- 1Department of Orthopedics, Xianyang Central Hospital, Xianyang, China
- 2Clinical Medical Research Center, Xianyang Central Hospital, Xianyang, China
- 3Department of Respiratory and Critical Care Medicine, The First People’s Hospital of Chongqing Liang Jiang New Area, Chongqing, China
- 4Department of Orthopaedic Surgery, The First Affiliated Hospital of Nanchang University, Nanchang, China
- 5Department of Dermatology, Xianyang Central Hospital, Xianyang, China
- 6School of Physics and Technology, Wuhan University, Wuhan, China
- 7Department of Spine Surgery, Second Affiliated Hospital of Dalian Medical University, Dalian, China
- 8Department of Orthopaedics, The Second Hospital of Jilin University, Changchun, China
- 9The First Clinical Medical College, Shaanxi University of Traditional Chinese Medicine, Xianyang, China
- 10Department of Spinal Surgery, Liuzhou People’s Hospital, Liuzhou, China
- 11Department of Business Management, Xiamen Bank, Xiamen, China
- 12Faculty of Medicine, Macau University of Science and Technology, Macau, China
Objective: In order to provide reference for clinicians and bring convenience to clinical work, we seeked to develop and validate a risk prediction model for lymph node metastasis (LNM) of Ewing’s sarcoma (ES) based on machine learning (ML) algorithms.
Methods: Clinicopathological data of 923 ES patients from the Surveillance, Epidemiology, and End Results (SEER) database and 51 ES patients from multi-center external validation set were retrospectively collected. We applied ML algorithms to establish a risk prediction model. Model performance was checked using 10-fold cross-validation in the training set and receiver operating characteristic (ROC) curve analysis in external validation set. After determining the best model, a web-based calculator was made to promote the clinical application.
Results: LNM was confirmed or unable to evaluate in 13.86% (135 out of 974) ES patients. In multivariate logistic regression, race, T stage, M stage and lung metastases were independent predictors for LNM in ES. Six prediction models were established using random forest (RF), naive Bayes classifier (NBC), decision tree (DT), xgboost (XGB), gradient boosting machine (GBM), logistic regression (LR). In 10-fold cross-validation, the average area under curve (AUC) ranked from 0.705 to 0.764. In ROC curve analysis, AUC ranged from 0.612 to 0.727. The performance of the RF model ranked best. Accordingly, a web-based calculator was developed (https://share.streamlit.io/liuwencai2/es_lnm/main/es_lnm.py).
Conclusion: With the help of clinicopathological data, clinicians can better identify LNM in ES patients. Risk prediction models established in this study performed well, especially the RF model.
Introduction
Ewing’s sarcoma (ES) can be identified in bone and soft tissue (1). The main symptom of ES is local pain with or without paresthesia. Highly aggressive small round blue cell malignant neoplasm is a pathological feature of ES (2). 80% of ES patients are under the age of 20, with a male to female ratio of 1.5:1 (3). ES accounted for 3% of malignant tumors in children, which is the second most common malignant bone tumor in children (4).
ES has metastatic potentiality. It is a highly anaplastic, round cell tumor, primarily arising in the intramedullary portion of bone, poor prognosis and metastases are not uncommon. It is well established when imaging patients with primary bone malignancy that on occasions computed tomography (CT) scanning can offer unique information for the occurrence of metastasis. EWS-FLI1, a fusion protein identified in ES, played a key role in many transcription and translation processes, and may affect the initiation and progression of tumors (3). MMR pathway may be be associated with the proliferation, invasion and migration of ES tumor cells (2). There are some reports suggesting that the incidence of LNM in ES is higher than that in osteosarcoma and chondrosarcoma (5). Lungs are the most commonly involved part of the body (6).
Once ES has metastasized, the prognosis is poor. The location of ES is a very important factor in the prognosis. Even if the same tumor grows in different locations, the prognosis can vary greatly. In the past, multi-drug combination chemotherapy with surgery and radiotherapy increased its 5-year survival rate to 65–75% in a limited period of time. However, the 5-year survival rate in the metastatic period was usually less than 30% (3, 7, 8). The ability to accurately and non-invasively assess the risk of tumor metastasis has significant implications for treatment planning, post-operative follow-up and rehabilitation, precision medicine, and long-term public health policy (9–12).
LNM in ES is relatively uncommon. In addition to tumor stage, tumor size, primary location, age and treatment, regional lymph node involvement is a new independent adverse prognostic factor for ES (13). LNM can also contribute to risk stratification. The 5-year survival rate of ES decreased from 60.3 to 45.9% once LNM exist (5).
Predicting models of tumor LNM have been established in thyroid papillary carcinoma, bladder urothelial carcinoma and early esophageal squamous cell carcinoma (14–16). To our knowledge, there are no predicting models for LNM in ES. SEER database covers almost 30% population of the United States and is an major resource for the study of ES (17). Machine learning and medical big data have become a key step in the leap from evidence-based medicine to precision medicine, and there has been research, research, and research on a variety of diseases (18–21). More and more scholars are discovering the value of artificial intelligence and big data in medicine (12, 22, 23). Based on the data of ES in the SEER database, a model for predicting LNM was established by using the ML method and verified internally and externally.
