- 1Department of Internal Medicine, Dow University of Health Sciences, Karachi, Pakistan
- 2Department of Internal Medicine, Dr. Sampurnanand Medical College, Jodhpur, Rajasthan, India
- 3Department of Internal Medicine, Mayo Clinic Health System, Mankato, MN, United States
- 4Division of Nephrology and Hypertension, Mayo Clinic, Rochester, MN, United States
- 5Department of Pulmonology, Texas A&M University College of Medicine, Bryan, TX, United States
- 6Department of Anesthesiology, Mayo Clinic, Rochester, MN, United States
Background: Early hospital readmission (EHR) within 30 days after kidney transplantation is a significant quality indicator of transplant centers and patient care. This meta-analysis aims to evaluate the incidence, predictors, and outcomes of EHR after kidney transplantation.
Methods: We comprehensively searched the databases, including PubMed, Cochrane CENTRAL, and Embase, from inception until December 2021 to identify studies that assessed incidence, risk factors, and outcome of EHR. The outcomes included death-censored graft failure and mortality. Data from each study were combined using the random effect to calculate the pooled incidence, mean difference (MD), odds ratio (OR), and hazard ratio (HR) with 95% confidence interval (CI).
Results: A total of 17 studies were included. The pooled EHR incidence after kidney transplant was 24.4% (95% CI 21.7–27.3). Meta-analysis showed that recipient characteristics, including older recipient age (MD 2.05; 95% CI 0.90–3.20), Black race (OR 1.31; 95% CI 1.11, 1.55), diabetes (OR 1.32; 95% CI 1.22–1.43), and longer dialysis duration (MD 0.85; 95% CI 0.41, 1.29), donor characteristics, including older donor age (MD 2.02; 95% CI 0.93–3.11), and transplant characteristics, including delayed graft function (OR 1.75; 95% CI 1.42–2.16) and longer length of hospital stay during transplantation (MD 1.93; 95% CI 0.59–3.27), were significantly associated with the increased risk of EHR. EHR was significantly associated with the increased risk of death-censored graft failure (HR 1.70; 95% CI 1.43–2.02) and mortality (HR 1.46; 95% CI 1.27–1.67) within the first year after transplantation.
Conclusion: Almost one-fourth of kidney transplant recipients had EHR within 30 days after transplant, and they had worse post-transplant outcomes. Several risk factors for EHR were identified. This calls for future research to develop and implement for management strategies to reduce EHR in high-risk patients.
Introduction
Kidney transplantation is the best renal replacement therapy option for end-stage kidney disease patients. Kidney transplant recipients have a higher long-term survival and quality of life than those who remains on dialysis (1, 2). Despite the advances in kidney transplantation and post-transplant care, hospital readmission is still frequent. Kidney transplant recipients are at higher risk of readmission given more comorbidity burden and vulnerability to complications (3, 4).
Early hospital readmission (EHR), defined as any hospitalization within 30 days of discharge following kidney transplantation, is a significant quality indicator of transplant centers and patient care (5). EHR is related to an increased morbidity, decreased quality of life, and higher medical expenditure and resource utilization (6). Reduced reimbursements from Medicare for hospitals with higher-than-expected readmission rates have been implemented due to recent policy changes aimed to reduce avoidable hospital readmissions and to improve health outcomes while reducing medical expenditure (6, 7). The incidence of EHR after kidney transplantation reported in the literature is variable. Different risk factors for EHR after kidney transplantation have been described (5, 8–10). Recognizing the risk factors for EHR is critical for identifying kidney transplant recipients who may benefit from additional post-transplant surveillance and the development of new strategies to reduce EHR.
The objective of this meta-analysis was to determine the incidence of EHR, identify the risks factors for EHR, and assess the impact of EHR on post-transplant outcomes in kidney transplant recipients.
Materials and methods
This article has been reported in accordance with the Preferred Reporting Items for Systematic Reviews and Meta-Analysis (PRISMA) guidelines (11).
Data sources and search strategy
We conducted a systematic literature search for relevant articles in the databases, including Pubmed, Embase, and Cochrane CENTRAL, using a comprehensive search strategy from inception until December 20th, 2021. The combination of the following MeSH keywords was used: “renal transplant,” “kidney transplant,” “readmission,” “early hospital readmission,” “30-day readmission,” “incidence,” “rate,” “predictor,” “risk factors,” and “association.” The detailed search strategy is presented in Supplementary Table 1.
Study selection and inclusion criteria
We included studies that reported the incidence, predictors, or outcomes of EHR after kidney transplantation. EHR was defined as 30-day readmission to any institution, due to any cause, after kidney transplantation. We excluded studies with (1) readmission >30 days after kidney transplantation, (2) combined kidney transplantations with other organs, (3) no outcomes of interest, and (4) reviews and letters. Duplicated studies retrieved from the systemic search were identified and eliminated using Endnote (Clarivate Analytics, Thomson Reuters Corporation, Philadelphia, Pennsylvania). The articles were screened based on titles and abstracts by two independent researchers (KI, AI) and subsequently assessed for relevance by reviewing full-text articles. References of the articles were also screened to identify additional studies.
