- 1Department of Radiology, Children’s Hospital of Chongqing Medical University, National Clinical Research Center for Child Health and Disorders, Ministry of Education Key Laboratory of Child Development and Disorders, Chongqing Key Laboratory of Pediatrics, Chongqing, China
- 2GE Healthcare, MR Research China, Beijing, China
Objective: To explore the application of quantitative magnetic resonance imaging in the diagnosis of autism in children.
Methods: Sixty autistic children aged 2–3 years and 60 age- and sex-matched healthy children participated in the study. All the children were scanned using head MRI conventional sequences, 3D-T1, diffusion kurtosis imaging (DKI), enhanced T2*- weighted magnetic resonance angiography (ESWAN) and 3D-pseudo continuous Arterial Spin-Labeled (3D-pcASL) sequences. The quantitative susceptibility mapping (QSM), cerebral blood flow (CBF), and brain microstructure of each brain area were compared between the groups, and correlations were analyzed.
Results: The iron content and cerebral blood flow in the frontal lobe, temporal lobe, hippocampus, caudate nucleus, substantia nigra, and red nucleus of the study group were lower than those in the corresponding brain areas of the control group (P < 0.05). The mean kurtosis (MK), radial kurtosis (RK), and axial kurtosis (AK) values of the frontal lobe, temporal lobe, putamen, hippocampus, caudate nucleus, substantia nigra, and red nucleus in the study group were lower than those of the corresponding brain areas in the control group (P < 0.05). The mean diffusivity (MD) and fractional anisotropy of kurtosis (FAK) values of the frontal lobe, temporal lobe and hippocampus in the control group were lower than those in the corresponding brain areas in the study group (P < 0.05). The values of CBF, QSM, and DKI in frontal lobe, temporal lobe and hippocampus could distinguish ASD children (AUC > 0.5, P < 0.05), among which multimodal technology (QSM, CBF, DKI) had the highest AUC (0.917) and DKI had the lowest AUC (0.642).
Conclusion: Quantitative magnetic resonance imaging (including QSM, 3D-pcASL, and DKI) can detect abnormalities in the iron content, cerebral blood flow and brain microstructure in young autistic children, multimodal technology (QSM, CBF, DKI) could be considered as the first choice of imaging diagnostic technology.
Clinical Trial Registration: [http://www.chictr.org.cn/searchprojen.aspx], identifier [ChiCTR2000029699].
Background
Autism spectrum disorder (ASD) is a group of neurodevelopmental disorder with the onset in early childhood, characterized by impairment in communication, and social interaction, rigidity of interests, and repetitive stereotypical behaviors (1–4). The incidence of ASD is increasing worldwide, while the etiology and pathogenesis of autism are poorly understood and no drug is available (5, 6). Children with autism are mainly treated with rehabilitation intervention, and there is no specific treatment yet. Studies have shown that early and reasonable systematic rehabilitation intervention can improve the symptoms of most children, and even obtain a basic “recovery.” The earlier the rehabilitation intervention, the better the recovery of children, which requires clinicians to accurately diagnose children with autism at an early stage (6, 7).
Currently, the early diagnosis of ASD is mainly based on the patients’ clinical manifestations, but the clinical manifestations of most ASD children are atypical and the symptoms-based early diagnosis is difficult, especially for low-age children with ASD, which eventually leads to many ASD children missing effective early intervention expectations. Therefore, there is an urgent need for early, fast, convenient and feasible diagnostic methods for ASD (8–11).
In previous studies, only a few researchers explored whether the brain blood flow or gray matter of ASD children was abnormal by 3D-pseudo continuous Arterial Spin-Labeled (3D-pcASL), quantitative susceptibility mapping (QSM) and diffusion kurtosis imaging (DKI) sequences (7, 12–15). For example, Tang et al. (13) found a decrease in iron content in some brain regions of ASD children. Mori et al. (14) confirmed that the cerebral blood flow in some brain areas of ASD children decreased. McKenna et al. (15) demonstrated that gray matter microstructure in some brain regions of autistic children was abnormal. However, they did not prove whether there is spatial overlap in brain regions with reduced iron content, cerebral blood flow perfusion, abnormal gray matter microstructure, and whether there is a correlation between them.
In this study, we performed multimodal MRI (conventional MRI sequences, ESWAN, DKI, 3D-pcASL, and 3D-T1 MRI sequences) to scan the brains of autistic children and healthy children. The quantitative maps of the QSM, DKI, and CBF MRI data and a 3D-T1 structure map of the brain were obtained through post-processing. The quantitative and structural maps of the two groups were compared and analyzed. The correlation was analyzed to obtain a specific MRI diagnostic basis of autism in children if any differences were found.
Materials and Methods
Ethics Statement
The study protocol was approved by the Human Ethics Committee of the Children’s Hospital of Chongqing Medical University (No. 2018-47). Written informed consent was obtained from the parents or guardians of all the patients before the examinations.
Patients
From June 2018 to December 2020, we recruited 65 2- to 3-year-old autistic children at the Children’s Hospital of Chongqing Medical University as the research group. All the children were tested for iron trace elements, hemoglobin, and the red blood cell volume at our hospital. The inclusion criteria of the study group were as follows: body mass index between 15 and 18; right handedness; fulfillment of the diagnostic criteria of autism in the American Diagnostic and Statistical Manual of Mental Disorders (DSM-IV); no evidence of neurological functional diseases; no evidence of other organ-associated diseases; no evidence of other diseases that may affect brain function and structure; no history of drug treatment in the past; and no abnormality in routine MRI examinations of the head. Five children snored with excessive breathing activity after sedation, resulting in image quality defects. Finally, the data of 60 children (32 male and 28 female; mean age, 2.72 years; mean body mass index, 16.89) were included in the study.
During the same period, we recruited 63 healthy children of matched age and sex as the control group, and all the children were tested for iron trace elements, hemoglobin, and the red blood cell volume at the laboratory in our hospital. The inclusion criteria of the control group were as follows: body mass index between 15 and 18; right handedness; no evidence of neurological functional diseases; no evidence of other organ-associated diseases; no evidence of other diseases that may affect brain function and structure; no abnormality in routine MRI examination of the head; iron trace elements in venous blood within the normal range (6.5–9.85 μmol/L); and a hemoglobin value and mean corpuscular volume (MCV) value in the normal range (Hb, 110–150 g/L; MCV, 80–100 fl). Three children snored and breathed with excessive motion after sedation, resulting in image quality defects. Finally, 60 children (31 male and 29 female; average age, 2.76 years; average body mass index, 16.54) were included in the study.