Materials and Methods
Patients Population
By recruiting patients diagnosed with ES from 2010 to 2016 in the SEER database as a training set. Patients diagnosed with ES from 2010 to 2018 from the Second Affiliated Hospital of Jilin University, the Second Affiliated Hospital of Dalian Medical University, Liuzhou People’s Hospital, and Xianyang Central Hospital were included as the validation set. The inclusion criteria were listed as follows: (1) patients confirmed as primary ES with pathologically evidence; (2) ES patients with ICD-O-3/WHO 2008 morphology code 60; (3) clinicopathological information and survival time is complete. The exclusion criteria were listed as follows: (1) patients with other kinds of the primary tumor and unknown metastasis; (2) patients without available information such as clinicopathological and survival time. Given that the study was retrospective and the data came from an open database, informed consent was not used.
Data Collection
Index such as race, age, sex, primary site, laterality, T stage, M stage, surgery, radiation, chemotherapy, bone metastases, lung metastases and survival time were collected both in the training set and validation set. In the training set, data were extracted using SEER * STAT (8.3.5) software. In the validation set, data were obtained and processed by two investigators independently. If there were any objections, the third investigator would participate in judgment. Microsoft spreadsheet (Microsoft spreadsheet, 2013, Redmond, United States) was utilized to check the consistency of all data.
Statistical Methods
Continuous parameters following normal distribution were described by mean SD. Categorical parameters were presented as numerical values and proportions. Chi-square tests, Fisher’s exact tests, t-tests and logistic regression analysis were performed using R software (version 4.0.5). The difference was statistically significant when P < 0.05 with bilateral test. ML algorithms and web application were performed with the help of Python.
Demographic characteristics of the training set from SEER database and the validation set from multi-center were compared to identify the difference. We also divided the total study population into two sets based on the presence or absence of LNM and compared baseline information. To identify risk factors for LNM in patients with ES, we conducted univariate and multivariate logistic regression. Factors with P < 0.05 in the univariate logistic regression analysis were determined as variables for model establishment. RF, NBC, DT, XGB, GBM, and LR were performed in the training set to develop a prediction model. Analysis of relative importance ranking of each input variable was performed in each model. We adopted 10-fold cross-validation in the training set and ROC curve analysis in multi-center data to check the performance of each model. Define the model with the best performance based on the maximum AUC in internal and external validation. At last, a web-based calculator was appropriate to provide for clinical application of the final prediction model.
Results
Demographic Characteristics
A total of 974 ES patients were included in this study, of which 923 were from the SEER database and 51 were from the multi-center external validation set. Baseline data of the training set and the validation set were listed in Table 1. Race and radiation were two variables with P < 0.05. The main race was white race (81.8%) in the training set and other (yellow race, etc.) (100%) in the verification set. Radiation history accounted for 21.7% in the training set and 43.1% in the validation set. The differences were not statistically significant in all other indexes such as age, sex, primary site, laterality, T stage, M stage, surgery, chemotherapy, bone metastases, lung metastases and survival times.
Baseline data of lymphatic metastases were shown in Table 2. In all study populations, there were a total of 135 cases with lymphatic metastasis or status that could not be assessed, including 128 cases from the training set and seven cases from the validation set. After comparing two sets, results revealed T stage, M stage, surgery, bone metastases, survival time was variables with P < 0.05.
Univariate and Multivariate Logistic Regression Analyses
As shown in Table 3, logistics regression analysis was performed to define risk factors for LNM in ES. Firstly, clinicopathological characteristics of two sets were compared using univariate logistic regression analysis. Race, T stage, M stage, surgery, lung metastases were factors with P < 0.05 in univariate logistic regression analysis and entered multivariate regression analysis. Finally, we came to the conclusion that race (black, OR = 2.270, 95% CI = 1.020–5.052, P = 0.045), T stage (T2, OR = 1.733, 95% CI = 1.044–2.876, P = 0.033; Tx, OR = 2.712, 95% CI = 1.511–4.870, P = 0.001), M stage (M1, OR = 2.038, 95% CI = 1.157–3.591, P = 0.014), lung metastases (yes, OR = 1.877, 95%CI = 1.067–3.301, P = 0.029) were independent predictors for LNM in ES.