The potential of sample dependence arises when multiple papers included in the review report findings from analyses on the same cohort of patients. When the studies had overlapping periods, the potential for sample dependence was minimized by the selection of studies with the longest period of data collection as the representative study for that cohort, for each variable. However, when two or more studies had the same period of data collection, the study with higher methodological quality was selected as the representative study.
Data extraction and outcomes
Two independent researchers (KI and AI) extracted data from the eligible articles. The following information was extracted: name of the first author, year of publication, study design, country of origin, sample size, subject demographics, comorbidities, and incidence of EHR. Data regarding the risk factors for EHR included the following recipient characteristics: age, gender, black race, body mass index (BMI), diabetes, prior dialysis, and dialysis duration; donor characteristics: age, donor type, and expanded donor criteria; and transplant characteristics: delayed graft function (DGF) and length of hospital stay during transplantation. The outcomes of EHR included death-censored graft failure and mortality within 1 year after kidney transplant. Raw data and adjusted estimates were extracted.
For studies that provided medians and ranges instead of means and standard deviations or provided only means in the absence of standard deviations, the means and/or standard deviations were calculated using the formula described by Hozo et al. (12). Some studies reported readmissions within 30 days of the procedure and others reported those within 30 days of discharge, readmissions data were extracted according to either definition, and the definition used by each study was recorded.
Statistical analysis and quality assessment
We utilized Review Manager v.5.3 (The Nordic Cochrane Center, The Cochrane Collaboration, 2014) and MedCalc v 20.027 to perform all the analyses. A random-effects model was used to calculate the Mantel Haenszel odds ratios (OR) for dichotomous variables and mean difference (MD) for continuous variables. Adjusted estimates were reported using inverse variance adjusted Hazard ratios (aHR). All estimates were reported with a confidence interval (CI) of 95% and a p-value < 0.05 was considered significant in all cases. We examined the correlation between the risk factors and EHR; risk factors reported in 3 or more studies were statistically