- 1Department of Hepatobiliary Oncology, Liver Cancer Center, Tianjin Medical University Cancer Institute and Hospital, National Clinical Research Center for Cancer, Key Laboratory of Cancer Prevention and Therapy, Tianjin's Clinical Research Center for Cancer, Tianjin Medical University, Tianjin, China
- 2Tianjin's Clinical Research Center for Cancer, Tianjin Medical University, Tianjin, China
- 3Department of Hepatology, Tianjin Third Central Hospital, Tianjin, China
- 4Department of Medicine, University of New South Wales, Sydney, NSW, Australia
- 5St George & Sutherland Clinical School, Microbiome Research Centre, University of New South Wales, Sydney, NSW, Australia
- 6Key Laboratory of Cancer Prevention and Therapy, Department of Gastrointestinal Cancer Biology, Liver Cancer Center, National Clinical Research Center for Cancer, Tianjin Medical University Cancer Institute and Hospital, Tianjin Medical University, Tianjin, China
Background: The association between gut microbiota and microvascular invasion (MVI) in patients with hepatocellular carcinoma (HCC) remains unclarified. Hence, the microbiome analysis of patients with HCC might predict MVI development as an accurate, non-invasive, and convenient assessment. The aim of this study was to investigate the characteristics of gut microbiota in patients with HCC-MVI and establish a microbial prediction model of HCC-MVI based on a microbiome study.
Methods: Fecal samples were collected from 59 patients with HCC (24 of the total with MVI disease and 16 healthy controls) and were further analyzed by 16S rRNA amplicon sequencing followed by a comprehensive bioinformatic analysis. The diagnostic performance of microbiome characteristics in predicting MVI was assessed by receiver operating characteristic (ROC) curves. The correlation between gut microbiota and tumor microenvironment (TME) in the HCC-MVI group was further analyzed by using immunohistochemistry and immunofluorescence assay.
Results: A significant differentiation trend of microbiota composition and structure was observed between the HCC-MVI group and those without vascular invasion (HCC-NVI). Compared with HCC-NVI group and healthy controls, gut bacteria Klebsiella, Proteobacteria, Prevotellaceae, and Enterobacteriaceae were significantly enriched, whereas Firmicutes, Ruminococcus, and Monoglobaceae were significantly decreased in patients with HCC-MVI. Klebsiella was considered to be the key microbiome signature for patients with HCC-MVI. The area under the curve (AUC) of the established HCC-MVI microbial prediction model was 94.81% (95% CI: 87.63–100%). The percentage of M2-type tumor-associated macrophages (TAMs) was increased in the HCC-MVI group compared with the HCC-NVI group (p < 0.001). M2-type TAMs in TME were negatively correlated with Shannon and Simpson index of HCC-MVI gut microbiota (all p < 0.01). In addition, predicted KEGG pathways showed that the functional differences in the metabolic pathways of microbiota varied among the groups.
Conclusion: The results indicated that differences existed in the fecal microbiome of patients with HCC-MVI and healthy controls. The prediction model of HCC-MVI established with certain gut bacterial signatures may have the potential to predict HCC-MVI outcome, and the characteristics of the fecal microbiome in patients with HCC may be associated with TME, though future larger-cohort studies are required to validate this supposition.
Introduction
Liver cancer is considered to be the fifth leading cause of cancer-related death (1, 2). The risk of recurrence after resection is up to 70% after 5 years, and the 5-year survival rate is as low as 18% (3). Hepatocellular carcinoma (HCC) is the most prevalent form of liver cancer (4), and most patients with HCC have metastatic disease or vascular invasion at their initial diagnosis (5–7). HCC tumors are heterogeneous, and patients at the same disease stage may have very different treatment outcomes (1).
Vascular invasion, including microvascular invasion (MVI), is one of the most critical factors in determining long-term survival and tumor recurrence for patients with HCC (8, 9). MVI is defined as a histological feature that is based on postoperative pathology (10–14). Once MVI develops, the tumor invades the blood vessels, and the current postoperative diagnosis of MVI leaves not much time window for therapeutic intervention. Accurate preoperative tests to predict MVI are thus urgently needed to reduce recurrence and improve the survival of patients with HCC. However, no such related methods, especially in a non-invasive manner, established to date have adequate sensitivity, specificity, or reproducibility for this purpose (15).
More than 100 trillion gut microorganisms form a complex microbial community, namely microbiota, that has a major impact on human health (16). Accumulating evidence has suggested a link between the microbiome, the metagenome of microbiota, and liver diseases. It is estimated that gut microbiota could be associated with 15–20% of patients with HCC (17). The gut–liver axis refers to the bidirectional relationship between the gut with its microbiota and the liver, which enables the transport of gut-derived products including microbial metabolites directly to the liver, and the liver feedback route of bile and antibody secretion to the intestine (18, 19). Previously, interactions between the intestinal microbiome and liver diseases were also reported in several studies, which showed the predictive value of gut microbiome in tumor prognosis, the effect on chemotherapy, and immunotherapy responses (20–22). Gut microbiota can regulate corresponding miRNAs through impacting circRNA expression and play a role in microbiota-mediated cancer metastasis (23). Li et al. identified CTSK as a mediator between the dysbiosis of gut microbiota and CRC metastasis (24). Gut or mammary duct colonization with enterotoxigenic bacteria Bacteroides fragilis triggers epithelial hyperplasia and augments breast cancer growth and metastasis (25).
The underlying mechanism of MVI in patients with HCC which induces metastasis and recurrence is very complicated and remains largely unclear (26). To the best of our knowledge, the correlation between gut microbiota and HCC-MVI remains uninvestigated, despite numerous reports linking gut dysbiosis with HCC pathogenesis. We hereby hypothesized that gut microbiota has a potential influence on HCC-MVI development, which may give credit to its role in cancer metastasis. Therefore, in this clinical study, we wanted to further investigate the relationship between the gut microbiota and HCC-MVI to gain a better understanding of the underlying interaction. To validate the previous research and provide deeper insights into the mechanism of fecal microbiome affecting HCC patients' MVI development, the current study was intended to further apply 16S rRNA amplicon sequencing and microbiome analysis to investigate the differences in taxonomic and derived functional profiles in the fecal microbiota from patients with HCC and healthy controls, clarify the predictive role of a key microbial signature in HCC-MVI, and further demonstrate its relevance to host immunity.
Methods
Subject Recruitment and Sample Collection
In this study, we identified 123 patients between January 2020 and December 2020 who had an initial presentation of hepatitis B virus (HBV)-related HCC at the Department of Liver Cancer Center, Tianjin Medical University Cancer Institute and Hospital. HBV-related HCC was defined here as seropositive for hepatitis B surface antigen (HBsAg), non-hepatitis C, and non-alcohol-related liver disease. The initial diagnosis of primary HCC is determined by pathology. Macrovascular invasion (MaVI) diagnosis is based on imaging (CT/MRI). The diagnosis of MVI is based on the postoperative pathological diagnosis. Patients with HCC without vascular invasion are grouped as HCC-NVI. Gender- and age-matched healthy volunteers with no history of diseases were enroll (1) concomitant with other malignant tumors; (2) nonnative patients who have received antitumor therapy; (3) combined with chronic diseases such as hypertension, diabetes, and gastrointestinal diseases; (4) use or take antibiotics, proton pump inhibitors, probiotic products 1 month before sampling; (5) missing clinical parameters. This study was approved by the Medical Ethics Committee at the Tianjin Cancer Hospital and written informed consent was obtained from all participants.