Imaging Data Collection
Data collection was performed in the MRI room of Children’s Hospital of Chongqing Medical University. A GE Discover MR750 3.0T MR scanner (GE Medical Systems, Milwaukee, WI, United States) and an 8-channel head and neck coil were used to scan the head with routine MRI sequences, 3D-pcASL, DKI, ESWAN, and 3D-T1 sequences. All the children were examined after sedation and sleeping in Sedation Center (sedation mode: Dexmedetomidine nasal drip, 2 ug/kg). The routine sequence included sagittal T2, transverse T2, T1 Flair, T2 Flair, and DWI; FOV, 24 cm, matrix of acquired images, 256*256, slice thickness, 5 mm, and 16 layers, total scanning time, 4 min and 32 s. 3D-pcASL: FOV, 24 cm; slice thickness, 4 mm; TR, 4628 ms; PLD, 1525 ms; slab, 1; and 36 layers; scanning time, 4 min and 29 s. DKI: FOV, 24 cm; matrix of acquired images, 128*128, slice thickness, 3 mm; TR, 4,500 ms; 43 layers; total diffusion direction, 30; and B values of 0, 1,000, 2,000; scanning time, 5 min and 20 s. ESWAN: FOV, 24 cm; slice thickness, 3 mm; TR, 81.8; flip angle, 20; TE, 4 ms; Locs per slab, 50; slab, 1; scanning time, 7 min and 47 s. 3D-T1: FOV, 24 cm; slice thickness, 1 mm; TR, 7.9 ms; flip angle, 12; TE, 3.1 ms; and 156 layers; scanning time, 3 min and 43 s. Before the children were scanned, the conventional sequence and study sequence were scanned once using a special magnetic resonance head water model (Model 2152220; MRS Sphere with Solution; GE, Milwaukee, WI, United States). When the image signals were uniform, the children were examined again.
Imaging Data Post-processing
To calculate the quantitative parameters, including the gray matter volume (GMV), white matter volume (WMV), QSM, cerebral blood flow (CBF), mean diffusivity (MD), fractional anisotropy (FA), fractional anisotropy of kurtosis (FAK), radial kurtosis (RK), mean kurtosis (MK), and axial kurtosis (AK) values of different brain regions, we followed an atlas-based image processing approach (Figure 1). Quantitative susceptibility mapping calculation was conducted with the phase ESWAN images using a publicly available implementation tool (STI Suite version 3.0).1 The CBF, MD, FA, FAK, MK, RK, and AK maps were further calculated from the 3D-pcASL and DKI data using an AW4.6 GE Workstation. Second, we performed a rigid registration between the QSM/ASL/DKI quantitative maps and 3D-T1WIs using SPM12 software2 based on MATLAB (MathWorks, Natick, MA, United States). Third, 3D-T1WIs were segmented and non-linearly normalized into the MNI space using the CAT12 toolbox3 implemented in SPM12 to obtain the tissue probability maps and normalized QSM/ASL/DKI quantitative maps. Finally, the brain was parcellated into 38 anatomical regions based on automated anatomical labeling atlas (AAL-3) for quantitative parameter extraction. The brain regional volumes, QSM, CBF, and DKI, including MD, FA, FAK, MK, AK, and RK, can be extracted by averaging the values from voxels in specific brain regions. The estimated global GMV and WMV were further normalized by correction of the intracranial volume.
Statistical Analysis
SPSS 25.0 statistical software was used, and the measurement data are expressed as mean ± standard deviation. All data were tested for normality and variance homogeneity prior to analyses, and the independent two-sample T-test was used if the data conform to the normality and variance homogeneity. The brain volume, iron content and cerebral blood flow data in the same brain area of children in the study group and control group were compared by T-test for two independent samples. The DKI data, the AK, MD, FA, FAK, MK, and RK values, in the same brain region of children in the study and control groups were compared by T-test for two independent samples. The iron, hemoglobin and red blood cell volume data of children in the study and control groups were compared by T-test for two independent samples.
Receiver operating characteristic (ROC) curve analysis was performed to evaluate the diagnostic value of ASD children. The area under the curve (AUC) of more than 0.5 indicated significant diagnostic value, and the AUC value closer to 1 was indicative of the better diagnostic value.
Results
Comparison of the Cerebral Blood Flow and Iron Content Measurement Data Between the Study and Control Groups
The CBF value and iron content of the frontal lobe, temporal lobe, hippocampus, caudate nucleus, substantia nigra and red nucleus in the study group was significantly lower than that of the corresponding brain areas in the control group (P < 0.05) (Table 1 and Figure 2).

Table 1. Comparison of the cerebral blood flow (CBF) and iron content measurement data between the study and control groups [± s, n = 60].
Comparison of the Diffusion Kurtosis Imaging Results Between the Study and Control Groups
The MK value of the frontal lobe, temporal lobe, putamen, hippocampus, caudate nucleus, substantia nigra, and red nucleus of the study group was significantly lower than that of the corresponding brain areas in the control group (P < 0.05). The RK and FA values of the frontal lobe, temporal lobe, and hippocampus in the study group were significantly lower than those in the corresponding brain regions in the control group (P < 0.05). The MD and FAK values of the frontal lobe, temporal lobe, and hippocampus in the control group were significantly lower than those in the corresponding brain regions in the study group (P < 0.05). The AK values of the frontal lobe, temporal lobe, hippocampus and caudate nucleus in the study group were significantly lower than those in the corresponding brain regions of the control group (P < 0.05) (Table 2 and Figure 3).
