- 1Department of Clinical Laboratory Sciences, College of Applied Medical Sciences, King Saud University, Riyadh, Saudi Arabia
- 2Department of Medical and Molecular Virology, Prince Sultan Military Medical City, Riyadh, Saudi Arabia
- 3College of Applied Medical Sciences, Riyadh Elm University, Riyadh, Saudi Arabia
- 4College of Dentistry, Riyadh Elm University, Riyadh, Saudi Arabia
Background: Many COVID-19 patients reveal a marked decrease in their lymphocyte counts, a condition that translates clinically into immunodepression and is common among these patients. Outcomes for infected patients vary depending on their lymphocytopenia status, especially their T-cell counts. Patients are more likely to recover when lymphocytopenia is resolved. When lymphocytopenia persists, severe complications can develop and often lead to death. Similarly, IL-10 concentration is elevated in severe COVID-19 cases and may be associated with the depression observed in T-cell counts. Accordingly, this systematic review and meta-analysis aims to analyze T-cell subsets and IL-10 levels among COVID-19 patients. Understanding the underlying mechanisms of the immunodepression observed in COVID-19, and its consequences, may enable early identification of disease severity and reduction of overall morbidity and mortality.
Methods: A systematic search was conducted covering PubMed MEDLINE, Scopus, Web of Science, and EBSCO databases for journal articles published from December 1, 2019 to March 14, 2021. In addition, we reviewed bibliographies of relevant reviews and the medRxiv preprint server for eligible studies. Our search covered published studies reporting laboratory parameters for T-cell subsets (CD4/CD8) and IL-10 among confirmed COVID-19 patients. Six authors carried out the process of data screening, extraction, and quality assessment independently. The DerSimonian-Laird random-effect model was performed for this meta-analysis, and the standardized mean difference (SMD) and 95% confidence interval (CI) were calculated for each parameter.
Results: A total of 52 studies from 11 countries across 3 continents were included in this study. Compared with mild and survivor COVID-19 cases, severe and non-survivor cases had lower counts of CD4/CD8 T-cells and higher levels of IL-10.
Conclusion: Our findings reveal that the level of CD4/CD8 T-cells and IL-10 are reliable predictors of severity and mortality in COVID-19 patients. The study protocol is registered with the International Prospective Register of Systematic Reviews (PROSPERO); registration number CRD42020218918.
Systematic Review Registration: https://www.crd.york.ac.uk/prospero/display_record.php?ID=CRD42020218918, identifier: CRD42020218918.
Introduction
Coronavirus disease 2019 (COVID-19) is a viral infection caused by the severe acute respiratory syndrome coronavirus 2 (SARS-CoV-2), first identified in December 2019 when patients with an unknown type of pneumonia were admitted to Hubei hospital in Wuhan, China (1, 2). The fear of encountering a novel strain from the notorious coronavirus family, of which SARS-CoV-1 and MERS-CoV are members, was thus realized (3). This novel disease spread rapidly from its country of origin to other regions of the world, affecting people in 192 countries and resulting in 280,001,617 confirmed cases and 5,402,083 deaths (4). COVID-19 was declared a global health emergency and a pandemic by the World Health Organization (WHO) in March 2020 (5).
Infection with SARS-CoV-2 does not immediately cause disease, and patients can be divided into four major classes based on their presentation on the clinical spectrum. Patients in the first class are asymptomatic with no clinically reported signs, although anosmia and dysgeusia are common among this group (6–8). The second develop flu-like symptoms with fever, sore throat, and cough (9, 10). Additional signs and symptoms of varying severity are present in the third group, including frequent chest pain, difficulty breathing, and unproductive cough (10, 11). Finally, in the fourth group, life-threatening complications become evident as the disease progresses, and patients begin to exhibit critical signs and symptoms, including pneumonia, acute lung injury (ALI), acute respiratory distress syndrome (ARDS), septic shock, and multiple organ failure (12, 13). Furthermore, the incubation period from infection to the onset of disease varies greatly, ranging from 2 to 14 days (14, 15). The virus's basic reproduction number (R0) is estimated to be around 1.4 and 3.8, indicating the potential for a pandemic and recurrent infection within populations (1, 16–18).
It is common for patients with COVID-19 to show a marked decrease in their leukocyte counts, specifically their lymphocyte counts, a condition that translates clinically into immunodepression or immunosuppression (19–25). Outcomes depend on lymphocytopenia status, especially patients' T-cell counts. Recovery is more likely when lymphocytopenia is resolved, and severe complications arising from lymphocytopenia may lead to death (25–29). In addition, studies report elevated levels of IL-10 in severe and non-survivor cases relative to mild or survivor COVID-19 cases (23–26, 30–32). Thus, as an anti-inflammatory cytokine, IL-10 may be responsible for the reduced T-cell counts that lead to immunodepression in COVID-19 patients (33).
It is essential for our understanding of the disease course to identify the influence of T-cell subsets and IL-10 in patients with mild and severe COVID-19, as well as in survivor and non-survivor cases. This systematic review and meta-analysis aimed to analyze T-cell subsets (CD4/CD8) and IL-10 in severe and fatal cases of COVID-19. Our understanding of the mechanisms causing the immunodepression observed in COVID-19—and its consequences—may enable the early identification of disease severity predictors and development of more effective interventions.
Methods
Protocol and Registration
The systematic review and meta-analysis were performed and reported following the guidelines of the Preferred Reporting Items for Systematic Reviews and Meta-Analysis (PRISMA), including the flow diagram (Figure 1) and checklist (Supplementary Table 1.1) (34). Guidelines provided by the Meta-Analyses Of Observational Studies in Epidemiology (MOOSE), including the checklist (Supplementary Table 1.2) (35) were also followed. The study protocol was registered with the International Prospective Register of Systematic Reviews (PROSPERO); registration number CRD42020218918 (36).
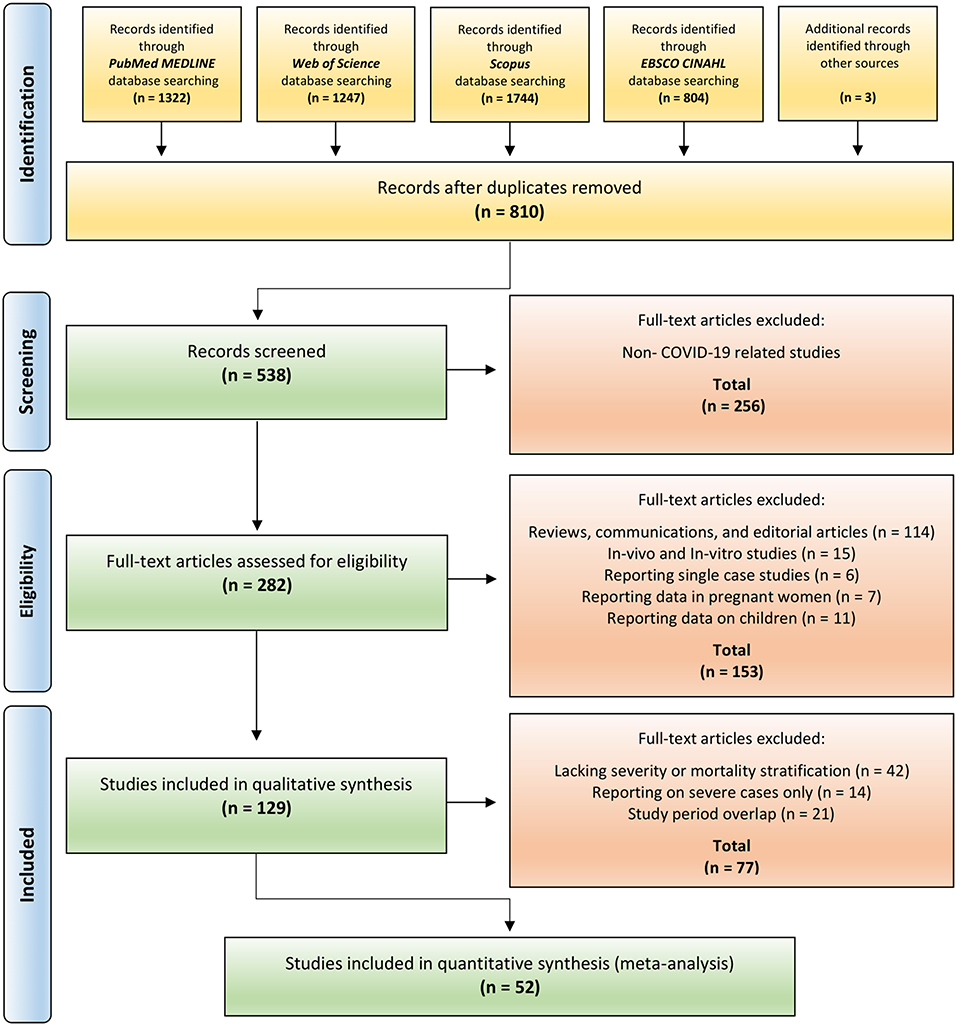
Figure 1. PRISMA flow diagram for T-cell subsets and IL-10 in COVID-19 studies. Figure is adapted from PRISMA flow diagram 2009 (34).
Eligibility Criteria
The PICOS framework—problem/patients/population, intervention/indicator, compare, outcome, and study designs—was used to formulate our research question (37, 38) as follows: Patients: confirmed COVID-19 patients; Indicator: T-cell subsets (CD4/CD8) and IL-10; Comparison: mild vs. severe or survivors vs. non-survivors; Outcome: severity or mortality; Study designs: hospital-based published studies including retrospective, cohort, prospective, descriptive or observational studies, and case series, in which T-cell subsets (CD4/CD8) and IL-10 levels are documented across COVID-19 severity and mortality groups.
Our review also included studies reporting clinical laboratory parameters among confirmed COVID-19 patients, in which clinical diagnosis and classification of patients were carried out following either the WHO guidelines or guidelines published by an official national or regional health governing body. We allowed this criterion to be flexible as the majority of studies only followed WHO guidelines after their recognition of COVID-19 as a pandemic on March 11, 2020 (5, 39). However, studies following national or regional guidelines were included if the criteria for COVID-19 classification was outlined clearly and matches the criteria outlined by the WHO.
The selected studies were hospital-based, and those not reporting on CD4/CD8 T-cells and IL-10, or lacking proper stratification of COVID-19 patients, were excluded. Moreover, to reduce population heterogeneity, we included studies reporting laboratory values for male or female adults and excluded studies reporting on children and pregnant women. However, given that some studies only stratified patients based on sex or provided the mean age of their entire sample, we anticipated a proportion of no more than 10% where studies stated inclusion of children or pregnant women. Furthermore, to eliminate possible differences arising in clinical data obtained from the infection of various SARS-CoV-2 strains, we included studies reported during the first emergence of SARS-CoV-2. Included studies were published in 2020/2021, and data acquisition covered the period from December 2019 to July 2020.
Information Sources
A systematic search was conducted for published journal articles from databases including PubMed MEDLINE, Scopus, Web of Science, and EBSCO CINAHL. Eligible studies identified from the bibliographies of relevant systematic reviews were also included, and a manual search on the preprint server for health sciences, medRxiv, was used to identify unpublished relevant studies. Reviews, opinion articles, editorial material, communications, conference proceedings and abstracts, in-vivo, and in-vitro studies were excluded.
Search Strategy
A systematic search was conducted for journal articles published from December 1, 2019 to March 14, 2021. We used the text words (severe acute respiratory syndrome coronavirus 2), or (SARS-CoV-2), or (coronavirus infection disease 19), or (COVID-19), in conjunction with (T lymphocytes), (T-cells), (CD4), (CD8), (lymphocytes), (interleukin 10), and (IL-10) independently (Supplementary Tables 2.1–2.4). We also applied filters to our search results specifying journal articles and articles published in English.
Study Selection
Articles obtained from each database were imported into a designated EndNote (Clarivate) folder (40). A new folder was created to combine all articles from each database to eliminate duplicates. Retained articles were then exported into an electronic review manager, Colandr (41), to facilitate title, abstract, and full-text screening using the specified eligibility criteria. While performing full-text screening, we performed a manual search of the full article text, including the Supplementary Material, to confirm T-cell subsets (CD4/CD8) and IL-10 were included in each study. This process was carried out independently by six authors, with articles selected by one author being verified by the others. In any cases of disagreement, the final decision about each study was made by consensus from all authors, and decisions were documented in an Excel spreadsheet (Microsoft Corporation) (42).
Data Collection Process
Extracted data from the studies were exported into an Excel spreadsheet. For studies with unclear classification of patients, the corresponding authors were contacted for clarification. Authors were also contacted to request data where studies were missing values for T-cell subsets or IL-10. If no response was received, we performed data extraction from study figures using WebPlotDigitizer, a web-based tool for extracting data from figures (Supplementary Table 3) (43).
Data Items
Data items included author name, publication year, study location (country/city/hospital), study period (duration), study design, assessment of COVID-19 severity (WHO or national/regional health care authority guidelines), timing of proposed classification (on admission or later), sample size (number), sample characteristics (sex, age, underlying conditions), number of cases classified as mild or survivor, number of cases classified as severe or non-survivor, T-cell subsets (CD4/CD8), and IL-10 values stratified by severity or mortality. For laboratory values reported on a continuous scale, the mean and standard deviation (SD), the median with minimum/maximum range, or median and interquartile (IQR) values were extracted. This process was carried out independently by three authors, AFA, JMA, and HFA, and data extracted by one author were verified by the others. Consensus was reached by all authors in any instances of disagreement, and decisions were documented in Excel.
