- 1Division of Pulmonary and Critical Care Medicine, Mayo Clinic, Rochester, MN, United States
- 2Department of Internal Medicine, Mayo Clinic, Rochester, MN, United States
- 3Anesthesia and Critical Care Research Unit, Mayo Clinic, Rochester, MN, United States
- 4Department of Quantitative Health Sciences, Mayo Clinic, Rochester, MN, United States
- 5Department of Infectious Disease, Multi-disciplinary Epidemiology and Translational Research in Intensive Care Research Group, Mayo Clinic, Rochester, MN, United States
Background: Meaningful patient centered outcomes of critical illness such as functional status, cognition and mental health are studied using validated measurement tools that may often be impractical outside the research setting. The Electronic health record (EHR) contains a plethora of information pertaining to these domains. We sought to determine how feasible and reliable it is to assess meaningful patient centered outcomes from the EHR.
Methods: Two independent investigators reviewed EHR of a random sample of ICU patients looking at documented assessments of trajectory of functional status, cognition, and mental health. Cohen's kappa was used to measure agreement between 2 reviewers. Post ICU health in these domains 12 month after admission was compared to pre- ICU health in the 12 months prior to assess qualitatively whether a patient's condition was “better,” “unchanged” or “worse.” Days alive and out of hospital/health care facility was a secondary outcome.
Results: Thirty six of the 41 randomly selected patients (88%) survived critical illness. EHR contained sufficient information to determine the difference in health status before and after critical illness in most survivors (86%). Decline in functional status (36%), cognition (11%), and mental health (11%) following ICU admission was observed compared to premorbid baseline. Agreement between reviewers was excellent (kappa ranging from 0.966 to 1). Eighteen patients (44%) remained home after discharge from hospital and rehabilitation during the 12- month follow up.
Conclusion: We demonstrated the feasibility and reliability of assessing the trajectory of changes in functional status, cognition, and selected mental health outcomes from EHR of critically ill patients. If validated in a larger, representative sample, these outcomes could be used alongside survival in quality improvement studies and pragmatic clinical trials.
Introduction
The measurement of important outcomes of critical illness continues to evolve. Medical advances have resulted in significant improvement in mortality despite increasing age, severity of illness, and number of comorbidities (1, 2). Most randomized controlled trials in critical care research are negative studies, with problems pertaining not just to statistical analysis and heterogenous biological attributes but also the choice of “mortality” as a measurable but crude outcome of critical illness (3, 4). Mortality is just one side of the coin, since challenges of critical illness survivorship come at a cost of pain, anxiety, depression, impaired cognition, and debility, collectively described as post-ICU syndrome (PICS)(5, 6). Amongst the most relevant predictors of outcomes of survival are older age, comorbidities, and pre- ICU health status (7–9).
Significant effort has been dedicated toward identifying the most relevant domains by which clinicians and scientists can measure outcomes meaningful to patients. About 250 tools have been used in clinical research to capture relevant health domains hindering comparison of results (10). Measurement properties of such tools per consensus-based standards are inadequately assessed for in studies reporting them (11). The National Institute of Health (NIH) has developed a framework of key components of patient reported outcomes that can be used in clinical studies (12–16) to help address survivorship. Health trajectories comparing baseline/ pre- ICU status to post ICU follow ups have been accomplished in individual domains such as cognitive status, functional status or as health-related quality of life (HRQOL) using some of these measurement tools (17–21). Today, survival, HRQOL, pain, cognitive function, physical function, mental health, muscle/nerve function and pulmonary function have been identified as the most salient domains from the standpoint of clinicians, researchers, and patients and their families (12–16).
Days alive and out of hospital (DAOH), also known as hospital free days has also gained increased interest in studies as a pragmatic patient centered outcome (22). It has been captured in larger registries in the frail population and after hip fracture (23, 24). Elderly patients have expressed, that time spent at home, especially near end of life, as a very important preference (25).
Administration of validated measurement tools can be time consuming, costly, and impractical outside large research settings. A previous comparison between health survey and EHR of outcomes of multimorbidity showed that both methods were equivalent in the elderly group (26). No study has evaluated the quantity and quality of EHR representation of patient centered outcomes when approaching a combination of outcomes of functional status, cognitive status and mental health, or the trajectory of these outcomes before and after critical illness.
We therefore hypothesized that we could abstract variables from “free text” contained in the EHR which could provide valuable insight into the pre to post ICU trajectory in these domains. We chose DAOH to represent days alive and out of hospital/ health care facility, as a complimentary meaningful outcome to assess from EHR. If feasible, EHR assessments could be automated to provide a scalable, clinically useful method for studying meaningful patient centered outcomes in pragmatic trials and quality improvement interventions.
Methods
Study Design
This is a feasibility study comprising of a single center retrospective review of the EHRs in a random sample of elderly ICU patients between 2015 and 2019 at the Mayo Clinic, Rochester, MN. The study was approved by the Mayo Clinic Institutional Review Board (#18-004302). The institutional EHR was changed to Epic in May of 2018 and patient information documented prior to 2018 was also uploaded into Epic (Figure 1). The Anesthesia and Critical Care Research Unit (ACRU) at the Mayo Clinic assisted with data extraction and manual chart review; latter was performed by SA and LB.