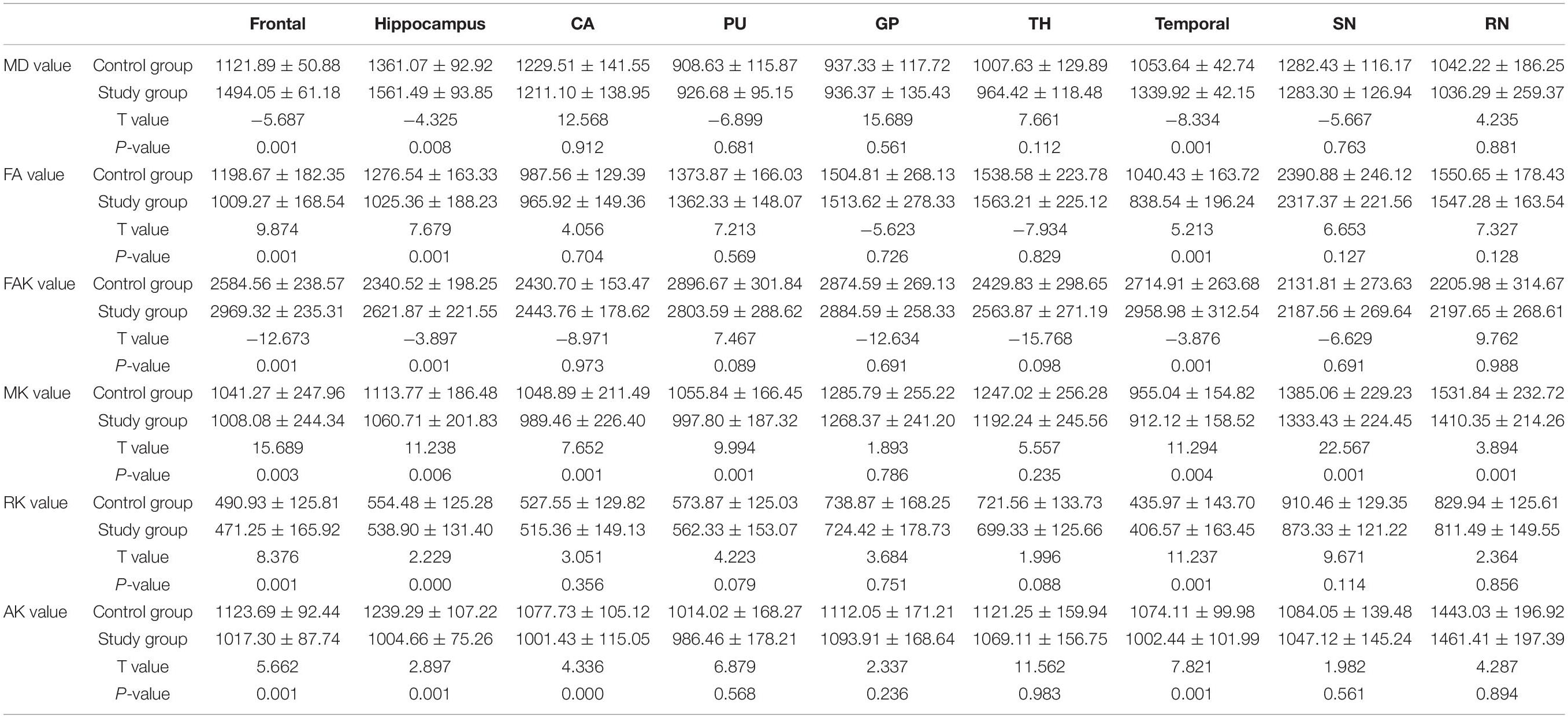
Table 2. Comparison of the diffusion kurtosis imaging results between the study and control groups [10–4 μm2/ms± s, n = 60].
Comparison of the Brain Volume Measurement Data Between the Study and Control Groups
The volume of the caudate nucleus in the study group was significantly smaller than that in the control group (P < 0.05). No significant difference was found in the volume of whole brain between the study and control groups (P > 0.05) (Table 3).

Table 3. Comparison of the brain volume measurement data between the study and control groups [cm3, ± s, n = 60].
Comparison of the Test Results of the Trace Iron, Hemoglobin Content, and Red Blood Cell Volume Between the Study and Control Groups
No significant difference was found in the trace iron, hemoglobin content and red blood cell volume between the study and control groups (P > 0.05) (Table 4).
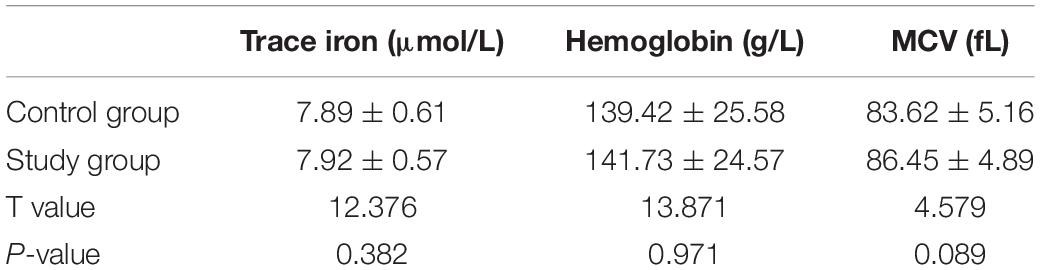
Table 4. Comparison of the test results of the trace iron, hemoglobin content, and red blood cell volume between the study and control groups [± s, n = 60].
Correlation Analysis
The values of CBF, QSM, and DKI in frontal lobe, temporal lobe and hippocampus could distinguish ASD children (AUC > 0.5, P < 0.05), among which multimodal technology (QSM, CBF, DKI) had the highest AUC (0.917) and DKI had the lowest AUC (0.642) (Table 5 and Figure 4).