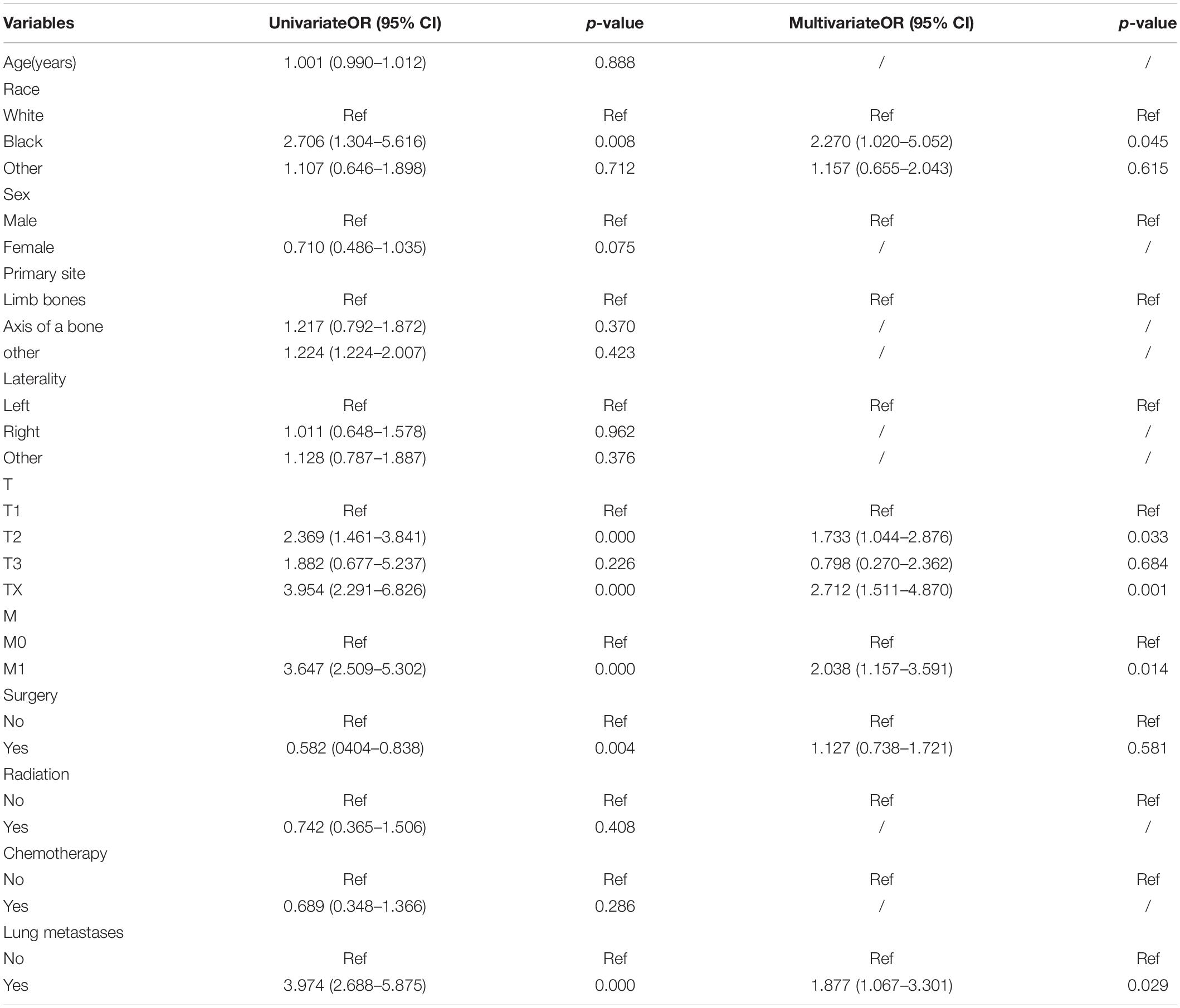
Table 3. Univariate and multivariate logistic regression analysis of risk factors for Lymph node metastases in patients with Ewing sarcoma.
The Performance of Machine Learning Algorithms
LNM status was regarded as the outcome index five factors with P < 0.05 in univariate logistic regression analysis mentioned above were defined as variables entered the model. ML algorithms including RF, NBC, DT, XGB, GBM, and LR were performed in the training set to develop prediction models. We adopted 10-fold cross-validation for internal validation to check the performance of each model (Figure 1). RF model performed best in predicting LNM in ES (average AUC = 0.764, std = 0.034). As shown in Figure 2, the RF model still showed the best performance in ROC curve analysis among 6 ML algorithms in the external validation (AUC = 0.727). Therefore, we chose the RF model as the final prediction model.
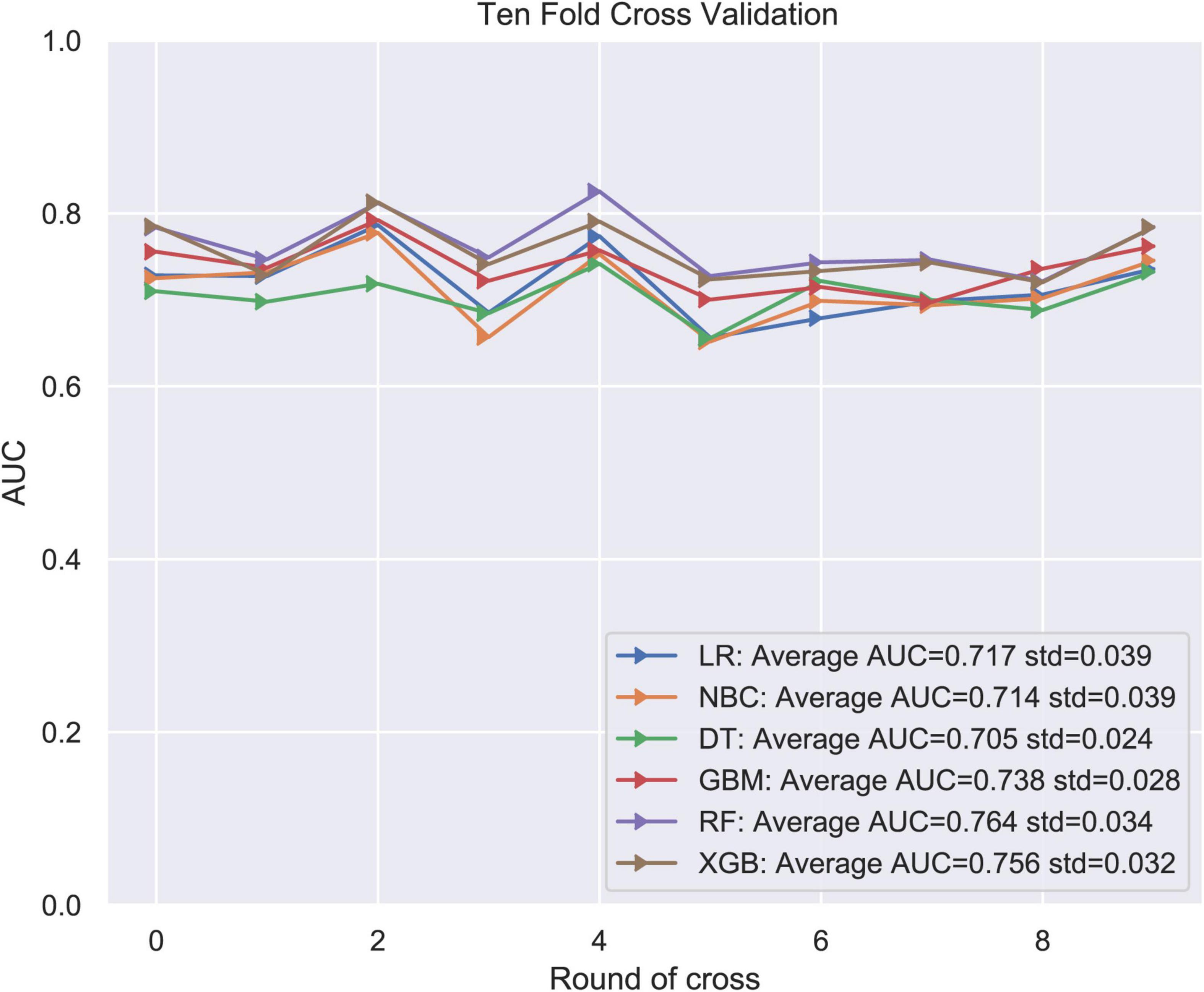
Figure 1. Ten-fold cross-validation of 6 ML algorithms for predicting LNM in patients with ES in the training set.