Data on demographics, diagnoses, hospital outcomes and patient related outcomes including functional status, cognition, and mental health was stored in a REDCap data base. The REDCap data collection questions pertaining to patient outcomes were designed using the qualitative technique of data saturation, whereby questions were continually developed and locations for such information were identified during chart review (27). Charts were continually revisited and studied until the relevant content identified by each reviewer was abstracted by both reviewers. The information was then evaluated by each reviewer to determine if trajectory of functional status, cognition, and mental health in the 12-month time frame prior to illness and within 12-months after discharge for survivors could be established to look for meaningful changes in survivors. Discrepancies were identified by each reviewer for resolution during intergroup discussions and when needed by a third reviewer: AT.
Each domain was reviewed for relevant chart documentation with key words used to identify the outcome in the chart (Supplementary Table 1), reflective of aspects of body function and structures, activities and participation described in World Health Organization (WHO) description of International Classification of Function (ICF) in 2001. For this feasibility study, functional status included aspects of physical function and activities of daily living. Some key themes of cognition included that of attention, alertness, and memory. Similarly, mental health included the search for depression, anxiety, and post -traumatic stress disorder (PTSD). For example, functional status could be captured as a diagnosis of “repeated falls” or “frailty”, a description of a specific activity of daily living, or the need of a certain support such as a walking aid. Other parameters included specific medications, validated tools documenting the functional, cognitive, and mental health status and ICD-10 (international classification of diseases) codes. For example, the use or new prescription of antidepressant could help identify encounters discussing depression. Similarly, validated tools such as PHQ (patient health questionnaire)-9 for depression or mental status exam tools can be found in charts as well. Of note, ICD- 10 codes were an automated query and were available for charts abstracted prior to institutional EMR change.
Particular attention was paid to documentation and clinical discourse capturing patient/provider quotes and any descriptions of patient's narratives as they related to the outcome of interest (Supplementary Table 2). For charts where there was inadequate information to support the presence or absence of a particular outcome, we categorized it as “unable to determine”.
Since chart review included 12 months EHR follow up, discharge dates, number of admissions and integrated rehabilitation days were also tracked in the EMR to assess the ability to compute DAOH.
Participants
Patients over 65 years old admitted to the ICU from 8/23/2015 to 8/7/2019 for >24 h and requiring one or more of the following: high-flow nasal cannula, bilevel or continuous positive pressure ventilation, invasive mechanical ventilation, vasopressor need were selected for potential inclusion to this study. Despite variable age cut offs in the aging population, for the purpose of this study, we defined elderly as age more than 65 years. The patients included in the study were residents of Olmsted County, Minnesota, with research authorization on file. Patients in a vegetative state as baseline or those requiring intubation for elective surgical procedures were excluded. In total, 41 patient chart reviews were completed, with 23 having index ICU admission occur prior to EHR change and 18 after.
Outcomes
The primary end point was to evaluate whether it is possible to establish the pre- to post-ICU trajectory in the domains of functional status, cognition and mental health using the EHR in a 12-month chart follow up.
The secondary end point was to evaluate whether DAOH, can be extracted from the EHR as a complimentary meaningful patient outcome up to the 12-month EHR follow up.
Statistical Analysis
Patient characteristics, interventions, and outcomes were summarized using median and interquartile range (IQR) for continuous variables and frequency counts and percentages for nominal variables. Cohen's kappa coefficient was used to measure agreement between the two reviewers regarding change in pre- and post-ICU quality of life (28).
DAOH was considered zero if a patient was unable to be discharged home after short term rehabilitation. Days after ICU admission were calculated, observing for survival post ICU, date of death within the 12-month EHR follow up, hospital discharge day and number of days of recurrent admissions to the hospital. The category of “some days at home” contained a range of calculated DAOH.
Data management and statistical analysis were performed in SAS Studio 3.8 (SAS Institute Inc, Cary, North Carolina) and Microsoft Excel 365 (Microsoft Corporation, Redmond, WA).
Results
Two independent investigators completed EHR reviews of a random sample of 41 patients (Figure 1). Five (12%) patients died during the hospitalization. Table 1 describes demographic and clinical characteristics of these patients. A variety of note sources were reviewed where pertinent information was found. Change in each of the specific domains of functional status, cognitive status and mental health were ascertained by evaluating a combination of notes before and after critical illness in the EHR. For example, if there was clinical documentation of normal activities of daily living pre-ICU and wheelchair dependence post ICU, that would result in “worsening” functional status. Another example, if there was no cognitive dysfunction in pre-ICU assessment and no concern raised in sequential follow up notes despite adequate documented dialogue, then the impression was “no change”. Where there was insufficient follow up or documentation, we considered those situations as “unable to determine.” Some high yield notes besides routine inpatient and outpatient notes included social work, physical/occupational therapy, nursing and subspecialty notes (Supplementary Table 3). Very detailed pre- ICU functional status, cognition and mental health were best captured in social work notes and physical and occupational therapy notes. Post- ICU, outpatient notes at rehabilitation or clinic were higher yield. Free texts in “chart documentation” were the most common source of information (Supplementary Table 1). Specific medications were most helpful for identifying and exploring mental health domain. Validated tools for each domain were found less commonly in the before and after critical illness time frame, such as in 4 charts for each functional and cognitive status and 7 charts for mental health (Supplementary Table 1).