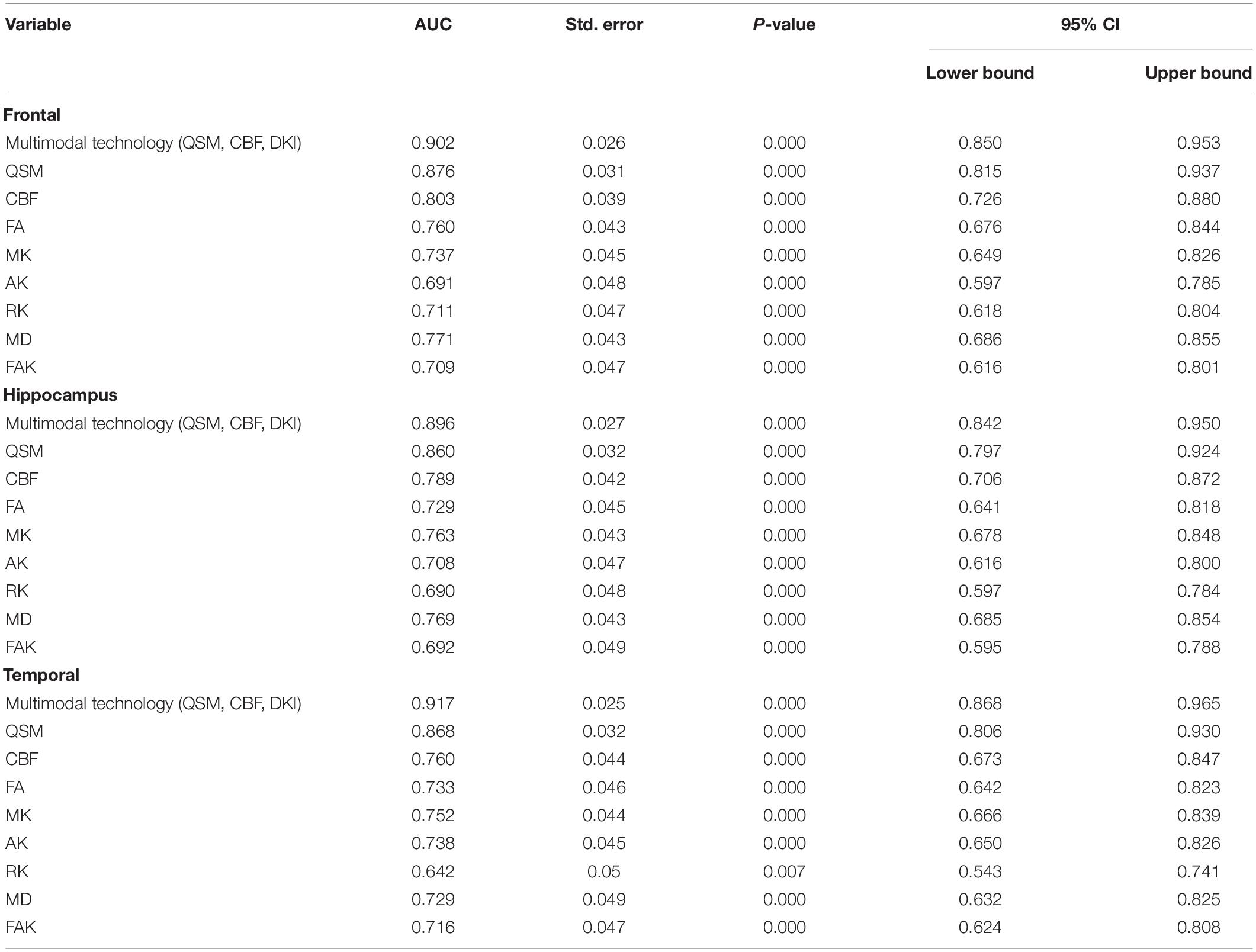
Table 5. Receiver operating characteristic (ROC) curve analysis results of quantitative susceptibility mapping (QSM), cerebral blood flow (CBF), and diffusion kurtosis imaging (DKI) values in frontal lobe, temporal lobe and hippocampus (n = 60).
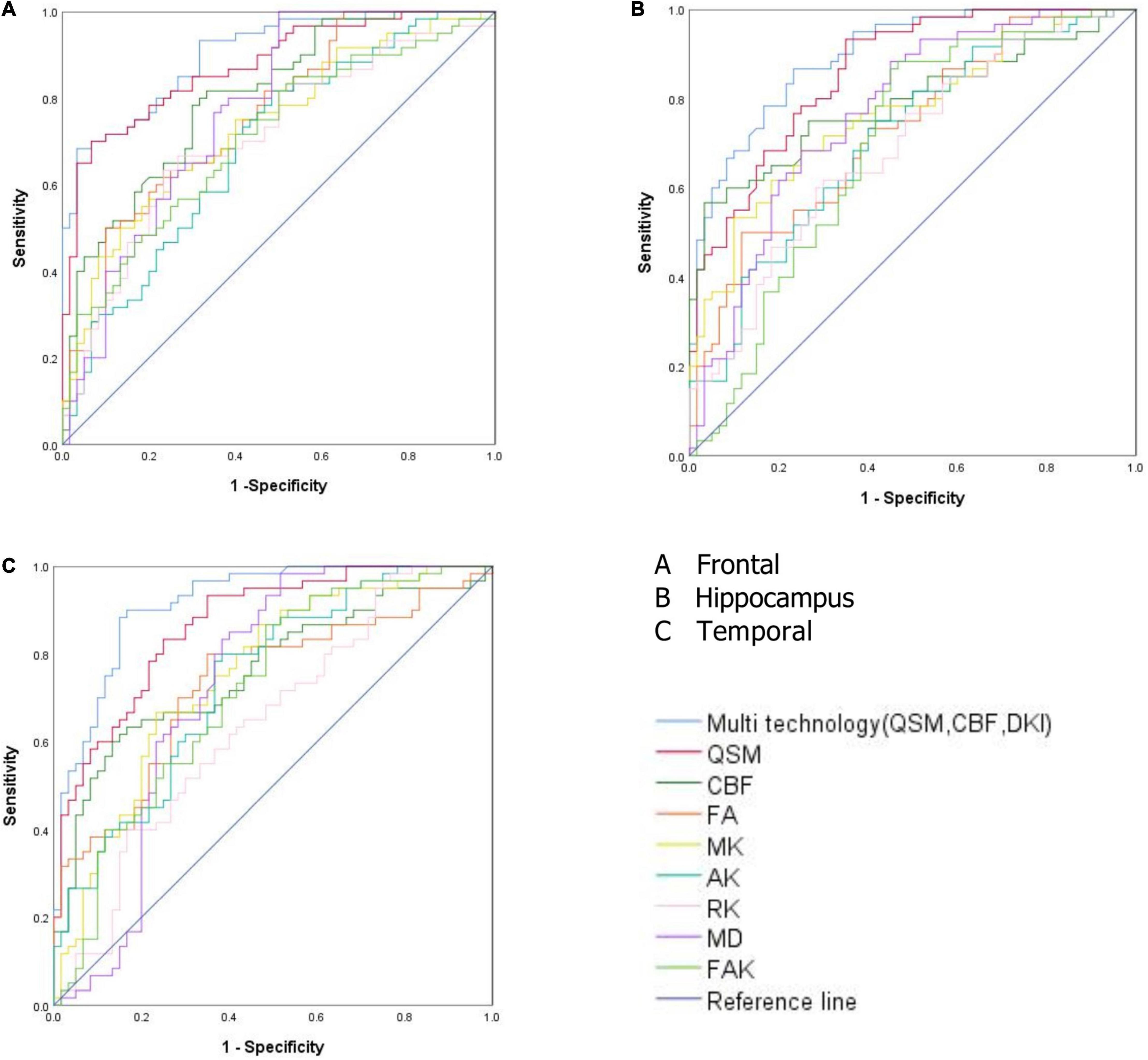
Figure 4. (A) Receiver operating characteristic (ROC) curve analysis results of quantitative susceptibility mapping (QSM), cerebral blood flow (CBF), diffusion kurtosis imaging (DKI), and multimodal technology (QSM, CBF, DKI) values in frontal lobe. (B) ROC curve analysis results of QSM, CBF, DKI, and multimodal technology (QSM, CBF, DKI) values in hippocampus. (C) ROC curve analysis results of QSM, CBF, DKI, and multimodal technology (QSM, CBF, DKI) values in temporal lobe.