analyzed. When available, risk factors based on multivariate analysis were also collected. To rule out the possibility of any single study disproportionately affecting the results, a leave-one-out sensitivity analysis was carried out by removing one study at a time. The quality appraisal of the included studies was performed by using the Newcastle–Ottawa Quality Assessment Scale (13). Each study was graded as: low bias risk (8–9 points), moderate bias risk (5–7 points), or significant bias risk (0–4 points).
Results
Literature search and baseline characteristics
The initial search strategy identified a total of 700 potentially relevant articles. After excluding the duplicates, 408 articles were screened for relevance based on their titles and abstracts. Out of these, 52 full-text articles that aligned with the objective of the manuscript were reviewed. Ultimately, 17 studies were included in the final analysis, out of which twelve were cohort studies (10 retrospective, 1 prospective, and 1 ambispective) and one was a case-control study (5, 8–10, 14–25). Supplementary Figure 1 presents the PRISMA flowchart outlining the search process. Table 1 summarizes the study characteristics of the included articles and the causes of EHR. The results of the meta-analysis of potential risk factors for EHR are presented in Table 2. Figure 1 illustrates the results of all pooled analyses, while Supplementary Figures 2–19 present the individual plots of each potential risk factor of EHR.
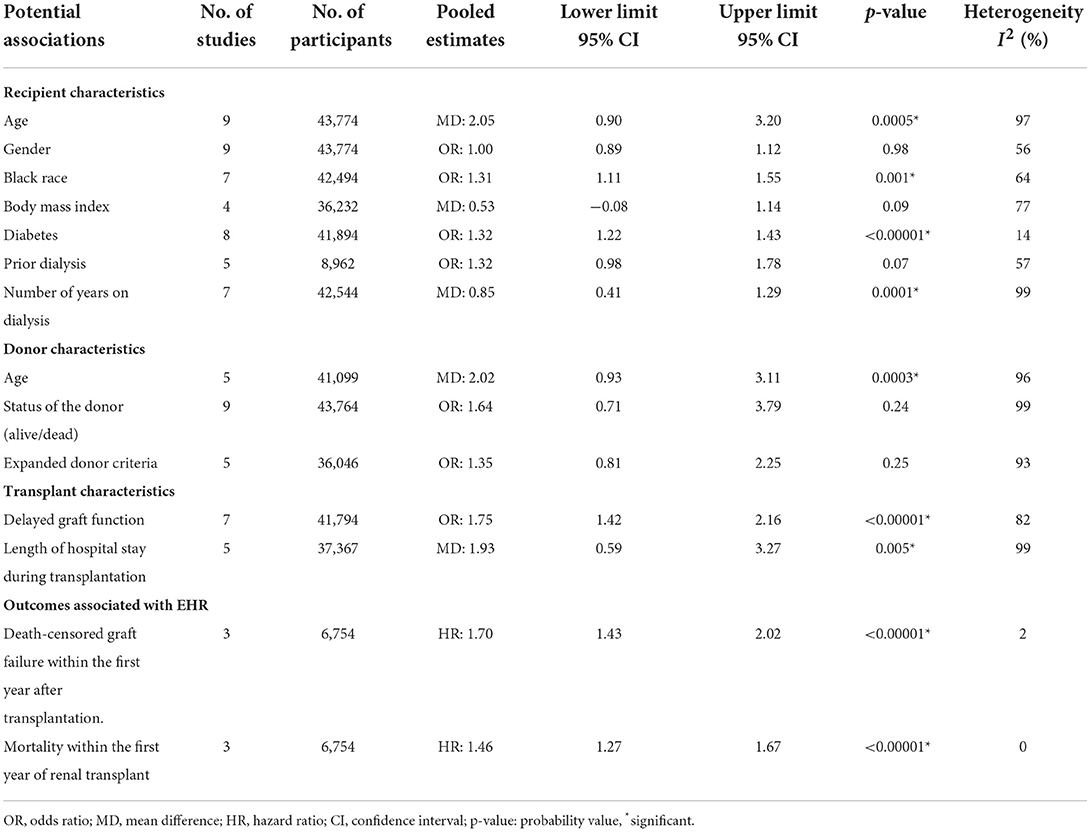
Table 2. Meta-analysis of the risk factors and outcomes associated with early hospital readmission (30-day) after kidney transplantation.
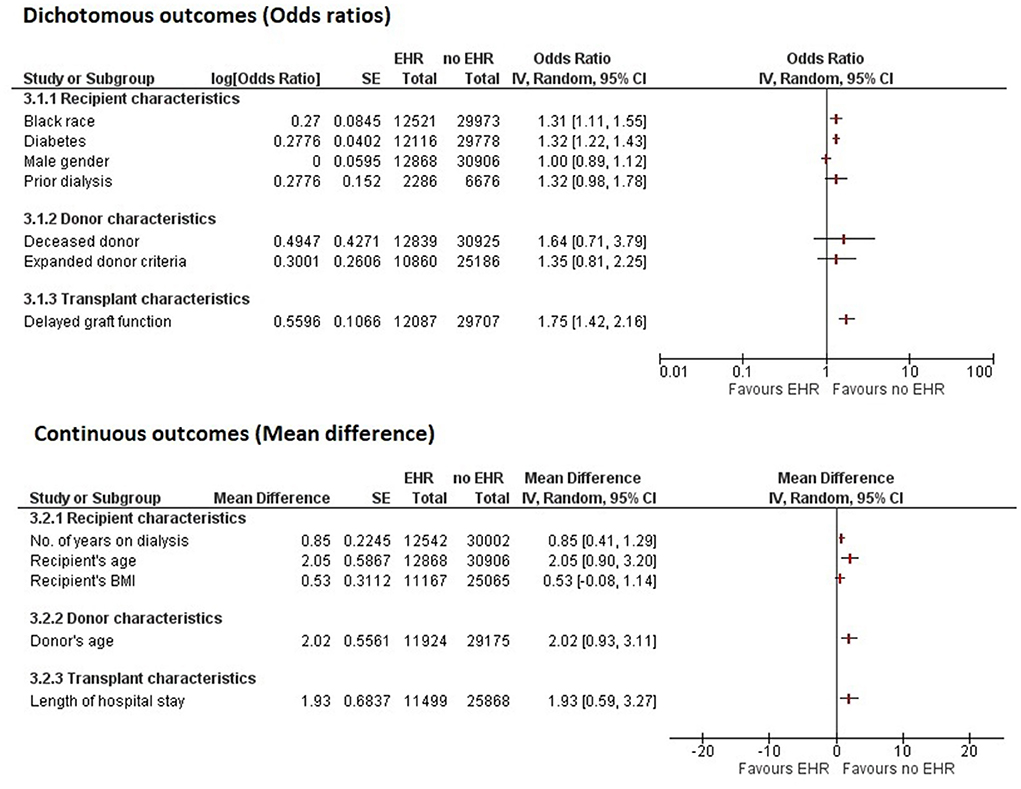
Figure 1. Forest plot summarizing the pooled analyses of all potential factors associated with early hospital readmission (30-day) after kidney transplantation. IV, inverse variance; SE, standard error; CI, confidence interval; HER, early hospital readmission.
Quality assessment and publication bias
The methodological quality assessment of included studies (Supplementary Table 2) showed that seven studies had low risk of bias, while 10 had moderate risk of bias. Therefore, all the studies were eligible for quantitative analysis. The funnel plots of publication bias are illustrated in Supplementary Figures 20, 21. There was no significant publication bias among all the outcomes, and the individual p-values of Begg-Mazumdar's rank correlation test and Egger's regression test are presented in Supplementary Table 3.