The majority (86%) of charts contained sufficient information to determine the pre- ICU to post- ICU health state trajectories (Table 2). There was almost perfect inter-rater agreement between the two reviewers with kappa values of 0.966, 1 and 1 in functional status, cognitive status, and mental health trajectories, respectively.
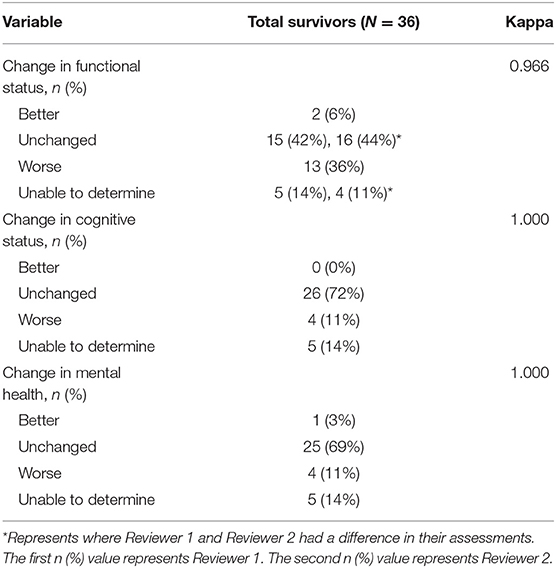
Table 2. Change in pre- and post-ICU functional status, cognition, and mental health, per EHR review with corresponding agreement between two independent reviewers.
Eighteen (44%) of patients remained at home at 12 months after discharge from hospital or short-term rehabilitation, whereas 13 (32%) had zero DAOH, including those who died or ended up in long term health care facility (Table 3). The category “some days at home” contained a range of calculated DAOH between 1 and 288 days.
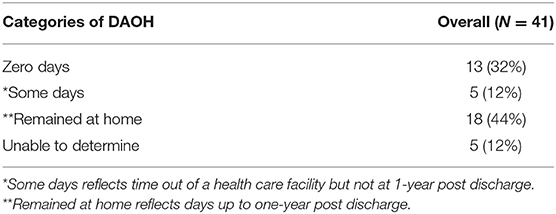
Table 3. Days alive and out of hospital/ health facility (DAOH) after discharge within 12 months per EHR.
Discussion
This pilot study shows that it is feasible to use EHR to extract qualitative change to reveal a trajectory in meaningful patient centered outcomes after critical illness including functional status, cognition, mental health, and days alive and out of hospital/health care facility (DAOH). EHR sources included a variety of multi-professional notes. Notably, social worker and physical and occupational therapy notes were quite comprehensive in capturing a combination of these domains as patient's pre-ICU baseline. There was excellent agreement between two independent reviewers.
A multifaceted approach to the search was needed to explore each domain to adequately assess the health status before and after critical illness rather than rely on any one parameter. The highest yield parameter was free texts. Medications helped identify mental health. Trajectories relying alone on validated tools were inadequate in this exploration. For example, Barthel index may be used in rehabilitation, but not in an outpatient clinical encounter or even in the hospital where another type of tool is used, and hence comparison is difficult.
Despite concerns that EHR contained inadequate documentation to be a reliable source for outcomes of functional status, cognitive status, or mental health (29–32), it has been widely used in clinical research. A review of implementing WHO Internation Classification of Function (ICF) in EHR, revealed benefits of universal applicability of ICF, collaboration and user satisfaction, though also picked on challenges with clinical terminologies and development of ontologies (33).
The Barthel index, one of the validated tools to assess functional status, has been computed using EHR nursing documentation to capture pre-ICU baseline functional status and at 1 year follow up to assess new functional impairments in patients receiving mechanical ventilation (34). A strategy of deriving functional status from documented activities of daily living (ADL) has previously been applied with the help of EHR (35–37). Nursing documentation of mobility of elderly patients identified those at risk for poor outcomes (38). EHR chart review of physical and occupational therapy and discharge notes, revealed association of higher functional dependence in COVID-19 survivors with higher burden of disease and increased age (39). EHR has been successfully used to derive variables using ICD-10 codes to develop frailty risk score (40).
For cognitive function, documentation is more consistent in the EHR for dementia than for milder cognitive impairment (31). Validated test scores for dementia are rarely available in EHR, though algorithms for diagnosis codes and prescriptions for dementia are found to be reliable (41). In contrast, the ability of detecting cognitive impairment with ICD codes and frailty index in annual Medicare wellness checks of survivors of critical illness was very low (42). However, using key terms such as cognitive deficit, impaired memory, impaired judgement, to develop an EHR algorithm resulted in excellent sensitivity and specificity to capture cognitive impairment that outperformed ICD codes (43).