Assessment of Quality and Risk of Bias in Individual Studies
The Newcastle-Ottawa Scale (NOS) for cohort studies was adopted to evaluate selected studies independently (44). The NOS scale uses a star system (0–9) to judge a study based on three domains: sample selection, sample comparability against controls, and assessment of outcome. A higher NOS score (above 7 stars) reflects better quality and a lower risk of bias. In contrast, lower NOS scores (below 4 stars) reflect lower quality and a higher risk of bias. NOS scores of 4–6 stars indicate moderate quality and risk of bias (Supplementary Table 4). All authors independently rated the included studies. Each author documented their scoring decision and subsequently verified the scoring decision of other authors. The final decision for each study was made by consensus from all authors, and scores were documented in Excel.
Synthesis of Extracted Data
Extracted data values from the selected studies were reported on a continuous scale. The mean and SD from each study were pooled to compute the effect size or the standardized mean difference (SMD) and 95% confidence intervals (CI). In the case of median and IQR (minimum and maximum) reporting, we computed the mean and SD based on Wan et al.'s formula (45). The mean and SD were calculated using Cochran's formula for median and IQR reporting without minimum and maximum ranges (46). Furthermore, the means and SDs of similar subgroups were combined into a single group using Cochran's formula for combining similar subgroups (46). Formulas used in this study are listed in Supplementary Tables 5.1–5.2.
Meta-Analysis Summary Measures
Because heterogeneity was expected between individual studies, our meta-analysis used the DerSimonian-Laird inverse variance method for a continuous random-effects model (46, 47). To calculate the SMD, we applied Hedge's g formula to avoid bias in a small study size (48, 49). Statistical analysis of extracted data in this study took place using STATA 17 (StataCorp) (50).
Synthesis of Meta-Analysis Results
The SMD and 95% CI were computed using the mean, SD, and sample size from each study (47). Meta-analysis results were displayed using forest and Galbraith plots following the same meta-analysis computational measures. Statistical analysis of extracted data, including the construction of the forest and Galbraith plots, also used STATA 17 (StataCorp) (50).
Assessment of Heterogeneity
Statistical heterogeneity was assessed using I2 values to measure the degree of inconsistency of collected results produced from the meta-analysis (51, 52). An I2 cut-off value of 0% indicates no heterogeneity, 25% is low, 50% is moderate, and 75% indicates a high level of heterogeneity (52). The Galbraith plot can also be used to assess heterogeneity visually through detecting outliers located outside the 95% CI shaded region (53). STATA 17 (StataCorp) (50) was used to analyze the I2 cut-off value and the Galbraith plot.
Between-study variations can be further explored through subgroup analysis to identify the source of heterogeneity if due to a specific study-level covariate. Heterogeneity induced by the relationship between specific-study effect size and study-level covariates can be measured by performing meta-regression, where the adjusted R2 is used to examine the proportion of between-study variance explained by study-level covariates (54). A bubble plot was also generated following meta-regression as a graphical presentation of the relationship between study-level covariates and the effect size of a specific study (54–56). STATA 17 (StataCorp) was used for the statistical analysis of extracted data, including meta-regression and the construction of the bubble plot (50).
Small Study Effects and Publication Bias
Standard funnel plots and non-parametric trim and fill funnel plots were constructed to detect possible cumulative bias in the studies. The standard funnel plot is used to detect small study effects and publication bias (57, 58). A symmetrical funnel denotes the absence of bias, while an asymmetrical funnel indicates clear publication bias (46). Publication bias may arise because smaller studies with non-significant results are suppressed from publication, leading to a biased sample. With the nonparametric trim and fill analysis, smaller studies inducing the funnel plot asymmetry are removed to estimate the funnel plot true center. This is followed by the addition of removed studies and their missing counterparts around the center (46, 59, 60). This method enables detection and measurement of the influence of missing studies on the overall effect size (46, 59, 60).
The regression-based Egger test was also performed to statistically assess plot distribution asymmetry: P <0.1 indicates publication bias, whereas P > 0.1 indicates no publication bias (61, 62). Measurement of publication bias was also performed using Begg's non-parametric rank correlation based on correlating the standardized effect with the variance using Kendall's tau b (63). Begg's test investigates whether Kendall's rank correlation between the effect size and its variance equals zero: a significant correlation indicates bias (P < 0.05), whereas a non-significant correlation indicates the absence of bias (P > 0.05). Standard funnel plots, nonparametric trim and fill funnel plot analysis, regression-based Egger test, and the nonparametric rank correlation (Begg) test were performed using STATA 17 (StataCorp) (50).
Meta-Analysis Sensitivity Test
The influence of studies harboring a high risk of bias was quantified by performing the Leave-One-Out sensitivity test, where each study is omitted sequentially to measure its influence on the overall combined effect size (64). STATA 17 (StataCorp) was again used for this purpose (50).
Results
Study Selection
An outline of the systematic review search results is presented in Figure 1. We identified 5,120 studies by searching four major databases, including PubMed (1,322), Web of Science (1,247), Scopus (1,744), and EBSCO CINAHL (804). In addition, three records were identified from the bibliographies of relevant systematic reviews and the manual search on the preprint server medRxiv. After removal of duplicates, 810 records were screened. Of those, 272 were excluded following title and abstract review. Retained articles were assessed for study eligibility. Of these, 256 records were excluded as they were not COVID-19 related studies. A further 153 papers were excluded as they were reviews, communications, editorial articles, in-vivo/in-vitro studies or studies reporting on pregnant women or children. Included studies were thoroughly assessed for eligibility criteria. Of those, 77 records were excluded because of lack of stratification based on severity/mortality, for reporting on severe cases only (Supplementary Table 6.1), and because of overlapping study periods (Supplementary Table 6.2). Finally, 52 studies were retained for the synthesis of meta-analysis results (19, 21–25, 27, 30–33, 65–105).
Study Characteristics
The general characteristics of the studies are described in Table 1. They were all hospital-based and included 34 retrospective, 1 retrospective case series, 1 retrospective cohort, 1 prospective, 1 prospective observational, 1 prospective cohort, 10 cohort, and 3 observational cohort studies. The majority of studies were from China with 37 investigations across multiple cities [Wuhan (21), Beijing (4), Hangzhou (3), Guangzhou (2), Taizhou (2), Shanghai (1), Wenzhou (1), Nanchang (1), Nanjing (1), and Jiangsu (1)]. The remaining studies were from the United States [New York (1), New Haven (1), and Cincinnati (1)], United Kingdom [London (2), and Manchester (1)], France [Paris (1), and Creteil (1)], Ireland [Dublin (1)], Italy [Brescia/Monza/Pavia (1)], Spain [Madrid (1)], Netherlands [Breda (1)], Brazil [Curitiba (1)], Korea [Seoul (1)], and Singapore [Singapore (1)] (Figure 2).
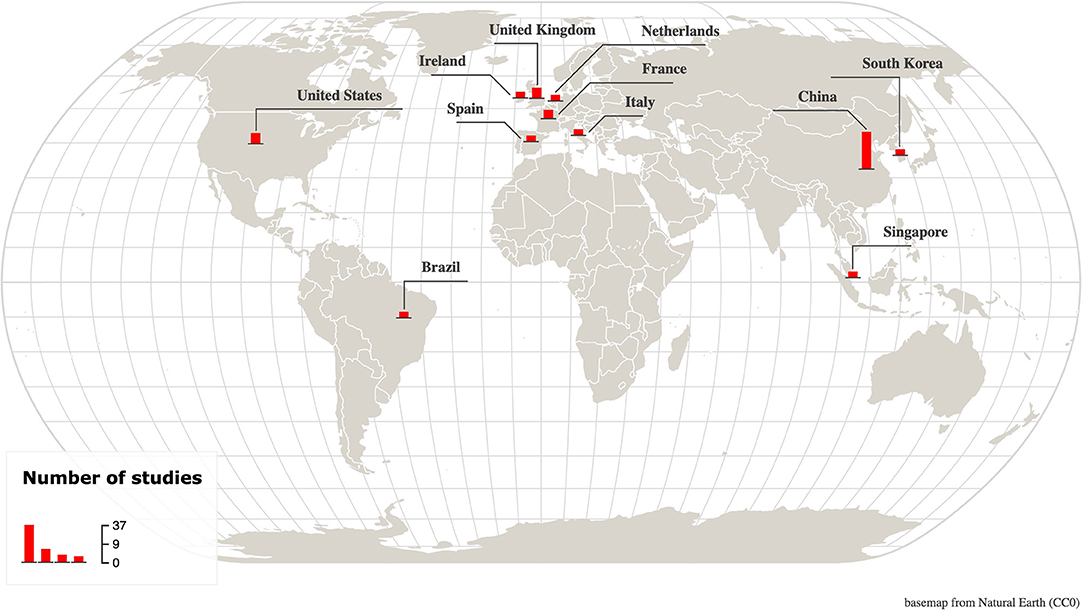
Figure 2. Map showing the location and number of included studies. A total of 52 studies from 11 countries across 3 continents were included. Made with Khartis (106).
The main characteristics and methods for COVID-19 classification in the selected studies are shown in Supplementary Table 7.1. A total of 15 studies classified COVID-19 severity based on WHO guidelines, while 25 studies followed the Chinese National Health Commission or the Chinese Centre for Disease Control and Prevention, and 12 studies used national or local guidelines. With regard to the clinical laboratory tests performed for COVID-19 patients, 37 studies reported sample acquisition within 24 h of admission, 8 reported sample acquisition 1–7 days post-admission, 1 study reported sample acquisition 7–14 days post-admission, and 6 studies did not specify the sample acquisition time (Supplementary Table 7.2). All studies confirmed COVID-19 infection through RT-PCR. Comorbidities varied, with most studies reporting cardio-cerebrovascular disease, hypertension, diabetes, renal disease, liver disease, chronic obstructive pulmonary disease (COPD), and cancer (Supplementary Table 7.3).
Reporting of COVID-19 severity subgroups (e.g., discharged vs. hospitalized; non-ICU vs. ICU) varied greatly between studies. Therefore, each subgroup was assigned to either mild (including moderate) or severe (including critical) categories based on the clinical presentation described in each study. Similarly, the reporting of COVID-19 mortality contained a slight degree of variability (e.g., cured vs. died; hospitalized vs. deceased). Each subgroup was thus classified into either survivors or non-survivors. Some studies reported more than two groups (up to four), and accordingly, we performed a subgroup combination if the stratification for COVID-19 patients was defined clearly in the study. Thus, our meta-analysis only included studies satisfying this criterion. Supplementary Table 7.4 illustrates the method for subgroup assignment from each study, and Supplementary Table 5.2 shows the formula used for combining subgroups.
The population size and age of COVID-19 patients from the included studies are shown in Supplementary Tables 8.1–8.4. The total number of patients by sex is reported as an approximate number because some studies did not describe gender. The mean population size based on severity was 7,913, including 3,905 males and 3,680 females. Of those, 5,109 (2,357 males/2,493 females) were classified as mild, and 2,804 (1,548 males/1,087 females) were severe. In contrast, the mean population size based on mortality was 3,420, including 1,813 males and 1,508 females. Of those, 2,662 (1,350 males/1,268 females) were sub-grouped as survivors, and 758 (463 males/240 females) as non-survivors. The mean age was 53.03 ± 25.6 for the mild group, and 63.39 ± 22.61 for the severe patients. In contrast, the mean age was 59.36 ± 16.11 for the survivors, and 70.67 ± 12.82 for the non-survivors.
Risk of Bias Within Studies
Studies were examined for quality to avoid bias, and a score was assigned to each study using the NOS scale for quality assessment of cohort studies, shown in Table 1 and detailed in Supplementary Table 4. The majority of studies received scores indicating high quality: 5 studies received a score of 9, 8 studies received scores of 8, and 26 studies received scores of 7. A total of 13 studies received scores of 6, indicating moderate quality. The sensitivity analysis of our results did not differ markedly following the exclusion of moderate quality studies.
Meta-Analysis Results of Individual Studies
For the pairwise comparison between mild and severe COVID-19, 24 studies reported decreased counts for CD4 T-cells in the severe cases compared with mild ones (SMD = −1.39 to −0.11), and only 2 studies reported increased counts for CD4 T-cells (SMD = 0.25 to 0.34) (Figure 3A and Supplementary Table 9.1). Similarly, 25 studies reported decreased counts for CD8 T-cells in severe cases relative to mild ones (SMD = −1.49 to 0.02), and only 1 study reported increased counts for CD8 T-cells (SMD = 0.55) (Figure 3B and Supplementary Table 9.2). In contrast, all 38 studies of IL-10 reported increased IL-10 levels in the severe cases relative to mild ones (SMD = 0.19 to 1.57) (Figure 4 and Supplementary Table 9.3).
