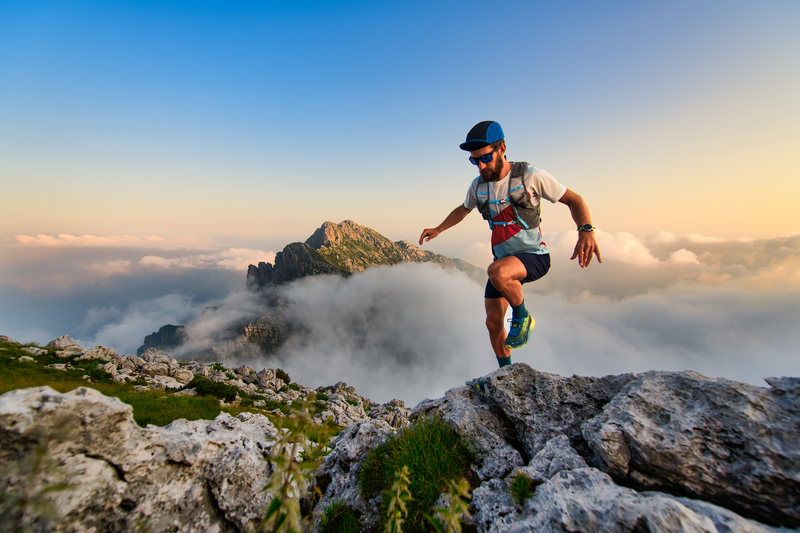
94% of researchers rate our articles as excellent or good
Learn more about the work of our research integrity team to safeguard the quality of each article we publish.
Find out more
SYSTEMATIC REVIEW article
Front. Med. , 06 October 2022
Sec. Nephrology
Volume 9 - 2022 | https://doi.org/10.3389/fmed.2022.1009358
This article is part of the Research Topic Glomerular Filtration Rate in Chronic Kidney Disease - Volume II View all 7 articles
Background: Knowledge of the biological variation of serum or plasma creatinine (Cr) and the estimated glomerular filtration rate (eGFR) is important for understanding disease dynamics in Chronic Kidney Disease (CKD). The aim of our study was to determine the magnitude of random fluctuation of eGFR by determining its reference change value (RCV).
Methods: We performed a systematic review and meta-analysis of studies on biological variation of Cr. Relevant studies were identified by systematic literature search on PubMed. Additional studies were retrieved from the European Federation of Clinical Chemistry and Laboratory Medicine (EFLM) Biological Variation Database. Random-effects meta-analysis was conducted to derive an overall estimate of intra-individual variation of creatinine (CVICr). Based on our estimate of CVICr and RCV for Cr, the RCV for the eGFR was determined.
Results: Among identified studies, 37 met our inclusion criteria. Meta-analysis of all studies yielded a CVICr of 5.2% (95% confidence interval [CI] 4.6–5.8%), however high between-study heterogeneity (I2 = 82.3%) was found. Exclusion of outliers led to a significant reduction of heterogeneity while still including 85% of all studies and resulted in a slightly lower CVICr of 5.0% (95% CI 4.7–5.4%). Assuming an analytical variation of CVA 1.1%, we found an overall RCV for eGFR of ±16.5%. After exclusion of outlier studies, we found a minimum conservative RCV for eGFR of ±12.5%.
Conclusion: The RCV of the eGFR represents a valuable tool for clinicians to discern true changes in kidney function from random fluctuation.
Serum or plasma creatinine (Cr), along with the estimated glomerular filtration rate (eGFR), is the most commonly used marker of kidney function. In everyday clinical practice, diagnostic and therapeutic decisions are based on changes in serially determined eGFR (1). According to the KDIGO Clinical Practice Guidelines for the Evaluation and Management of Chronic Kidney Disease (CKD), eGFR and albuminuria should be assessed at least annually in patients with CKD and even more frequently in individuals with a high risk of progression (2). Based on these serial measurements of eGFR, clinicians should draw conclusions on initiation or change of treatment, yet, there is considerable controversy as to what constitutes a significant change in eGFR.
Due to biological variation, serially determined eGFR may vary without necessarily corresponding to an actual change in kidney function. Therefore, an understanding of the magnitude of the biological variation of eGFR is essential for a correct interpretation of serial test results in order to discern true changes in kidney function from reversible fluctuation (3). Since eGFR is estimated based on Cr, biological variation of Cr is directly reflected in the biological variation of eGFR.
There are many sources of variation in the process of generating laboratory test results. The main sources of variation can be attributed to preanalytical, analytical (CVA) and intra-individual variation (CVI). In real-life setting, pre-analytical variation accounts for the largest part of biological variation, however, its contribution is considered negligible in the context of clinical studies where samples are collected and handled under standardized conditions. CVA occurs during the analysis of the sample and is associated with the analytical accuracy and precision. To meet analytical quality specifications CVA must be strictly controlled by clinical laboratories, but unlike preanalytical variation, it cannot be completely avoided. CVI represents physiological fluctuations of the analytes concentration around the individual’s homeostatic set point in a steady state condition. CVI is considered to be random and the homeostatic set point varies between individuals (4).
