyright owner(s) are credited and that the original publication in this journal is cited, in accordance with accepted academic practice. No use, distribution or reproduction is permitted which does not comply with these terms.
*Correspondence: Nicole Y. K. Li-Jessen, bmljb2xlLmxpQG1jZ2lsbC5jYQ==
Disclaimer: All claims expressed in this article are solely those of the authors and do not necessarily represent those of their affiliated organizations, or those of the publisher, the editors and the reviewers. Any product that may be evaluated in this article or claim that may be made by its manufacturer is not guaranteed or endorsed by the publisher.
Research integrity at Frontiers
Learn more about the work of our research integrity team to safeguard the quality of each article we publish.