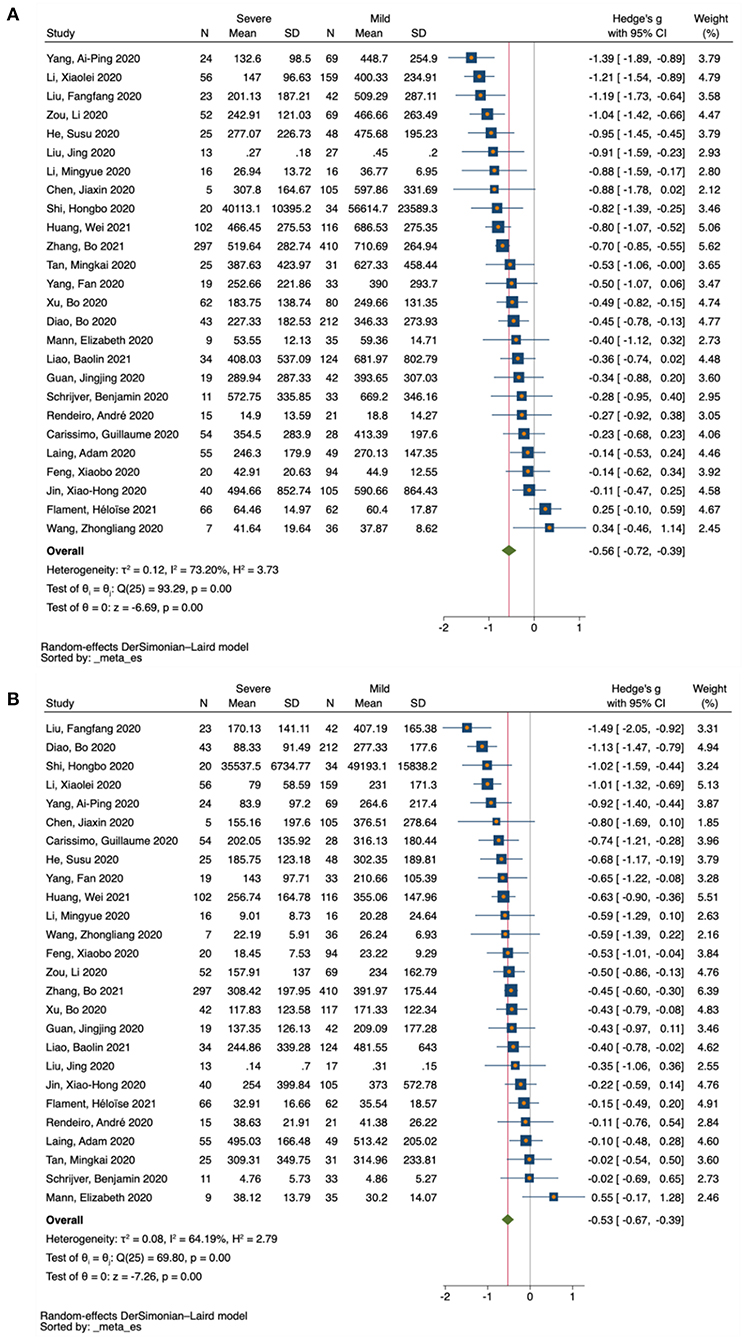
Figure 3. Forest plot of T-cell subsets in COVID-19 severity studies. (A) CD4 T-cells in COVID-19 severity studies. (B) CD8 T-cells in COVID-19 severity studies. The no-effect line is represented at the value of zero. The diamond symbol represents estimated combined effect size.
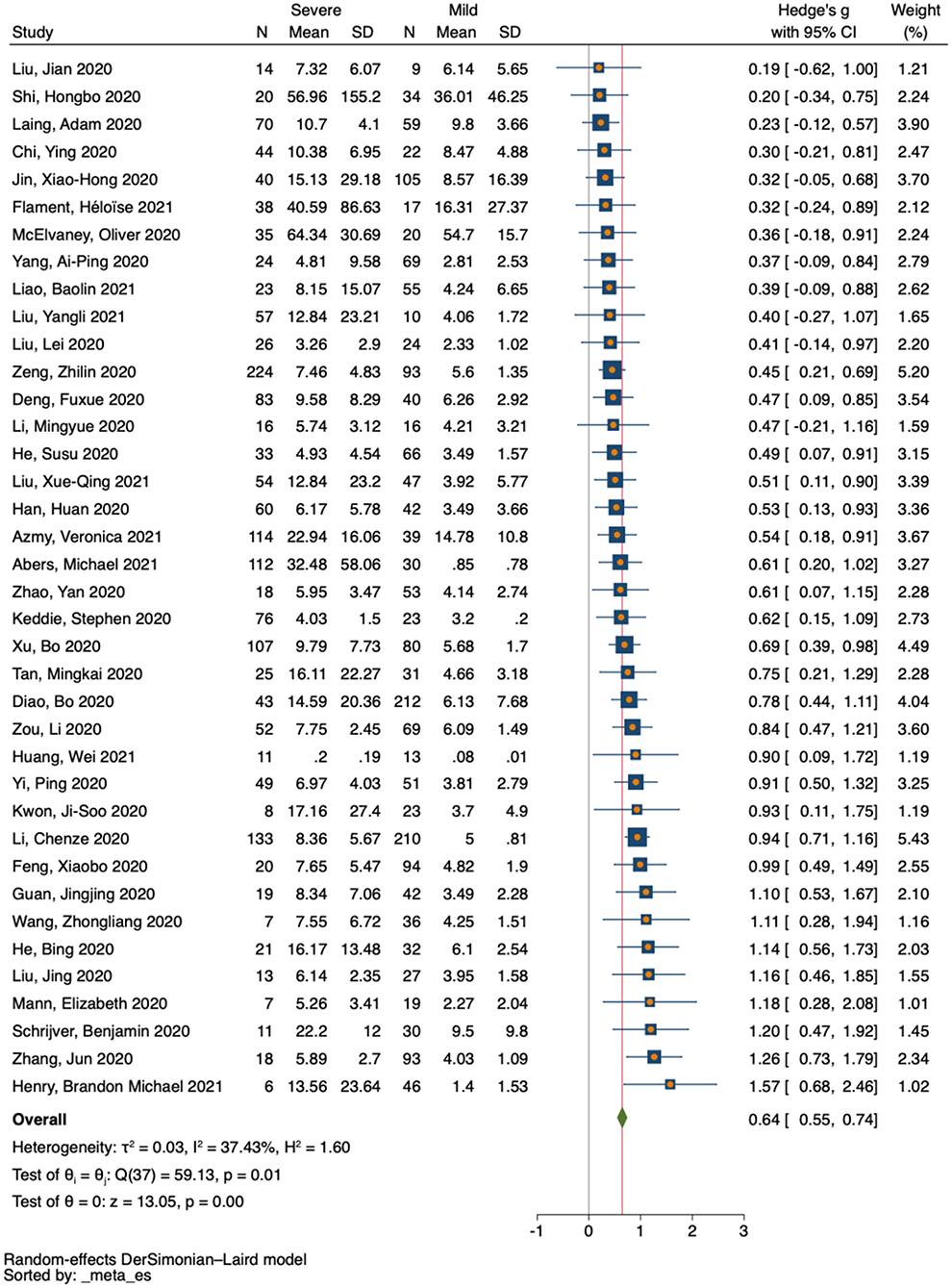
Figure 4. Forest plot of IL-10 in COVID-19 severity studies. The no-effect line is represented at the value of zero. The diamond symbol represents estimated combined effect size.
All included studies for CD4 T-cells (six studies) reported decreased counts in the non-survivors relative to survivors (SMD = −0.99 to −0.03) (Figure 5A and Supplementary Table 9.4), for the pairwise comparison between COVID-19 survivors and non-survivors. Similarly, four studies reported decreased counts for CD8 T-cells among non-survivors compared with survivors (SMD = −0.89 to −0.47), and only two studies reported increased counts for CD8 T-cells (SMD = 0.17 to 0.36) (Figure 5B and Supplementary Table 9.5). In contrast, nine studies reported increased levels of IL-10 in the non-survivors relative to survivors (SMD = 0.29 to 1.6), and only one study reported decreased levels of IL-10 (SMD = −0.01) (Figure 5C and Supplementary Table 9.6).
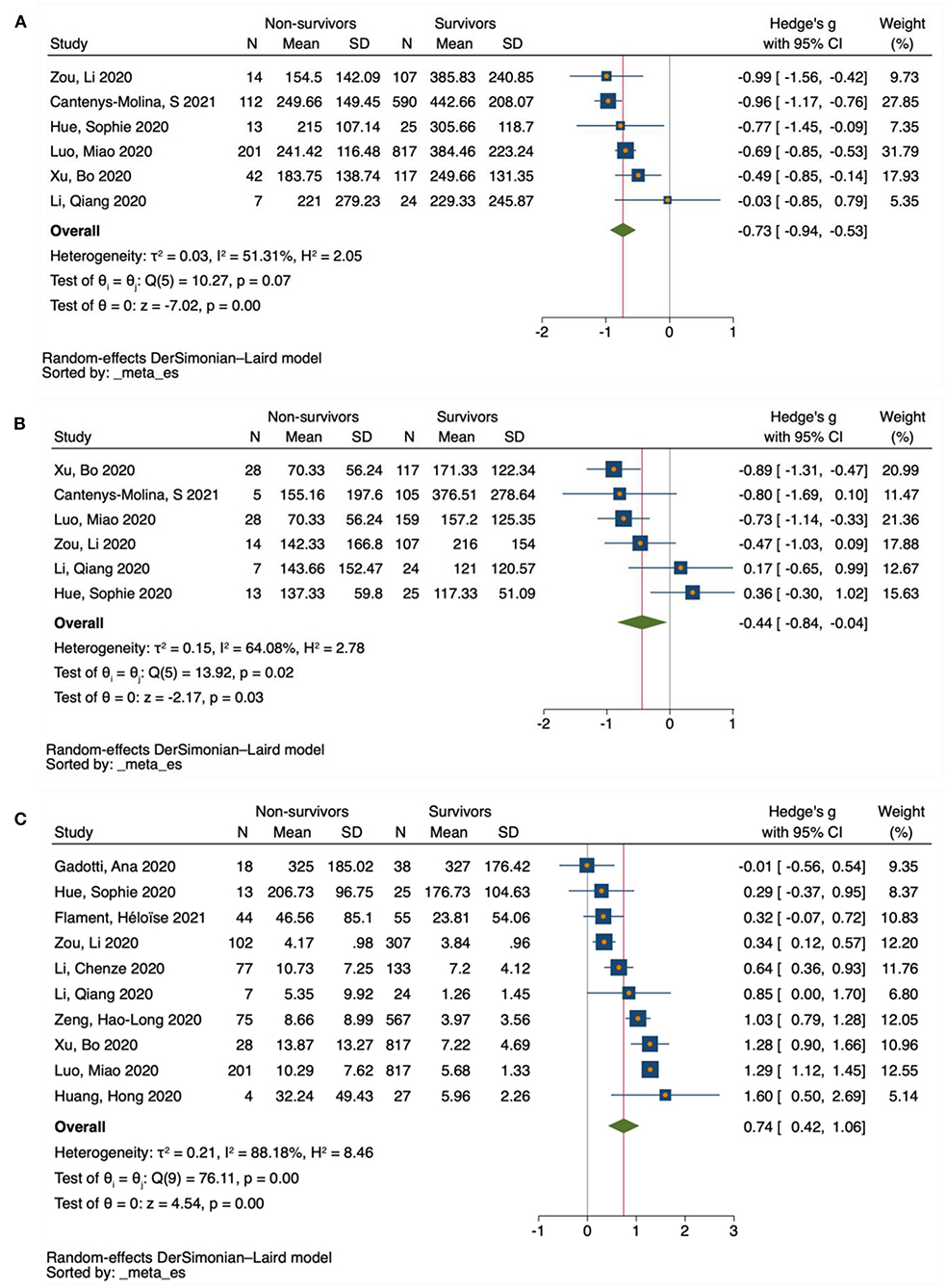
Figure 5. Forest plot of T-cell subsets and IL-10 in COVID-19 mortality studies. (A) CD4 T-cells in COVID-19 mortality studies. (B) CD8 T-cells in COVID-19 mortality studies. (C) IL-10 in COVID-19 mortality studies. The no-effect line is represented at the value of zero. The diamond symbol represents estimated combined effect size.
Meta-Analysis Results of Combined Studies
Forest Plot Meta-Analysis
Based on severity, a total of 26 eligible studies were used for pairwise comparison between mild and severe COVID-19 cases for T-cell subsets. There were 2080 subjects in the mild group vs. 1,112 in the severe group for CD4 T-cells, and 2,107 subjects in the mild group vs. 1,092 in the severe group for CD8 T-cells. Our forest plot meta-analysis revealed an increased effect size of having reduced counts of CD4 and CD8 T-cells in the severe group relative to the mild group (SMD = −0.56, 95% CI = −0.72 to −0.39; SMD = −0.53, 95% CI = −0.67 to −0.39, respectively) (Figures 3A,B). Similarly, a total of 38 eligible studies were used for pairwise comparison between mild and severe COVID-19 cases for IL-10, with a total of 1,981 subjects in the mild group vs. 1,731 in the severe group. The forest plot meta-analysis revealed an increased effect size of having elevated levels of IL-10 in the severe group compared with the mild group (SMD = 0.64, 95% CI = 0.55 to 0.74) (Figure 4).
In terms of mortality, six eligible studies were used for pairwise comparison between survivor and non-survivor COVID-19 cases for T-cell subsets. A total of 1,680 cases were noted in the survivor group vs. 389 in the non-survivor group for CD4 T-cells, and 537 in the survivor group vs. 95 in the non-survivor group for CD8 T-cells. Our forest plot meta-analysis revealed an increased effect size of having reduced CD4 and CD8 T-cell counts in the non-survivor group compared with the survivor group (SMD = −0.73, 95% CI = −0.94 to −0.53, and SMD = −0.44, 95% CI = −0.84 to −0.04, respectively) (Figures 5A,B). Similarly, considering mortality, a total of 10 studies were used for pairwise comparison between survivor and non-survivor COVID-19 cases for IL-10, with a total of 2810 subjects in the survivor group vs. 569 in the non-survivor group. The forest plot meta-analysis revealed an increased effect size of having elevated levels of IL-10 in the non-survivor group compared with the survivor group (SMD = 0.74, 95% CI = 0.42 to 1.06) (Figure 5C).