Knowledge about the magnitude of biological variation is important, as only changes exceeding this variation can be considered a true change in kidney function. This concept is formally expressed in the notion of the reference change value (RCV), a statistic originally derived by Harris et al. (5) and further developed by Fraser et al. (6). A 95% RCV represents the smallest difference of two serial results from the same individual that cannot be explained by the underlying CVI and CVA of the analyte of interest. Thus, the 95% RCV marks the limit of change needed to exceed inherent biological variation, allowing a type I error of 5% (7).
The aim of this study was to determine the magnitude of biological variation of eGFR in humans to discern reversible physiological fluctuation from true deterioration or improvement of kidney function. In addition, we aimed to investigate the impact of CKD on biological variation. For this purpose, we systematically reviewed literature on biological variation of Cr and calculated the RCV of eGFR based on reported estimates of CVI.
This systematic review was conducted in accordance with the Preferred Reporting Items for Systematic Review and Meta-Analyses (PRISMA).
PubMed was searched for literature on biological variation of Cr up to November 18th, 2021.
For the electronic literature search a combination of keywords including (“creatinine” OR “estimated glomerular filtration rate”) AND (“biological variability”) was employed. The complete search strategy is provided in the Supplementary materials. No restrictions on language, publication year or article type was made. In addition, publications on biological variation were retrieved from the European Federation of Clinical Chemistry and Laboratory Medicine (EFLM) Biological Variation Database (8) using the search term “creatinine.” Finally, reference lists of relevant articles were manually reviewed for any additional information.
The complete search results were merged and duplicates were removed using Endnote. Next, titles and abstracts were scanned by one reviewer. If the abstract was not informative, we retrieved the full text to check eligibility. During initial screening animal studies, studies in pediatric patients and studies not related to the aim of this review were excluded.
To assess methodological quality of the identified publications, they were then appraised and graded by the biological variation data critical appraisal checklist (BIVAC) (9). The checklist was published by the EFLM in 2018 and was designed to enable critical assessment of existing literature on biological variation data. The BIVAC is based on 14 quality items, each of which can be rated A, B, C, or D, indicating decreasing compliance. An overall BIVAC score is set based on the lowest grade achieved in any of the 14 items. For instance, if the lowest grade in any of the 14 quality items is C, the overall BIVAC score is C. For publications already appraised by EFLM Biological Variation Database (8), the provided score was adopted; publications not listed by the EFLM were assessed by two independent reviewers using the BIVAC. Any disagreement regarding the BIVAC score was resolved by reaching consensus between the two reviewers and referral to a third author for adjudication. Studies were excluded if they met any of the following exclusion criteria: (i) retrospective study design, (ii) biological variation data is estimated based on less than three samples per study participant, (iii) studies with an overall BIVAC grade D.
Data was extracted by one reviewer using a standardized data collection form. Among other variables, data on publication year, study design, number of subjects, age, sex, health status, study duration, sampling intervals, samples per patient, CVI, and CVA was extracted.
To derive the RCV of eGFR, we first performed a meta-analysis of CVICr. This overall estimate of intra-individual variation was then used to calculate the RCV of Cr, which we then used to derive the RCV of eGFR. A similar approach was shown by Badrick et al. (10), who transform the CVICr to the CVI of the eGFR before calculating the RCV of eGFR. As eGFR is estimated and not measured, no CVA for eGFR is available. In contrast to Badrick et al. (10) our approach does not assume a value of CVA, but rather transforms the RCV of Cr, for which a measure of CVA is available. For both equations, MDRD and CKD-EPI, the only parameter subject to physiological fluctuation is Cr. The relationship between eGFR and Cr is described by a power function. Therefore, the magnitude of the exponent of Cr directly represents the magnitude of a relative change in eGFR as response to a relative change in Cr (11). Due to this relation between eGFR and Cr, the RCV of eGFR corresponds to the RCV of Cr in the same fashion. Following the equation, a relative change of Cr beyond physiological limits proxies a relative change of eGFR beyond physiological limits.
Based on the MDRD equation, a 1% change in Cr ceteris paribus corresponds to a 1.154% change of the eGFR. In contrast to the MDRD equation, the CKD-EPI equation has different exponents for a given value of Cr, depending on whether it is greater or lower than 0.7 mg/dl in women and 0.9 mg/dl in men. Therefore, assessment of the RCV based on the CKD-EPI equation leads to four different RCVs depending on sex and Cr value. Compared to measured GFR, the CKD-EPI equation performs better than the MDRD equation, especially at higher eGFR. However, as the focus of this analysis is on assessment of progression, i.e., a change in eGFR, rather than a static measurement, our analyses are performed based on the MDRD equation delivering one RCV independent of sex and Cr.
For calculation of the RCV, a CVA of 1.1% was assumed. This value for CVA was derived from the routine laboratory of the Medical University in Innsbruck for the enzymatic method. The value is in line with CVA values reported by recent literature and corresponds to today’s analytical performance (12–14).