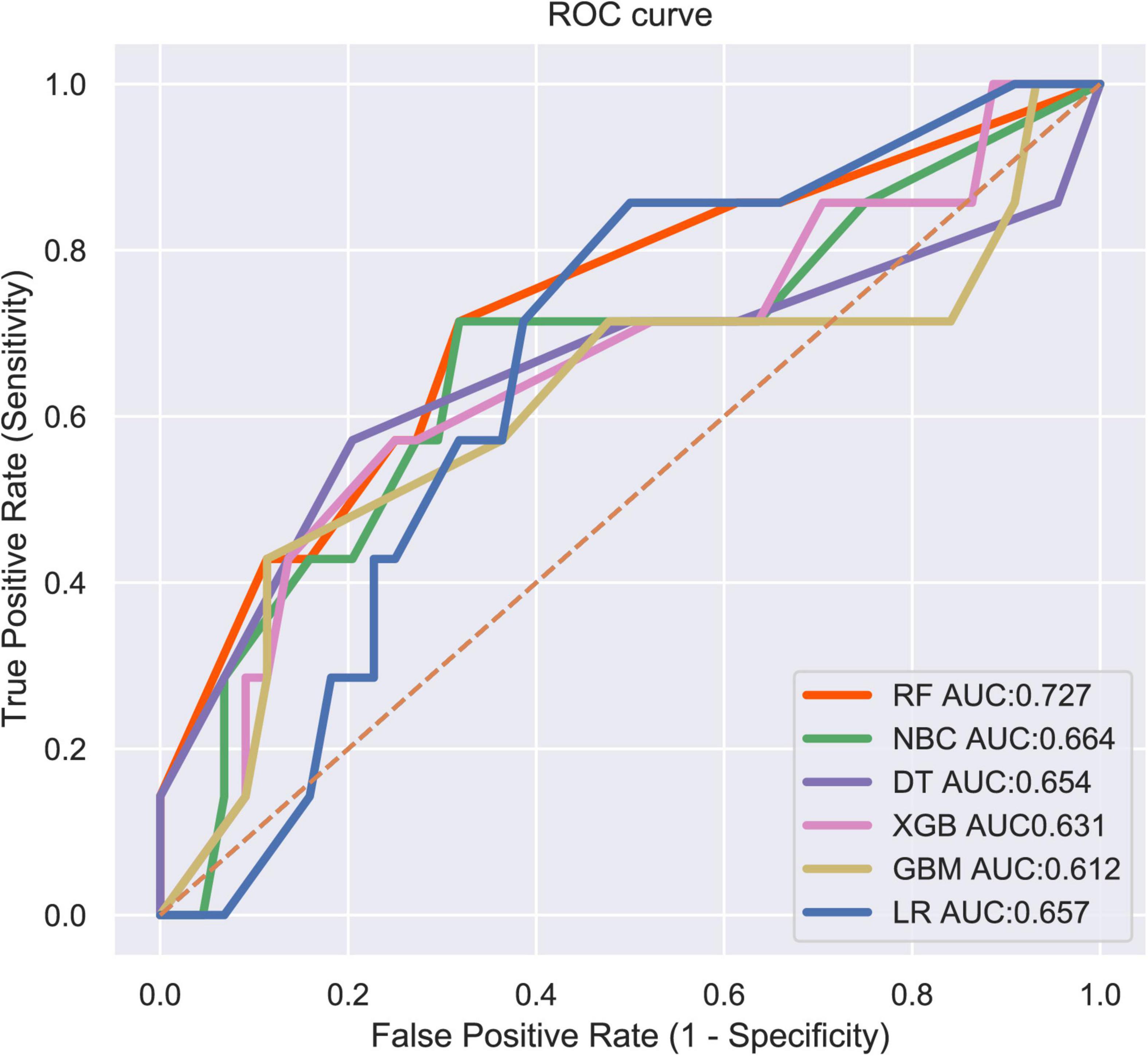
Figure 2. ROC curve analysis of 6 ML algorithms for predicting LNM in patients with ES in the validation set.