Galbraith Plot Meta-Analysis
A total of 26 studies were used for pairwise comparison between mild and severe COVID-19 cases for T-cell subsets. For CD4 T-cell studies, the Galbraith meta-analysis revealed one study located outside the 95% CI region, and one on the 95% CI borderline (Figure 6A). For CD8 T-cell studies, the meta-analysis revealed two studies located outside the 95% CI region (Figure 6B). Similarly, using severity, a total of 38 eligible studies were used for pairwise comparison between mild and severe COVID-19 cases for IL-10. The meta-analysis for IL-10 revealed two studies on the 95% CI borderline (Figure 6C).
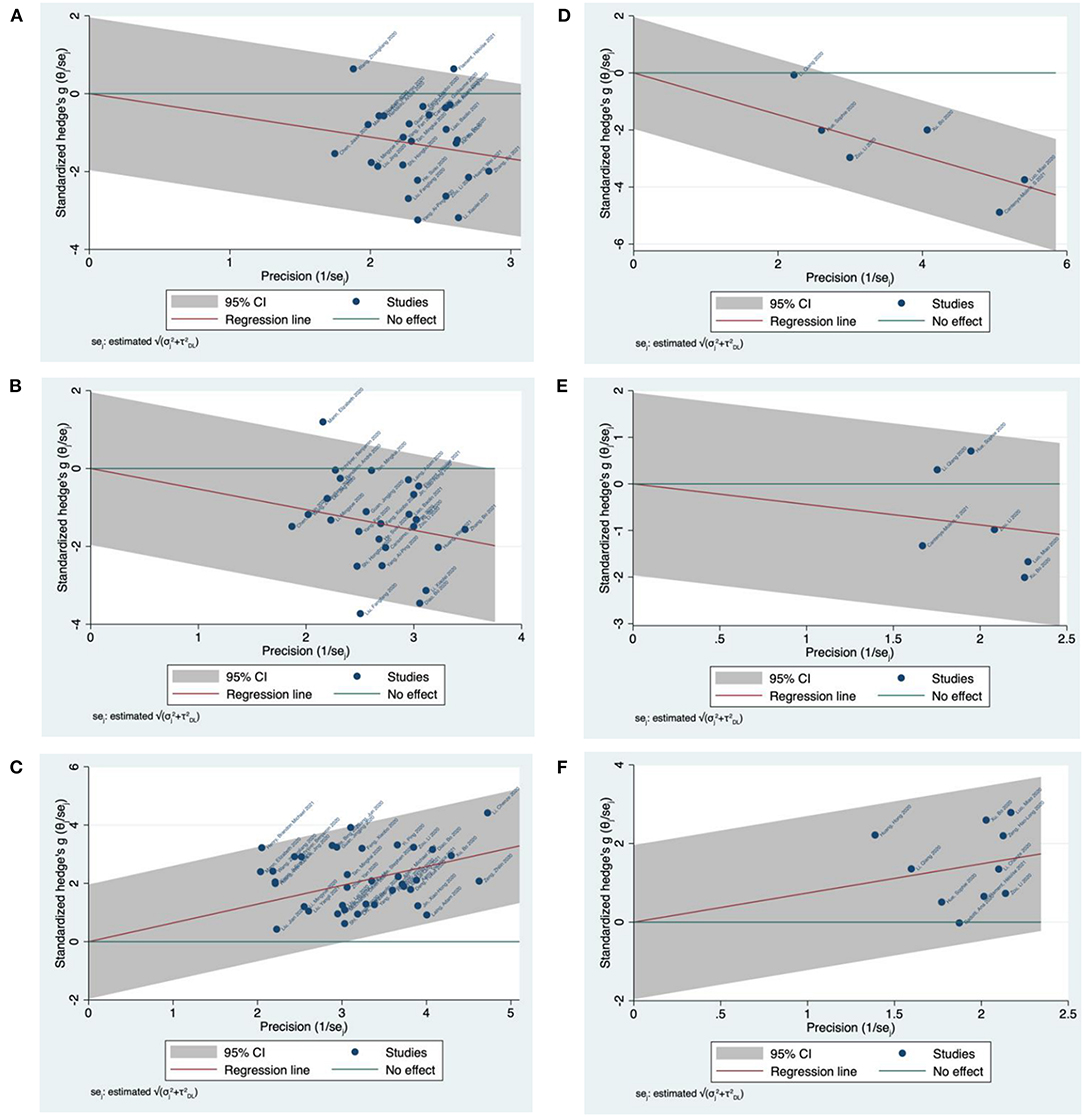
Figure 6. Galbraith plot meta-analysis for CD4/CD8 T-cells and IL-10 in COVID-19 severity and mortality studies. (A) CD4 T-cells in COVID-19 severity studies. (B) CD8 T-cells in COVID-19 severity studies. (C) IL-10 in COVID-19 severity studies. (D) CD4 T-cells in COVID-19 mortality studies. (E) CD8 T-cells in COVID-19 mortality studies. (F) IL-10 in COVID-19 mortality studies.
Focusing on mortality, six studies were used for pairwise comparison between survivor and non-survivor COVID-19 cases for T-cell subsets. For CD4 and CD8 T-cell studies, all included studies were located within the 95% CI region (Figure 6D) and (Figure 6E), respectively. Similarly, a total of 10 eligible studies were used for pairwise comparison between survivor and non-survivor COVID-19 cases for IL-10. The meta-analysis revealed all included studies were located within the 95% CI region (Figure 6F).
Assessment of Heterogeneity
The degree of heterogeneity among results from the meta-analysis was assessed using I2 values. Based on severity, a moderate level of heterogeneity was observed among studies reporting on CD4 T-cells (P < 0.001, I2 = 73.20%) (Figure 3A), and CD8 T-cells (P < 0.001, I2 = 64.19%) (Figure 3B). Thus, a low level of heterogeneity was observed among studies reporting on IL-10 (P < 0.001, I2 = 37.43%) (Figure 4). Similarly, based on mortality, a moderate level of heterogeneity was observed in studies reporting on CD4 T-cells (P< 0.001, I2 = 51.31%) (Figure 5A), and CD8 T-cells (P < 0.001, I2 = 64.08%) (Figure 5B). However, a high level of heterogeneity was observed for studies reporting on IL-10 (P < 0.001, I2 = 88.18%) (Figure 5C).
Subgroup Analysis
A subgroup analysis and meta-regression test were used to assess the source of heterogeneity using study-level covariates stratifying studies based on location (city, country, and continent), study design (retrospective, prospective, or cohort), classification protocol (WHO NCP, CDC, National Health Commission of China, or National/Regional Guidelines), sample acquisition time (at admission or later), population influence (total number of male/female, and mean age of patients), and laboratory influence (test procedure) followed by construction of a bubble plot. Moreover, the covariate of continent was stratified as America, Asia, and Europe. Thus, studies published in America and Europe were sometimes combined due to insufficient studies in each group. The covariate city was stratified as Wuhan or other cities because most studies were based in Wuhan. Similarly, the covariate country was stratified as China or other countries because most studies were based in China. In addition, the total number of male/female patients in each study was indicated as (more or <50), and the mean age of patients was indicated as (more or <50 or 60). We found city, continent, classification protocol, total number of male/female, and mean age of patients were study-level covariates successful in reducing the heterogeneity observed in included studies (in varying degrees based on the parameter being tested).
Based on severity, between-study variability was detected and measured for CD4/CD8 T-cell and IL-10 studies. For CD4 T-cells, the stratification of studies based on the continent covariate revealed a greater heterogeneity among studies conducted in Asia (P < 0.001, I2 = 66.76%) than for those in Europe and the Americas (P < 0.001, I2 = 16.79%) (Supplementary Figure 10.3A). Furthermore, the meta-regression analysis revealed that 25.15% of heterogeneity could be explained by the selected covariate (continent) (Supplementary Figure 10.3B) and the effect size increase in studies conducted in Europe or the Americas compared with Asia (Supplementary Figure 10.3C). Similarly, the stratification of CD8 T-cell studies based on the continent covariate revealed a higher heterogeneity among studies conducted in Asia (P < 0.001, I2 = 56.01%) than for studies conducted in Europe and the Americas (P < 0.001, I2 = 0.00%) (Supplementary Figure 10.13A). The meta-regression analysis indicated that 42.38% of the heterogeneity could be explained by the selected covariate (continent) (Supplementary Figure 10.13B) and the effect size increase in studies conducted in Europe or the Americas compared with Asia (Supplementary Figure 10.13C). However, the stratification of IL-10 studies, based on the covariate city, revealed greater heterogeneity among studies conducted in Wuhan (P < 0.001, I2 = 36.10%) compared with other cities (P < 0.001, I2 = 26.39%) (Supplementary Figure 10.21A). In addition, the meta-regression analysis showed that 10.91% of heterogeneity could be explained by the selected covariate (city) (Supplementary Figure 10.21B) and the effect size increase in studies conducted in Wuhan compared with other cities (Supplementary Figure 10.21C).
The total number of included studies was insufficient to perform a subgroup analysis focusing on mortality. However, between-study variability was measured for CD4/CD8 T-cell and IL-10 studies. For CD4 T-cells, the stratification of studies based on the covariate (classification protocol) revealed more heterogeneity among studies conducted following the WHO NCP or CDC guidelines (P < 0.001, I2 = 37.41%) in comparison to those following national or local guidelines (P < 0.001, I2 = 0.00%) (Supplementary Figure 10.35A). The analysis revealed that 100% of heterogeneity could be explained by the selected covariate (classification protocol) (Supplementary Figure 10.35B) and the effect size increase in studies conducted following WHO NCP or CDC guidelines compared with those following national or local guidelines (Supplementary Figure 10.35C). Stratification of CD8 T-cell studies based on the covariate (total number of male/female patients) revealed homogeneity among studies conducted in studies when the total number of male/female patients is (<50) (P < 0.001, I2 = 0.00%) or (>50) (P < 0.001, I2 = 0.00%) (Supplementary Figures 10.47A, 10.48A). Furthermore, the meta-regression analysis revealed that 100% of the heterogeneity was explained by the selected covariate (total number of male/female patients) (Supplementary Figures 10.47B, 10.48B), and the effect size decrease in studies when the number of male/female patients is (>50) compared with those (<50) (Supplementary Figures 10.47C, 10.48C). Moreover, the stratification of IL-10 studies, based on the covariate (mean age), revealed greater heterogeneity among studies when the mean age of patients is (>60) (P < 0.001, I2 = 82.18%) compared with those (<60) (P<0.001, I2 = 0.00%) (Supplementary Figure 10.59A). The analysis revealed that 44.16% of heterogeneity could be explained by the selected covariate (mean age) (Supplementary Figure 10.59B) and the effect size decrease in studies when the mean age of patients is (>60) compared with those (<60) (Supplementary Figure 10.59C).
Small Study Effects and Publication Bias
Where heterogeneity was due to small study effects or publication bias, funnel plots were constructed to compare COVID-19 severity and mortality studies. In addition, the regression-based Egger test (using study-level covariates) and the non-parametric rank correlation (Begg) test were performed to statistically evaluate the funnel plot symmetry. Visual inspection of the funnel plot for CD4 T-cell severity studies suggested symmetrical distribution among included studies (Supplementary Figure 11.1A). The regression-based Egger's test with study-level covariates showed no evidence of small study effects or publication bias (P > 0.1) (Supplementary Figure 11.1B), a finding supported by the non-parametric rank correlation Begg's test (P > 0.05) (Supplementary Figure 11.1C). Funnel plot symmetry was also confirmed by the non-parametric trim and fill analysis that revealed no missing studies (Figure 7A). Similarly, visual inspection of the funnel plot for CD8 T-cell severity studies suggested symmetrical distribution among the studies (Supplementary Figure 11.2A). The regression-based Egger's test with study-level covariates indicated no small study effects or publication bias (P > 0.1) (Supplementary Figure 11.2B), along with the non-parametric rank correlation Begg's test (P > 0.05) (Supplementary Figure 11.2C). Funnel plot symmetry was also confirmed by the non-parametric trim and fill analysis that revealed no missing studies (Figure 7B). In contrast, visual inspection of the funnel plot for IL-10 severity studies suggested asymmetrical distribution among included studies (Supplementary Figure 11.3A), and the regression-based Egger's test with study-level covariates indicated small study effects or publication bias (P<.1) (Supplementary Figure 11.3B). Although the non-parametric rank correlation Begg's test revealed no evidence of small study effects or publication bias (P > 0.05) (Supplementary Figure 11.3C), funnel plot asymmetry was confirmed by the non-parametric trim and fill analysis that revealed four missing studies. However, the overall effect size did not vary greatly with the addition of the four imputed studies (observed SMD = 0.644, 95% CI = 0.547 to 0.741 and observed + imputed SMD = 0.605, 95% = 0.503 to 0.707) (Figure 7C).