If the observed measurand follows a normal distribution, a symmetric, bidirectional RCV can be calculated. If the observed measurand follows a right-skewed distribution, separate limits for positive and negative changes might be more appropriate (15).
The MDRD and CKD-EPI equations for estimating eGFR, as well as the RCV formula are shown in Table 1.
Due to differences between studies, a random effects meta-analysis with inverse variance weighting was performed. As inference about coefficients of variation is not common, standard errors for this statistics were often not reported. Thus, to gain equally comparable weights for each study, we used approximations of the standard errors of all CVIs, i.e., the CVICr divided by the square root of two times the number of samples employed in estimation (16).
Studies that analyzed and compared different subgroups (i.e., different age groups or different health status) and thus reported biological variation data for each subgroup, were treated as separate studies in our meta-analysis. Different subgroups of the same study are marked with a number in square brackets after the author’s name.
We expected heterogeneity induced by age, sex, study duration, sampling interval, and health status. Though sample size is fairly small, we ran respective univariate and multivariate random effects meta-regressions.
In addition, we performed a subgroup analysis for CKD status.
Outliers analysis was performed following Viechtbauer and Cheung (17). Studies that showed significantly high studentized residuals, i.e., a significant deviation from the pooled overall estimate, were removed.
Statistical analysis was conducted using R, version 4.2.1 (18). For meta-analysis the package meta in version 5.2-0 was used.
Our systematic review on biological variation of Cr yielded 390 publications on PubMed and 41 publications on the EFLM Biological Variation Database. One additional study was retrieved via screening of the reference lists. After removal of duplicates and exclusion of studies not related to the aim of our review, 49 publications were left for quality assessment by the BIVAC. Of these, 12 additional studies met the exclusion criteria leaving 37 studies for analysis. Hilderink et al. (14), Meijers et al. (19) and Reinhard et al. (20) reported biological variation data separately for healthy vs. non-healthy study groups and are therefore each analyzed separately. Similarly, Carobene et al. (21), Larsson et al. (22), Hölzel et al. (23) and Pineda-Tenor et al. (24) compared different groups and were therefore each analyzed as two, three, or four separate subgroups. In total, 37 studies and 48 different subgroups with 2,770 participants were analyzed. A flowchart of the study selection process is presented in Figure 1.
Figure 1. Flowchart of the study selection process. The image is adapted from Page et al. (30).
Studies included in the present review were published between 1971 and 2021, the sample sizes ranged from 2 to 1,105 participants. A total of 19 studies (51%) measured creatinine by Jaffe method, 8 studies (22%) by the enzymatic method. Sampling intervals differed among the studies, ranging from hourly to daily, weekly, and monthly sampling. On average, studies achieved an overall BIVAC score C, only 4 (11%) and 3 (8%) studies were rated with an overall BIVAC score A or B, respectively. A total of 25 (68%) studies were conducted in healthy study participants, 9 (24%) in non-healthy study participants, and 3 (8%) studies compared healthy vs. non-healthy study participants. For 6 (12%) studies the presence of CKD was specified. CVI ranged from 1.2 to 13.4%, CVA ranged from 0.6 to 6.7%.
The main study characteristics are shown in Table 2. Additional information on study characteristics are provided in the Supplementary material.
Analysis of the 37 included studies showed a high between-study heterogeneity (I2 = 82.3%). Meta- analysis of all studies yielded an overall CVICr of 5.2% (95% confidence Interval [CI], 4.6–5.8%). Heterogeneity is also reflected in a rather large 95% prediction interval ranging from 1.8 to 8.6% (25). A Forest-Plot of the meta-analysis is shown in Figure 2.
By analysis of outliers we found the studies of Biosca et al. (26), Fraser et al. (27), Hilderink et al. (14), Hölzel et al. (23), Ozturk et al. (28), and Winkel et al. (29) to significantly increase heterogeneity. Exclusion of these studies lead to a considerable decrease of heterogeneity (I2 = 34.2%) while only slightly decreasing the overall estimate of CVICr to 5.0% (95% CI, 4.7–5.4%). Exclusion of outliers led to a narrower 95% prediction interval ranging from 3.8 to 6.3%.
CVICr tended to be higher in subjects affected by CKD but no significant difference between the two groups was found. Subgroup analysis for the presence of CKD is shown in the Supplementary material.
No significant impact of age, sex, CKD status, analytical method, study duration, and sampling interval on the CVICr was found, hinting toward heterogeneous groups. CVA tended to decrease over time showing lower CVA values in more recent publications. On average, CVA was lower for the enzymatic method as compared to the Jaffe method. Since CVI is derived using ANOVA methods, it is by design separated and unaffected by CVA.
Based on the CVICr at the lower end of our prediction interval after exclusion of outliers, we found a minimum conservative RCV for eGFR of ±12.5%.
Based on all included studies before exclusion of outliers, we found an overall RCV for eGFR of ±16.5%.