No previous EHR study observed mental health outcomes of depression and anxiety in critical illness. Mental health diagnoses of depression and anxiety have been explored with EHR looking at some combination of variables such as rates of psychotropic medications, diagnoses codes, referrals for mental health services and in validated tools such as PHQ-9 (44–46). Documentation for anxiety in critical illness reviewing provider notes was found in about 45% of sampled chart reviews with terms such as panic, anxiety, and distress (47). Capturing PTSD is particularly challenging. An algorithm for diagnosis of PTSD in EHR has compared very well with chart review (48). However, documentation of PTSD in EHR has been fraught with omission of information resulting in missing data in studies from the Veteran Affairs and primary care (49, 50). In our sample of charts, we did not find any documentation of PTSD.
DAOH has surfaced as a promising pragmatic patient centered outcome but further refinement is required prior to more widespread use (22). There is heterogeneity in the intervals of measurements from hospital discharge or time of randomization to ranges in between 28 days and 365 days (51–55). The accuracy of DAOH calculation from EHR is limited since long term acute care days may be imprecisely accounted for (22), similar to our experience.
Our study differs in several ways. We assess the feasibility of extracting a combination of more than one or two outcomes, provide a methodology of the process, and track a trajectory of pre-ICU to post- ICU health. This identified high yield areas in the EHR that progressively made the process of chart review easier and for the reviewers to form mutual agreement. We employed a multifaceted approach using search for relevant medications, tools, diagnosis code, and chart review with specific documentation in the form of free text. Free text dialogue observed in our study captured a discourse, such as a new description of “wheelchair bound” after critical illness to reflect a change in functional status, or “mood is down and sad” to support clinical documentation of mental health change. This is similar to how free texts in EHR have been used to map presence of anxiety and depression symptoms, “easy to wake u” and “depressed or sad mood” (56). This approach could be further developed into an ontology for free text data mining with automated Natural Language Processing (NLP). Such ontologies have been previously built to capture frailty in cardiac patients and functional status in patients with colorectal cancer (57, 58). NLP and machine learning has also been extensively used to in studies for mental health (59, 60). In critical care, NLP has been used to predict outcomes, using discrete clinical free texts such as sepsis, pupils fixed and coagulopathy (61). NLP for inclusion criteria has also been used for recruiting patients for a randomized clinical trial in sepsis (62).
Several weaknesses limit the interpretation of this feasibility study. First, much of what can be abstracted in the free text depends on the quality and variability of documentation and identifying relevant note sources. Data saturation by two reviewers was used to help minimize this limitation. The substantial documentation of these outcomes encountered may be reflective of our multi- disciplinary approach toward patient care at our institution. Second, chart review is subjective, allowing for reviewers to have unique interpretations of the same data. These discrepancies were resolved within our group discussions. Third, the timeline of this study includes a change in the EHR system and aspects of documentation have changed with refining the EHR since. Fourth, in computing DAOH, documentation on length of stay in rehabilitation facilities was not always accurately observed in the EHR. Fifth, this is a single center feasibility study with a small sample size, and it was not possible to assess the incidence or prevalence of the outcomes, nor was this the intention of the study. Sixth, and perhaps the most important limitation is that the trends in functional status, cognition, and mental health identified from EHR may not always directly compare to validated tools, since one is the impression of the provider, and the tools reflect those of the patient. Both methods certainly offer unique perspectives and can potentially complement each other.
With an increasing focus on post- ICU recovery, improving the efficiency of extracting these domains will be essential. Annotating data, identifying themes and texts to develop automated queries in EHR, could help create algorithms and a NLP ontology (63) to develop ‘meaningful patient centered outcomes’ and for mapping to tools for validation as recommended in https://www.improvelto.com. Such an outcome measure if reliable would be ideal for use in pragmatic trials, quality improvement interventions as well as a humanized digital platform for patient care. The ability to identify such outcomes in EHR can help then analyze a chart more efficiently to determine trajectory of health before and after critical illness. DAOH, could also be automated, if rehabilitation encounters are more accurately captured in the EHR. Future directions to this work would include repeating such EHR explorations from academic tertiary centers as well as community hospitals to account for the differences in documentation and content present in EHR. There may be variability in availability of resources, such as physical and occupational therapy and social work to account for differences in EHR content. There is also an opportunity to simplify validated tools for consistent patient interactive use in EHR.
Eventually, the goal is to develop a pragmatic method to measure a combination of meaningful patient centered outcomes complementary to routine outcomes like mortality and health care lengths of stay usual in critical care research.
Conclusion
The health domains of functional status, cognitive status, mental health, trajectory of pre to post ICU health, and days at home after critical illness are some of the key domains of meaningful patient centered outcomes. It is feasible to observe a trajectory of these outcomes pre-ICU and post-ICU in EHR reviews which could be further developed for use in pragmatic critical care research.
Data Availability Statement
The original contributions presented in the study are included in the article/Supplementary Material, further inquiries can be directed to the corresponding author/s.
Ethics Statement
The studies involving human participants were reviewed and approved by Mayo Clinic Institutional Review Board (#18-004302). Written informed consent for participation was not required for this study in accordance with the national legislation and the institutional requirements.