DNA Isolation From Fecal Samples and 16S rRNA Amplicon Sequencing
Fecal specimens (10 ml) were thawed and centrifuged at 7,500 g, 4°C for 10 min. Total genomic DNA was extracted by DNA Extraction Kit (Tiangen, China) from fecal samples. The 16S rRNA genes of distinct regions (16S V3-V4) were amplified using specific primers with the barcode. The PCR reactions were performed by 0.2 μM of forward and reverse primers, 15 μl of Phusion® High-Fidelity PCR Master Mix (New England Biolabs, USA), and 10 ng of template DNA. PCR cycling was carried out by the following program: 98°C 1 min, 25 cycles of 98°C 15 s, 55°C 30 s, 72°C 30 s, and 72°C 5 min. After the PCR process, the products were detected by operating electrophoresis on a 2% agarose gel. Then, Qiagen Gel Extraction Kit (Qiagen, Germany) was used to purify the mixture PCR products. Sequencing libraries were produced by TruSeq® DNA PCR-Free Sample Preparation Kit (Illumina, USA) following the manufacturer's recommendations, and the index codes were added. At last, the library was sequenced on an Illumina NovaSeq platform and 250 bp paired-end reads were generated.
Immunohistochemical Staining
The HCC tissues were serially sectioned at a thickness of 3–5 mm. Then, the tissues were dewaxed, deparaffinized in xylene, and rehydrated. The samples were then boiled for 15 min in a microwave oven for the purpose of increasing antigen retrieval. Then, 3% hydrogen peroxidase was used to block the endogenous peroxidases for 30 min. The slides were incubated with primary antibody CD8+ (PeproTech, 67786-1, 1:8000) overnight. Biotin-free horseradish peroxidase-labeled polymer of the Envision Plus detection system (Dako, Denmark) was used in the process of detection. Double immunofluorescence staining was performed as previously described. Paraffin-embedded sections were incubated with the following primary antibodies: F4/80 (Santa Cruz Biotechnology, SC-365340, 1:500), CD206 (PeproTech, 18704-1-AP, 1:800), and CD86 (CST, 91882S, 1:100) at 4°C overnight. Then, the sections were incubated at 37°C with the matched fluorescently labeled secondary antibodies (1:500; Invitrogen; CA, USA) for 30 min.
Bioinformatics and Statistical Analyses
According to the QIIME (V1.9.1) quality control process, quality filtering of raw tags was conducted under specific filtering conditions for the purpose of obtaining the high-quality clean tag. Uparse software (Uparse v7.0.1001) was used for the analysis of the sequences. Similar sequences higher than 97% were defined by the same operational taxonomic units (OTUs). Silva Database (http://www.arb-silva.de/) based on the Mothur algorithm was applied to annotate taxonomic information of each representative sequence. All the indices were calculated with QIIME and displayed with R Software (Version 2.15.3). Cluster analysis was preceded by principal component analysis (PCA), which was used to reduce the dimension of the original variables by FactoMineR package and ggplot2 package. Principal coordinate analysis (PCoA) was displayed to get principal coordinates and visualized with the WGCNA package, stat packages, and ggplot2 package. To determine the enrichment in the assigned taxonomic and functional profiles, LEfSe analysis was performed. Taxonomic levels with LEfSe values higher than 4 at a p < 0.05 were statistically significant. Operating characteristic curves (receiving operational curve, ROC) were constructed, and then the area under the curve (AUC) was calculated to evaluate the discriminatory ability of the random forest model. General statistical analysis was performed in Prism v8.2.1 (GraphPad Software, Inc.), and one-way ANOVA with Tukey's multiple comparisons test was used to determine differences between the groups. Spearman's rank correlation was performed in R (v3.6.1) to analyze the relationships between the microbiome and disease status.
All p-values were corrected using Benjamini–Hochberg multiple test correction. p-values of < 0.05 were considered statistically significant.
Results
Baseline Clinical Characteristics of the Participants
Fecal samples were collected from a total of 75 subjects including 16 healthy controls (NC), 20 patients with HCC-NVI, 24 patients with HCC-MVI, and 15 patients with HCC-MaVI (Figure 1). The demographic and clinical data of the four groups of participants including 59 patients with HCC and 16 healthy controls were analyzed and displayed in Table 1. Based on the published clinical studies employing microbiome analyses of four or more groups, we performed sample size calculation using G*power software and estimated that at least 15 participants in each group would provide an estimated effect size of 0.405, which is adequate to detect clinically meaningful differences between the subgroups with a power of at least 80% with a two-tailed p-value of < 0.05. Comparisons of all four groups and three HCC groups demonstrated significant differences among the levels of total bilirubin (TBIL), which is regarded as an indicator for liver dysfunction (Supplementary Figure 1A) (1). Although there was no significant difference in alpha-fetoprotein (AFP) level among the three HCC groups, a statistically significant difference in AFP level among all the four groups was observed when healthy controls were taken into consideration (Supplementary Figure 1B). No significant difference in age, gender, BMI, and other available clinical parameters was elicited among these four groups.
Alpha and Beta Diversities of Fecal Microbiome
Richness and evenness analysis was performed in the fecal microbiome of the patients with HCC and healthy controls (NC) using the Shannon and Simpson index to display the alpha diversity (Figures 2A,B). No significant difference was detected. When compared with the NC or HCC-NVI group, the microbial alpha diversity within the HCC-VI (HCC-MVI or HCC-MaVI) group showed significant statistical differences (Figures 2C,D). However, the beta diversity in the patients with HCC and NC group exhibited significant differences under the multiple response permutation procedure (MRPP; A = 0.0291, P = 0.001). The result of MRPP in NC, HCC-MVI, and HCC-MaVI groups all showed significant differences (all A > 0, P < 0.001).
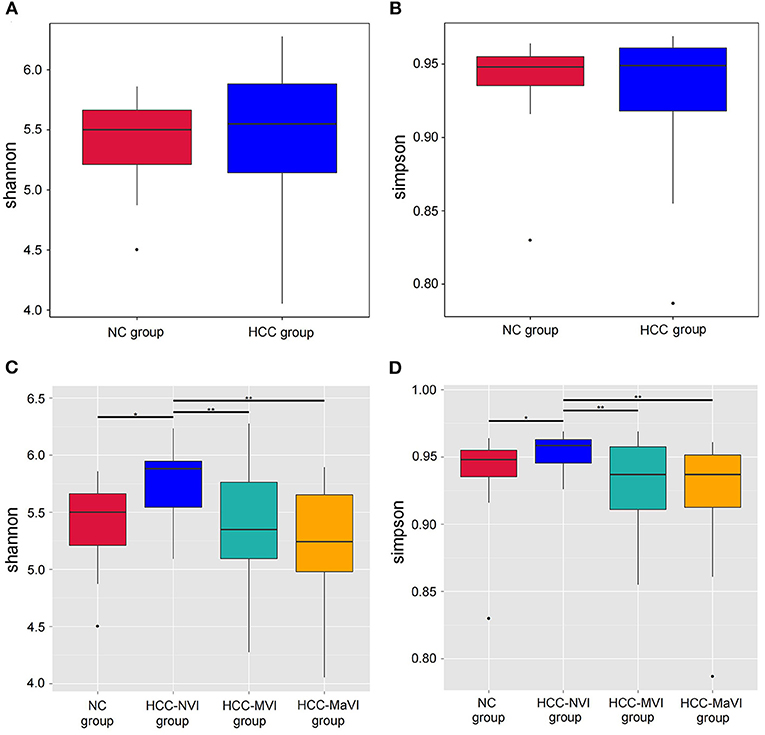
Figure 2. Comparison of alpha-diversity of the gut microbiota between patients with HCC and the NC group. Shannon (A) and Simpson (B) indexes were used to evaluate the evenness and richness in the HCC and NC groups; Shannon (C) and Simpson (D) indexes were used for comparing the evenness and richness in the NC, HCC-NVI, HCC-MVI, and HCC-MaVI groups. Difference in microbiota composition between the groups based on Bray-Curtis metrics was displayed by box-plot according to the Wilcoxon rank-sum test, *P < 0.05, **P < 0.01.