As seen in Figure 3, the overall symmetric RCV and the unidirectional, asymmetric RCV only differed slightly.
Figure 3. Reference change value (RCV) of estimated glomerular filtration rate (eGFR) as a function of CVI of creatinine.
Estimated glomerular filtration rate is used in everyday clinical practice as a marker of excretory kidney function. Especially in patients with CKD, where regular assessment of the eGFR is required, a correct interpretation of serial changes in eGFR is crucial for understanding disease dynamics and for clinical decision making.
Based on the present systematic review, we found a minimum conservative RCV of 12.5%. This means, that for a patient with a baseline eGFR of 60 ml/min/1.73 m2 a change to an eGFR >68 ml/min/1.73 m2 or <53 ml/min/1.73 m2 represents a true improvement or deterioration of kidney function. By definition, changes within this range would have a 95% probability of being due to biological variation. For the overall RCV estimate of 16.5% the same applies to an eGFR range of 50–70 ml/min/1.73 m2.
Assuming the KDIGO definition of CKD progression with a 25% drop in eGFR (31), only a change to an eGFR <45 ml/min/1.73 m2 would imply disease progression.
This shows how true deterioration of kidney function may occur at smaller changes than currently defined.
To date, there is considerable controversy as to what constitutes a progression of CKD and different definitions can be found in literature (31, 32). At the same time, the availability of evidence-based interventions to slow CKD progression highlights the importance of an early recognition of kidney function decline (33).
The implementation of the RCV as an additional decision making tool for clinicians has been suggested before (34). Especially in the context of monitoring disease dynamics, the use of the RCV rather than a population-based reference interval seems reasonable. While population-based reference intervals (RI) reflect the variation around the homeostatic set point of different individuals, also known as the between-subject biological variation (CVG), the RCV reflects the variation around one individual’s homeostatic set point (CVI), i.e., the within-subject biological variation. This explains why the use of the RCV would represent a step toward personalized medicine.
For laboratory parameters with a high index of individuality (II), defined as II = CVI/CVG, and especially for an II >1.4, the use of RIs is considered suitable. For laboratory parameters with a low II, i.e., when CVI is lower compared to CVG, the individual itself, rather than the reference population, is considered to be the best point of reference for the assessment of serial change (35). With an II of 0.3 (12), the latter also applies to the eGFR.
The high CVG (12, 14) of eGFR is also reflected in highly heterogeneous patterns of disease progression in patients with CKD, and explains why finding a uniform definition of CKD progression is challenging and may not be suitable for everyone.
This was also shown by Kerschbaum et al. (36) who analyzed the longitudinal eGFR trajectory of patients with diabetic kidney disease by applying various definitions of CKD progression. The allocation of patients to groups with a certain confirmed drop of eGFR (e.g., ≥25, ≥30, ≥35 or ≥40%) did not result in similar patterns of disease progression over time. Next to the high variation of disease progression between subjects, also non-linearity of eGFR trajectories was frequently observed. For example, in patients with a confirmed eGFR drop of >30% only 60.3% and 45.2% lost at least the same amount between baseline and year 4 or 5. The remainder did not show a disease progression but rather a recovery of kidney function. This behavior was also shown for patients on stable medication (36).
The overall CVICr reported by our study is in broad agreement with the overall estimate of the EFLM Biological Variation Database (CVI 4.4% [95% CI 4.2–5.7%]) (8). Similarly, our overall RCV is in line with previously published RCVs (12, 14).
Subgroup analysis by the presence of CKD tended to show higher CVI values in subjects affected by CKD, however, literature on this behalf is discrepant: In 2018, Hilderink et al. (14) found a significantly higher CVICr in subjects without CKD (CVICr 6.4%, mean eGFR 73.4 ± 18.5 ml/min/1.73 m2) compared to subjects with CKD (CVICr 2.5%, mean eGFR 19.2 ± 6.4 ml/min/1.73 m2) (14). Reinhard et al. (20) had previously compared healthy subjects to subjects with mild to moderate CKD. In this case, although not significantly, CVICr was almost twice as high in the CKD group compared to the healthy group (8.9 vs. 4.7%) (20).
The main limitation of our meta-analysis is the high between-study heterogeneity. Nevertheless, after exclusion of seven studies, we see a marked reduction of heterogeneity while still including 85% of all studies. An important driver of heterogeneity may be the health status of the study populations. While subjects classified as healthy form a more homogeneous group, non-healthy study populations included patients affected by a variety of different conditions.
Although choosing more stringent eligibility criteria would have had advantages in terms of heterogeneity, our approach aimed at investigating the impact of different variables on biological variation. Knowledge on how biological variation changes in relation to kidney function is of great importance from a clinical perspective. Analysis by health status as well as by presence of CKD showed no significant impact on biological variation in our analysis, however, our analysis was limited by a small sample size. Altogether, literature on this behalf remains inconclusive. We do believe that an influence of health and disease on biological variation is likely–especially for CKD–and should therefore be subject of further research. Despite the between-study heterogeneity, our estimate of CVICr is comparable to the one reported by the EFLM (8).