Results of meta-analysis
Incidence of early hospital readmission
A total of 16 studies reported the incidence of EHR after kidney transplantation in 26,285 out of total 87,124 transplant recipients. The pooled incidence of EHR in kidney transplant recipients was 24.4% [95% CI = 21.7–27.3 %; I2 = 98.26%; Figure 2).
Predictors
Recipient characteristics
Recipient characteristics assessed across included studies were age, gender, black race, body mass index, diabetes, prior dialysis, and number of years on dialysis. Meta-analysis revealed that recipient's older age (MD = 2.05 [95% CI 0.90, 3.20]; p = 0.0005; I2 = 97%), black race (OR = 1.31 [95% CI 1.11, 1.55]; p = 0.001; I2 = 64%), diabetes (OR = 1.32 [95% CI 1.22, 1.43]; p < 0.00001; I2 = 14%), and longer dialysis duration (MD = 0.85 [95% CI 0.41, 1.29]; p = 0.0001; I2 = 99%) were significantly associated with increased EHR (Figure 1, Supplementary Figures 2–5). However, no significant association of EHR was found with recipient's gender (OR = 1.00 [95% CI 0.89, 1.12]; p = 0.98; I2 = 56%), body mass index (MD = 0.53 [95% CI −0.08, 1.14]; p = 0.09; I2 = 77%), and prior dialysis (OR = 1.32 [95% CI 0.98, 1.78]; p = 0.07; I2 = 57%) (Figure 1, Supplementary Figures 6–8).
On pooling studies that reported adjusted data, we observed that recipient's older age (MD = 1.16 [95% CI 1.00, 1.35]; p = 0.05; I2 = 89%) and longer dialysis duration (MD = 1.01 [95% CI 1.00, 1.02]; p = 0.04; I2 = 76%) remained significantly associated with EHR (Supplementary Figures 9, 10).
Donor characteristics and transplant characteristics
The meta-analyzed donor characteristics included older age, donor type, and expanded donor criteria. Older donor age was significantly associated with increased EHR (MD = 2.02 [95% CI 0.93, 3.11]; p = 0.0003; I2 = 96%) (Figure 1, Supplementary Figure 11). However, deceased donor (OR = 1.64 [95% CI 0.71, 3.79]; p = 0.24; I2 = 99%) and expanded donor criteria (OR = 1.35 [95% CI 0.81, 2.25]; p = 0.25; I2 = 93%) were not significantly associated with EHR (Figure 1, Supplementary Figures 12, 13).
Transplant characteristics, including delayed graft function (OR = 1.75 [95% CI 1.42, 2.16]; p < 0.00001; I2 = 82%) and longer length of hospital stay during transplantation (MD = 1.93 [95% CI 0.59, 3.27]; p = 0.0003; I2 = 99%), were significantly associated with increased EHR (Figure 1, Supplementary Figures 14, 15). On adjusted analysis, delayed graft function (aOR = 1.43 [95% CI 1.11, 1.85]; p = 0.006; I2 = 74%) and longer length of hospital stay (aOR = 1.20 [95% CI 1.07, 1.36]; p = 0.002; I2 = 91%) and remained significantly associated with increased EHR (Supplementary Figures 16, 17).
The results of leave-one-out sensitivity analysis and studies that caused a significant drop in heterogeneity are shown in Supplementary Table 4. Deceased donor (OR = 1.35 [0.81, 2.25]; p = 0.0010; I2 = 68%) and expanded donor criteria (OR = 1.60 [1.11, 2.32]; p = 0.01; I2 = 66%) became significant predictors of EHR on performing leave-one-out sensitivity analysis.
Outcomes
Overall, three studies with a total of 1,460 kidney transplant recipients with EHR and 5,294 recipients without EHR documented the association of EHR with death-censored graft failure and mortality within 1 year after kidney transplant. EHR was significantly associated with increased risk of death-censored graft failure within the first year after transplantation (aHR = 1.70 [95% CI 1.43, 2.02]; p < 0.00001; I2 = 2%) (Supplementary Figure 18). EHR was significantly associated with increased mortality (aHR = 1.46 [95% CI 1.27, 1.67]; p < 0.00001; I2 = 0%) (Supplementary Figure 19).
Discussion
In the current meta-analysis, we have summarized pertinent evidence on the incidence, risk factors, and outcomes of EHR in kidney transplantation. Significant recipient-related risk factors of EHR after kidney transplantation included age, gender, black race, BMI, diabetes, and a higher number of years on dialysis. Similarly, older donor age and deceased donor were significant donor-related predictors of EHR. Delayed graft function (DGF) and a longer length of hospital stay during transplantation were significant transplant characteristics that increased the odds of EHR. Moreover, EHR was significantly associated with incident death-censored graft failure and mortality within the first year of transplantation.