Author Contributions
SA: conceptualization, methodology, software, investigation, formal analysis, visualization, writing original draft, project administration, and funding acquisition. AT: validation, investigation, and writing original draft. LB: investigation and data curation. AL: formal analysis, visualization, and writing original draft. BA: resources and project administration. KV: methodology and software. LK: resources and writing-review and editing. OG: conceptualization, supervision, and writing-review and editing. All authors contributed to the article and approved the submitted version.
Funding
This work was supported by Small Grant Award, Mayo Clinic PI SA, FP00100342 and Critical Care Research Subcommittee, Mayo Clinic PI SA, Company 300, PAU 43306.
Conflict of Interest
The authors declare that the research was conducted in the absence of any commercial or financial relationships that could be construed as a potential conflict of interest.
Publisher's Note
All claims expressed in this article are solely those of the authors and do not necessarily represent those of their affiliated organizations, or those of the publisher, the editors and the reviewers. Any product that may be evaluated in this article, or claim that may be made by its manufacturer, is not guaranteed or endorsed by the publisher.
Acknowledgments
The authors thank Kimberly Evans, RRT, Anesthesia and Critical Care Research Unit for the automated retrieval of patients and Amy Spitzner, RN, for manual data extraction using EPIC.
Supplementary Material
The Supplementary Material for this article can be found online at: https://www.frontiersin.org/articles/10.3389/fmed.2022.826169/full#supplementary-material
References
1. Zimmerman JE, Kramer AA, Knaus WA. Changes in hospital mortality for United States intensive care unit admissions from 1988 to 2012. Critical care (London, England). (2013) 17:R81. doi: 10.1186/cc12695
2. Prescott HC, Angus DC. Enhancing recovery from sepsis: a review. JAMA. (2018) 319:62–75. doi: 10.1001/jama.2017.17687
3. Legrand M. Negative trials in critical care medicine and the hurdles. Lancet Res Med. (2018) 6:e53. doi: 10.1016/S2213-2600(18)30342-4
4. Laffey JG, Kavanagh BP. Negative trials in critical care: why most research is probably wrong. Lancet Respir Med. (2018) 6:659–60. doi: 10.1016/S2213-2600(18)30279-0
5. Colbenson GA, Johnson A, Wilson ME. Post-intensive care syndrome: impact, prevention, and management. Breathe (Sheff). (2019) 15:98–101. doi: 10.1183/20734735.0013-2019
6. Needham DM, Davidson J, Cohen H, Hopkins RO, Weinert C, Wunsch H, et al. Improving long-term outcomes after discharge from intensive care unit: report from a stakeholders' conference. Crit Care Med. (2012) 40:502–9. doi: 10.1097/CCM.0b013e318232da75
7. Ferrante LE, Pisani MA, Murphy TE, Gahbauer EA, Leo-Summers LS, Gill TM. Functional trajectories among older persons before and after critical illness. JAMA Intern Med. (2015) 175:523–9. doi: 10.1001/jamainternmed.2014.7889
8. Haas B, Wunsch H. How does prior health status (age, comorbidities and frailty) determine critical illness and outcome? Curr Opin Crit Care. (2016) 22:500–5. doi: 10.1097/MCC.0000000000000342
9. Cuthbertson BH, Wunsch H. Long-Term outcomes after critical illness. the best predictor of the future is the past. Am J Respir Crit Care Med. (2016) 194:132–4. doi: 10.1164/rccm.201602-0257ED
10. Turnbull AE, Rabiee A, Davis WE, Nasser MF, Venna VR, Lolitha R, et al. Outcome measurement in ICU survivorship research from 1970 to 2013: a scoping review of 425 publications. Crit Care Med. (2016) 44:1267–77. doi: 10.1097/CCM.0000000000001651
11. Robinson KA, Davis WE, Dinglas VD, Mendez-Tellez PA, Rabiee A, Sukrithan V, et al. A systematic review finds limited data on measurement properties of instruments measuring outcomes in adult intensive care unit survivors. J Clin Epidemiol. (2017) 82:37–46. doi: 10.1016/j.jclinepi.2016.08.014
12. Rothrock NE, Hays RD, Spritzer K, Yount SE, Riley W, Cella D. Relative to the general US population, chronic diseases are associated with poorer health-related quality of life as measured by the Patient-Reported Outcomes Measurement Information System (PROMIS). J Clin Epidemiol. (2010) 63:1195–204. doi: 10.1016/j.jclinepi.2010.04.012
13. Eakin MN, Patel Y, Mendez-Tellez P, Dinglas VD, Needham DM, Turnbull AE. Patients' outcomes after acute respiratory failure: a qualitative study with the PROMIS framework. Am J Crit Care. (2017) 26:456–65. doi: 10.4037/ajcc2017834