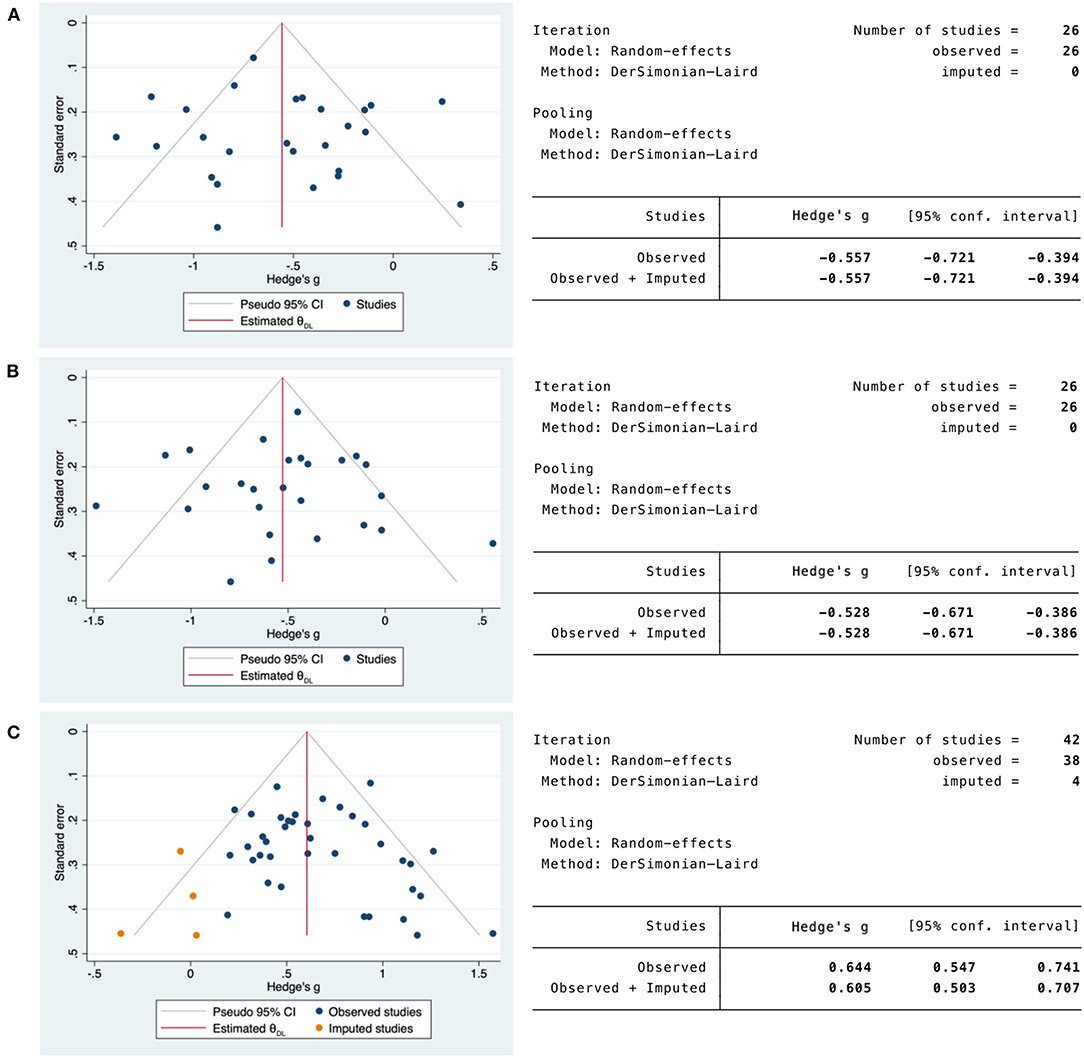
Figure 7. Non-parametric trim and fill funnel plot analysis of publication bias for CD4/CD8 T-cells and IL-10 in COVID-19 severity studies. (A) CD4 T-cells in COVID-19 severity studies. (B) CD8 T-cells in COVID-19 severity studies. (C) IL-10 in COVID-19 severity studies. Observed studies (blue). Imputed studies (orange).
Visual inspection of the funnel plot for CD4/CD8 T-cell and IL-10 mortality studies suggested symmetrical distribution among included studies (Supplementary Figures 11.4A, 11.5A, 11.6A). The regression-based Egger's test with study-level covariates showed no evidence of small study effects or publication bias (P > 0.1) (Supplementary Figures 11.4B, 11.5B, 11.6B), a finding supported by the nonparametric rank correlation Begg's test (P > 0.05) (Supplementary Figures 11.4C, 11.5C, 11.6C). Funnel plot symmetry for CD4/CD8 T-cell and IL-10 mortality studies was also confirmed by the non-parametric trim and fill analysis that revealed no missing studies (Figures 8A–C).
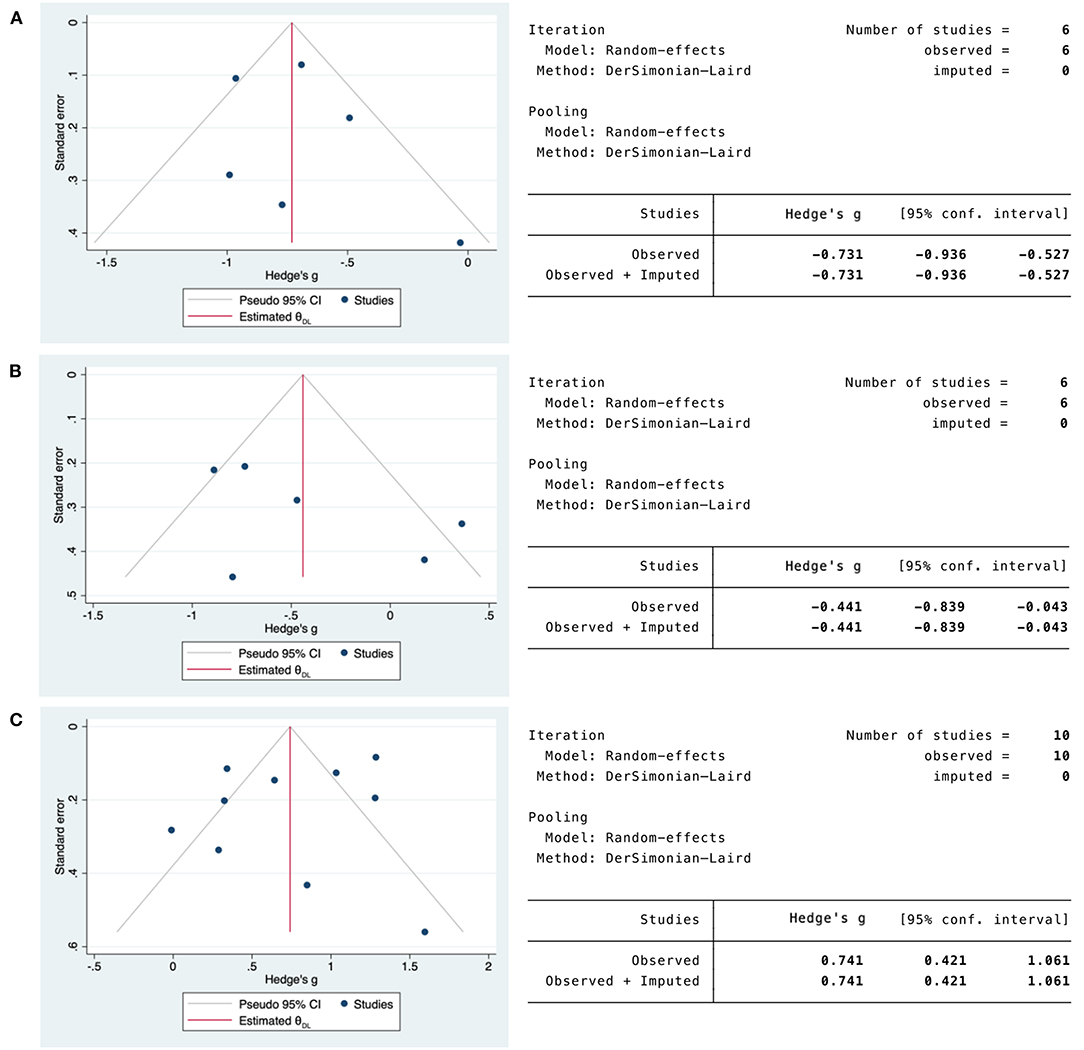
Figure 8. Non-parametric trim and fill funnel plot analysis of publication bias for CD4/CD8 T-cells and IL-10 in COVID-19 mortality studies. (A) CD4 T-cells in COVID-19 mortality studies. (B) CD8 T-cells in COVID-19 mortality studies. (C) IL-10 in COVID-19 mortality studies. Observed studies (blue). Imputed studies (orange).
Meta-Analysis Sensitivity Test
We conducted a Leave-One-Out sensitivity analysis to compare mild vs. severe cases and for comparison between survivors vs. non-survivors. Results from each study in the severity group contributed similarly to the overall results for the combined effect size [SMD = −0.52 to −0.58 for CD4 T-cell studies (Supplementary Figure 12.1), SMD = −0.50 to −0.56 for CD8 T-cell studies (Supplementary Figure 12.2), and SMD = 0.65 to 0.63 for IL-10 studies (Supplementary Figure 12.3)]. Results from each study in the mortality group contributed similarly to the overall result of the combined effect size [SMD = −0.70 to −0.77 for CD4 T-cell studies (Supplementary Figure 12.4), (SMD = −0.32 to −0.63 for CD8 T-cell studies (Supplementary Figure 12.5), and SMD = 0.82 to 0.69 for IL-10 studies (Supplementary Figure 12.6)].
Discussion
The SARS-CoV-2 infection continues to spread globally, with millions of lives lost and many individuals at high risk of developing serious or life-threatening complications. Although vaccines can effectively reduce risks associated with SARS-CoV-2, new variants have been identified worldwide that may challenge the effectiveness of current vaccines (107–109). In addition, vaccination may not be a feasible option for immunocompromised patients or in cases where infection precedes vaccination. Therefore, examining the underlying pathophysiological manifestations of SARS-CoV-2 infection within its human host, as reflected in the resulting clinical picture and laboratory parameters, is paramount to our ability to prevent disease progression or mortality in the present time and the future.
Lymphocytopenia is a common outcome among COVID-19 patients, observed mainly as decreased counts of CD4 and CD8 T-cells (26, 96, 110–112). Several studies have shown that patients with recovered T-cell counts experience improvement in their health status, while the condition of patients with non-recovered T-cell counts worsens and sometimes leads to death (110, 113). Thus, the main objective of this review was to assess the magnitude of this immunodepression and its role in enabling the early identification of mild and severe COVID-19 cases and potential survivor and non-survivor cases. Elucidating the influence of SARS-CoV-2 infection on host immunity among COVID-19 cases allows for a better understanding of the disease course and intervention needed during the early phase following infection.
Our meta-analysis shows that COVID-19 alters the status of human host immunity by driving the depression of adaptive immunity, manifesting in the lower counts of CD4 and CD8 T-cells more evident in severe and non-survivor cases. This observation aligns with recent systematic reviews and meta-analyses in which T-cell subsets were also reduced (114–121). The immune system's ability to overcome the disease by reversing immunosuppression is critical, considering that our systematic review and meta-analysis have shown that mild cases and survivors exhibit a slight depression or possibly recovered T-cell counts compared with severe cases or non-survivors. T-cells play a crucial role in adaptive immunity, essential for fighting infection. Several lines of evidence reveal that in COVID-19, innate immunity is mainly responsible for inducing the inflammation observed following infection with SARS-CoV-2. This translates into a cytokine storm prevalent among patients diagnosed with COVID-19. However, adaptive immunity is essential for virus recognition and immunity against subsequent infection. The fact that this arm of the immune system is paralyzed following infection with SARS-CoV-2 indicates that host ability to produce specific immunity against the virus is compromised. Furthermore, the initiation of the adaptive immune response requires the CD4 T-cells to recognize viral-associated antigens necessary to initiate recognition through antigen-presenting cells (APCs) (122). Similarly, viral clearance through cytotoxic CD8 T-cells is critical following viral infections (123, 124). However, if the very tool that enables antigen recognition and viral clearance is downregulated, the whole arm of adaptive immunity may be affected. This clinical picture is noticed in COVID-19 patients, as described by the cumulative studies in our meta-analysis showing that following infection with SARS-CoV-2, CD4, and CD8 T-cell counts are reduced.
Several mechanisms have been put forward to explain the reduced T-cell counts observed in COVID-19 patients, which may be due to direct infection of T-cells with SARS-CoV-2, impaired T-cell proliferation and activation, T-cell exhaustion and apoptosis, or as a consequence of excessive production of inflammatory cytokines (33, 93, 125–129). Moreover, several cytokines have been found to inversely correlate with T-cell counts in COVID-19, including IL-10, suggesting a possible involvement in T-cell reduction following infection with SARS-CoV-2 (33). This aligns with the fact that during viral infections, the secretion of IL-10 has been found to inhibit the expression of major histocompatibility class II (MHC II) and co-stimulatory molecules CD80 and CD86 on APCs. This restricts CD4 T-cells' ability to recognize viral antigens, and as a consequence CD8 T-cells lose the activation signals initiated by CD4 T-cells (124, 130, 131).