Discussion
Most of the routine brain MRI results of children with autism are negative, which is not helpful for the early diagnosis of children with autism (16, 17). In this study, we performed multimodal MRI scans on the brains of children with autism, and obtained the microstructures of the brains through image post-processing to understand whether the brain microstructure of children with autism is abnormal and obtain the criteria for early objective imaging diagnosis of the brain of children with autism.
Quantitative susceptibility mapping (QSM) is a relatively new technology developed based on SWI imaging technology in recent years. Compared with traditional SWI based on magnetic susceptibility differences, QSM can detect tiny changes in the iron content in the brain and provide better specific spatial image contrast; thus, it has higher sensitivity and credibility (18–22). 3D-pcASL technology is an MR perfusion imaging technology, it uses water molecules in the blood in the artery as endogenous contrast agents for vascular perfusion imaging. The images of blood water molecules reaching the target at the threshold level for imaging before and after being labeled are collected, and the two images are subtracted to obtain the final image with blood perfusion information (23–29). The iron content and cerebral blood flow in the frontal lobe, temporal lobe, hippocampus, caudate nucleus, substantia nigra, and red nucleus in the autistic children was lower than that in the healthy children. The reason for the differences may be the decrease in the iron content in these brain regions due to insufficient cerebral blood flow in the autistic children (30, 31). In the human body, iron exists mainly in the form of hemoglobin iron and non-hemoglobin iron. Hemoglobin iron exists mainly in the form of oxygenated hemoglobin, deoxyhemoglobin, and methemoglobin in the blood, accounting for approximately 2/3 of the total iron content in the human body. The levels of myoglobin and various enzymes are very small. Non-hemoglobin iron includes ferritin and hemosiderin in blood. More than 1/3 of non-pigment iron in the brain exists in the form of ferritin. The decrease in cerebral blood flow in the brain regions described above leads to a decrease in hemoglobin iron and non-hemoglobin iron in the blood of these regions, leading to a decrease in the iron content in these above brain regions (30–32).
Diffusion kurtosis imaging (DKI) is a magnetic resonance imaging technique that reflects the non-Gaussian distribution of water molecules in brain tissue. It introduces kurtosis (K) defined in terms of probability and statistics and is used to solve the problem of fiber direction at fiber intersections, which cannot be solved by the second-order tensor, and to quantify the degree of water molecular diffusion deviating from a Gaussian distribution (33–37). The comparison of MK values of DKI parameters showed that the MK value of the frontal lobe, temporal lobe, putamen, hippocampus, caudate nucleus, substantia nigra, and red nucleus in the autistic children was lower than that of the corresponding brain regions in the healthy children. The MK value depends on the structural complexity of the tissues in the region of interest. More complex structures exhibit more significant the limitations of the diffusion of non-normally distributed water molecules and larger MK values. Therefore, the low MK value in some brain regions of the autistic children may be caused by the tight and irregular brain tissue structure and destruction of tissue structure complexity, indicating that microstructural changes may have occurred in these brain regions. The comparison of DKI parameters showed that the MD and FAK values of the frontal lobe, temporal lobe and hippocampus in autistic children were higher than those in the control group, while the FA value was lower than that in the control group. The increase in the MD value and decrease in the FA value in the brains of autistic children may indicate damaged axonal integrity due to an increase in axonal membrane permeability, axonal microstructure damage, or neurogenesis defects. The increase in the FAK value may be due to neuron remodeling and the loss of special fiber bundles, changes in fiber composition or an increase in cell permeability, which all can lead to a change in the FAK value.
The AK and RK values of the frontal lobe, temporal lobe and hippocampus in the autistic children were lower than those in the healthy children. AK and RK are the average values of diffusion kurtosis parallel and perpendicular to the long axis of the diffusion tensor, respectively. Both measures describe information related to the direction of the DKI and enable comprehensive observations of micropathological changes in biological tissue structures. AK and RK are sensitive to changes in gray matter microstructure. The decrease in AK and RK values may be due to the destruction of myelin integrity, nerve fiber density and parallelism and disorder of a microstructure. In the study, we selected only the six aforementioned parameters for the DKI study because the MD, FAK and FA values represent white matter structure parameters and MK, AK, and RK values represent gray matter parameters. The results showed that all six parameters in the frontal lobe, temporal lobe, hippocampus, and other brain regions were changed, indicating that microstructures in both gray matter and white matter in the brain regions of interest in the autistic children were changed.