Our analysis is based on the MDRD equation. This seemed the more feasible approach, as the exponent of Cr is independent of sex and level of Cr. Besides, the aim of our work is to determine the significance of change of the eGFR, rather than to determine the eGFR, for which the formula by CKD-EPI has shown to be more accurate. Technically, the RCV can also be derived based on the CKD-EPI formula. However, given the different exponents of Cr, the use of the CKD-EPI equation implies that RCV is lower in subjects with higher eGFR and vice versa. As discussed above, there is limited knowledge on how biological variation changes according to GFR, thus, the use of the MDRD seemed more appropriate.
Our analysis included studies with different analytical methods for the measurement of Cr. Since CVI is derived using ANOVA methods, it is by design separated and unaffected by CVA. However, the greater imprecision of the Jaffe method delivers higher values of CVA and thus higher RCVs. If RCVs are applied to monitor kidney function in a clinical context, knowledge on the analytical method by which creatinine is measured is required.
Data on biological variation reported here were collected under idealized and standardized conditions.
This is also required by Quality Item 5 of the BIVAC to ensure that estimates of CVI are not affected by preanalytical variation. According to the BIVAC, authors are encouraged to provide information on preanalytical procedures and to follow a standardized protocol (9). For instance, in many of our studies, blood samples were drawn by the same investigator from fasting patients at the same time of the day. The samples were then further processed and analyzed in the same, standardized manner. If preanalytical procedures are not standardized, increased preanalytical variation could lead to an overestimation of CVI. Even though many procedures (i.e., test ordering, patient preparation, specimen collection and specimen processing, transportation, and storage) may also follow a standardized protocol in clinical routine, they are more difficult to monitor outside of clinical studies. Therefore, it is likely that the CVICr, and therefore the RCV, is higher in real-life settings.
Knowledge about the biological variation of the eGFR is essential for understanding disease dynamics and monitoring kidney function. The RCV provides a valuable tool for clinicians to interpret changes in serial eGFR, however, more studies on biological variation in CKD need to be performed to understand how impairment of kidney function affects biological variation and if higher biological variation is associated with disease progression.
The raw data supporting the conclusions of this article will be made available by the authors, without undue reservation.
ST did the systematic literature research and wrote the first draft of the manuscript. FK conceived and conducted the statistical analyses. ST, LB, and FK scored and assessed included studies. ST, FK, LB, SD, and GM reviewed and approved the final version of the manuscript.
This research activity is part of the project DC-ren that has received funding from the European Union’s Horizon 2020 research and innovation programme under grant agreement No. 848011.
The authors declare that the research was conducted in the absence of any commercial or financial relationships that could be construed as a potential conflict of interest.
All claims expressed in this article are solely those of the authors and do not necessarily represent those of their affiliated organizations, or those of the publisher, the editors and the reviewers. Any product that may be evaluated in this article, or claim that may be made by its manufacturer, is not guaranteed or endorsed by the publisher.
The Supplementary Material for this article can be found online at: https://www.frontiersin.org/articles/10.3389/fmed.2022.1009358/full#supplementary-material
Supplementary Image 1 | Subgroup analysis for presence of CKD.
Supplementary Table 2 | Main study characteristics.
1. Kashani K, Rosner MH, Ostermann M. Creatinine: from physiology to clinical application. Eur J Intern Med. (2020) 72:9–14. doi: 10.1016/j.ejim.2019.10.025
2. Inker LA, Astor BC, Fox CH, Isakova T, Lash JP, Peralta CA, et al. KDOQI US commentary on the 2012 KDIGO clinical practice guideline for the evaluation and management of CKD. Am J Kidney Dis. (2014) 63:713–35. doi: 10.1053/j.ajkd.2014.01.416
3. Fraser CG. Biological variation: a rapidly evolving aspect of laboratory medicine. J Lab Precis Med. (2017) 2:35. doi: 10.21037/jlpm.2017.06.09
4. Braga F, Panteghini M. Generation of data on within-subject biological variation in laboratory medicine: an update. Crit Rev Clin Lab Sci. (2016) 53:313–25. doi: 10.3109/10408363.2016.1150252
5. Harris EK, Yasaka T. On the calculation of a “reference change” for comparing two consecutive measurements. Clin Chem. (1983) 29:25–30. doi: 10.1093/clinchem/29.1.25
6. Fraser CG. Inherent biological variation and reference values. Clin Chem Lab Med. (2004) 42:758–64. doi: 10.1515/CCLM.2004.128
7. Aarsand AK, Røraas T, Bartlett WA, Cos̨kun A, Carobene A, Fernandez-Calle P, et al. Harmonization initiatives in the generation, reporting and application of biological variation data. Clin Chem Lab Med. (2018) 56:1629–36. doi: 10.1515/cclm-2018-0058
8. Aarsand AK, Fernandez-Calle P, Webster C, Coskun A, Gonzales-Lao E, Diaz-Garzon J, et al. The EFLM Biological Variation Database. (2022). Available online at: https://biologicalvariation.eu/ (accessed July 25, 2022).