In addition, studies in our meta-analysis revealed that IL-10 concentration is increased following SARS-CoV-2 infection, suggesting that IL-10 may function as a predictor of patients' clinical status and survival. Our meta-analysis results show that IL-10 is increased in severe cases and non-survivors relative to mild or survivor COVID-19 cases. This observation has also been recorded in similar systematic reviews and meta-analysis studies conducted with COVID-19 confirmed patients. Their IL-10 levels were also increased following infection with SARS-CoV-2 (115–117, 119, 120, 132, 133). IL-10 downregulates an exacerbated immune response or excessive inflammation as an anti-inflammatory cytokine (134, 135). However, in COVID-19, IL-10 is associated with severe cases and mortality because the cytokine storm intensifies. Thus, as a counterbalancing cytokine, IL-10 should facilitate the recovery from the cytokine storm initiated primarily through the secretion of IL-6, given that several lines of evidence indicate the involvement of IL-6 in the cytokine storm following SARS-CoV-2 infection (25, 136). Interestingly, IL-6 and IL-10 are increased among severe and non-survivor COVID-19 cases (25). This may indicate that in COVID-19, IL-10 enhances the pro-inflammatory environment. Further, IL-10 inhibits the activation of adaptive immunity by suppressing the function of antigen recognition on APCs, pointing to the possible role of IL-10 as the main driver of the immunosuppression observed in patients with COVID-19. However, more research is needed to verify this hypothesis.
Conclusion
Our systematic review and meta-analysis revealed that CD4 and CD8 T-cells are reduced in COVID-19 patients. The studies show that this reduction is more evident in the severe and non-survivor cases than in mild or survivor cases. In addition, our meta-analysis indicated that the IL-10 concentration increases, especially in the severe and non-survivor cases relative to mild or survivor cases. We conclude that the immunodepression observed following infection with SARS-CoV-2 is possibly driven by IL-10. Moreover, evidence demonstrates that the levels of CD4 and CD8 T-cells, and IL-10, are associated with severity and mortality, suggesting the importance of including such critical parameters in the routine diagnostic panel for COVID-19 patients as predictors of severity and mortality following SARS-CoV-2 infection.
Study Limitations
This systematic review and meta-analysis investigated the levels of T-cell subsets and IL-10 in COVID-19 patients. Conclusive data are still emerging because of the relatively short time since the emergence of SARS-CoV-2. Studies that described T-cell subsets together with IL-10 were also scarce, and lymphocyte counts were sometimes reported without dissecting the subsets. These limitations made it challenging to obtain a focused larger study size. In addition, including studies published only in the English language may also have limited our results. However, we believe that the quality of our search and the data obtained could assist in understanding the COVID-19 clinical picture, consistent with the goal of collating reliable sources to aid the scientific community and health care providers in their combat against this novel disease. Nonetheless, further studies are needed to support our conclusions and findings.
Data Availability Statement
The original contributions presented in the study are included in the article/Supplementary Material, further inquiries can be directed to the corresponding author.
Author Contributions
AA was involved in study conceptualization, literature search, data curation, analysis and interpretation of the data, generation of the figures, and in drafting and revising the manuscript. JA and HaA were involved in the literature search, data curation, analysis and interpretation of the data, and in revising the manuscript. KA and AA-S were involved in the data curation, analysis and interpretation of the data, and in revising the manuscript. HeA was involved in the data curation, analysis and interpretation of the data, generation of the figures, and in revising the final version of the manuscript. All authors have approved the final version of the manuscript.
Funding
The research project was supported by a grant from Research Centre of the Female Scientific and Medical Colleges, Deanship of Scientific Research, King Saud University.
Conflict of Interest
The authors declare that the research was conducted in the absence of any commercial or financial relationships that could be construed as a potential conflict of interest.
Publisher's Note
All claims expressed in this article are solely those of the authors and do not necessarily represent those of their affiliated organizations, or those of the publisher, the editors and the reviewers. Any product that may be evaluated in this article, or claim that may be made by its manufacturer, is not guaranteed or endorsed by the publisher.
Acknowledgments
The authors acknowledge the resources and support from the research core facilities at King Saud University and Riyadh Elm University.
Supplementary Material
The Supplementary Material for this article can be found online at: https://www.frontiersin.org/articles/10.3389/fmed.2022.852749/full#supplementary-material
References
1. Li Q, Guan X, Wu P, Wang X, Zhou L, Tong Y, et al. Early transmission dynamics in Wuhan, China, of novel coronavirus-infected pneumonia. N Engl J Med. (2020) 382:1199–207. doi: 10.1056/NEJMoa2001316
2. Gorbalenya AE, Baker SC, Baric RS, de Groot RJ, Drosten C, Gulyaeva AA, et al. The species severe acute respiratory syndrome-related coronavirus: classifying 2019-nCoV and naming it SARS-CoV-2. Nat Microbiol. (2020) 5:536–44. doi: 10.1038/s41564-020-0695-z
3. Zhou P, Yang XL, Wang XG, Hu B, Zhang L, Zhang W, et al. A pneumonia outbreak associated with a new coronavirus of probable bat origin. Nature. (2020) 579:270–3. doi: 10.1038/s41586-020-2012-7
4. CSSE. Coronavirus COVID-19 Global Cases: The Center for Systems Science and Engineering (CSSE) at Johns Hopkins University (JHU). (2021). Available online at: https://gisanddata.maps.arcgis.com/apps/opsdashboard/index.html#/bda7594740fd40299423467b48e9ecf6
5. WHO. Director-General's Opening Remarks at the Media Briefing on COVID-19. Geneva: World Health Organization (2020).
6. ENT. Loss of Sense of Smell as Marker of COVID-19 Infection. London: Ear, Nose and Throat Surgery at The Royal College of Surgeons of England (2020).
7. Bagheri SH, Asghari A, Farhadi M, Shamshiri AR, Kabir A, Kamrava SK, et al. Coincidence of COVID-19 epidemic and olfactory dysfunction outbreak in Iran. Med J Islam Repub Iran. (2020) 34:62. doi: 10.1101/2020.03.23.20041889
8. Kaye R, Chang CWD, Kazahaya K, Brereton J, Denneny JC III. COVID-19 anosmia reporting tool: initial findings. Otolaryngol Head Neck Surg. (2020) 163:132–4. doi: 10.1177/0194599820922992
9. Guan W-j, Ni Z-y, Hu Y, Liang W-h, Ou C-q, He J-x, et al. Clinical characteristics of coronavirus disease 2019 in China. N Engl J Med. (2020) 382:1708–20. doi: 10.1056/NEJMoa2002032
10. Chen N, Zhou M, Dong X, Qu J, Gong F, Han Y, et al. Epidemiological and clinical characteristics of 99 cases of 2019 novel coronavirus pneumonia in Wuhan, China: a descriptive study. Lancet. (2020) 395:507–13. doi: 10.1016/S0140-6736(20)30211-7
11. Zhu N, Zhang D, Wang W, Li X, Yang B, Song J, et al. A novel coronavirus from patients with pneumonia in China, 2019. N Engl J Med. (2020) 382:727–33. doi: 10.1056/NEJMoa2001017
12. Hui DS, Azhar EI, Madani TA, Ntoumi F, Kock R, Dar O, et al. The continuing 2019-nCoV epidemic threat of novel coronaviruses to global health - the latest 2019 novel coronavirus outbreak in Wuhan, China. Int J Infect Dis. (2020) 91:264–6. doi: 10.1016/j.ijid.2020.01.009
13. Lee PI, Hsueh PR. Emerging threats from zoonotic coronaviruses-from SARS and MERS to 2019-nCoV. J Microbiol Immunol Infect. (2020) 53:365–7. doi: 10.1016/j.jmii.2020.02.001
14. Lauer SA, Grantz KH, Bi Q, Jones FK, Zheng Q, Meredith HR, et al. The incubation period of coronavirus disease 2019 (COVID-19) from publicly reported confirmed cases: estimation and application. Ann Intern Med. (2020) 172:577–82. doi: 10.7326/M20-0504
15. Wang Y, Wang Y, Chen Y, Qin Q. Unique epidemiological and clinical features of the emerging 2019 novel coronavirus pneumonia (COVID-19) implicate special control measures. J Med Virol. (2020) 92:568–76. doi: 10.1002/jmv.25748
16. Liu Y, Gayle AA, Wilder-Smith A, Rocklöv J. The reproductive number of COVID-19 is higher compared to SARS coronavirus. J Travel Med. (2020) 27:taaa021. doi: 10.1093/jtm/taaa021
17. Wu JT, Leung K, Bushman M, Kishore N, Niehus R, de Salazar PM, et al. Estimating clinical severity of COVID-19 from the transmission dynamics in Wuhan, China. Nat Med. (2020) 26:506–10. doi: 10.1038/s41591-020-0822-7
18. Zhao S, Lin Q, Ran J, Musa SS, Yang G, Wang W, et al. Preliminary estimation of the basic reproduction number of novel coronavirus (2019-nCoV) in China, from 2019 to 2020: A data-driven analysis in the early phase of the outbreak. Int J Infect Dis. (2020) 92:214–7. doi: 10.1016/j.ijid.2020.01.050
19. Carissimo G, Xu W, Kwok I, Abdad MY, Chan Y-H, Fong S-W, et al. Whole blood immunophenotyping uncovers immature neutrophil-to-VD2 T-cell ratio as an early marker for severe COVID-19. Nat Commun. (2020) 11:5243. doi: 10.1038/s41467-020-19080-6
20. Gao M, Liu Y, Guo M, Wang Q, Wang Y, Fan J, et al. Regulatory CD4(+) and CD8(+) T cells are negatively correlated with CD4(+) /CD8(+) T cell ratios in patients acutely infected with SARS-CoV-2. J Leukoc Biol. (2020) 109:91–7. doi: 10.1002/JLB.5COVA0720-421RR
21. Guan J, Wei X, Qin S, Liu X, Jiang Y, Chen Y, et al. Continuous tracking of COVID-19 patients' immune status. Int Immunopharmacol. (2020) 89(Pt. A):107034. doi: 10.1016/j.intimp.2020.107034
22. Hue S, Beldi-Ferchiou A, Bendib I, Surenaud M, Fourati S, Frapard T, et al. Uncontrolled innate and impaired adaptive immune responses in patients with COVID-19 ARDS. Am J Respir Crit Care Med. (2020) 202:1509–19. doi: 10.1164/rccm.202005-1885OC
23. Liu J, Li S, Liu J, Liang B, Wang X, Wang H, et al. Longitudinal characteristics of lymphocyte responses and cytokine profiles in the peripheral blood of SARS-CoV-2 infected patients. EBioMedicine. (2020) 55:102763. doi: 10.1016/j.ebiom.2020.102763
24. Xu B, Fan CY, Wang AL, Zou YL, Yu YH, He C, et al. Suppressed T cell-mediated immunity in patients with COVID-19: a clinical retrospective study in Wuhan, China. J Infect. (2020) 81:e51–60. doi: 10.1016/j.jinf.2020.04.012
25. Zou L, Dai L, Zhang Y, Fu W, Gao Y, Zhang Z, et al. Clinical characteristics and risk factors for disease severity and death in patients with coronavirus disease 2019 in Wuhan, China. Front Med. (2020) 7:532. doi: 10.3389/fmed.2020.00532
26. Huang C, Wang Y, Li X, Ren L, Zhao J, Hu Y, et al. Clinical features of patients infected with 2019 novel coronavirus in Wuhan, China. Lancet. (2020) 395:497–506. doi: 10.1016/S0140-6736(20)30183-5
27. Li X, Liu Y, Li J, Sun L, Yang J, Xu F, et al. Immune characteristics distinguish patients with severe disease associated with SARS-CoV-2. Immunol Res. (2020) 68:398–404. doi: 10.1007/s12026-020-09156-2
28. Tang Y, Li Y, Sun J, Pan H, Yao F, Jiao X. Selection of an optimal combination panel to better triage COVID-19 hospitalized patients. J Inflamm Res. (2020) 13:773–87. doi: 10.2147/JIR.S273193
29. Gan J, Li J, Li S, Yang C. Leucocyte subsets effectively predict the clinical outcome of patients with COVID-19 pneumonia: a retrospective case-control study. Front Public Health. (2020) 8:299. doi: 10.3389/fpubh.2020.00299
30. Chi Y, Ge Y, Wu B, Zhang W, Wu T, Wen T, et al. Serum cytokine and chemokine profile in relation to the severity of coronavirus disease 2019 in China. J Infect Dis. (2020) 222:746–54. doi: 10.1093/infdis/jiaa363
31. Keddie S, Ziff O, Chou MKL, Taylor RL, Heslegrave A, Garr E, et al. Laboratory biomarkers associated with COVID-19 severity and management. Clin Immunol. (2020) 221:108614. doi: 10.1016/j.clim.2020.108614
32. Zhao Y, Qin L, Zhang P, Li K, Liang L, Sun J, et al. Longitudinal COVID-19 profiling associates IL-1RA and IL-10 with disease severity and RANTES with mild disease. JCI Insight. (2020) 5:e139834. doi: 10.1172/jci.insight.139834
33. Diao B, Wang C, Tan Y, Chen X, Liu Y, Ning L, et al. Reduction and functional exhaustion of T cells in patients with coronavirus disease 2019 (COVID-19). Front Immunol. (2020) 11:827. doi: 10.3389/fimmu.2020.00827
34. Moher D, Liberati A, Tetzlaff J, Altman DG, The PG. Preferred reporting items for systematic reviews and meta-analyses: the PRISMA statement. PLoS Med. (2009) 6:e1000097. doi: 10.1371/journal.pmed.1000097
35. Stroup DF, Berlin JA, Morton SC, Olkin I, Williamson GD, Rennie D, et al. Meta-analysis of observational studies in epidemiology: a proposal for reporting. Meta-analysis Of Observational Studies in Epidemiology (MOOSE) group. JAMA. (2000) 283:2008–12. doi: 10.1001/jama.283.15.2008
36. Alshammary H, Alshammary H. T-cell subsets and interleukin-10 levels are predictors of severity and mortality in COVID-19: a systematic review and metananalysis. PROSPERO (2020). Available online at: https://www.crd.york.ac.uk/prospero/display_record.php?ID=CRD42020218918
37. Richardson WS, Wilson MC, Nishikawa J, Hayward RS. The well-built clinical question: a key to evidence-based decisions. ACP J Club. (1995) 123:A12–3. doi: 10.7326/ACPJC-1995-123-3-A12
38. Saaiq M, Ashraf B. Modifying “Pico” question into “Picos” model for more robust and reproducible presentation of the methodology employed in a scientific study. World J Plast Surg. (2017) 6:390–2.