The blood test results showed no significant difference in the trace iron, hemoglobin content or red blood cell volume between the autistic children and healthy children (P > 0.05). However, QSM showed that the iron content in some brain regions of the autistic children was decreased (P < 0.05). This finding indicates that the iron content in some brain regions may have begun to decline with no obvious change in blood trace element iron detected in the early stage of autism in these children and that QSM technology is more sensitive to changes in the iron content in the brain regions of autistic children. Additionally, the volume of the caudate nucleus in the autistic children was lower than that in the healthy children (P < 0.05), but no significant difference was found in other brain regions (P > 0.05). However, a decrease in cerebral blood flow occurred in all the brain areas of the autistic children with a decreased iron content. This finding suggests that the decrease in the iron content in some brain regions of the autistic children may not be related to the volume in the brain regions but to the decrease in blood flow in these brain regions. The results show that the frontal lobe, temporal lobe and hippocampus not only have a decreased iron content and decreased cerebral blood flow but also have changes in the brain microstructure. Therefore, the frontal lobe, temporal lobe and hippocampal regions in autistic children may be the first to undergo changes in brain microstructure. These three brain regions may be the key areas in the brain images that can be used to diagnose autism in children.
The frontal lobe, temporal lobe, hippocampus and other brain regions in the autistic children not only showed a decreased iron content and decreased CBF but also a change in brain microstructure. We speculate that the frontal lobe, temporal lobe, and hippocampal regions may be the first areas to show microstructural changes in autistic children. The values of CBF, QSM, and DKI in frontal lobe, temporal lobe and hippocampus could distinguish ASD children (AUC > 0.5, P < 0.05), among which multimodal technology (QSM, CBF, DKI) had the highest AUC (0.917) and DKI had the lowest AUC (0.642). Therefore, these three brain regions could be served as the key areas of brain imaging in the diagnosis of ASD children, and multimodal technology (QSM, CBF, DKI) could be considered as the first choice of imaging diagnostic technology.
The frontal lobe is the most developed lobe of the brain, it determines the language ability and thinking ability of a person, if the development of the frontal lobe is damaged, these functions will be affected (38, 39). The main function of the temporal lobe is related to language comprehension, memory, and mental activity, if a person suffers from temporal lobe injury, he can’t understand the meaning of words spoken by other people and himself, and is unable to understand other people’s questions (40, 41). The hippocampus plays a role in memory and spatial orientation, and damage to the hippocampus can lead to memory decline and loss of sense of direction and perception (42). The results of the study show that the iron content and cerebral blood flow in the frontal lobe, temporal lobe, hippocampus, and other brain regions of autistic children are lower than those of healthy children, and the DKI value is also abnormal. The abnormal microstructure of the above brain regions may lead to poor development of these three brain regions in autistic children, eventually causing poor development of language, thinking, motor, comprehension, and other aspects in autistic children.
In the past, other researchers have found that the brain regions of autistic children that often undergo microstructural changes are mostly in the frontal lobe, temporal lobe, hippocampus, basal ganglia, and the above brain regions are closely related to children’s language development and intellectual development. Therefore, this study selected the above brain regions for prior study, and whether there are microstructural changes in other brain regions will be studied in the next step.
Limitations of This Study
First, we found that the autistic children who were diagnosed earlier and were given targeted treatment showed a higher attenuation rate. Therefore, we selected only 2- to 4-year-old children; other age groups were not included in the study and need to be studied in future research. Second, we collected only the data of autistic children in the local region. Thus, we did not perform a multicenter study; therefore, our data were limited to a certain extent.
In conclusion, the iron content and cerebral blood flow in the frontal lobe, temporal lobe, and hippocampus of autistic children are lower than those of healthy children, and the brain microstructures in the children with autism are changed. Quantitative magnetic resonance imaging (including QSM, 3D-pcASL, and DKI) can detect abnormalities in the iron content, cerebral blood flow and brain microstructure in young autistic children, multimodal technology (QSM, CBF, DKI) could be considered as the first choice of imaging diagnostic technology.
Data Availability Statement
Publicly available datasets were analyzed in this study. This data can be found here: https://figshare.com/s/4250d86ec77711b8c50a.
Ethics Statement
The studies involving human participants were reviewed and approved by Institutional Review Board of Children’s Hospital of Chongqing Medical University. Written informed consent to participate in this study was provided by the participants’ legal guardian/next of kin.
Author Contributions
ST: experimental design, project management, and statistics. LN: software support. XL: statistical analysis and image analysis. ZC and YZ: data acquisition and data analysis. ZP and LH: experimental design and project management. All authors contributed to the article and approved the submitted version.
Funding
This work was supported by Chongqing Municipal Education Commission (Grant/Award No. KJQN202000425) and National Clinical Research Center for Child Health and Disorders (Grant/Award No. NCRCCHD-2021-YP-07).
Conflict of Interest
LN was employed by GE Healthcare.
The remaining authors declare that the research was conducted in the absence of any commercial or financial relationships that could be construed as a potential conflict of interest.
Publisher’s Note
All claims expressed in this article are solely those of the authors and do not necessarily represent those of their affiliated organizations, or those of the publisher, the editors and the reviewers. Any product that may be evaluated in this article, or claim that may be made by its manufacturer, is not guaranteed or endorsed by the publisher.
Abbreviations
MRI, magnetic resonance imaging; DKI, diffusion kurtosis imaging; 3D-pcASL, 3D-pseudo continuous arterial spin-labeled; ASD, autism spectrum disorder; QSM, quantitative susceptibility mapping; DSM-IV, diagnostic and statistical manual of mental disordersfourth edition; MCV, mean corpuscular volume; VBM, voxel-based morphology; CBF, cerebral blood flow; ESWAN, enhanced T2*- weighted magnetic resonance angiography; MK, mean kurtosis; RK, radial kurtosis; AK, axial kurtosis; MD, mean diffusivity; FAK, fractional anisotropy of kurtosis; FA, fractional anisotropy.