14. Dinglas VD, Chessare CM, Davis WE, Parker A, Friedman LA, Colantuoni E, et al. Perspectives of survivors, families and researchers on key outcomes for research in acute respiratory failure. Thorax. (2018) 73:7–12. doi: 10.1136/thoraxjnl-2017-210234
15. Hodgson CL, Turnbull AE, Iwashyna TJ, Parker A, Davis W, Bingham CO, et al. Core domains in evaluating patient outcomes after acute respiratory failure: international multidisciplinary clinician consultation. Phys Ther. (2017) 97:168–74. doi: 10.2522/ptj.20160196
16. Needham DM, Sepulveda KA, Dinglas VD, Chessare CM, Friedman LA, Bingham CO. 3rd, Turnbull AE. Core outcome measures for clinical research in acute respiratory failure survivors. an international modified delphi consensus study Am J Respir Crit Care Med. (2017) 196:1122–30. doi: 10.1164/rccm.201702-0372OC
17. Ferrante LE, Murphy TE, Gahbauer EA, Leo-Summers LS, Pisani MA, Gill TM. Pre-ICU cognitive status, subsequent disability, and new nursing home admission among critically ill older adults. Ann Am Thorac Soc. (2018) 15:622–29. doi: 10.1513/AnnalsATS.201709-702OC
18. Pfoh ER, Chan KS, Dinglas VD, Girard TD, Jackson JC, Morris PE, et al. Cognitive screening among acute respiratory failure survivors: a cross-sectional evaluation of the mini-mental state examination. Critical Care (London, England). (2015) 19:220. doi: 10.1186/s13054-015-0934-5
19. Biehl M, Kashyap R, Ahmed AH, Reriani MK, Ofoma UR, Wilson GA Li G, et al. Six-month quality-of-life and functional status of acute respiratory distress syndrome survivors compared to patients at risk: a population-based study. Critical Care (London, England). (2015) 19:356. doi: 10.1186/s13054-015-1062-y
20. Pandharipande PP, Girard TD, Jackson JC, Morandi A, Thompson JL, Pun BT, et al. Long-Term cognitive impairment after critical illness. N Engl J Med. (2013) 369:1306–16. doi: 10.1056/NEJMoa1301372
21. Hofhuis JG, Spronk PE, van Stel HF, Schrijvers GJ, Rommes JH, Bakker J. The impact of critical illness on perceived health-related quality of life during ICU treatment, hospital stay, and after hospital discharge: a long-term follow-up study. Chest. (2008) 133:377–85. doi: 10.1378/chest.07-1217
22. Auriemma CL, Taylor SP, Harhay MO, Courtright KR, Halpern SD. Hospital-Free days: a pragmatic and patient-centered outcome for trials among critically and seriously ill patients. Am J Respir Crit Care Med. (2021) 204:902–9. doi: 10.1164/rccm.202104-1063PP
23. McIsaac DI, Talarico R, Jerath A, Wijeysundera DN. Days alive and at home after hip fracture: a cross-sectional validation of a patient-centred outcome measure using routinely collected data. BMJ Qual Saf. (2021) (in press). doi: 10.1136/bmjqs-2021-013150
24. Hendin A, Tanuseputro P, McIsaac DI, Hsu AT, Smith GA, Begum J, et al. Frailty is associated with decreased time spent at home after critical illness: a population-based study. J Intensive Care Med. (2021) 36:937–44. doi: 10.1177/0885066620939055
25. Groff AC, Colla CH, Lee TH. Days spent at home - a patient-centered goal and outcome. N Engl J Med. (2016) 375:1610–2. doi: 10.1056/NEJMp1607206
26. Violan C, Foguet-Boreu Q, Hermosilla-Perez E, Valderas JM, Bolibar B, Fabregas-Escurriola M, et al. Comparison of the information provided by electronic health records data and a population health survey to estimate prevalence of selected health conditions and multimorbidity. BMC Public Health. (2013) 13:251. doi: 10.1186/1471-2458-13-251
27. Fusch PI, Ness LR. Are we there yet? data saturation in qualitative research. Qualitative Report. (2015) 20:1408. doi: 10.46743/2160-3715/2015.2281
28. McHugh ML. Interrater reliability: the kappa statistic. Biochem Med (Zagreb). (2012) 22:276–82. doi: 10.11613/BM.2012.031
29. Bogardus ST. Jr., Towle V, Williams CS, Desai MM, Inouye SK. What does the medical record reveal about functional status? a comparison of medical record and interview data J Gen Intern Med. (2001) 16:728–36. doi: 10.1111/j.1525-1497.2001.00625.x
30. Crum-Cianflone NF, Powell TM, LeardMann CA, Russell DW, Boyko EJ. Mental health and comorbidities in U.S. Military Members Mil Med. (2016) 181:537–45. doi: 10.7205/MILMED-D-15-00187
31. Chodosh J, Petitti DB, Elliott M, Hays RD, Crooks VC, Reuben DB, et al. Physician recognition of cognitive impairment: evaluating the need for improvement. J Am Geriatr Soc. (2004) 52:1051–9. doi: 10.1111/j.1532-5415.2004.52301.x