41. Cheng S, Augustin C, Bethel A, Gill D, Anzaroot S, Brun J, et al. Using machine learning to advance synthesis and use of conservation and environmental evidence. Conserv Biol. (2018) 32:762–4. doi: 10.1111/cobi.13117
43. Rohatgi A. WebPlotDigitizer. Web Based Tool to Extract Data From Plots, Images, Maps. Version 4.4 Released. Pacifica, CA (2020). Available online at: https://automeris.io/WebPlotDigitizer/
44. Peterson J, Welch V, Losos M, Tugwell P. The Newcastle-Ottawa Scale (NOS) for Assessing the Quality of Nonrandomised Studies in Meta-Analyses. Ottawa, ON: Ottawa Hospital Research Institute (2011).
45. Wan X, Wang W, Liu J, Tong T. Estimating the sample mean and standard deviation from the sample size, median, range and/or interquartile range. BMC Med Res Methodol. (2014) 14:135. doi: 10.1186/1471-2288-14-135
46. Higgins JPT, Chandler J, Cumpston M, Li T, Page M, Welch V, . (eds.). Cochrane Handbook for Systematic Reviews of Interventions Cochrane (2020). Available online at: www.training.cochrane.org/handbook
47. DerSimonian R, Laird N. Meta-analysis in clinical trials. Control Clin Trials. (1986) 7:177–88. doi: 10.1016/0197-2456(86)90046-2
49. Lakens D. Calculating and reporting effect sizes to facilitate cumulative science: a practical primer for t-tests and ANOVAs. Front Psychol. (2013) 4:863. doi: 10.3389/fpsyg.2013.00863
51. Higgins JP, Thompson SG. Quantifying heterogeneity in a meta-analysis. Stat Med. (2002) 21:1539–58. doi: 10.1002/sim.1186
52. Higgins JP, Thompson SG, Deeks JJ, Altman DG. Measuring inconsistency in meta-analyses. BMJ. (2003) 327:557–60. doi: 10.1136/bmj.327.7414.557
53. Galbraith RF. A note on graphical presentation of estimated odds ratios from several clinical trials. Stat Med. (1988) 7:889–94. doi: 10.1002/sim.4780070807
54. Berkey CS, Hoaglin DC, Mosteller F, Colditz GA. A random-effects regression model for meta-analysis. Stat Med. (1995) 14:395–411. doi: 10.1002/sim.4780140406
55. Thompson SG, Higgins JP. How should meta-regression analyses be undertaken and interpreted? Stat Med. (2002) 21:1559–73. doi: 10.1002/sim.1187
56. Thompson SG, Sharp SJ. Explaining heterogeneity in meta-analysis: a comparison of methods. Stat Med. (1999) 18:2693–708.
57. Light RJ, Pillemer DB. Summing Up: The Science of Reviewing Research Harvard University Press: Cambridge, MA. (1984) xiii+191 pp. Educ Res. (1986) 15:16–7.
58. Sterne JAC, Becker BJ, Egger M. The Funnel Plot. Publication Bias in Meta-Analysis. New York, NY: Wiley (2005). p. 73–98.
59. Duval S, Tweedie R. Trim and fill: a simple funnel-plot-based method of testing and adjusting for publication bias in meta-analysis. Biometrics. (2000) 56:455–63. doi: 10.1111/j.0006-341X.2000.00455.x
60. Duval S, Tweedie R. A nonparametric “Trim and Fill” method of accounting for publication bias in meta-analysis. J Am Stat Assoc. (2000) 95:89–98. doi: 10.1080/01621459.2000.10473905
61. Lin L, Chu H. Quantifying publication bias in meta-analysis. Biometrics. (2018) 74:785–94. doi: 10.1111/biom.12817
62. Egger M, Davey Smith G, Schneider M, Minder C. Bias in meta-analysis detected by a simple, graphical test. BMJ. (1997) 315:629–34. doi: 10.1136/bmj.315.7109.629
63. Begg CB, Mazumdar M. Operating characteristics of a rank correlation test for publication bias. Biometrics. (1994) 50:1088–101. doi: 10.2307/2533446
64. Harrer M, Cuijpers P, Furukawa T, Ebert D. Doing Meta-Analysis with R: A Hands-on Guide. Boca Raton, FL; London: Chapman & Hall/CRC Press (2019).
65. Abers MS, Delmonte OM, Ricotta EE, Fintzi J, Fink DL, de Jesus AAA, et al. An immune-based biomarker signature is associated with mortality in COVID-19 patients. JCI Insight. (2021) 6:e144455. doi: 10.1172/jci.insight.144455
66. Azmy V, Kaman K, Tang D, Zhao H, Dela Cruz C, Topal JE, et al. Cytokine profiles before and after immune modulation in hospitalized patients with COVID-19. J Clin Immunol. (2021) 41:738–47. doi: 10.1007/s10875-020-00949-6
67. Cantenys-Molina S, Fernández-Cruz E, Francos P, Lopez Bernaldo de Quirós JC, Muñoz P, Gil-Herrera J. Lymphocyte subsets early predict mortality in a large series of hospitalized COVID-19 patients in Spain. Clin Exp Immunol. (2021) 203:424–32. doi: 10.1111/cei.13547
68. Chen J, Han T, Huang M, Yang Y, Shang F, Zheng Y, et al. Clinical characteristics of asymptomatic carriers of novel coronavirus disease 2019: a multi-center study in Jiangsu Province. Virulence. (2020) 11:1557–68. doi: 10.1080/21505594.2020.1840122
69. Deng F, Zhang L, Lyu L, Lu Z, Gao D, Ma X, et al. Increased levels of ferritin on admission predicts intensive care unit mortality in patients with COVID-19. Med Clin. (2021) 156:324–31. doi: 10.1016/j.medcli.2020.11.030
70. Flament H, Rouland M, Beaudoin L, Toubal A, Bertrand L, Lebourgeois S, et al. Outcome of SARS-CoV-2 infection is linked to MAIT cell activation and cytotoxicity. Nat Immunol. (2021) 22:322–35. doi: 10.1038/s41590-021-00870-z
71. Gadotti AC, de Castro Deus M, Telles JP, Wind R, Goes M, Garcia Charello Ossoski R, et al. IFN-gamma is an independent risk factor associated with mortality in patients with moderate and severe COVID-19 infection. Virus Res. (2020) 289:198171. doi: 10.1016/j.virusres.2020.198171
72. Han H, Ma Q, Li C, Liu R, Zhao L, Wang W, et al. Profiling serum cytokines in COVID-19 patients reveals IL-6 and IL-10 are disease severity predictors. Emerg Microbes Infect. (2020) 9:1123–30. doi: 10.1080/22221751.2020.1770129
73. He B, Wang J, Wang Y, Zhao J, Huang J, Tian Y, et al. The metabolic changes and immune profiles in patients with COVID-19. Front Immunol. (2020) 11:2075. doi: 10.3389/fimmu.2020.02075
74. He S, Zhou C, Lu D, Yang H, Xu H, Wu G, et al. Relationship between chest CT manifestations and immune response in COVID-19 patients. Int J Infect Dis. (2020) 98:125–9. doi: 10.1016/j.ijid.2020.06.059
75. Henry BM, Benoit SW, Vikse J, Berger BA, Pulvino C, Hoehn J, et al. The anti-inflammatory cytokine response characterized by elevated interleukin-10 is a stronger predictor of severe disease and poor outcomes than the pro-inflammatory cytokine response in coronavirus disease 2019 (COVID-19). Clin Chem Lab Med. (2021) 59:599–607. doi: 10.1515/cclm-2020-1284
76. Huang H, Zhang M, Chen C, Zhang H, Wei Y, Tian J, et al. Clinical characteristics of COVID-19 in patients with preexisting ILD: a retrospective study in a single center in Wuhan, China. J Med Virol. (2020) 92:2742–50. doi: 10.1002/jmv.26174
77. Huang W, Li M, Luo G, Wu X, Su B, Zhao L, et al. The inflammatory factors associated with disease severity to predict COVID-19 progression. J Immunol. (2021) 206:1597–608. doi: 10.4049/jimmunol.2001327
78. Jin XH, Zhou HL, Chen LL, Wang GF, Han QY, Zhang JG, et al. Peripheral immunological features of COVID-19 patients in Taizhou, China: a retrospective study. Clin Immunol. (2021) 222:108642. doi: 10.1016/j.clim.2020.108642
79. Kwon JS, Kim JY, Kim MC, Park SY, Kim BN, Bae S, et al. Factors of severity in patients with COVID-19: cytokine/chemokine concentrations, viral load, and antibody responses. Am J Trop Med Hyg. (2020) 103:2412–18. doi: 10.4269/ajtmh.20-1110
80. Laing AG, Lorenc A, Del Molino Del Barrio I, Das A, Fish M, Monin L, et al. A dynamic COVID-19 immune signature includes associations with poor prognosis. Nat Med. (2020) 26:1623–35. doi: 10.1038/s41591-020-1038-6
81. Li C, Jiang J, Wang F, Zhou N, Veronese G, Moslehi JJ, et al. Longitudinal correlation of biomarkers of cardiac injury, inflammation, and coagulation to outcome in hospitalized COVID-19 patients. J Mol Cell Cardiol. (2020) 147:74–87. doi: 10.1016/j.yjmcc.2020.08.008
82. Li M, Guo W, Dong Y, Wang X, Dai D, Liu X, et al. Elevated exhaustion levels of NK and CD8(+) T cells as indicators for progression and prognosis of COVID-19 disease. Front Immunol. (2020) 11:580237. doi: 10.3389/fimmu.2020.580237
83. Li Q, Xu W, Li WX, Huang CL, Chen L. Dynamics of cytokines and lymphocyte subsets associated with the poor prognosis of severe COVID-19. Eur Rev Med Pharmacol Sci. (2020) 24:12536–44. doi: 10.26355/eurrev_202012_24051
84. Liao B, Liu Z, Tang L, Li L, Gan Q, Shi H, et al. Longitudinal clinical and radiographic evaluation reveals interleukin-6 as an indicator of persistent pulmonary injury in COVID-19. Int J Med Sci. (2021) 18:29–41. doi: 10.7150/ijms.49728
85. Liu F, Ji C, Luo J, Wu W, Zhang J, Zhong Z, et al. Clinical characteristics and corticosteroids application of different clinical types in patients with corona virus disease 2019. Sci Rep. (2020) 10:13689. doi: 10.1038/s41598-020-70387-2
86. Liu J, Dong YQ, Yin J, He G, Wu X, Li J, et al. Critically ill patients with COVID-19 with ECMO and artificial liver plasma exchange: a retrospective study. Medicine. (2020) 99:e21012. doi: 10.1097/MD.0000000000021012
87. Liu L, Zheng Y, Cai L, Wu W, Tang S, Ding Y, et al. Neutrophil-to-lymphocyte ratio, a critical predictor for assessment of disease severity in patients with COVID-19. Int J Lab Hematol. (2020) 43:329–35. doi: 10.1111/ijlh.13374
88. Liu X-Q, Xue S, Xu J-B, Ge H, Mao Q, Xu X-H, et al. Clinical characteristics and related risk factors of disease severity in 101 COVID-19 patients hospitalized in Wuhan, China. Acta Pharmacol Sin. (2021) 43:64–75. doi: 10.1038/s41401-021-00627-2
89. Liu Y, Tan W, Chen H, Zhu Y, Wan L, Jiang K, et al. Dynamic changes in lymphocyte subsets and parallel cytokine levels in patients with severe and critical COVID-19. BMC Infect Dis. (2021) 21:79. doi: 10.1186/s12879-021-05792-7
90. Luo M, Liu J, Jiang W, Yue S, Liu H, Wei S. IL-6 and CD8+ T cell counts combined are an early predictor of in-hospital mortality of patients with COVID-19. JCI Insight. (2020) 5:e139024. doi: 10.1172/jci.insight.139024
91. Mann ER, Menon M, Knight SB, Konkel JE, Jagger C, Shaw TN, et al. Longitudinal immune profiling reveals key myeloid signatures associated with COVID-19. Sci Immunol. (2020) 5:eabd6197. doi: 10.1126/sciimmunol.abd6197
92. McElvaney OJ, McEvoy NL, McElvaney OF, Carroll TP, Murphy MP, Dunlea DM, et al. Characterization of the inflammatory response to severe COVID-19 illness. Am J Respir Crit Care Med. (2020) 202:812–21. doi: 10.1164/rccm.202005-1583OC
93. Rendeiro AF, Casano J, Vorkas CK, Singh H, Morales A, DeSimone RA, et al. Profiling of immune dysfunction in COVID-19 patients allows early prediction of disease progression. Life Sci Alliance. (2021) 4:e202000955. doi: 10.26508/lsa.202000955
94. Schrijver B, Assmann J, van Gammeren AJ, Vermeulen RCH, Portengen L, Heukels P, et al. Extensive longitudinal immune profiling reveals sustained innate immune activation in COVID-19 patients with unfavorable outcome. Eur Cytok Netw. (2020) 31:154–67. doi: 10.1684/ecn.2020.0456
95. Shi H, Wang W, Yin J, Ouyang Y, Pang L, Feng Y, et al. The inhibition of IL-2/IL-2R gives rise to CD8(+) T cell and lymphocyte decrease through JAK1-STAT5 in critical patients with COVID-19 pneumonia. Cell Death Dis. (2020) 11:429. doi: 10.1038/s41419-020-2636-4
96. Tan M, Liu Y, Zhou R, Deng X, Li F, Liang K, et al. Immunopathological characteristics of coronavirus disease 2019 cases in Guangzhou, China. Immunology. (2020) 160:261–8. doi: 10.1111/imm.13223
97. Wang Z, Yang B, Li Q, Wen L, Zhang R. Clinical features of 69 cases with coronavirus disease 2019 in Wuhan, China. Clin Infect Dis. (2020) 71:769–77. doi: 10.1093/cid/ciaa272
98. Yang A-P, Li H-M, Tao W-Q, Yang X-J, Wang M, Yang W-J, et al. Infection with SARS-CoV-2 causes abnormal laboratory results of multiple organs in patients. Aging. (2020) 12:10059–69. doi: 10.18632/aging.103255
99. Yang F, Shi S, Zhu J, Shi J, Dai K, Chen X. Clinical characteristics and outcomes of cancer patients with COVID-19. J Med Virol. (2020) 92:2067–73. doi: 10.1002/jmv.25972
100. Yi P, Yang X, Ding C, Chen Y, Xu K, Ni Q, et al. Risk factors and clinical features of deterioration in COVID-19 patients in Zhejiang, China: a single-centre, retrospective study. BMC Infect Dis. (2020) 20:943. doi: 10.1186/s12879-020-05682-4
101. Zeng HL, Lu QB, Yang Q, Wang X, Yue DY, Zhang LK, et al. Longitudinal profile of laboratory parameters and their application in the prediction for fatal outcome among patients infected with SARS-CoV-2: a retrospective cohort study. Clin Infect Dis. (2020) 72:626–33. doi: 10.1093/cid/ciaa574
102. Zeng Z, Yu H, Chen H, Qi W, Chen L, Chen G, et al. Longitudinal changes of inflammatory parameters and their correlation with disease severity and outcomes in patients with COVID-19 from Wuhan, China. Crit Care. (2020) 24:525. doi: 10.1186/s13054-020-03255-0
103. Zhang B, Yue D, Wang Y, Wang F, Wu S, Hou H. The dynamics of immune response in COVID-19 patients with different illness severity. J Med Virol. (2021) 93:1070–7. doi: 10.1002/jmv.26504
104. Zhang J, Yu M, Tong S, Liu LY, Tang LV. Predictive factors for disease progression in hospitalized patients with coronavirus disease 2019 in Wuhan, China. J Clin Virol. (2020) 127:104392. doi: 10.1016/j.jcv.2020.104392
105. Feng X, Li P, Ma L, Liang H, Lei J, Li W, et al. Clinical characteristics and short-term outcomes of severe patients with COVID-19 in Wuhan, China. Front Med. (2020) 7:491. doi: 10.3389/fmed.2020.00491
106. Durand B. (Khartis): Interim Administrator of the Institut d'études Politiques de Paris the Fondation Nationale des Sciences Politiques. (2021). Available online at: https://www.sciencespo.fr/cartographie/khartis/en/#home (accessed March 15, 2022).