Relative Importance of Variables in 6 Models
As we can see in Figure 3, the relative importance ranking of each input variable was slightly different among the 6 models. However, it was obvious that T Stage, M Stage, lung metastasis were the top three indicators in each model. Race and surgery were low-ranking variables. In the RF model, the relative importance rank of all variables from high to low was M Stage, T Stage, lung metastasis, surgery and race.
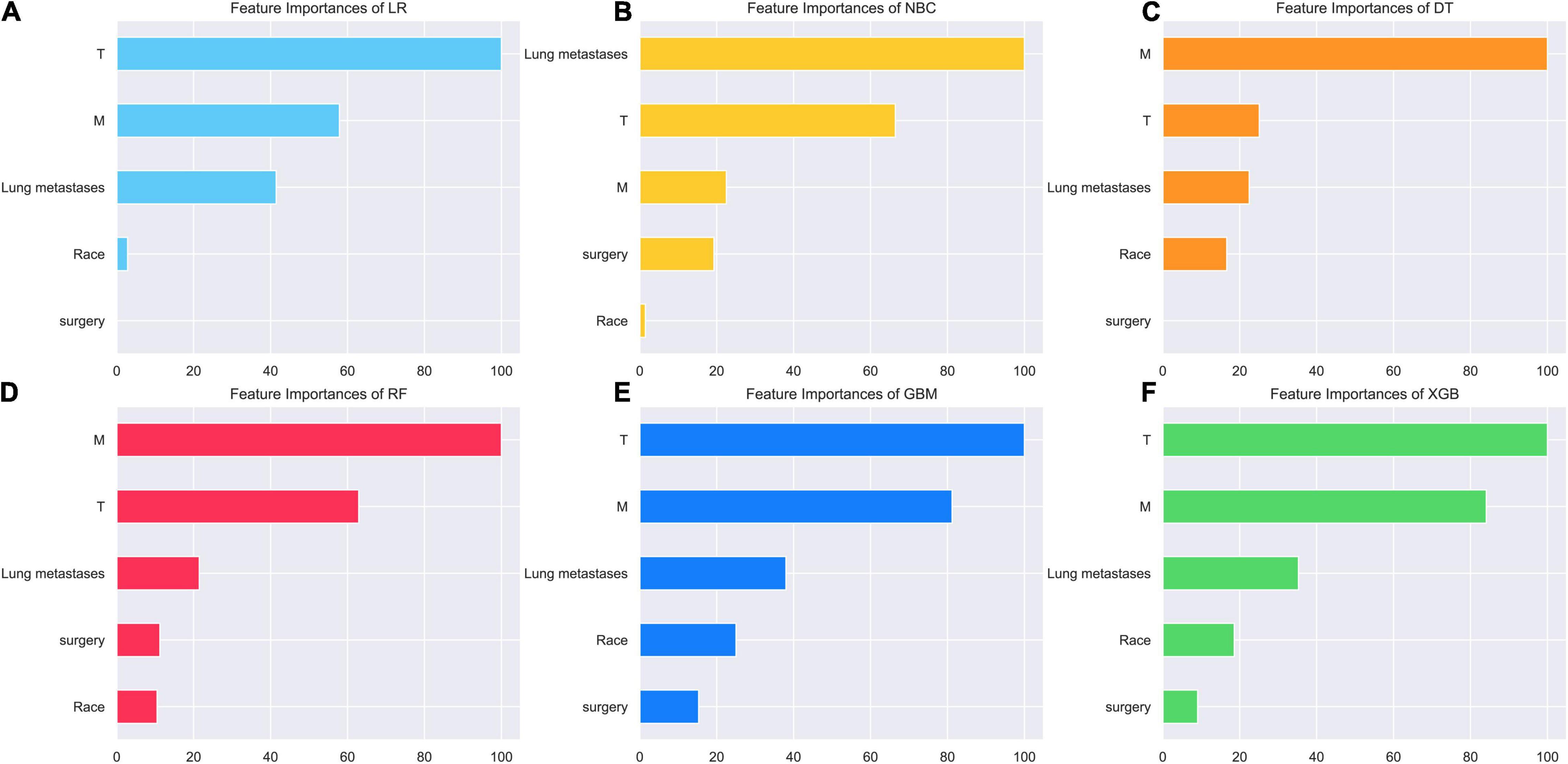
Figure 3. Relative importance ranking of each input variable for predicting models. (A) Random forest (RF). (B) Naive Bayes classifier (NBC). (C) Decision tree (DT). (D) Xgboost (XGB). (E) Gradient boosting machine (GBM). (F) Logistic regression (LR).
Web-Based Calculator
RF model performed best in 6 models. Accordingly, we established a web-based calculator to facilitate the clinical application of this prediction model (see text footnote 1; Figure 4).
Discussion
ES is highly invasive, and LNM worsens the prognosis. This study included 923 cases of ES in the SEER database for model establishment and 51 cases of ES from four independent institutions in China for external validation. In the total study population, 135 cases of ES had LNM or unknown condition of lymph node involvement. Machine learning (ML) has emerged as a powerful computer-based method and as a “prediction tool” in medical domain. It has been applied to model clinical outcome to detect more interactions between variables and to improve cognition of tumor growth and progression (24–26). We adopted six ML methods: RF, NBC, DT, XGB, GBM and LR. RF model performed best. Importance from highest to lowest, M stage, T stage, lung metastases (LM), surgery and race were the five variables in the final model. Finally, we established an online network calculator to facilitate clinical application.