Our study showed a pooled incidence of 30-day readmission of 24.4% [95% CI = 21.7–27.3 %). This is higher than the incidence of readmission previously reported in patients undergoing orthopedic procedures (5.4%), colectomy (14.7%), and pancreatic resection (19.1%) (27–29). However, other organ transplantation studies on liver (30.6%) and lung transplantation (45.4%) have reported higher incidence of EHR (30, 31).
We found an increased risk of EHR in black recipients. This finding is consistent with a greater risk of readmission in black recipients in conditions such as congestive heart failure, myocardial infarction, and pneumonia (32). Moreover, a longer time on dialysis was observed to be a significant risk factor for EHR in kidney transplantation. This could be explained by the immunological modifications, associated comorbidity burden, and physiological reserve decline (17, 33). An increase in risk of EHR and mortality due to infections have been shown in both hemodilaysis and peritoneal dialysis patients (34). Meier-Kriesche et al. reported that a longer duration of dialysis pre-transplant was associated with an increased risk of death censored graft loss (p < 0.001). Dialysis treatment of 6–12, 12, and 12–24 months was associated with a 37, 55, and 68% greater risk for death-censored graft loss, respectively (35).
In our analysis diabetes increased the risk of EHR. A previous retrospective study of 366 kidney transplant, transplant due to diabetic nephropathy was significantly associated with more and earlier post-transplant readmissions compared with patients who underwent transplants due to non-diabetic end-stage kidney disease (36). Diabetes is one of the most important factors for recurrent urinary tract infection after the transplant, and these types of infections are the most frequent in renal transplant patients (37). Moreover, Enomoto et al. (38) reported that diabetic patients were more likely to be readmitted (adjusted OR = 1.17, 95% CI 1.15–1.19; p < 0.001) compared to non-diabetics. Factors associated with readmissions included infections (9.4 vs. 7.7%), heart failure (6.0 vs. 3.1%), and chest pain/myocardial infarction (5.5 vs. 3.3%) (39).
DGF leads to an increased risk of EHR and short-term as well as long-term graft loss (39). Dialysis-dependent states and various other comorbidities are also linked with a longer length of hospital stay. Prolonged hospital stay could lead to higher chances of contracting infections. A shorter length of stay may indicate a low-risk recipient receiving a kidney from a low-risk donor (19). EHR was also significantly associated with death-censored graft failure and mortality within the first year of kidney transplant. Heldal et al. showed that DGF was an independent risk factor for death-censored graft loss in patients aged 60 years or more, while Faravardeh et al. reported that DGF and acute rejection were predictors for graft failure in younger recipients as well (40, 41). Mortality is associated with infectious, cardiovascular, and cerebrovascular complications but also depends on transplant center practices and the quality of post-transplant follow-up (5).
Our systematic review suggests patients at high risk of EHR can be identified through relevant risk factors. Patients are likely to have more than one of the above-mentioned risk factors and, therefore, predictive models should be developed to identify patients at high risk of EHR at the time of discharge. It may provide the basis of a robust risk predictive model given the number of studies included. Such patients could be selected in clinical trials to experiment with interventions to prevent early readmissions. A systematic review by Leppin et al. observed that tested interventions prevented 30-day readmissions in patients admitted to an inpatient ward for a minimum of 24 h for any medical or surgical reason. They reported that multidisciplinary strategies which increase patients' easy access to post-discharge care were the most successful (42). Quality improvement initiatives decreased the risk of readmissions by 23.7, 12.1, and 6.3% in chronic obstructive pulmonary disease, congestive heart failure patients and the general population, respectively (43, 44). Taber et al. implemented a multi-faceted strategy to improve health care value for kidney transplant patients, particularly those who developed DGF. They reported that the length of hospital stay during transplantation in DGF patients decreased from 8 to 4 days at the start of the intervention, while the national length of hospital stay during this time was 10 days (45). Moreover, focus on patient education, improved discharge planning, post-discharge phone calls, patient hotlines, and follow-up home visits also yielded positive results (46).
There are several limitations in our meta-analysis. First, most of the included articles were single-center observational studies, which limits cohort size and generalization of data. Most studies were retrospective in nature; therefore, the inherent confounding of the study type was inevitable. Second, some included articles defined EHR as readmission within 30 days from the date of the transplant procedure, while others measured EHR from the date of discharge after transplantation. Third, our study reported significant heterogeneity across various risk factors. Heterogeneity in our meta-analysis could be due to various factors that lead to effect size variability. The high I-squared values in the incidence of EHR and other risk factors in our study could be attributed to differences in sex, age, surgeon training, and time from enrollment in the included studies. Last, there was insufficient data to assess the role of type of immunosuppression on EHR after kidney transplantation.
Conclusion
This meta-analysis reported a high incidence of EHR in kidney transplant patients and summarized the evidence available on the risk factors associated with it. The most prominent risk factors include recipient's black race, diabetes, a higher number of years on dialysis, delayed graft function (DGF), and a longer length of hospital stay during transplantation. EHR is associated with death censored graft failure and mortality within the first year of transplantation. Hence, future research should aim to develop and implement predictive models for patient identification and novel management strategies to reduce EHR in patients at risk.