32. Ratcliff CG, Barrera TL, Petersen NJ, Sansgiry S, Kauth MR, Kunik ME, et al. Recognition of anxiety, depression, and PTSD in patients with COPD and CHF: who gets missed? Gen Hosp Psychiatry. (2017) 47:61–7. doi: 10.1016/j.genhosppsych.2017.05.004
33. Maritz R, Aronsky D, Prodinger B. The International Classification of Functioning, Disability and Health (ICF) in electronic health records. a systematic literature review. Appl Clin Inform. (2017) 8:964–80. doi: 10.4338/ACI-2017050078
34. Wilson ME, Barwise A, Heise KJ, Loftsgard TO, Dziadzko M, Cheville A, et al. Long-Term return to functional baseline after mechanical ventilation in the ICU. Crit Care Med. (2018) 46:562–9. doi: 10.1097/CCM.0000000000002927
35. Lage DE, Jernigan MC, Chang Y, Grabowski DC, Hsu J, Metlay JP, et al. Living alone and discharge to skilled nursing facility care after hospitalization in older adults. J Am Geriatr Soc. (2018) 66:100–5. doi: 10.1111/jgs.15150
36. Wojtusiak J, Levy CR, Williams AE, Alemi F. Predicting functional decline and recovery for residents in veterans affairs nursing homes. Gerontologist. (2016) 56:42–51. doi: 10.1093/geront/gnv065
37. Munoz MA, Real J, Del Val JL, Vinyoles E, Mundet X, Frigola-Capell E, et al. Determinants of survival and hospitalization in older, heart failure patients receiving home healthcare. Int J Cardiol. (2016) 207:145–9. doi: 10.1016/j.ijcard.2016.01.170
38. Sinvani L, Kozikowski A, Patel V, Mulvany C, Smilios C, Qiu G, et al. Measuring functional status in hospitalized older adults through electronic health record documentation. South Med J. (2018) 111:220–5. doi: 10.14423/SMJ.0000000000000788
39. Leigh AE, McCall J, Burke RV, Rome R, Raines AM. Predictors of functional dependence after COVID-19: a retrospective examination among veterans. Am J Phys Med Rehabil. (2021) 100:34–8. doi: 10.1097/PHM.0000000000001614
40. Gilbert T, Neuburger J, Kraindler J, Keeble E, Smith P, Ariti C, et al. Development and validation of a Hospital Frailty Risk Score focusing on older people in acute care settings using electronic hospital records: an observational study. Lancet (London, England). (2018) 391:1775–82. doi: 10.1016/S0140-6736(18)30668-8
41. Harding BN, Floyd JS, Scherrer JF, Salas J, Morley JE, Farr SA, et al. Methods to identify dementia in the electronic health record: Comparing cognitive test scores with dementia algorithms. Healthc (Amst). (2020) 8:100430. doi: 10.1016/j.hjdsi.2020.100430
42. Palakshappa JA, Callahan KE, Pajewski NM, Files DC, Willard JJ, Williamson JD. Detection of cognitive impairment after critical illness with the medicare annual wellness visit: a cohort study. Ann Am Thorac Soc. (2021) 18:1702–7. doi: 10.1513/AnnalsATS.202011-1434OC
43. Amra S, O'Horo JC, Singh TD, Wilson GA, Kashyap R, Petersen R, et al. Derivation and validation of the automated search algorithms to identify cognitive impairment and dementia in electronic health records. J Crit Care. (2017) 37:202–5. doi: 10.1016/j.jcrc.2016.09.026
44. Carr MJ, Steeg S, Webb RT, Kapur N, Chew-Graham CA, Abel KM, et al. Effects of the COVID-19 pandemic on primary care-recorded mental illness and self-harm episodes in the UK: a population-based cohort study. Lancet Public Health. (2021) 6:e124–35. doi: 10.1016/S2468-2667(20)30288-7
45. Chen CK, Nehrig N, Wash L, Wang B. The impact of brief dynamic interpersonal therapy (DIT) on veteran depression and anxiety. Psychotherapy (Chic). (2020) 57:464–8. doi: 10.1037/pst0000282
46. Bishop TM, Walsh PG, Ashrafioun L, Lavigne JE, Pigeon WR. Sleep, suicide behaviors, and the protective role of sleep medicine. Sleep Med. (2020) 66:264–70. doi: 10.1016/j.sleep.2019.07.016
47. May AD, Parker AM, Caldwell ES, Hough CL, Jutte JE, Gonzalez MS, et al. Provider-Documented anxiety in the ICU: prevalence, risk factors, and associated patient outcomes. J Intensive Care Med. (2021) 36:1424–30. doi: 10.1177/0885066620956564
48. Harrington KM, Quaden R, Stein MB, Honerlaw JP, Cissell S, Pietrzak RH, et al. Validation of an electronic medical record-based algorithm for identifying posttraumatic stress disorder in U.S. Veterans J Trauma Stress. (2019) 32:226–37. doi: 10.1002/jts.22399
49. Tuepker A, Zickmund SL, Nicolajski CE, Hahm B, Butler J, Weir C, et al. Providers' note-writing practices for post-traumatic stress disorder at five United States veterans affairs facilities. J Behav Health Serv Res. (2016) 43:428–42. doi: 10.1007/s11414-015-9472-9
50. Meltzer EC, Averbuch T, Samet JH, Saitz R, Jabbar K, Lloyd-Travaglini C, et al. Discrepancy in diagnosis and treatment of post-traumatic stress disorder (PTSD): treatment for the wrong reason. J Behav Health Serv Res. (2012) 39:190–201. doi: 10.1007/s11414-011-9263-x