The PCA and unweighted Unifrac principal coordinate analysis (PCoA) analysis indicated that the NC and HCC groups had no clustering with members of their own group (Figures 3A,B). Subgroup analysis demonstrated that no identified microbiota clusters were observed in NC, HCC-NVI, HCC-MVI, and HCC-MaVI groups with PCA and unweighted Unifrac PCoA analysis (Figures 3C,D).
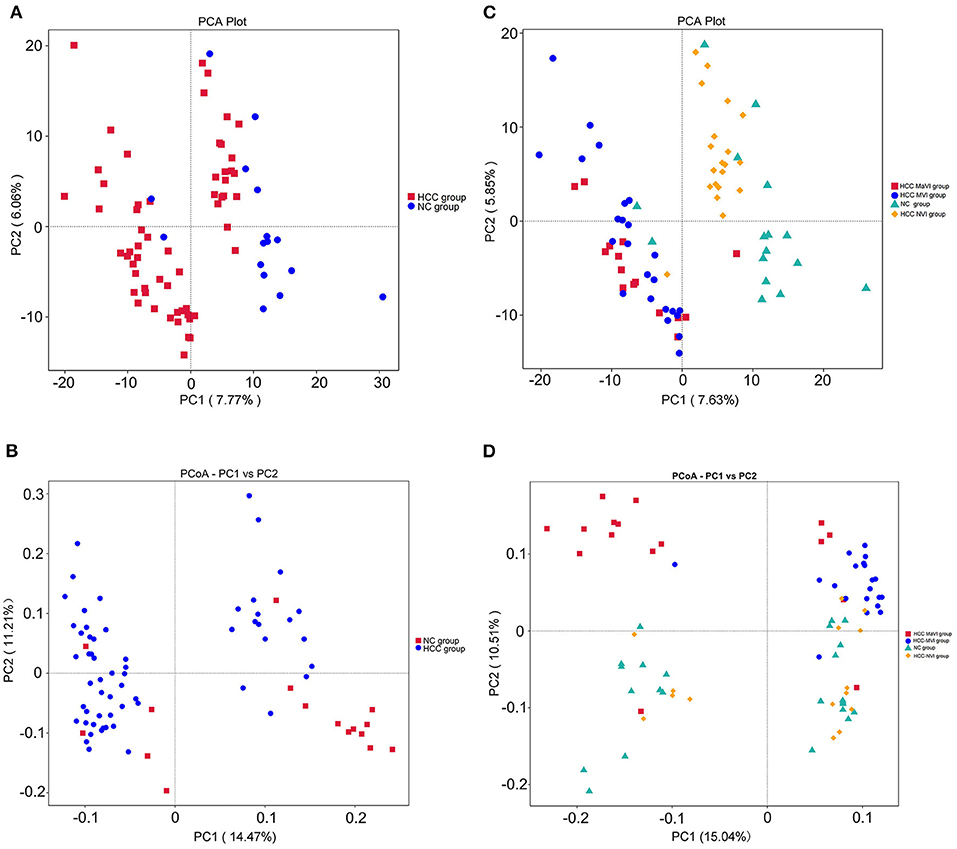
Figure 3. Comparison of beta diversity by principal component analysis (PCA) and principal coordinate analysis (PCoA) plots. PCA (A) and PCoA analysis of variation based on unweighted UniFrac distances (B) of NC and HCC groups. Red and blue dots represent HCC and normal controls, respectively. Each point represents an individual sample. PCA (C) and PCoA analysis of variation based on unweighted UniFrac distances (D) of NC, HCC-NVI, HCC-MVI, and HCC-MaVI groups. Red dots represent HCC-MaVI, blue dots represent HCC-MVI, yellow dots represent HCC-NVI, and green dots represent the NC group, respectively. Each point represents an individual sample.
Taxonomic Profiles of the Gut Microbiome
According to the relative abundance of the microbiota in the NC group, HCC samples, and their subgroups, classification and analysis were carried out at the levels of phylum, class, order, family, and genus. At the phylum level, the relative abundance of Firmicutes and Actinomycetes in the NC group was significantly greater than that of the HCC group (P = 0.004; 0.002), while the Bacteroidetes was significantly enriched in patients with HCC (P < 0.001; Figure 4A and Supplementary Figure 2). Firmicutes showed a significant decrease in the HCC-VI group when compared with the HCC-NVI group and the NC group. The relative abundance of Proteobacteria in the HCC-MVI group was greater than that of the HCC-NVI group and the NC group; the relative abundance of Bacteroides in the HCC-MaVI group was higher than that of the HCC-NVI group and the NC group, while the relative abundance of Actinobacteria was lower (Figure 4B and Supplementary Figure 3).
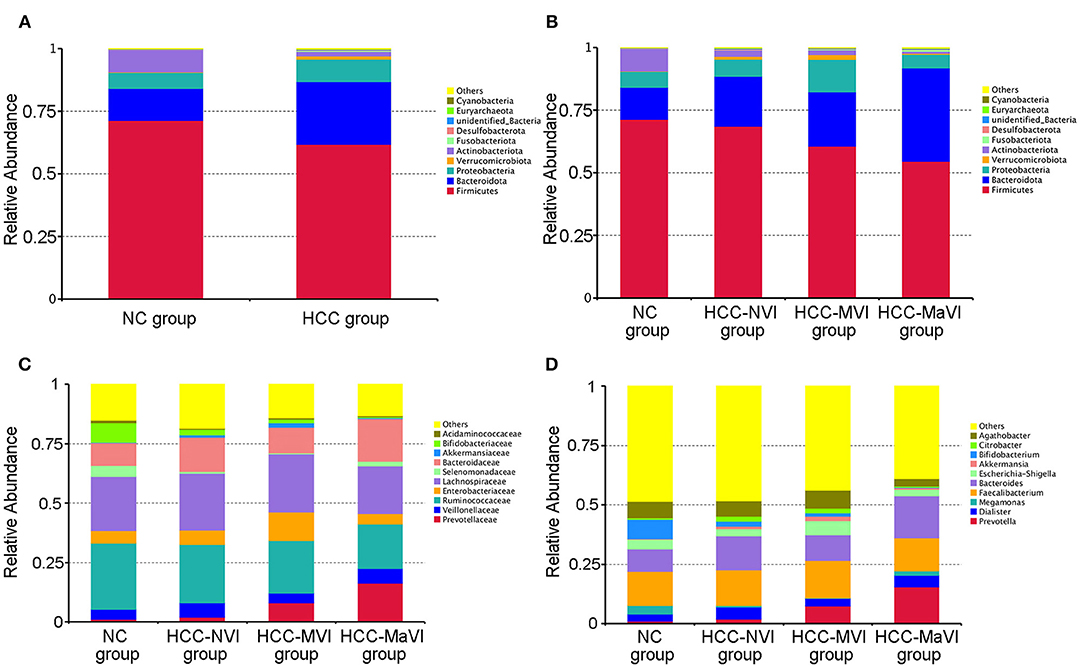
Figure 4. Relative abundance of microbiome across different groups. (A) The heatmaps of the NC and HCC bacteria groups at the phylum level. Heatmaps of the NC, HCC-NVI, HCC-MVI, and HCC-MaVI bacteria groups at the phylum level (B), at the family level (C), at the genus level (D).
Under the phylum level, the abundance of Bifidobacterium was decreased in patients with HCC (P = 0.002), while Prevotella and Klebsiella were increased compared with the NC group. Compared with the HCC-NVI group and the NC group, the abundance of Prevotellaceae and Enterobacteriaceae was significantly increased in the HCC-MVI group while that of Ruminococcaceae was decreased. The abundance of Ruminococcaceae, Bifidobacteriaceae, and Veillonellaceae was significantly decreased in the HCC-MaVI group while Bacteroidaceae showed a substantial increase (Figure 4C). Compared with the HCC-NVI group and the NC group, the abundance of Prevotella and Klebsiella was significantly increased in the HCC-MVI group. The abundance of Bifidobacterium significantly decreased and Prevotella significantly increased in the HCC-MaVI group (Figure 4D). Differential abundance of microbes at the class, order, family, and genus levels are displayed in Supplementary Figures 4–10. Briefly, Clostridia and Bacterodia exhibited higher abundance in HCC groups at the class level, Bacteroidales was significantly increased in HCC groups at the order level, the abundance of Prevotellaceae in HCC groups was significantly increased while Bifidobacteriaceae was significantly decreased at the family level, and Faecalibacterium showed the highest abundance in either NC group or HCC groups at the genus level.