M stage was the most important variable in the model. The risk ratio for LNM in M1 patients was 2.038. At the initial diagnosis of ES, 15–30% of cases have multiple metastases. Even after treatment, 30–40% of ES patients still have local or distant metastases (27). Paulussen and colleagues studied 171 cases of primary metastatic ES and found the metastasis incidence of lung, bone, lymph node, brain and liver was 35.7, 37.4, 2.9, 1.2, and 0%, respectively (17). LNM is more common when ES occurs in extra-skeletal sties (5). Lymph node involvement may promote tumor progression. The invaded regional lymph nodes can play as metastasis stations for tumor cell proliferation (28).
T was factor next to M stage in importance ranking. Many studies have proposed a correlation between tumor size and LNM. Some scholars studied 73 patients of orbital sarcoma (eight cases of ES) and found a higher risk of LNM was related to disease category of at least T3 (OR = 13.33, 95%CI = 1.77–602.30, P = 0.004) (29). In previous studies of thyroid papillary carcinoma, bladder urothelial carcinoma, early esophageal squamous cell carcinoma, osteosarcoma, rhabdomyosarcoma, breast cancer and other tumors, the size of tumor is significantly related to lymph node involvement (30, 31). Edwards and others believe that lymphatic vessels are absent in normal bones or bone tumors but can be found in tumors that have extended to periosteum and surrounding soft tissue (28). Larger tumors may have invaded the periosteum and surrounding soft tissue, which may explain the relationship between tumor size and LNM.
Lung metastases was another critical indicator. ES frequently metastasizes to the lungs (6). In this study, the LNM risk ratio for ES patients with LM was 1.877. In our previous study, N stage was the most significant predictor of LM and about 30.8% of LM patients had N1 or Nx status. Similarly, the study of Kato Y suggested that the severity of lymph node involvement was strongly correlated with lung metastasis in colorectal cancer patients (32). The exact correlation mechanism between LM and LNM of ES patients is needed to be further revealed.
Surgery was also one of the indicators in the RF model. In univariate analysis, the surgery rate of patients whose LNM or status cannot be assessed was significantly lower than that of patients without LNM (46.7 vs. 60.1%, P = 0.005). The focus of ES may shrink after induction treatment. As a result, the micro focus may not be found by MRI. The residual living tumor cells at the primary tumor site may cause secondary metastasis. The current consensus is that all anatomical structures involved in the extension of the original pretreated tumor should be removed during surgery (33). In a Cox regression model of pelvic ES, complete resection of affected bone and disappearance of extraosseous tumor components were associated with a lower risk of death (33). Several studies have also shown that surgical resection of the primary tumor is significantly associated with improved overall survival (OS) in patients with metastatic primary bone ES (8).
Race remains an important predictor, even if the importance ranking is the lowest. The incidence rate of ES varies from race to race. The incidence rate of ES was higher in Asians and Caucasians than that in blacks (17). The incidence rate in China was 2–3 times lower than that in Europe and America (34). Compared with patients of other races, black ES patients had a lower 10-year survival rate and an increased incidence of metastatic diseases at diagnosis. ES larger than 10cm in Hispanic patients were more frequent (7). As we have previously mentioned, larger tumors are closely associated with LNM.
Since LNM of ES strongly affects prognosis, we strongly recommend the evaluation of suspicious regional lymph nodes. Fine needle aspiration cytology (FNAC) in the expanded regional scope with full evaluation is helpful for early diagnosis, resection and prognosis improvement (35). Sentinel lymph node biopsy (SLNB) can conduct targeted sampling of local lymph nodes to avoid radical surgery or random biopsy. However, the accuracy of SLNB will be reduced when performed in previously treated areas because distortion of lymphatic channels may lead to bypassing the real sentinel lymph nodes (31). FDG-PET scan can well identify LNM. However, the reliability of FDG-PET scan was lower than SLNB, especially for small volume metastatic lymph node diseases in sarcoma. This limitation was linked to possible false-positive uptake in the benign process (28, 36). Some scholars have developed an ES-specific probe named CS2-N-E9R with high sensitivity and selectivity for E/F fusion protein. There was a certain prospect of CS2-N-E9R for accurate identification of LNM (3). Patients with LNM can be regarded as candidates for new treatment strategies and clinical trials (28).
The innovation of this paper lies in the technical and methodological innovation. By using the machine learning method, we have performed better than other methods in terms of clinical data and its application. There were a few limitations in this study. Firstly, the LNM prevalence of ES in the SEER database may be underestimated (1). This suggests that more careful evaluation is important in order to improve the prognosis of ES patients. In addition, data on specific chemotherapy drugs and intensity, radiotherapy dose, detailed surgical information and treatment response in the SEER database is absent (17). Further, data from different modalities such as radiomics we did not include in the model, otherwise the model would have a more powerful predictive power (25, 37, 38). In the last, our validation cohort were all from China. Further multi-center, prospective and multi-ethnic validation is needed to test the effectiveness of the model.
Conclusion
In this study, we applied ML algorithms to establish clinical risk models to predict LNM in ES patients. The RF model performed the best in internal and external validation. This model was an effective, non-invasive and convenient tool for clinical work. Further validation is needed.
Data Availability Statement
The raw data supporting the conclusions of this article will be made available by the authors, without undue reservation.
Ethics Statement
All participants signed written informed consents following the recommendations of the Institutional Review Board of the Xianyang Central Hospital. The ethical approval certificate is 20210022.