9. Aarsand AK, Røraas T, Fernandez-Calle P, Ricos C, Díaz-Garzón J, Jonker N, et al. The biological variation data critical appraisal checklist: a standard for evaluating studies on biological variation. Clin Chem. (2018) 64:501–14. doi: 10.1373/clinchem.2017.281808
10. Badrick T, Turner P. The uncertainty of the eGFR. Indian J Clin Biochem. (2013) 28:242–7. doi: 10.1007/s12291-012-0280-1
11. Farrance I, Frenkel R. Uncertainty of measurement: a review of the rules for calculating uncertainty components through functional relationships. Clin Biochem Rev. (2012) 33:49–75.
12. Rowe C, Sitch AJ, Barratt J, Brettell EA, Cockwell P, Dalton RN, et al. Biological variation of measured and estimated glomerular filtration rate in patients with chronic kidney disease. Kidney Int. (2019) 96:429–35. doi: 10.1016/j.kint.2019.02.021
13. Carobene A, Marino I, Cos̨kun A, Serteser M, Unsal I, Guerra E, et al. The EuBIVAS project: within- and between-subject biological variation data for serum creatinine using enzymatic and alkaline picrate methods and implications for monitoring. Clin Chem. (2017) 63:1527–36. doi: 10.1373/clinchem.2017.275115
14. Hilderink JM, van der Linden N, Kimenai DM, Litjens EJR, Klinkenberg LJJ, Aref BM, et al. Biological variation of creatinine, cystatin C, and eGFR over 24 Hours. Clin Chem. (2018) 64:851–60. doi: 10.1373/clinchem.2017.282517
15. Fraser CG. Reference change values. Clin Chem Lab Med. (2011) 50:807–12. doi: 10.1515/cclm.2011.733
16. Curto JD, Pinto JC. The coefficient of variation asymptotic distribution in the case of non-iid random variables. J Appl Stat. (2009) 36:21–32. doi: 10.1080/02664760802382491
17. Viechtbauer W, Cheung MW. Outlier and influence diagnostics for meta-analysis. Res Synth Methods. (2010) 1:112–25. doi: 10.1002/jrsm.11
18. R Core Team. R: A Language and Environment for Statistical Computing. Vienna: R Foundation for Statistical Computing (2020).
19. Meijers WC, van der Velde AR, Muller Kobold AC, Dijck-Brouwer J, Wu AH, Jaffe A, et al. Variability of biomarkers in patients with chronic heart failure and healthy controls. Eur J Heart Fail. (2017) 19:357–65. doi: 10.1002/ejhf.669
20. Reinhard M, Erlandsen EJ, Randers E. Biological variation of cystatin C and creatinine. Scand J Clin Lab Invest. (2009) 69:831–6. doi: 10.3109/00365510903307947
21. Carobene A, Graziani MS, Lo Cascio C, Tretti L, Cremonese E, Yabarek T, et al. Age dependence of within-subject biological variation of nine common clinical chemistry analytes. Clin Chem Lab Med. (2012) 50:841–4. doi: 10.1515/cclm-2011-0868
22. Larsson A, Akerstedt T, Hansson LO, Axelsson J. Circadian variability of cystatin C, creatinine, and glomerular filtration rate (GFR) in healthy men during normal sleep and after an acute shift of sleep. Chronobiol Int. (2008) 25:1047–61. doi: 10.1080/07420520802553614
23. Hölzel WG. Intra-individual variation of some analytes in serum of patients with insulin-dependent diabetes mellitus. Clin Chem. (1987) 33:57–61. doi: 10.1093/clinchem/33.1.57
24. Pineda-Tenor D, Laserna-Mendieta EJ, Timón-Zapata J, Rodelgo-Jiménez L, Ramos-Corral R, Recio-Montealegre A, et al. Biological variation and reference change values of common clinical chemistry and haematologic laboratory analytes in the elderly population. Clin Chem Lab Med. (2013) 51:851–62. doi: 10.1515/cclm-2012-0701
25. IntHout J, Ioannidis JP, Rovers MM, Goeman JJ. Plea for routinely presenting prediction intervals in meta-analysis. BMJ Open. (2016) 6:e010247. doi: 10.1136/bmjopen-2015-010247
26. Biosca C, Ricós C, Lauzurica R, Petersen PH. Biological variation at long-term renal post-transplantation. Clin Chim Acta. (2006) 368:188–91. doi: 10.1016/j.cca.2005.12.018
27. Fraser CG, Hearne CR. Components of variance of some plasma constituents in patients with myocardial infarction. Ann Clin Biochem. (1982) 19:431–4. doi: 10.1177/000456328201900608
28. Ozturk OG, Paydas S, Balal M, Sahin G, Karacor ED, Ariyurek SY, et al. Biological variations of some analytes in renal posttransplant patients: a different way to assess routine parameters. J Clin Lab Anal. (2013) 27:438–43. doi: 10.1002/jcla.21625
29. Winkel P, Statland BE, Bokelund H. Factors contributing to intra-individual variation of serum constituents: 5. Short-term day-to-day and within-hour variation of serum constituents in healthy subjects. Clin Chem. (1974) 20:1520–7. doi: 10.1093/clinchem/20.12.1520
30. Page MJ, McKenzie JE, Bossuyt PM, Boutron I, Hoffmann TC, Mulrow CD, et al. The PRISMA 2020 statement: an updated guideline for reporting systematic reviews. BMJ (2021) 372:n71 doi: 10.1136/bmj.n71
31. Kidney Disease. Improving global outcomes (KDIGO) CKD work group: KDIGO 2012 clinical practice guideline for the evaluation and management of chronic kidney disease. Kidney Int Suppl. (2012) 3:1.