Footnotes
- ^ https://people.eecs.berkeley.edu/∼chunlei.liu/software.html
- ^ http://www.fil.ion.ucl.ac.uk/spm/
- ^ http://www.neuro.uni-jena.de/cat/
References
1. Gabrielsen TP, Anderson JS, Stephenson KG, Beck J, King JB, Kellems R, et al. Functional MRI connectivity of children with autism and low verbal and cognitive performance. Mol Autism. (2018) 27:67–75. doi: 10.1186/s13229-018-0248-y
2. Cox AD, Virues-Ortega J, Julio F, Martin TL. Establishing motion control in children with autism and intellectual disability: applications for anatomical and functional MRI. J Appl Behav Anal. (2017) 50:8–26. doi: 10.1002/jaba.351
3. Peterson BS, Zargarian A, Peterson JB, Goh S, Sawardekar S, Williams SCR, et al. Hyperperfusion of frontal white and subcortical gray matter in autism spectrum disorder. Biol Psychiatry. (2019) 85:584–95. doi: 10.1016/j.biopsych.2018.11.026
4. Dell’Osso L, Lorenzi P, Carpita B. Autistic Traits and Illness Trajectories. Clin Pract Epidemiol Ment Health. (2019) 30:94–8. doi: 10.2174/1745017901915010094
5. Nuntanee S, Daranee S. Effect of motorized elephant-assisted therapy program on balance control of children with autism spectrum disorder. Occup Ther Int. (2019) 2019:5914807. doi: 10.1155/2019/5914807
6. Dean DC III, Freeman A, Lainhart J. The development of the social brain in baby siblings of children with autism. Curr Opin Psychiatry. (2020) 33:110–6. doi: 10.1097/YCO.0000000000000572
7. Sen B, Borle NC, Greiner R, Brown MRG. A general prediction model for the detection of ADHD and Autism using structural and functional MRI. PLoS One. (2018) 13:e0194856. doi: 10.1371/journal.pone.0194856
8. Tseng PT, Cheng YS, Chen YW, Stubbs B, Whiteley P, Carvalho AF, et al. Peripheral iron levels in children with autism spectrum disorders vs controls: a systematicreview and meta-analysis. Nutr Res. (2018) 50:44–52. doi: 10.1016/j.nutres.2017.11.004
9. Hrdlicka M, Sanda J, Urbanek T, Kudr M, Dudova I, Kickova S, et al. Diffusion Tensor Imaging And Tractography In Autistic, Dysphasic, And Healthy Control Children. Neuropsychiatr Dis Treat. (2019) 15:2843–52. doi: 10.2147/NDT.S219545
10. Wei H, Dibb R, Zhou Y, Sun Y, Xu J, Wang N, et al. Streaking artifact reduction for quantitative susceptibility mapping of sources with large dynamic range. NMR Biomed. (2015) 28:1294–303. doi: 10.1002/nbm.3383
11. Wei H, Xie L, Dibb R, Li W, Decker K, Zhang Y, et al. Imaging whole-brain cytoarchitecture of mouse with MRI-based quantitative susceptibility mapping. Neuroimage. (2016) 15:107–15. doi: 10.1016/j.neuroimage.2016.05.033
12. Andrews DS, Lee JK, Solomon M, Rogers SJ, Amaral DG, Nordahl CW. A diffusion-weighted imaging tract-based spatial statistics study of autism spectrum disorder in preschool-aged children. J Neurodev Disord. (2019) 11:32–40. doi: 10.1186/s11689-019-9291-z
13. Tang S, Xu Y, Liu X, Chen Z, Zhou Y, Nie L, et al. Quantitative susceptibility mapping shows lower brain iron content in children with autism. Eur Radiol. (2021) 31:2073–83. doi: 10.1007/s00330-020-07267-w
14. Mori T, Ito H, Harada M, Hisaoka S, Matsumoto Y, Goji A, et al. Multi-delay arterial spin labeling brain magnetic resonance imaging study for pediatric autism. Brain Dev. (2020) 42:315–21. doi: 10.1016/j.braindev.2020.01.007
15. McKenna F, Miles L, Donaldson J, Castellanos FX, Lazar M. Diffusion kurtosis imaging of gray matter in young adults with autism spectrum disorder. Sci Rep. (2020) 10:21465. doi: 10.1038/s41598-020-78486-w
16. Feng W, Liu G, Zeng K, Zeng M, Liu Y. A review of methods for classification and recognition of ASD using fMRI data. J Neurosci Methods. (2022) 15:109456. doi: 10.1016/j.jneumeth.2021.109456
17. Liu M, Li B, Hu D. Autism spectrum disorder studies using fMRI data and machine learning: a review. Front Neurosci. (2021) 15:697870. doi: 10.3389/fnins.2021.697870
18. Bener A, Khattab AO, Bhugra D, Hoffmann GF. Iron and vitamin D levels among autism spectrum disorders children. Ann Afr Med. (2017) 16:186–91. doi: 10.4103/aam.aam_17_17
19. Yan F, He N, Lin H, Li R. Iron deposition quantification: applications in the brain and liver. J Magn Reson Imaging. (2018) 48:301–17. doi: 10.1002/jmri.26161
20. Pivina L, Semenova Y, Doşa MD, Dauletyarova M, Bjørklund G. Iron deficiency, cognitive functions, and neurobehavioral disorders in children. J Mol Neurosci. (2019) 68:1–10. doi: 10.1007/s12031-019-01276-1
21. Gunes S, Ekinci O, Celik T. Iron deficiency parameters in autism spectrum disorder: clinical correlates and associated factors. Ital J Pediatr. (2017) 43:86–92. doi: 10.1186/s13052-017-0407-3
22. Azuma M, Hirai T, Nakaura T, Kitajima M, Yamashita S, Hashimoto M, et al. Combining quantitative susceptibility mapping to the morphometric index in differentiating between progressive supranuclear palsy and Parkinson’s disease. J Neurol Sci. (2019) 15:116443. doi: 10.1016/j.jns.2019.116443
23. Cheng Z, Zhang J, He N, Li Y, Wen Y, Xu H, et al. Radiomic features of the nigrosome-1 region of the Substantia Nigra: using quantitative susceptibility mapping to assist the diagnosis of idiopathic Parkinson’s disease. Front Aging Neurosci. (2019) 16:167. doi: 10.3389/fnagi.2019.00167
24. Zhang S, Nguyen TD, Hurtado Rúa SM, Kaunzner UW, Pandya S, Kovanlikaya I, et al. Quantitative susceptibility mapping of time-dependent susceptibility changes in multiple sclerosis lesions. AJNR Am J Neuroradiol. (2019) 40:987–93. doi: 10.3174/ajnr.A6071