Author Contributions
CLY, SRK, and QL designed the study. WLL collected and interpreted the data. WLL, QZ, and WCL drafted the manuscript. CX, ZRT, STD, HSW, WYL, and KZ provided expert consultations and clinical suggestions. RL, WSZ, ZHH, and SSB revised the manuscript. All authors reviewed the final version of the manuscript. All authors contributed to the article and approved the submitted version.
Conflict of Interest
The authors declare that the research was conducted in the absence of any commercial or financial relationships that could be construed as a potential conflict of interest.
Publisher’s Note
All claims expressed in this article are solely those of the authors and do not necessarily represent those of their affiliated organizations, or those of the publisher, the editors and the reviewers. Any product that may be evaluated in this article, or claim that may be made by its manufacturer, is not guaranteed or endorsed by the publisher.
Acknowledgments
We would like to express our sincere gratitude to the volunteer participants for their cooperation throughout the study and their commitment toward all the required tests.
References
1. Applebaum MA, Goldsby R, Neuhaus J, DuBois SG. Clinical features and outcomes in patients with Ewing sarcoma and regional lymph node involvement. Pediatr Blood Cancer. (2012) 59:617–20.
2. Li G, Zhang P, Zhang W, Lei Z, He J, Meng J, et al. Identification of key genes and pathways in Ewing’s sarcoma patients associated with metastasis and poor prognosis. Onco Targets Ther. (2019) 12:4153–65.
3. Wang Y, Mai H, Yuan Y, Chen H, Wu S, Hu X, et al. EWS-FLI1-targeting peptide identifies Ewing sarcoma tumor boundaries and lymph node metastasis via near-infrared imaging. Mol Oncol. (2021) 15:3706–20.
4. Kondo T. Current status and perspectives of patient-derived models for Ewing’s sarcoma. Cancers. (2020) 12:2520.
5. Dong Y, Wu W, Kang H, Xiong W, Ye D, Fang Z, et al. Risk factors of regional lymph node (RLN) metastasis among patients with bone sarcoma and survival of patients with RLN-positive bone sarcoma. Ann Transl Med. (2021) 9:48.
6. Stork T, Boemans R, Hardes J, Streitbürger A, Dirksen U, Schildhaus HU, et al. Number of metastases and their response to chemotherapy impact survival of patients with isolated lung metastases from bone-derived sarcoma. BMC Cancer. (2021) 21:375. doi: 10.1186/s12885-021-08073-3
7. Duchman KR, Gao Y, Miller BJ. Prognostic factors for survival in patients with Ewing’s sarcoma using the surveillance, epidemiology, and end results (SEER) program database. Cancer Epidemiol. (2015) 39:189–95.
8. Ren Y, Zhang Z, Shang L, You X. Surgical resection of primary Ewing’s sarcoma of bone improves overall survival in patients presenting with metastasis. Med Sci Monit. (2019) 25:1254–62.
9. Cavallo P, Pagano S, De Santis M, Capobianco E. General practitioners records are epidemiological predictors of comorbidities: an analytical cross-sectional 10-year retrospective study. J Clin Med. (2018) 7:184.
10. Li W, Dong S, Wang B, Wang H, Xu C, Zhang K, et al. The construction and development of a clinical prediction model to assess lymph node metastases in osteosarcoma. Front Public Health. (2021) 9:813625. doi: 10.3389/fpubh.2021.813625
11. Salmasi L, Capobianco E. Predictive assessment of cancer center catchment area from electronic health records. Front Public Health. (2017) 5:303. doi: 10.3389/fpubh.2017.00303
12. Capobianco E. Precision oncology: the promise of big data and the legacy of small data. Front ICT. (2017) 4:22. doi: 10.3389/fict.2017.00022
13. Morsy AM, Abdel-Hadi S, Rezk KM, Amira G, Ahmed BM, Hussien MT, et al. Ewing sarcoma outcomes in a country with limited resources: Egypt as an example. Am J Cancer Res. (2021) 11:3212–26.
14. Duan X, Shang X, Yue J, Ma Z, Chen C, Tang P, et al. A nomogram to predict lymph node metastasis risk for early esophageal squamous cell carcinoma. BMC Cancer. (2021) 21:431. doi: 10.1186/s12885-021-08077-z
15. Luo X, Wang J, Xu M, Zou X, Lin Q, Zheng W, et al. Risk model and risk stratification to preoperatively predict central lymph node metastasis in papillary thyroid carcinoma. Gland Surg. (2020) 9:300–10.
16. Ou N, Song Y, Liu M, Zhu J, Yang Y, Liu X. Development and validation of a nomogram to predict lymph node metastasis in patients with T1 high-grade urothelial carcinoma of the bladder. Front Oncol. (2020) 10:532924. doi: 10.3389/fonc.2020.532924
17. Zhang L, Xiong L, Wu LM, Shen WH, Zhou P, Lian CL, et al. The patterns of distant metastasis and prognostic factors in patients with primary metastatic Ewing sarcoma of the bone. J Bone Oncol. (2021) 30:100385.
18. Capobianco E. Next generation networks: featuring the potential role of emerging applications in translational oncology. J Clin Med. (2019) 8:664.
20. Li W, Wang H, Dong S, Tang ZR, Chen L, Cai X, et al. Establishment and validation of a nomogram and web calculator for the risk of new vertebral compression fractures and cement leakage after percutaneous vertebroplasty in patients with osteoporotic vertebral compression fractures. Eur Spine J. (2021).