51. Schjorring OL, Lange T, Krag M, Andersen-Ranberg NC, Meyhoff TS, Marker S, et al. Interactions in clinical trials: protocol and statistical analysis plan for an explorative study of four randomised ICU trials on use of pantoprazole, oxygenation targets, haloperidol and intravenous fluids. Acta Anaesthesiol Scand. (2022) 66:156–62. doi: 10.1111/aas.13990
52. Litton E, Anstey M, Broadhurst D, Chapman A, Currie A, Ferrier J, et al. Early and sustained Lactobacillus plantarum probiotic therapy in critical illness: the randomised, placebo-controlled, restoration of gut microflora in critical illness trial (ROCIT). Intensive Care Med. (2021) 47:307–15. doi: 10.1007/s00134-020-06322-w
53. Venkatesh B, Finfer S, Cohen J, Rajbhandari D, Arabi Y, Bellomo R, et al. Adjunctive glucocorticoid therapy in patients with septic shock. N Engl J Med. (2018) 378:797–808. doi: 10.1056/NEJMoa1705835
54. Target Investigators ftACTG, Chapman M, Peake SL, Bellomo R, Davies A, Deane A, et al. Energy-Dense vs. routine enteral nutrition in the critically Ill. N Engl J Med. (2018) 379:1823–34. doi: 10.1056/NEJMoa1811687
55. Self WH, Semler MW, Wanderer JP, Ehrenfeld JM, Byrne DW, Wang L, et al. Saline versus balanced crystalloids for intravenous fluid therapy in the emergency department: study protocol for a cluster-randomized, multiple-crossover trial. Trials. (2017) 18:178. doi: 10.1186/s13063-017-1923-6
56. Ge F, Jiang J, Wang Y, Wan M, Zhang W. Mapping the presence of anxiety symptoms in adults with major depressive disorder. Front Psychiatry. (2021) 12:595418. doi: 10.3389/fpsyt.2021.595418
57. Doing-Harris K, Bray BE, Thackeray A, Shah RU, Shao Y, Cheng Y, et al. Development of a cardiac-centered frailty ontology. J Biomed Semantics. (2019) 10:3. doi: 10.1186/s13326-019-0195-3
58. Agaronnik N, Lindvall C, El-Jawahri A, He W, Iezzoni L. Use of natural language processing to assess frequency of functional status documentation for patients newly diagnosed with colorectal cancer. JAMA Oncol. (2020) 6:1628–30. doi: 10.1001/jamaoncol.2020.2708
59. Le Glaz A, Haralambous Y, Kim-Dufor DH, Lenca P, Billot R, Ryan TC, et al. Machine learning and natural language processing in mental health: systematic review. J Med Internet Res. (2021) 23:e15708. doi: 10.2196/15708
60. Edgcomb JB, Zima B. Machine learning, natural language processing, and the electronic health record: innovations in mental health services research. Psychiatr Serv. (2019) 70:346–9. doi: 10.1176/appi.ps.201800401
61. Marafino BJ, Park M, Davies JM, Thombley R, Luft HS, Sing DC, et al. Validation of prediction models for critical care outcomes using natural language processing of electronic health record data. JAMA Netw Open. (2018) 1:e185097. doi: 10.1001/jamanetworkopen.2018.5097
62. Tissot HC, Shah AD, Brealey D, Harris S, Agbakoba R, Folarin A, et al. Natural language processing for mimicking clinical trial recruitment in critical care: a semi-automated simulation based on the LeoPARDS trial. IEEE J Biomed Health Inform. (2020) 24:2950–9. doi: 10.1109/JBHI.2020.2977925
Keywords: critical illness, trajectory, patient important outcomes, days alive and out of hospital/health care facility (DAOH), electronic health records (EHR)
Citation: Ahmad SR, Tarabochia AD, Budahn L, Lemahieu AM, Anderson B, Vashistha K, Karnatovskaia L and Gajic O (2022) Feasibility of Extracting Meaningful Patient Centered Outcomes From the Electronic Health Record Following Critical Illness in the Elderly. Front. Med. 9:826169. doi: 10.3389/fmed.2022.826169
Received: 11 February 2022; Accepted: 11 May 2022;
Published: 06 June 2022.
Edited by:
Ernestina Menasalvas, Polytechnic University of Madrid, SpainReviewed by:
Denis Newman-Griffis, University of Pittsburgh, United StatesHamza Rayes, University of Cincinnati, United States
Copyright © 2022 Ahmad, Tarabochia, Budahn, Lemahieu, Anderson, Vashistha, Karnatovskaia and Gajic. This is an open-access article distributed under the terms of the Creative Commons Attribution License (CC BY). The use, distribution or reproduction in other forums is permitted, provided the original author(s) and the copyright owner(s) are credited and that the original publication in this journal is cited, in accordance with accepted academic practice. No use, distribution or reproduction is permitted which does not comply with these terms.
*Correspondence: Sumera R. Ahmad, ahmad.sumera@mayo.edu