107. Cui J, Li F, Shi ZL. Origin and evolution of pathogenic coronaviruses. Nat Rev Microbiol. (2019) 17:181–92. doi: 10.1038/s41579-018-0118-9
108. Woo PC, Lau SK, Yip CC, Huang Y, Tsoi HW, Chan KH, et al. Comparative analysis of 22 coronavirus HKU1 genomes reveals a novel genotype and evidence of natural recombination in coronavirus HKU1. J Virol. (2006) 80:7136–45. doi: 10.1128/JVI.00509-06
109. Islam MR, Hoque MN, Rahman MS, Alam ASMRU, Akther M, Puspo JA, et al. Genome-wide analysis of SARS-CoV-2 virus strains circulating worldwide implicates heterogeneity. Sci Rep. (2020) 10:14004. doi: 10.1038/s41598-020-70812-6
110. Lippi G, Plebani M. Laboratory abnormalities in patients with COVID-2019 infection. Clin Chem Lab Med. (2020) 58:1131–4. doi: 10.1515/cclm-2020-0198
111. Xu Z, Shi L, Wang Y, Zhang J, Huang L, Zhang C, et al. Pathological findings of COVID-19 associated with acute respiratory distress syndrome. Lancet Respir Med. (2020) 8:420–2. doi: 10.1016/S2213-2600(20)30076-X
112. Zhong Y, Cao Y, Zhong X, Peng Z, Jiang S, Tang T, et al. Immunity and coagulation/fibrinolytic processes may reduce the risk of severe illness in pregnant women with COVID-19. Am J Obstet Gynecol. (2020) 224:393.e1–25. doi: 10.1016/j.ajog.2020.10.032
113. Tan L, Wang Q, Zhang D, Ding J, Huang Q, Tang YQ, et al. Lymphopenia predicts disease severity of COVID-19: a descriptive and predictive study. Signal Transduct Target Ther. (2020) 5:33. doi: 10.1038/s41392-020-0159-1
114. Elshazli RM, Toraih EA, Elgaml A, El-Mowafy M, El-Mesery M, Amin MN, et al. Diagnostic and prognostic value of hematological and immunological markers in COVID-19 infection: a meta-analysis of 6320 patients. PLoS ONE. (2020) 15:e0238160. doi: 10.1371/journal.pone.0238160
115. Zhang Z, Ai G, Chen L, Liu S, Gong C, Zhu X, et al. Associations of immunological features with COVID-19 severity: a systematic review and meta-analysis. BMC Infect Dis. (2021) 21:738. doi: 10.1186/s12879-021-06457-1
116. Qin R, He L, Yang Z, Jia N, Chen R, Xie J, et al. Identification of parameters representative of immune dysfunction in patients with severe and fatal COVID-19 infection: a systematic review and meta-analysis. Clin Rev Allergy Immunol. (2022) 18:1–33. doi: 10.1007/s12016-021-08908-8
117. Mulchandani R, Lyngdoh T, Kakkar AK. Deciphering the COVID-19 cytokine storm: systematic review and meta-analysis. Eur J Clin Invest. (2021) 51:e13429. doi: 10.1111/eci.13429
118. Moutchia J, Pokharel P, Kerri A, McGaw K, Uchai S, Nji M, et al. Clinical laboratory parameters associated with severe or critical novel coronavirus disease 2019 (COVID-19): a systematic review and meta-analysis. PloS ONE. (2020) 15:e0239802. doi: 10.1371/journal.pone.0239802
119. Akbari H, Tabrizi R, Lankarani KB, Aria H, Vakili S, Asadian F, et al. The role of cytokine profile and lymphocyte subsets in the severity of coronavirus disease 2019 (COVID-19): a systematic review and meta-analysis. Life Sci. (2020) 258:118167. doi: 10.1016/j.lfs.2020.118167
120. Liu K, Yang T, Peng XF, Lv SM, Ye XL, Zhao TS, et al. A systematic meta-analysis of immune signatures in patients with COVID-19. Rev Med Virol. (2021) 31:e2195. doi: 10.1002/rmv.2195
121. Yonas E, Alwi I, Pranata R, Huang I, Lim MA, Yamin M, et al. Elevated interleukin levels are associated with higher severity and mortality in COVID 19 - a systematic review, meta-analysis, and meta-regression. Diabetes Metab Syndr. (2020) 14:2219–30. doi: 10.1016/j.dsx.2020.11.011
122. Rogers MC, Lamens KD, Shafagati N, Johnson M, Oury TD, Joyce S, et al. CD4(+) regulatory T cells exert differential functions during early and late stages of the immune response to respiratory viruses. J Immunol. (2018) 201:1253–66. doi: 10.4049/jimmunol.1800096
123. Mescher MF, Curtsinger JM, Agarwal P, Casey KA, Gerner M, Hammerbeck CD, et al. Signals required for programming effector and memory development by CD8+ T cells. Immunol Rev. (2006) 211:81–92. doi: 10.1111/j.0105-2896.2006.00382.x
124. Zhu J, Yamane H, Paul WE. Differentiation of effector CD4 T cell populations (*). Ann Rev Immunol. (2010) 28:445–89. doi: 10.1146/annurev-immunol-030409-101212
125. De Biasi S, Meschiari M, Gibellini L, Bellinazzi C, Borella R, Fidanza L, et al. Marked T cell activation, senescence, exhaustion and skewing towards TH17 in patients with COVID-19 pneumonia. Nat Commun. (2020) 11:3434. doi: 10.1038/s41467-020-17292-4
126. Zheng HY, Zhang M, Yang CX, Zhang N, Wang XC, Yang XP, et al. Elevated exhaustion levels and reduced functional diversity of T cells in peripheral blood may predict severe progression in COVID-19 patients. Cell Mol Immunol. (2020) 17:541–3. doi: 10.1038/s41423-020-0401-3
127. Alshammary AF, Al-Sulaiman AM. The journey of SARS-CoV-2 in Human Hosts: A Review of Immune Responses, Immunosuppression, and their Consequences. Virulence. (2021) 12:1771–94. doi: 10.1080/21505594.2021.1929800
128. Tavakolpour S, Rakhshandehroo T, Wei EX, Rashidian M. Lymphopenia during the COVID-19 infection: what it shows and what can be learned. Immunol Lett. (2020) 225:31–2. doi: 10.1016/j.imlet.2020.06.013
129. Tang Y, Liu J, Zhang D, Xu Z, Ji J, Wen C. Cytokine storm in COVID-19: the current evidence and treatment strategies. Front Immunol. (2020) 11:1708. doi: 10.3389/fimmu.2020.01708
130. Bogdan C, Vodovotz Y, Nathan C. Macrophage deactivation by interleukin 10. J Exp Med. (1991) 174:1549–55. doi: 10.1084/jem.174.6.1549
131. de Waal Malefyt R, Haanen J, Spits H, Roncarolo MG, te Velde A, Figdor C, et al. Interleukin 10 (IL-10) and viral IL-10 strongly reduce antigen-specific human T cell proliferation by diminishing the antigen-presenting capacity of monocytes via downregulation of class II major histocompatibility complex expression. J Exp Med. (1991) 174:915–24. doi: 10.1084/jem.174.4.915
132. Udomsinprasert W, Jittikoon J, Sangroongruangsri S, Chaikledkaew U. Circulating levels of interleukin-6 and interleukin-10, but not tumor necrosis factor-alpha, as potential biomarkers of severity and mortality for COVID-19: systematic review with meta-analysis. J Clin Immunol. (2021) 41:11–22. doi: 10.1007/s10875-020-00899-z
133. Zawawi A, Naser AY, Alwafi H, Minshawi F. Profile of circulatory cytokines and chemokines in human coronaviruses: a systematic review and meta-analysis. Front Immunol. (2021) 12:666223. doi: 10.3389/fimmu.2021.666223
134. Moore KW, de Waal Malefyt R, Coffman RL, O'Garra A. Interleukin-10 and the interleukin-10 receptor. Annu Rev Immunol. (2001) 19:683–765. doi: 10.1146/annurev.immunol.19.1.683
135. Fiorentino DF, Zlotnik A, Mosmann TR, Howard M, O'Garra A. IL-10 inhibits cytokine production by activated macrophages. J Immunol. (1991) 147:3815–22.
Keywords: COVID-19, SARS-CoV-2, coronavirus, interleukin 10, CD4, CD8, IL-10
Citation: Alshammary AF, Alsughayyir JM, Alharbi KK, Al-Sulaiman AM, Alshammary HF and Alshammary HF (2022) T-Cell Subsets and Interleukin-10 Levels Are Predictors of Severity and Mortality in COVID-19: A Systematic Review and Meta-Analysis. Front. Med. 9:852749. doi: 10.3389/fmed.2022.852749
Received: 11 January 2022; Accepted: 01 April 2022;
Published: 28 April 2022.
Edited by:
Pasquale Esposito, University of Genoa, ItalyReviewed by:
Carmen Silvia Valente Barbas, University of São Paulo, BrazilWang-Dong Xu, Southwest Medical University, China
Copyright © 2022 Alshammary, Alsughayyir, Alharbi, Al-Sulaiman, Alshammary and Alshammary. This is an open-access article distributed under the terms of the Creative Commons Attribution License (CC BY). The use, distribution or reproduction in other forums is permitted, provided the original author(s) and the copyright owner(s) are credited and that the original publication in this journal is cited, in accordance with accepted academic practice. No use, distribution or reproduction is permitted which does not comply with these terms.
*Correspondence: Amal F. Alshammary, YWFsc2hhbW1hcnlAa3N1LmVkdS5zYQ==