Data availability statement
Publicly available datasets were analyzed in this study. This data can be found here: Data is available upon reasonable request from the authors.
Author contributions
KI and SSR: concept/design. AI and SKK: data analysis/interpretation. MH, KI, AI, and SKK: drafting article. FY, TK, CT, and SS: critical revision of the article. TK, CT, and SS: approval of the article. FY: statistics. AI and SSR: data collection. All authors contributed to the article and approved the submitted version.
Conflict of interest
The authors declare that the research was conducted in the absence of any commercial or financial relationships that could be construed as a potential conflict of interest.
Publisher's note
All claims expressed in this article are solely those of the authors and do not necessarily represent those of their affiliated organizations, or those of the publisher, the editors and the reviewers. Any product that may be evaluated in this article, or claim that may be made by its manufacturer, is not guaranteed or endorsed by the publisher.
Supplementary material
The Supplementary Material for this article can be found online at: https://www.frontiersin.org/articles/10.3389/fmed.2022.1038315/full#supplementary-material
Abbreviations
EHR, early hospital readmission; DGF, delayed graft function.
References
1. Suthanthiran M, Strom TB. Renal transplantation. N Engl J Med. (1994) 331:365–76. doi: 10.1056/NEJM199408113310606
2. Wolfe RA, Ashby VB, Milford EL, Ojo AO, Ettenger RE, Agodoa LYC, et al. Comparison of mortality in all patients on dialysis, patients on dialysis awaiting transplantation, and recipients of a first cadaveric transplant. N Engl J Med. (1999) 341:1725–30. doi: 10.1056/NEJM199912023412303
3. Weinhandl ED, Snyder JJ, Israni AK, Kasiske BL. Effect of comorbidity adjustment on CMS criteria for kidney transplant center performance. Am J Transpl. (2009) 9:506–16. doi: 10.1111/j.1600-6143.2008.02527.x
4. Harhay MN, Hill AS, Wang W, Even-Shoshan O, Mussell AS, Bloom RD, et al. Measures of global health status on dialysis signal early rehospitalization risk after kidney transplantation. PLoS ONE. (2016) 11:e0156532. doi: 10.1371/journal.pone.0156532
5. Kim SH, Baird GL, Bayliss G, Merhi B, Osband A, Gohh R, et al. single-center analysis of early readmission after renal transplantation. Clin Transpl. (2019) 33:e13520. doi: 10.1111/ctr.13520
6. Jencks SF, Williams M V, Coleman EA. Rehospitalizations among patients in the medicare fee-for-service program. N Engl J Med. (2009) 360:1418–28. doi: 10.1056/NEJMsa0803563
7. Epstein AM. Revisiting readmissions—changing the incentives for shared accountability. N Engl J Med. (2009) 360:1457–9. doi: 10.1056/NEJMe0901006
8. Bergman J, Tennankore K, Vinson A. Early and recurrent hospitalization after kidney transplantation: analysis of a contemporary Canadian cohort of kidney transplant recipients. Clin Transpl. (2020) 34:e14007. doi: 10.1111/ctr.14007
9. Chu A, Zhang T, Fang Y, Yuan L, Guan X, Zhang H. Unplanned hospital readmissions after kidney transplantation among patients in Hefei, China: incidence, causes and risk factors. Int J Nurs Sci. (2020) 7:291–6. doi: 10.1016/j.ijnss.2020.05.002
10. McAdams-Demarco MA, Grams ME, Hall EC, Coresh J, Segev DL. Early hospital readmission after kidney transplantation: patient and center-level associations. Am J Transpl. (2012) 12:3283–8. doi: 10.1111/j.1600-6143.2012.04285.x
11. Page MJ, McKenzie JE, Bossuyt PM, Boutron I, Hoffmann TC, Mulrow CD, et al. The PRISMA 2020 statement: an updated guideline for reporting systematic reviews. J Clin Epidemiol. (2021) 134:178–89. doi: 10.1016/j.jclinepi.2021.02.003
12. Hozo SP, Djulbegovic B, Hozo I. Estimating the mean and variance from the median, range, and the size of a sample. BMC Med Res Methodol. (2005) 5:1–10. doi: 10.1186/1471-2288-5-13
13. Luchini C, Stubbs B, Solmi M, Veronese N. Assessing the quality of studies in meta-analyses: advantages and limitations of the Newcastle Ottawa scale. World J Meta-Anal. (2017) 5:80–4. doi: 10.13105/wjma.v5.i4.80
14. Covert KL, Fleming JN, Staino C, Casale JP, Boyle KM, Pilch NA, et al. Predicting and preventing readmissions in kidney transplant recipients. Clin Transpl. (2016) 30:779–86. doi: 10.1111/ctr.12748
15. Dols JD, Chargualaf KA, Spence AI, Flagmeier M, Morrison ML. Impact of population differences: post-kidney transplant readmissions. Nephrol Nurs J. (2018) 45:273–80.