Predicted Functional Profiles of the Gut Microbiome
In addition to diversity, the LEfSe analysis was applied to identify microbiome signatures that were differentially enriched among the NC, HCC-MVI, and HCC-MaVI groups. The result showed that genus_Agathobacter, genus Citrobacter, genus_Klebsiella, and species_Klebsiella_pneumoniae were significantly enriched in HCC-MVI samples. Accordingly, several microbes, such as Prevotellaceae, Bacteroidia, Prevotella, Bacteroidales, and Bacteroidota exhibit enrichment in patients with HCC-MaVI (p < 0.05, LDA score > 4; Figures 5A,B).
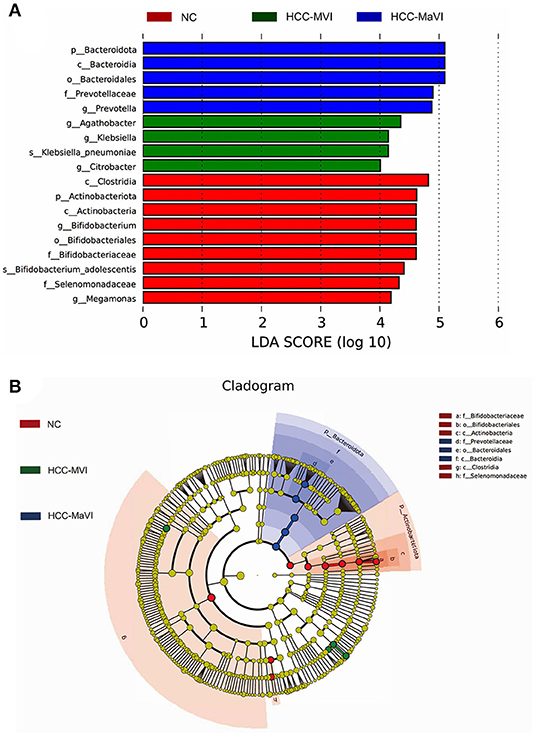
Figure 5. LEfSe analysis of gut microbiota. (A) LefSe analysis showed significant bacterial differences among NC, HCC-MVI, and HCC-MaVI groups; (B) A cladogram of different taxonomic compositions among NC, HCC-MVI, and HCC-MaVI groups.
The Potential Microbial Model Established for HCC-MVI Prediction
A random forest model was applied to predict HCC-MVI using 20 bacterial families (including Clostridiaceae, UCG_010, Tannerellaceae, Ruminococcaceae, Eubacterium coprostanoligenes group, Synergistaceae, Monoglobaceae, Rikenellaceae, unidentified Gastranaerophilales, Rhodobacteraceae, Dysgonomonadaceae, Bifidobacteriaceae, Burkholderiaceae, Enterobacteriaceae, Morganellaceae, Atopobiaceae, Marinifilaceae, Erysipelatoclostridiaceae, Bacteroidaceae, and Desulfovibrionaceae) with a significant difference among groups, which was filtered out based on mean decrease accuracy and mean decrease gin analysis. The receiver operating characteristic (ROC) analysis was performed to validate the prediction accuracy of this potential model for HCC-MVI with the calculated area under the curve (AUC) being 94.81% (95% CI: 87.63–100%), illustrating a good predictive performance of this model (Figure 6 and Supplementary Figure 11).
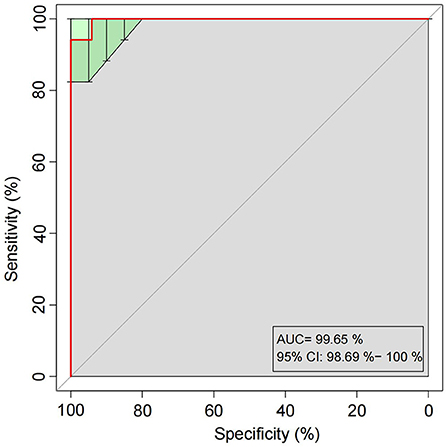
Figure 6. Receiver operating characteristic (ROC) curves built with twenty bacterial families for HCC-MVI prediction.
Gut Microbiota Associated With TME Changes in HCC-MVI Patients
Intratumoral interactions between the cellular and structural components of the tumor microenvironment (TME) can regulate cancer cell survival, local invasion, and metastatic dissemination (27). Immunohistochemical staining was thus applied to analyze the positive rate of CD8+ T cells in the TME group of liver cancer with or without MVI. Compared with the HCC-NVI group, the positive rate of CD8+ T cells in the HCC-MVI TME was significantly decreased (41.3 vs. 20.8% P = 0.001; Figures 7A,B).
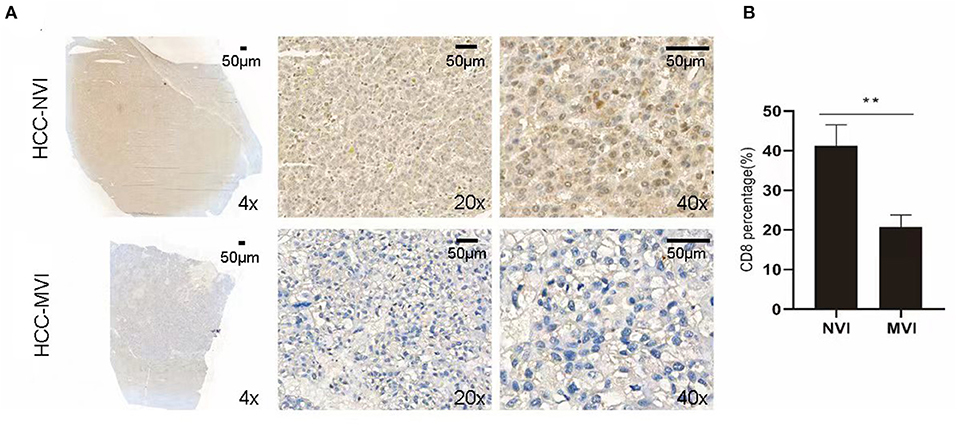
Figure 7. Immunohistochemical profiles of HCC. (A) Representative immunohistochemistry images reveal increased expression of CD8+ T cell in the HCC-MVI and HCC-NVI group (4 × , 20 × and 40 × magnification, respectively). Scale bar: 50 μm. (B) Percentage of CD8+ T cell in HCC-MVI and HCC-NVI groups, **P < 0.01 by t-test.
An immunofluorescence study was applied to further analyze M1 and M2 tumor-associated macrophage (TAM) cells in the TME of patients with HCC. The results indicate that compared with the HCC-NVI group, the positive rate of M1 TAM in the HCC-MVI group was significantly lower (32.5 vs. 54.0%, P < 0.001), whereas the positive rate of M2-type TAM cells in the HCC-MVI group was significantly increased (51.1 vs. 31.0%, P < 0.001; Figure 8).