25. Kaunzner UW, Kang Y, Zhang S, Morris E, Yao Y, Pandya S, et al. Quantitative susceptibility mapping identifies inflammation in a subset of chronic multiple sclerosis lesions. Brain. (2019) 142:133–45. doi: 10.1093/brain/awy296
26. Arabi M, Saberi Kakhki A, Sohrabi M, Soltani Kouhbanani S, Jabbari Nooghabi M. Is visuomotor training an effective intervention for children with autism spectrum disorders? Neuropsychiatr Dis Treat. (2019) 8:3089–102. doi: 10.2147/NDT.S214991
27. Tang S, Liu X, He L, Liu B, Qin B, Feng C. Application of a 3D pseudocontinuous arterial spin-labeled perfusion MRI scan combined with a postlabeling delay value in the diagnosis of neonatal hypoxic-ischemic encephalopathy. PLoS One. (2019) 14:e0219284. doi: 10.1371/journal.pone.0219284
28. Tang S, Liu X, He L, Liu B, Qin B, Feng C. Application of postlabeling delay time in 3-dimensional pseudocontinuous arterial spin-labeled perfusion imaging in normal children. J Comput Assist Tomogr. (2019) 43:697–707. doi: 10.1097/RCT.0000000000000911
29. Morana G, Piccardo A, Tortora D, Puntoni M, Severino M, Nozza P, et al. Grading and outcome prediction of pediatric diffuse astrocytic tumors with diffusion and arterial spin labeling perfusion MRI in comparison with 18F-DOPA PET. Eur J Nucl Med Mol Imaging. (2017) 44:2084–93. doi: 10.1007/s00259-017-3777-2
30. Wong AM, Yeh CH, Liu HL, Wu TW, Lin KL, Wang HS, et al. Arterial spin-labeling perfusion imaging of children with subdural hemorrhage: perfusion abnormalities in abusive head trauma. J Neuroradiol. (2017) 44:281–7. doi: 10.1016/j.neurad.2017.02.003
31. Boulouis G, Dangouloff-Ros V, Boccara O, Garabedian N, Soupre V, Picard A, et al. Arterial spin-labeling to discriminate pediatric cervicofacial soft-tissue vascular anomalies. AJNR Am J Neuroradiol. (2017) 38:633–8. doi: 10.3174/ajnr.A5065
32. Hu HH, Li Z, Pokorney AL, Chia JM, Stefani N, Pipe JG, et al. Assessment of cerebral blood perfusion reserve with acetazolamide using 3D spiral ASL MRI: preliminary experience in pediatric patients. Magn Reson Imaging. (2017) 35:132–40. doi: 10.1016/j.mri.2016.08.019
33. Zeng Q, Jiang B, Shi F, Ling C, Dong F, Zhang J, et al. 3D Pseudocontinuous arterial spin-labeling MR imaging in the preoperative evaluation of gliomas. AJNR Am J Neuroradiol. (2017) 38:1876–83. doi: 10.3174/ajnr.A5299
34. Andica C, Kamagata K, Hatano T, Saito Y, Ogaki K, Hattori N, et al. Biomarkers of degenerative brain disorders derived from diffusion imaging. J Magn Reson Imaging. (2020) 52:1620–36. doi: 10.1002/jmri.27019
35. Arab A, Wojna-Pelczar A, Khairnar A, Szabó N, Ruda-Kucerova J. Principles of diffusion kurtosis imaging and its role in early diagnosis of neurodegenerative disorders. Brain Res Bull. (2018) 139:91–8. doi: 10.1016/j.brainresbull.2018.01.015
36. Kamagata K, Andica C, Hatano T, Ogawa T, Takeshige-Amano H, Ogaki K, et al. Advanced diffusion magnetic resonance imaging in patients with Alzheimer’sand Parkinson’s diseases. Neural Regen Res. (2020) 15:1590–600.
37. Abdalla G, Dixon L, Sanverdi E, Machado PM, Kwong JSW, Panovska-Griffiths J, et al. The diagnostic role of diffusional kurtosis imaging in glioma grading and differentiation of gliomas from other intra-axial brain tumours: a systematic review with critical appraisal and meta-analysis. Neuroradiology. (2020) 62:791–802. doi: 10.1007/s00234-020-02425-9
38. Bedini M, Baldauf D. Structure, function and connectivity fingerprints of the frontal eye field versus the inferior frontal junction: a comprehensive comparison. Eur J Neurosci. (2021) 54:5462–506. doi: 10.1111/ejn.15393
39. Dixon BJ, Kumar J, Danielmeier C. Frontal neural metabolite changes in schizophrenia and their association with cognitive control: a systematic review. Neurosci Biobehav Rev. (2022) 1:224–47. doi: 10.1016/j.neubiorev.2021.11.010
40. Herlin B, Navarro V, Dupont S. The temporal pole: from anatomy to function-A literature appraisal. J Chem Neuroanat. (2021) 4:101925. doi: 10.1016/j.jchemneu.2021.101925
41. Braunsdorf M, Blazquez Freches G, Roumazeilles L, Eichert N, Schurz M, Uithol S, et al. Does the temporal cortex make us human? A review of structural and functional diversity of the primate temporal lobe. Neurosci Biobehav Rev. (2021) 12:400–10. doi: 10.1016/j.neubiorev.2021.08.032
Keywords: children, autism, iron, magnetic resonance imaging, brain
Citation: Tang S, Nie L, Liu X, Chen Z, Zhou Y, Pan Z and He L (2022) Application of Quantitative Magnetic Resonance Imaging in the Diagnosis of Autism in Children. Front. Med. 9:818404. doi: 10.3389/fmed.2022.818404
Received: 25 January 2022; Accepted: 26 April 2022;
Published: 12 May 2022.
Edited by:
Amit Khairnar, National Institute of Pharmaceutical Education and Research, Ahmedabad, IndiaReviewed by:
Vinod Tiwari, Indian Institute of Technology (BHU), IndiaEva Drazanova, Institute of Scientific Instruments (ASCR), Czechia
Nikoletta Szabó, University of Szeged, Hungary
Copyright © 2022 Tang, Nie, Liu, Chen, Zhou, Pan and He. This is an open-access article distributed under the terms of the Creative Commons Attribution License (CC BY). The use, distribution or reproduction in other forums is permitted, provided the original author(s) and the copyright owner(s) are credited and that the original publication in this journal is cited, in accordance with accepted academic practice. No use, distribution or reproduction is permitted which does not comply with these terms.
*Correspondence: Zhengxia Pan, cGFuemhlbmd4aWEwMDVAc2luYS5jb20=; Ling He, NDgwMDU2QGhvc3BpdGFsLmNxbXUuZWR1LmNu