32. National Collaborating Centre for Chronic Conditions. National Institute for Health and Clinical Excellence: Guidance. Chronic Kidney Disease: National Clinical Guideline for Early Identification and Management in Adults in Primary and Secondary Care. London: Royal College of Physicians (2008).
33. Shlipak MG, Tummalapalli SL, Boulware LE, Grams ME, Ix JH, Jha V, et al. The case for early identification and intervention of chronic kidney disease: conclusions from a kidney disease: improving global outcomes (KDIGO) controversies conference. Kidney Int. (2021) 99:34–47.
34. Baysoy A, Karakoyun I, Arslan FD, Basok BI, Colak A, Duman C. Biological variation data for kidney function related parameter: serum beta trace protein, creatinine and cystatin C from 22 apparently healthy Turkish subjects. Clin Chem Lab Med. (2022) 60:584–92. doi: 10.1515/cclm-2021-0543
35. Carobene A, Banfi G, Locatelli M, Vidali M. Within-person biological variation estimates from the European biological variation study (EuBIVAS) for serum potassium and creatinine used to obtain personalized reference intervals. Clin Chim Acta Int J Clin Chem. (2021) 523:205–7. doi: 10.1016/j.cca.2021.09.018
36. Kerschbaum J, Rudnicki M, Dzien A, Dzien-Bischinger C, Winner H, Heerspink HL, et al. Intra-individual variability of eGFR trajectories in early diabetic kidney disease and lack of performance of prognostic biomarkers. Sci Rep. (2020) 10:19743. doi: 10.1038/s41598-020-76773-0
37. Levey AS, Bosch JP, Lewis JB, Greene T, Rogers N, Roth D. A more accurate method to estimate glomerular filtration rate from serum creatinine: a new prediction equation. Modification of diet in renal disease study group. Ann Intern Med. (1999) 130:461–70. doi: 10.7326/0003-4819-130-6-199903160-00002
38. Levey AS, Stevens LA, Schmid CH, Zhang YL, Castro AF III, Feldman HI, et al. A new equation to estimate glomerular filtration rate. Ann Intern Med. (2009) 150:604–12. doi: 10.7326/0003-4819-150-9-200905050-00006
39. Bandaranayake N, Ankrah-Tetteh T, Wijeratne S, Swaminathan R. Intra-individual variation in creatinine and cystatin C. Clin Chem Lab Med. (2007) 45:1237–9. doi: 10.1515/CCLM.2007.256
40. Biosca C, Ricós C, Jiménez CV, Lauzurica R, Galimany R. Model for establishing biological variation in non-healthy situations: renal posttransplantation data. Clin Chem. (1997) 43:2206–8. doi: 10.1093/clinchem/43.11.2206
41. Carter JL, Parker CT, Stevens PE, Eaglestone G, Knight S, Farmer CK, et al. Biological variation of plasma and urinary markers of acute kidney injury in patients with chronic kidney disease. Clin Chem. (2016) 62:876–83. doi: 10.1373/clinchem.2015.250993
42. Costongs GM, Janson PC, Bas BM, Hermans J, van Wersch JW, Brombacher PJ. Short-term and long-term intra-individual variations and critical differences of clinical chemical laboratory parameters. J Clin Chem Clin Biochem. (1985) 23:7–16. doi: 10.1515/cclm.1985.23.7.405
43. Dimitri G, Bolner A, Gheller A. Variabilità biologica ed analitica e differenza critica di 21 analiti “comuni”. Biochim Clin. (1992) 16:401–3.