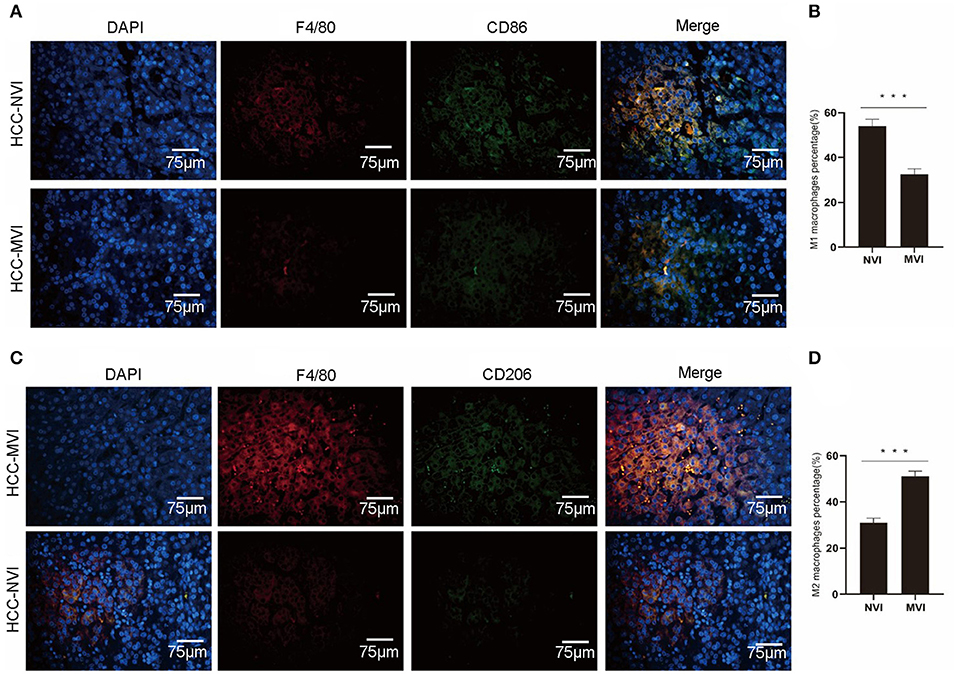
Figure 8. Immunofluorescence profiles of HCC. Representative image (A) and calculation (B) of M1 TAM positive rate in the HCC-MVI and HCC-NVI groups (40 × magnification). Representative image (C) and calculation (D) of the M2-type TAM positive rate in the HCC-MVI and HCC-NVI groups (40 × magnification). Scale bar: 75 μm, ***P < 0.001 by t-test.
Correlation Analysis Between the Intestinal Microbiome and TME Indicators
Cumulative contribution analysis (CCA) is used to explore the correlation among the positive rate of CD8+ T cells, M1- and M2-type TAM cells in TME, and the intestinal microbiome of different vascular invasions. As shown in Figure 9A, the cumulative contribution of TME indicators is 37.29% for CCA1 and 22.17% for CCA2, and the results indicate that there is a significant correlation between M2-type TAM and HCC-MVI group intestinal microbiome.
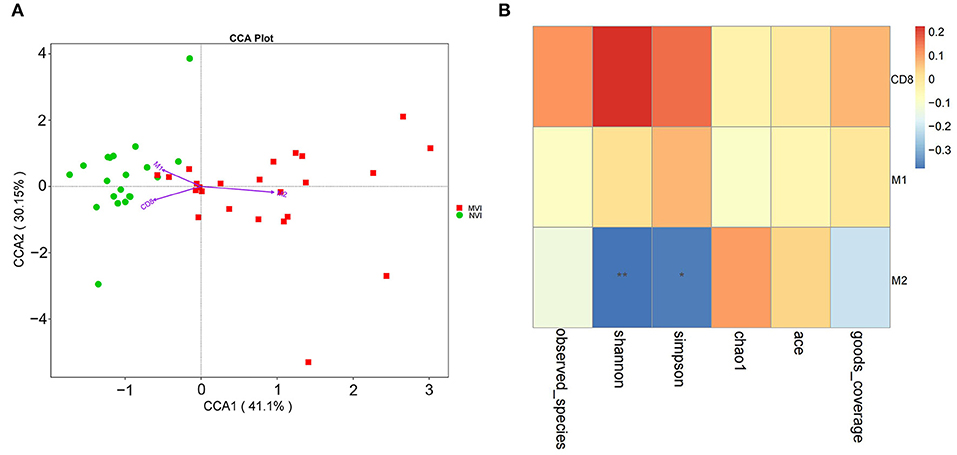
Figure 9. Correlation analysis of TME indicators and intestinal flora. (A) CCA analysis of intestinal flora and CD8+ T cells, M1 and M2-type TAM cells in HCC-MVI and HCC-NVI groups; (B) Spearman correlation analysis of CD8+ T cells, M1 and M2 type TAM cells in HCC-MVI group.
Based on Spearman correlation analysis, correlation among CD8+ T cells, M1/M2-type TAM cells, and HCC-MVI group intestinal microbiome was explored in TME. The positive rate of M2-type TAM cells is negatively correlated with the alpha diversity of the HCC-MVI intestinal microbiome (P < 0.01, Figure 9B). The positive rate of M2-type TAM in its TME increases once the diversity of the HCC-MVI intestinal microbiome decreases or the microbiome becomes disordered (dysbiosis).
Functional Analysis of HCC–MVI Microbiome and Its Correlation With M2-type TAM
Based on Tax4Fun, we further explored the functional alterations of the microbiome in the HCC-MVI and HCC-NVI groups (Supplementary Figure 12). The function of the HCC-MVI intestinal microbiome was significantly concentrated in pathways, including replication and repair, translation, energy metabolism, nucleotide metabolism, glycan biosynthesis, and metabolism, while the intestinal microbiome function of HCC-NVI patients was significantly enriched in pathways such as membrane transport, signal transduction, and cell motility (all p < 0.05). Based on the KEGG database, we further explored the correlation between the functional pathway of HCC-MVI microbiome and the positive rate of M2-type TAM in TME, and the mTOR signaling pathway was found to be positively correlated with a positive rate of M2-type TAM in TME (correlation coefficient 0.37, P = 0.01; Table 2). Taken together, these results suggest that the increase of TAM M2 positive rate in HCC-MVI TME may be involved in the mTOR signaling pathway.
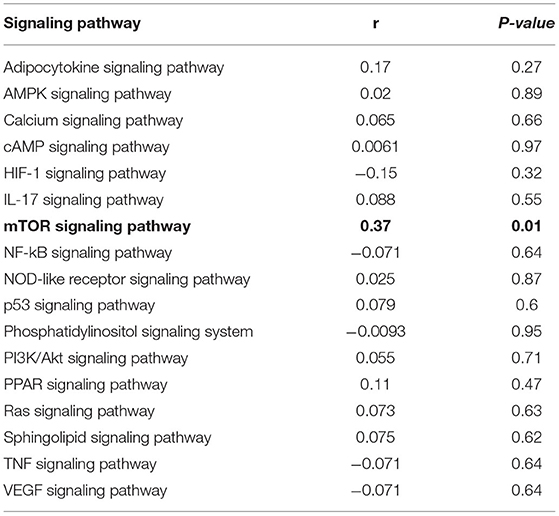
Table 2. The correlation between the functional pathway of microbiome and the positive rate of M2 type TAM in HCC-MVI group based on the KEGG database.
Discussion
In this study, we comprehensively explored the effects of the fecal microbiome on vascular invasion in patients with HCC, correlating them with clinical data and assessing whether a microbiome study can gain insight into a potential HCC-MVI prediction model. We showed that HCC-MVI significantly affects the relative abundance of several gut bacteria and that a microbial prediction model (based on 20 significant signature microbes) has the potential for predicting and discriminating high-risk patients with HCC-MVI. We also demonstrated that the characteristics of the fecal microbiome in patients with HCC may be associated with TME.