16. Famure O, Kim ED, Au M, Zyla RE, Huang JW, Chen PX Li Y, et al. What are the burden, causes, and costs of early hospital readmissions after kidney transplantation? Prog Transpl. (2021) 31:160–7. doi: 10.1177/15269248211003563
17. Hogan J, Arenson MD, Adhikary SM Li K, Zhang X, Zhang R, Valdez JN, et al. Assessing predictors of early and late hospital readmission after kidney transplantation. Transpl Direct. (2019) 5:918. doi: 10.1097/TXD.0000000000000918
18. Kang IC, Kim IK, Son S, Ju MK. Impact of early hospital readmissions after kidney transplantation on graft function. Transpl Proc. (2018) 50:2359–62. doi: 10.1016/j.transproceed.2017.12.062
19. Lichvar AB, Patel A, Pierce D, Gimbar RP, Tzvetanov I, Benedetti E, et al. Factors influencing emergency department utilization and hospital re-admissions in a predominantly obese, racially diverse urban renal transplant population. Prog Transpl. (2021) 31:72–9. doi: 10.1177/1526924820978596
20. Luan FL, Barrantes F, Roth RS, Samaniego M. Early hospital readmissions post-kidney transplantation are associated with inferior clinical outcomes. Clin Transpl. (2014) 28:487–93. doi: 10.1111/ctr.12347
21. Lubetzky M, Yaffe H, Chen C, Ali H, Kayler LK. Early readmission after kidney transplantation: examination of discharge-level factors. Transplantation. (2016) 100:1079–85. doi: 10.1097/TP.0000000000001089
22. Naylor KL, Knoll GA, Slater J, McArthur E, Garg AX, Lam NN, et al. Risk factors and outcomes of early hospital readmission in Canadian kidney transplant recipients: a population-based multi-center cohort study. Can J Kidney Health Dis. (2021) 8:20543581211060926. doi: 10.1177/20543581211060926
23. Nguyen MC, Avila CL, Brock GN, Benedict JA, James I, El-Hinnawi A, et al. “Early” and “Late” hospital readmissions in the first year after kidney transplant at a single center. Clin Transpl. (2020) 34:e13822. doi: 10.1111/ctr.13822
24. Schucht J, Davis EG, Jones CM, Cannon RM. Incidence of and risk factors for multiple readmissions after kidney transplantation. Am Surg. (2020) 86:116–20. doi: 10.1177/000313482008600230
25. Tavares MG, Cristelli MP, Ivani de Paula M, Viana L, Felipe CR, Proença H, et al. Early hospital readmission after kidney transplantation under a public health care system. Clin Transpl. (2019) 33:e13467. doi: 10.1111/ctr.13467
26. Whitlock RS, Seals S, Seawright A, Wynn JJ, Anderson C, Earl TM. Socioeconomic factors associated with readmission after deceased donor renal transplantation. Am Surg. (2017) 83:755–60.
27. Bernatz JT, Tueting JL, Anderson PA. Thirty-day readmission rates in orthopedics: a systematic review and meta-analysis. PLoS ONE. (2015) 10:e0123593. doi: 10.1371/journal.pone.0123593
28. Fisher A V, Fernandes-Taylor S, Campbell-Flohr SA, Clarkson SJ, Winslow ER, Abbott DE, et al. 30-day readmission after pancreatic resection: a systematic review of the literature and meta-analysis. Ann Surg. (2017) 266:242–50. doi: 10.1097/SLA.0000000000002230
29. Bliss LA, Maguire LH, Chau Z, Yang CJ, Nagle DA, Chan AT, et al. Readmission after resections of the colon and rectum: predictors of a costly and common outcome. Dis Colon Rectum. (2015) 58:1164–73. doi: 10.1097/DCR.0000000000000433
30. Mumtaz K, Lee-Allen J, Porter K, Kelly S, Hanje J, Conteh LF, et al. Thirty-day readmission rates, trends and its impact on liver transplantation recipients: a national analysis. Sci Rep. (2020) 10:1–11. doi: 10.1038/s41598-020-76396-5
31. Osho AA, Castleberry AW, Yerokun BA, Mulvihill MS, Rucker J, Snyder LD, et al. Clinical predictors and outcome implications of early readmission in lung transplant recipients. J Heart Lung Transpl. (2017) 36:546–53. doi: 10.1016/j.healun.2016.11.001
32. Joynt KE, Orav EJ, Jha AK. Thirty-day readmission rates for Medicare beneficiaries by race and site of care. JAMA. (2011) 305:675–81. doi: 10.1001/jama.2011.123
33. Chopra B, Sureshkumar KK. Kidney transplantation in older recipients: Preemptive high KDPI kidney vs. lower KDPI kidney after varying dialysis vintage. World J Transpl. (2018) 8:102–9. doi: 10.5500/wjt.v8.i4.102