44. Fraser CG, Williams P. Short-term biological variation of plasma analytes in renal disease. Clin Chem. (1983) 29:508–10. doi: 10.1093/clinchem/29.3.508
45. Fraser CG, Cummings ST, Wilkinson SP, Neville RG, Knox JD, Ho O, et al. Biological variability of 26 clinical chemistry analytes in elderly people. Clin Chem. (1989) 35:783–6. doi: 10.1093/clinchem/35.5.783
46. Gallagher SK, Johnson LK, Milne DB. Short- and long-term variability of selected indices related to nutritional status. II. Vitamins, lipids, and protein indices. Clin Chem. (1992) 38(8 Pt 1):1449–53. doi: 10.1093/clinchem/38.8.1449
47. González-Revaldería J, García-Bermejo S, Menchén-Herreros A, Fernandez-Rodriguez E. Towards narrower analytical goals in routine laboratory work. Clin Chem. (1991) 37:596. doi: 10.1093/clinchem/37.4.596
48. Gowans EM, Fraser CG. Biological variation of serum and urine creatinine and creatinine clearance: ramifications for interpretation of results and patient care. Ann Clin Biochem. (1988) 25(Pt 3):259–63. doi: 10.1177/000456328802500312
49. Keevil BG, Kilpatrick ES, Nichols SP, Maylor PW. Biological variation of cystatin C: implications for the assessment of glomerular filtration rate. Clin Chem. (1998) 44:1535–9. doi: 10.1093/clinchem/44.7.1535
50. Matsubara A, Ichihara K, Fukutani S. Determination of reference intervals for 26 commonly measured biochemical analytes with consideration of long-term within-individual variation. Clin Chem Lab Med. (2008) 46:691–8. doi: 10.1515/CCLM.2008.140
51. Nunes LA, Brenzikofer R, de Macedo DV. Reference change values of blood analytes from physically active subjects. Eur J Appl Physiol. (2010) 110:191–8. doi: 10.1007/s00421-010-1493-8
52. Qi Z, Chen Y, Zhang L, Ma X, Wang F, Cheng Q, et al. Biological variations of thirteen plasma biochemical indicators. Clin Chim Acta. (2016) 452:87–91. doi: 10.1016/j.cca.2015.11.008
53. Ravn B, Larsson A, Mårtensson J, Martling CR, Bell M. Intra-day variability of cystatin C, creatinine and estimated GFR in intensive care patients. Clin Chim Acta. (2016) 460:1–4. doi: 10.1016/j.cca.2016.06.014
54. Rosano TG, Brown HH. Analytical and biological variability of serum creatinine and creatinine clearance: implications for clinical interpretation. Clin Chem. (1982) 28:2330–1. doi: 10.1093/clinchem/28.11.2330
55. Statland BE, Winkel P, Bokelund H. Factors contributing to intra-individual variation of serum constituents. 1. Within-day variation of serum constituents in healthy subjects. Clin Chem. (1973) 19:1374–9. doi: 10.1093/clinchem/19.12.1374
56. Toffaletti JG, McDonnell EH. Variation of serum creatinine, cystatin C, and creatinine clearance tests in persons with normal renal function. Clin Chim Acta. (2008) 395:115–9. doi: 10.1016/j.cca.2008.05.020
57. Waikar SS, Rebholz CM, Zheng Z, Hurwitz S, Hsu CY, Feldman HI, et al. Biological variability of estimated GFR and albuminuria in CKD. Am J Kidney Dis. (2018) 72:538–46. doi: 10.1053/j.ajkd.2018.04.023
58. Wang S, Zhao M, Su Z, Mu R. Annual biological variation and personalized reference intervals of clinical chemistry and hematology analytes. Clin Chem Lab Med. (2022) 60:606–17. doi: 10.1515/cclm-2021-0479
59. Williams GZ, Widdowson GM, Penton J. Individual character of variation in time-series studies of healthy people: II. Differences in values for clinical chemical analytes in serum among demographic groups, by age and sex. Clin Chem. (1978) 24:313–20. doi: 10.1093/clinchem/24.2.313
Keywords: chronic kidney disease, CKD progression, biological variation, reference change value (RCV), estimated glomerular filtration rate
Citation: Thöni S, Keller F, Denicolò S, Buchwinkler L and Mayer G (2022) Biological variation and reference change value of the estimated glomerular filtration rate in humans: A systematic review and meta-analysis. Front. Med. 9:1009358. doi: 10.3389/fmed.2022.1009358
Received: 01 August 2022; Accepted: 20 September 2022;
Published: 06 October 2022.
Edited by:
Carmen Tzanno-Martins, Hospital Alemão Oswaldo Cruz, BrazilReviewed by:
Maria-Eleni Roumelioti, University of New Mexico, United StatesCopyright © 2022 Thöni, Keller, Denicolò, Buchwinkler and Mayer. This is an open-access article distributed under the terms of the Creative Commons Attribution License (CC BY). The use, distribution or reproduction in other forums is permitted, provided the original author(s) and the copyright owner(s) are credited and that the original publication in this journal is cited, in accordance with accepted academic practice. No use, distribution or reproduction is permitted which does not comply with these terms.
*Correspondence: Felix Keller, ZmVsaXgua2VsbGVyQGktbWVkLmFjLmF0
Disclaimer: All claims expressed in this article are solely those of the authors and do not necessarily represent those of their affiliated organizations, or those of the publisher, the editors and the reviewers. Any product that may be evaluated in this article or claim that may be made by its manufacturer is not guaranteed or endorsed by the publisher.
Research integrity at Frontiers
Learn more about the work of our research integrity team to safeguard the quality of each article we publish.