Several studies have shown that gut microbiota plays a crucial role in tumor prognosis and response to immunotherapy/chemotherapy (28). However, the effects of gut microbiota on MVI are largely unknown. As an important prognosis factor of HCC, current MVI assessment mainly depends on pathology following surgery. Therefore, preoperative prediction of MVI, especially in a non-invasive manner, is of great significance. Several studies have evaluated the performance of MVI predictive models constructed with clinical and molecular indicators, and the AUC of these models ranged from 0.73 to 0.81, suggesting that higher specificity and sensitivity are crucial for future studies (29–31). Intestinal microbiome analysis becomes an important direction for predictive model construction considering its convenience and non-invasive sample collecting requirements. Previously, Zheng et al. (32) screened out six important microbial markers at the genus level among hepatitis B virus (HBV)-related patients with HCC and healthy controls. They constructed predict models to explore the predictive value of intestinal microbiome on posthepatectomy recurrence and survival. The AUC of 2-year RFS survival predicted value was 68%, and the 5-year OS predicted AUC was 81%. Currently, there is no research that can predict HCC-MVI based on intestinal microbiome analysis.
In the present study, we performed comprehensive fecal microbiome analysis among the healthy controls (NC) and patients with HCC with different vascular invasion statuses (the HCC-NVI, HCC-MVI, and HCC-MaVI groups). The major microbiome component of healthy controls is found to be Firmicutes (with the percentage of 50–80%), followed by Bacteroides, Proteobacteria, Actinomycetes, and Verrucomicrobia. Our research indicated that the abundance of Bacteroides significantly enriched in patients with HCC while Firmicutes were reduced, which is consistent with previous studies (32–34). Compared with the HCC-NVI group and NC group, the abundances of Prevotella and Klebsiella were significantly increased in the HCC-MVI group. Prevotella was enriched in hepatitis C virus-related HCC and regarded as an independent prognostic biomarker in ESCC patients (30, 35). Prevotella's lipopolysaccharides (LPS) have a proinflammatory effect and its abundance is negatively correlated with interleukin (IL)-17A in colorectal cancer samples while the correlation with IL-9 is positive (36). Klebsiella has been shown to have increased abundance in patients with a variety of cancers including pancreatic cancer, colon cancer, and esophageal cancer (37–39). It was also related to the lymphatic metastasis and prognosis of pancreatic cancer (38). Klebsiella pneumoniae may promote chemoresistance to adjuvant gemcitabine treatment while following intervention with antibiotic quinolone can improve survival (40). Interestingly, our results indicated that Monoglobales, a member of microbiota which has not been reported in liver disease or cancer study, shows gradually decreased abundancy in the four subgroups according to differential vascular invasion status (highest in NC, then in HCC-NVI, HCC-MVI, and lowest in HCC-MaVI). Therefore, it may serve as a potential biomarker for negatively predicting HCC vascular invasion. However, with a limited study reported and finite evidence, the role of gut bacteria Monoglobales in HCC-MVI needs to be further explored.
As an emerging investigative field, microbiome study has some advantages over clinical or molecular indicators, especially when patients prefer non-invasive and convenient sample collection procedures. Wang et al. conducted a nomogram that identified four risk factors (tumor size, number of tumors, neutrophils, and serum α-fetoprotein) for MVI prediction (29). Circulating tumor DNA (ctDNA) was also identified in operable patients with HCC, suggesting that ctDNA may function as an independent risk factor for MVI and facilitate precise treatment strategies (41). Compared with aforesaid MVI prediction methods, the microbial model we constructed for HCC-MVI prediction obtained better performance with higher AUC (94.81%). The advent of high throughput next-generation sequencing (NGS) technologies has revolutionized microbiome study, especially when combined with advances in bioinformatics analysis and machine learning. Thus, the gut microbiome should be considered as a viable diagnostic and therapeutic target, which can be applied for assessing the clinical impact of these findings in future studies.
Currently, the mechanisms underlying the effects of microbiota on tumor prognosis remain unclear. Several clinical and subcutaneous preclinical studies revealed that gut microbiota was associated with systemic inflammation, including inflammatory responses in tumors (42–46). Our study revealed that the M2-type TAM positive rate was significantly higher in the HCC-MVI group than that of the HCC-NVI group. Moreover, once the diversity of the HCC-MVI microbiome decreases and the intestinal microbiome becomes disordered, the M2-type TAM positive rate in TME shows a further increase. These results are in accordance with previous studies which have shown that high expression of M2-type TAM in tumor tissue can promote tumor invasion and metastasis, resulting in poor prognosis (24, 47). The correlation between functional pathways and TME M2-type TAM was further explored, and the results suggest that the increase of M2-type TAM positive rate may be involved in the mammalian target of rapamycin (mTOR) pathway. The mTOR is frequently deregulated in tumorigenesis, activating somatic mutations of mTOR, which were recently identified in several types of cancer, and hence mTOR is therapeutically targeted (48).
There are certain limitations in the present study. First, some of the results obtained were achieved based on limited sample size and/or lack of further validation, therefore future validation in larger cohorts may reduce the false positive value and unreliability during HCC-MVI screening. Although the M2-type TAM and mTOR metabolic pathways are initially predicted to be involved based on the microbiome data, the related mechanism of their interaction needs to be further explored. However, the fact that these correlations were evident even in this limited sample size testifies to the potentialities of the proposed model. Interpreting changes was not the aim of our current study, thus we did not assess other clinical parameters such as intestinal permeability, inflammatory status, dietary habits, comorbidities, comedications, and portal hypertension, resulting in insufficient functional understanding of observed microbial changes. Moreover, the present study focused on the differences of gut microbiota resulting from MVI among HBV-related patients with HCC. Involving benign controls with HBV infection in subsequent larger cohort studies may help us refine the prediction model with less variables and higher AUC. Finally, the concomitant fecal metabolomic analysis would be a valuable addition to microbiome data for a more comprehensive understanding, especially on how the observed microbiota changes during MVI development.
However, strengths also are worthy of mention. As far as we know based on a literature search, this is the first clinical study exploring the influence of the gut microbiome on HCC-MVI development, and, as such, its results should be regarded with attention. In particular, we generated the first prediction model for HCC-MVI based on microbiome correlative analysis, which obtains high prediction performance. The microbial model already showed discriminative properties under the current experimental condition. It is likely that expanding the research among a larger cohort as well as other clinical parameters as mentioned above will further disclose the diagnostic potential of this method. Based on the microbiome data, the metabolic pathways that may be involved are initially predicted; however, the mechanism of their interaction needs to be further explored.
In conclusion, the present study highlights that gut microbiome alterations may have an influence on HCC-MVI prediction and relate to M2-type TAM of TME. Moreover, it shows that the microbial prediction model is able to efficiently predict HCC–MVI development. These preliminary results need to be confirmed and expanded in larger HCC cohorts and other cancer populations. The animal study is also needed to understand whether the differential microbiome affects the development of HCC-MVI by exploring possible mechanisms or the other way around. If confirmed, they might open the way to a quick and low-cost non-invasive diagnosis with many potential perspectives not only in hepatic vascular invasion diseases. Therefore, this clinical study provides a strong potential of translational implications from bench to bedside helping the clinicians in terms of disease prediction and diagnosis.
Data Availability Statement
The data presented in the study are deposited in the China National Microbiology Data Center, accession number NMDCX0000120 (https://nmdc.cn/resource/attachment/detail/NMDCX0000120).
Ethics Statement
The studies involving human participants were reviewed and approved by Tianjin Medical University Cancer Institute and Hospital. The patients/participants provided their written informed consent to participate in this study.
Author Contributions
WLu, EE-O, XZ, and NZ conceived the study. NZ, ZW, JL, and SZ recruited participants. ZW, JL, SW, and YL conducted the analysis. TL and WLi analyzed fecal samples. ZW, LG, and YL wrote the article. All authors revised and approved the written manuscript.
Funding
This research was supported by the Tianjin Science and Technology Program (No. 19YFZCSY00020).
Conflict of Interest
The authors declare that the research was conducted in the absence of any commercial or financial relationships that could be construed as a potential conflict of interest.