34. Laurin LP, Harrak H, Elftouh N, Ouimet D, Vallée M, Lafrance JP. Outcomes of infection-related hospitalization according to dialysis modality. Clin J Am Soc Nephrol. (2015) 10:817–24. doi: 10.2215/CJN.09210914
35. Meier-Kriesche HU, Port FK, Ojo AO, Rudich SM, Hanson JA, Cibrik DM, et al. Effect of waiting time on renal transplant outcome. Kidney Int. (2000) 58:1311–7. doi: 10.1046/j.1523-1755.2000.00287.x
36. Ramezani M, Ghoddousi K, Hashemi M, Khoddami-Vishte HR, Fatemi-Zadeh S, Saadat SH, et al. Diabetes as the cause of end-stage renal disease affects the pattern of post kidney transplant rehospitalizations. Transpl Proc. (2007) 39:966–9. doi: 10.1016/j.transproceed.2007.03.074
37. Schachtner T, Stein M, Reinke P. Diabetic kidney transplant recipients: Impaired infection control and increased alloreactivity. Clin Transpl. (2017) 31:e12986. doi: 10.1111/ctr.12986
38. Enomoto LM, Shrestha DP, Rosenthal MB, Hollenbeak CS, Gabbay RA. Risk factors associated with 30-day readmission and length of stay in patients with type 2 diabetes. J Diabetes Compl. (2017) 31:122–7. doi: 10.1016/j.jdiacomp.2016.10.021
39. Ojo AO, Wolfe RA, Held PJ, Port FK, Schmouder RL. Delayed graft function: risk factors and implications for renal allograft survival. Transplantation. (1997) 63:968–74. doi: 10.1097/00007890-199704150-00011
40. Heldal K, Hartmann A, Leivestad T, Svendsen M V, Foss A, Lien B, et al. Clinical outcomes in elderly kidney transplant recipients are related to acute rejection episodes rather than pretransplant comorbidity. Transplantation. (2009) 87:1045–51. doi: 10.1097/TP.0b013e31819cdddd
41. Faravardeh A, Eickhoff M, Jackson S, Spong R, KGBRla A, Issa N, et al. Predictors of graft failure and death in elderly kidney transplant recipients. Transplantation. (2013) 96:1089–96. doi: 10.1097/TP.0b013e3182a688e5
42. Leppin AL, Gionfriddo MR, Kessler M, Brito JP, Mair FS, Gallacher K, et al. Preventing 30-day hospital readmissions: a systematic review and meta-analysis of randomized trials. JAMA Intern Med. (2014) 174:1095–107. doi: 10.1001/jamainternmed.2014.1608
43. Nuckols TK, Keeler E, Morton S, Anderson L, Doyle BJ, Pevnick J, et al. Economic evaluation of quality improvement interventions designed to prevent hospital readmission: a systematic review and meta-analysis. JAMA Intern Med. (2017) 177:975. doi: 10.1001/jamainternmed.2017.1136
44. Rohde J, Joseph A, Tambedou B, Jain NK, Khan SA, Surani S, et al. Reducing 30-day all-cause acute exacerbation of chronic obstructive pulmonary disease readmission rate with a multidisciplinary quality improvement project. Cureus. (2021) 13:19917. doi: 10.7759/cureus.19917
45. Taber DJ, Pilch NA, McGillicuddy JW, Bratton CF, Lin A, Chavin KD, et al. Improving the perioperative value of care for vulnerable kidney transplant recipients. J Am Coll Surg. (2013) 216:668–78. doi: 10.1016/j.jamcollsurg.2012.12.023
Keywords: readmission, early hospital readmission, kidney transplant, incidence, predictors
Citation: Iqbal K, Hasanain M, Rathore SS, Iqbal A, Kazmi SK, Yasmin F, Koritala T, Thongprayoon C and Surani S (2022) Incidence, predictors, and outcomes of early hospital readmissions after kidney transplantation: Systemic review and meta-analysis. Front. Med. 9:1038315. doi: 10.3389/fmed.2022.1038315
Received: 06 September 2022; Accepted: 17 October 2022;
Published: 04 November 2022.
Edited by:
Vivek Jha, Imperial College London, United KingdomReviewed by:
Woo Yeong Park, Keimyung University Dongsan Medical Center, South KoreaEva Gavela Martínez, Doctor Peset University Hospital, Spain
Copyright © 2022 Iqbal, Hasanain, Rathore, Iqbal, Kazmi, Yasmin, Koritala, Thongprayoon and Surani. This is an open-access article distributed under the terms of the Creative Commons Attribution License (CC BY). The use, distribution or reproduction in other forums is permitted, provided the original author(s) and the copyright owner(s) are credited and that the original publication in this journal is cited, in accordance with accepted academic practice. No use, distribution or reproduction is permitted which does not comply with these terms.
*Correspondence: Farah Yasmin, ZmFyYWh5YXNtaW45NzJAeWFob28uY29t; Kinza Iqbal, a2luemFpcWJhMTJAZ21haWwuY29t