21. Li W, Wang J, Liu W, Xu C, Li W, Zhang K, et al. Machine learning applications for the prediction of bone cement leakage in percutaneous vertebroplasty. Front Public Health. (2021) 9:812023. doi: 10.3389/fpubh.2021.812023
22. Capobianco E. Systems and precision medicine approaches to diabetes heterogeneity: a big data perspective. Clin Transl Med. (2017) 6:23.
23. Dominietto MD, Capobianco E. Expected impacts of connected multimodal imaging in precision oncology. Front Pharmacol. (2016) 7:451. doi: 10.3389/fphar.2016.00451
24. Capobianco E. On digital therapeutics. Front Digit Humanity. (2015) 2:6. doi: 10.3389/fdigh.2015.00006
25. Capobianco E, Dominietto M. From medical imaging to radiomics: role of data science for advancing precision health. J Pers Med. (2020) 10:15.
26. Preo N, Capobianco E. Significant EHR feature-driven T2D inference: predictive machine learning and networks. Front Big Data. (2019) 2:30. doi: 10.3389/fdata.2019.00030
27. Şahin K, Bayram S, Salduz A. Calcaneal Ewing’s sarcoma with skip metastases to tarsals and lymph node involvement: a case report. J Foot Ankle Surg. (2018) 57:162–6.
28. Wan L, Tu C, Li S, Li Z. Regional lymph node involvement is associated with poorer survivorship in patients with chondrosarcoma: a SEER analysis. Clin Orthop Rel Res. (2019) 477:2508–18.
29. Sa HS, Rubin ML, Ning J, Li W, Tetzlaff MT, McGovern SL, et al. Association of T and N categories of the American joint commission on cancer, 8th edition, with metastasis and survival in patients with orbital sarcoma. JAMA Ophthalmol. (2020) 138:374–81.
30. Chen W, Wang C, Fu F, Yang B, Chen C, Sun Y. A model to predict the risk of lymph node metastasis in breast cancer based on clinicopathological characteristics. Cancer Manag Res. (2020) 12:10439–47.
31. De Corti F, Dall’Igna P, Bisogno G, Casara D, Rossi CR, Foletto M, et al. Sentinel node biopsy in pediatric soft tissue sarcomas of extremities. Pediatr Blood Cancer. (2009) 52:51–4.
32. Kato Y, Shigeta K, Okabayashi K, Tsuruta M, Seishima R, Matsui S, et al. Lymph node metastasis is strongly associated with lung metastasis as the first recurrence site in colorectal cancer. Surgery. (2021) 170:696–702.
33. Andreou D, Ranft A, Gosheger G, Timmermann B, Ladenstein R, Hartmann W, et al. Which factors are associated with local control and survival of patients with localized pelvic Ewing’s sarcoma? A retrospective analysis of data from the Euro-EWING99 trial. Clin Orthop Relat Res. (2020) 478:290–302.
34. Lee JA, Lim J, Park D, Jin HY, Park M, Park HJ, et al. Incidence patterns and outcomes of Ewing sarcoma in South Korea (1999-2017): a retrospective analysis using Korea central cancer registry data. Cancer Res Treat. (2021).
35. Loya AC, Prayaga AK, Arora A, Sundaram C, Rao IS, Uppin SG, et al. Lymph node metastasis of soft tissue tumors: a cytomorphologic study. Acta Cytolog. (2007) 51:153–60.
36. Dall’Igna P, De Corti F, Alaggio R, Cecchetto G. Sentinel node biopsy in pediatric patients: the experience in a single institution. Eur J Pediatr Surg. (2014) 24:482–7.
37. Capobianco E, Deng J. Radiomics at a glance: a few lessons learned from learning approaches. Cancers. (2020) 12:2453.
Keywords: Ewing sarcoma, lymph node metastasis, SEER, multi-center, machine learning, web calculator
Citation: Li W, Zhou Q, Liu W, Xu C, Tang Z-R, Dong S, Wang H, Li W, Zhang K, Li R, Zhang W, Hu Z, Shibin S, Liu Q, Kuang S and Yin C (2022) A Machine Learning-Based Predictive Model for Predicting Lymph Node Metastasis in Patients With Ewing’s Sarcoma. Front. Med. 9:832108. doi: 10.3389/fmed.2022.832108
Received: 09 December 2021; Accepted: 24 February 2022;
Published: 06 April 2022.
Edited by:
Enrico Capobianco, University of Miami, United StatesReviewed by:
Ting Xiao, Chinese Academy of Medical Sciences and Peking Union Medical College, ChinaZhidong Zhu, Shanghai Outdo Biotech Co., Ltd., China
Copyright © 2022 Li, Zhou, Liu, Xu, Tang, Dong, Wang, Li, Zhang, Li, Zhang, Hu, Shibin, Liu, Kuang and Yin. This is an open-access article distributed under the terms of the Creative Commons Attribution License (CC BY). The use, distribution or reproduction in other forums is permitted, provided the original author(s) and the copyright owner(s) are credited and that the original publication in this journal is cited, in accordance with accepted academic practice. No use, distribution or reproduction is permitted which does not comply with these terms.
*Correspondence: Chengliang Yin, Y2hlbmdsaWFuZ3lpbkAxNjMuY29t; Sirui kuang, NjUxNDM4MzY3QHFxLmNvbQ==; Qiang Liu, bTEzOTkyMDc5NjY4QDE2My5jb20=
†These authors have contributed equally to this work