Publisher's Note
All claims expressed in this article are solely those of the authors and do not necessarily represent those of their affiliated organizations, or those of the publisher, the editors and the reviewers. Any product that may be evaluated in this article, or claim that may be made by its manufacturer, is not guaranteed or endorsed by the publisher.
Supplementary Material
The Supplementary Material for this article can be found online at: https://www.frontiersin.org/articles/10.3389/fmed.2022.836369/full#supplementary-material
References
1. Marrero JA, Kulik LM, Sirlin CB, Zhu AX, Finn RS, Abecassis MM, et al. Diagnosis, Staging, and Management of Hepatocellular Carcinoma: 2018 Practice Guidance by the American Association for the Study of Liver Diseases. Hepatology. (2018) 68:723–50. doi: 10.1002/hep.29913
2. Routy B, Le Chatelier E, Derosa L, Duong CPM, Alou MT, Daillere R, et al. Gut microbiome influences efficacy of PD-1-based immunotherapy against epithelial tumors. Science. (2018) 359:91–7. doi: 10.1126/science.aan3706
3. Craig AJ, von Felden J, Garcia-Lezana T, Sarcognato S, Villanueva A. Tumour evolution in hepatocellular carcinoma. Nat Rev Gastroenterol Hepatol. (2020) 17:139–52. doi: 10.1038/s41575-019-0229-4
4. Sayiner M, Golabi P, Younossi ZM. Disease burden of hepatocellular carcinoma: a global perspective. Dig Dis Sci. (2019) 64:910–7. doi: 10.1007/s10620-019-05537-2
5. Ringelhan M, McKeating JA, Protzer U. Viral hepatitis and liver cancer. Philos Trans R Soc Lond B Biol Sci. (2017) 372:20160274. doi: 10.1098/rstb.2016.0274
6. von Felden J, Karkmann K, Ittrich H, Gil-Ibanez I, Frundt T, Krause J, et al. Sequential Systemic Treatment in Advanced Hepatocellular Carcinoma Is Able to Prolong Median Survival to More than 3 Years in a Selected Real-World Cohort. Visc Med. (2021) 37:87–93. doi: 10.1159/000507381
7. Costentin CE, Ferrone CR, Arellano RS, Ganguli S, Hong TS, Zhu AX. Hepatocellular Carcinoma with Macrovascular Invasion: Defining the Optimal Treatment Strategy. Liver Cancer. (2017) 6:360–74. doi: 10.1159/000481315
8. Lee YH, Hsu CY, Huang YH, Su CW, Lin HC, Hsia CY, et al. alpha-fetoprotein-to-total tumor volume ratio predicts post-operative tumor recurrence in hepatocellular carcinoma. J Gastrointest Surg. (2013) 17:730–8. doi: 10.1007/s11605-012-2081-5
9. Rodriguez-Peralvarez M, Luong TV, Andreana L, Meyer T, Dhillon AP, Burroughs AK. A systematic review of microvascular invasion in hepatocellular carcinoma: diagnostic and prognostic variability. Ann Surg Oncol. (2013) 20:325–39. doi: 10.1245/s10434-012-2513-1
10. Sumie S, Kuromatsu R, Okuda K, Ando E, Takata A, Fukushima N, et al. Microvascular invasion in patients with hepatocellular carcinoma and its predictable clinicopathological factors. Ann Surg Oncol. (2008) 15:1375–82. doi: 10.1245/s10434-008-9846-9
11. Wang CC, Iyer SG, Low JK, Lin CY, Wang SH, Lu SN, et al. Perioperative factors affecting long-term outcomes of 473 consecutive patients undergoing hepatectomy for hepatocellular carcinoma. Ann Surg Oncol. (2009) 16:1832–42. doi: 10.1245/s10434-009-0448-y
12. Bhangui P, Vibert E, Majno P, Salloum C, Andreani P, Zocrato J, et al. Intention-to-treat analysis of liver transplantation for hepatocellular carcinoma: living versus deceased donor transplantation. Hepatology. (2011) 53:1570–9. doi: 10.1002/hep.24231
13. Mazzaferro V, Llovet JM, Miceli R, Bhoori S, Schiavo M, Mariani L, et al. Predicting survival after liver transplantation in patients with hepatocellular carcinoma beyond the Milan criteria: a retrospective, exploratory analysis. Lancet Oncol. (2009) 10:35–43. doi: 10.1016/S1470-2045(08)70284-5
14. Kudo A, Matsumura S, Ban D, Irie T, Ochiai T, Tanaka S, et al. Does the preoperative alpha-fetoprotein predict the recurrence and mortality after hepatectomy for hepatocellular carcinoma without macrovascular invasion in patients with normal liver function? Hepatol Res. (2014) 44:E437–46. doi: 10.1111/hepr.12335
15. Nitta H, Allard MA, Sebagh M, Ciacio O, Pittau G, Vibert E, et al. Prognostic Value and Prediction of Extratumoral Microvascular Invasion for Hepatocellular Carcinoma. Ann Surg Oncol. (2019) 26:2568–76. doi: 10.1245/s10434-019-07365-0
16. Guo Y, Kitamoto S, Kamada N. Microbial adaptation to the healthy and inflamed gut environments. Gut Microbes. (2020) 12:1857505. doi: 10.1080/19490976.2020.1857505
17. Bhatt AP, Redinbo MR, Bultman SJ. The role of the microbiome in cancer development and therapy. CA Cancer J Clin. (2017) 67:326–44. doi: 10.3322/caac.21398
18. Albillos A, de Gottardi A, Rescigno M. The gut-liver axis in liver disease: Pathophysiological basis for therapy. J Hepatol. (2020) 72:558–77. doi: 10.1016/j.jhep.2019.10.003
19. Tripathi A, Debelius J, Brenner DA, Karin M, Loomba R, Schnabl B, et al. The gut-liver axis and the intersection with the microbiome. Nat Rev Gastroenterol Hepatol. (2018) 15:397–411. doi: 10.1038/s41575-018-0011-z
20. Tzeng A, Sangwan N, Jia M, Liu CC, Keslar KS, Downs-Kelly E, et al. Human breast microbiome correlates with prognostic features and immunological signatures in breast cancer. Genome Med. (2021) 13:60. doi: 10.1186/s13073-021-00874-2
21. Zhao Z, Fei K, Bai H, Wang Z, Duan J, Wang J. Metagenome association study of the gut microbiome revealed biomarkers linked to chemotherapy outcomes in locally advanced and advanced lung cancer. Thorac Cancer. (2021) 12:66–78. doi: 10.1111/1759-7714.13711
22. Gopalakrishnan V, Spencer CN, Nezi L, Reuben A, Andrews MC, Karpinets TV, et al. Gut microbiome modulates response to anti-PD-1 immunotherapy in melanoma patients. Science. (2018) 359:97–103. doi: 10.1126/science.aan4236
23. Zhu Z, Huang J, Li X, Xing J, Chen Q, Liu R, et al. Gut microbiota regulate tumor metastasis via circRNA/miRNA networks. Gut Microbes. (2020) 12:1788891. doi: 10.1080/19490976.2020.1788891
24. Li R, Zhou R, Wang H, Li W, Pan M, Yao X, et al. Gut microbiota-stimulated cathepsin K secretion mediates TLR4-dependent M2 macrophage polarization and promotes tumor metastasis in colorectal cancer. Cell Death Differ. (2019) 26:2447–63. doi: 10.1038/s41418-019-0312-y
25. Parida S, Wu S, Siddharth S, Wang G, Muniraj N, Nagalingam A, et al. A Procarcinogenic Colon Microbe Promotes Breast Tumorigenesis and Metastatic Progression and Concomitantly Activates Notch and beta-Catenin Axes. Cancer Discov. (2021) 11:1138–57. doi: 10.1158/2159-8290.CD-20-0537
26. Yang M, Li J, Gu P, Fan X. The application of nanoparticles in cancer immunotherapy: Targeting tumor microenvironment. Bioact Mater. (2021) 6:1973–87. doi: 10.1016/j.bioactmat.2020.12.010
27. Kurebayashi Y, Ojima H, Tsujikawa H, Kubota N, Maehara J, Abe Y, et al. Landscape of immune microenvironment in hepatocellular carcinoma and its additional impact on histological and molecular classification. Hepatology. (2018) 68:1025–41. doi: 10.1002/hep.29904
28. Barko PC, McMichael MA, Swanson KS, Williams DA. The Gastrointestinal Microbiome: A Review. J Vet Intern Med. (2018) 32:9–25. doi: 10.1111/jvim.14875
29. Wang L, Jin YX, Ji YZ, Mu Y, Zhang SC, Pan SY. Development and validation of a prediction model for microvascular invasion in hepatocellular carcinoma. World J Gastroenterol. (2020) 26:1647–59. doi: 10.3748/wjg.v26.i14.1647
30. Lin S, Ye F, Rong W, Song Y, Wu F, Liu Y, et al. Nomogram to Assist in Surgical Plan for Hepatocellular Carcinoma: a Prediction Model for Microvascular Invasion. J Gastrointest Surg. (2019) 23:2372–82. doi: 10.1007/s11605-019-04140-0
31. Lee JC, Hung HC, Wang YC, Cheng CH, Wu TH, Lee CF, et al. Risk Score Model for Microvascular Invasion in Hepatocellular Carcinoma: The Role of Tumor Burden and Alpha-Fetoprotein. Cancers (Basel). (2021) 13:4403. doi: 10.3390/cancers13174403
32. Huang H, Ren Z, Gao X, Hu X, Zhou Y, Jiang J, et al. Integrated analysis of microbiome and host transcriptome reveals correlations between gut microbiota and clinical outcomes in HBV-related hepatocellular carcinoma. Genome Med. (2020) 12:102. doi: 10.1186/s13073-020-00796-5
33. Wu Y, Shi L, Li Q, Wu J, Peng W, Li H, et al. Microbiota Diversity in Human Colorectal Cancer Tissues Is Associated with Clinicopathological Features. Nutr Cancer. (2019) 71:214–22. doi: 10.1080/01635581.2019.1578394
34. Zhang L, Wu YN, Chen T, Ren CH, Li X, Liu GX. Relationship between intestinal microbial dysbiosis and primary liver cancer. Hepatobiliary Pancreat Dis Int. (2019) 18:149–57. doi: 10.1016/j.hbpd.2019.01.002
35. Simpson CA, Mu A, Haslam N, Schwartz OS, Simmons JG. Feeling down? A systematic review of the gut microbiota in anxiety/depression and irritable bowel syndrome. J Affect Disord. (2020) 266:429–46. doi: 10.1016/j.jad.2020.01.124
36. Niccolai E, Russo E, Baldi S, Ricci F, Nannini G, Pedone M, et al. Significant and Conflicting Correlation of IL-9 With Prevotella and Bacteroides in Human Colorectal Cancer. Front Immunol. (2020) 11:573158. doi: 10.3389/fimmu.2020.573158
37. Shen W, Tang D, Deng Y, Li H, Wang T, Wan P, et al. Association of gut microbiomes with lung and esophageal cancer: a pilot study. World J Microbiol Biotechnol. (2021) 37:128. doi: 10.1007/s11274-021-03086-3
38. Matsukawa H, Iida N, Kitamura K, Terashima T, Seishima J, Makino I, et al. Dysbiotic gut microbiota in pancreatic cancer patients form correlation networks with the oral microbiota and prognostic factors. Am J Cancer Res. (2021) 11:3163–75.
39. Cong J, Zhu H, Liu D, Li T, Zhang C, Zhu J, et al. A Pilot Study: Changes of Gut Microbiota in Post-surgery Colorectal Cancer Patients. Front Microbiol. (2018) 9:2777. doi: 10.3389/fmicb.2018.02777
40. Weniger M, Hank T, Qadan M, Ciprani D, Michelakos T, Niess H, et al. Influence of Klebsiella pneumoniae and quinolone treatment on prognosis in patients with pancreatic cancer. Br J Surg. (2021) 108:709–16. doi: 10.1002/bjs.12003
41. Wang D, Xu Y, Goldstein JB, Ye K, Hu X, Xiao L, et al. Preoperative evaluation of microvascular invasion with circulating tumour DNA in operable hepatocellular carcinoma. Liver Int. (2020) 40:1997–2007. doi: 10.1111/liv.14463
42. Iida N, Dzutsev A, Stewart CA, Smith L, Bouladoux N, Weingarten RA, et al. Commensal bacteria control cancer response to therapy by modulating the tumor microenvironment. Science. (2013) 342:967–70. doi: 10.1126/science.1240527
43. Villeger R, Lopes A, Veziant J, Gagniere J, Barnich N, Billard E, et al. Microbial markers in colorectal cancer detection and/or prognosis. World J Gastroenterol. (2018) 24:2327–47. doi: 10.3748/wjg.v24.i22.2327
44. Jarman R, Ribeiro-Milograna S, Kalle W. Potential of the Microbiome as a Biomarker for Early Diagnosis and Prognosis of Breast Cancer. J Breast Cancer. (2020) 23:579–87. doi: 10.4048/jbc.2020.23.e60
45. Derosa L, Routy B, Fidelle M, Iebba V, Alla L, Pasolli E, et al. Gut Bacteria Composition Drives Primary Resistance to Cancer Immunotherapy in Renal Cell Carcinoma Patients. Eur Urol. (2020) 78:195–206. doi: 10.1016/j.eururo.2020.04.044
46. Yi Y, Shen L, Shi W, Xia F, Zhang H, Wang Y, et al. Gut Microbiome Components Predict Response to Neoadjuvant Chemoradiotherapy in Patients with Locally Advanced Rectal Cancer: A Prospective, Longitudinal Study. Clin Cancer Res. (2021) 27:1329–40. doi: 10.1158/1078-0432.CCR-20-3445
47. Yuan H, Lin Z, Liu Y, Jiang Y, Liu K, Tu M, et al. Intrahepatic cholangiocarcinoma induced M2-polarized tumor-associated macrophages facilitate tumor growth and invasiveness. Cancer Cell Int. (2020) 20:586. doi: 10.1186/s12935-020-01687-w
Keywords: hepatocellular carcinoma, microvascular invasion, gut microbiota, predictive model, tumor microenvironment, M2-type TAM, mTOR signaling pathway
Citation: Zhang N, Wang Z, Lv J, Zhang S, Liu Y, Liu T, Li W, Gong L, Zhang X, El-Omar EM and Lu W (2022) Characterization of Gut Microbiota and Exploration of Potential Predictive Model for Hepatocellular Carcinoma Microvascular Invasion. Front. Med. 9:836369. doi: 10.3389/fmed.2022.836369
Received: 15 December 2021; Accepted: 09 February 2022;
Published: 17 March 2022.
Edited by:
Zhangran Chen, Xiamen University, ChinaReviewed by:
Lei Zhao, Tangdu Hospital, ChinaTao Zuo, Sun Yat-sen University, China
Kunkai Su, Zhejiang University, China
Copyright © 2022 Zhang, Wang, Lv, Zhang, Liu, Liu, Li, Gong, Zhang, El-Omar and Lu. This is an open-access article distributed under the terms of the Creative Commons Attribution License (CC BY). The use, distribution or reproduction in other forums is permitted, provided the original author(s) and the copyright owner(s) are credited and that the original publication in this journal is cited, in accordance with accepted academic practice. No use, distribution or reproduction is permitted which does not comply with these terms.
*Correspondence: Wei Lu, mail4luwei@163.com; Emad M. El-Omar, e.el-omar@unsw.edu.au; Xiaodong Zhang, zhangxiaodong@tjmuch.com
†These authors have contributed equally to this work