- 1Intestinal Microenvironment Treatment Center of General Surgery, Tenth People's Hospital of Tongji University, Shanghai, China
- 2Clinical Research Center for Digestive Diseases of Tongji University, Shanghai, China
- 3Realbio Genomics Institute, Shanghai, China
Slow transit constipation (STC) is one of the most frequent gastrointestinal diagnoses. In this study, we conducted a quantitative metagenomics study in 118 Chinese individuals. These participants were divided into the discovery cohort of 50 patients with STC and 40 healthy controls as well as a validation cohort of 16 patients and 12 healthy controls. We found that the intestinal microbiome of patients with STC was significantly different from that of healthy individuals at the phylum, genus, and species level. Patients with STC had markedly higher levels of Alistipes and Eubacterium and lower abundance of multiple species belonging to the Roseburia genus. Patients with STC gene expression levels and the Kyoto Encyclopedia of Genes and Genomes (KEGG) orthology pathway (such as fatty acid biosynthesis, butanoate metabolism, and methane metabolism pathways) enrichment were also substantially different from those of healthy controls. These microbiome and metabolite differences may be valuable biomarkers for STC. Our findings suggest that alteration of the microbiome may lead to constipation by changing the levels of microbial-derived metabolites in the gut. Above findings may help us in the development of microbial drugs.
Introduction
Chronic constipation is characterized by various symptoms such as straining, lumpy or hard stool, sensation of incomplete evacuation, sensation of anorectal obstruction, and infrequent defecation (<3 defecations/week) (1, 2). Slow transit constipation (STC) is the major category of chronic constipation (3). The characteristics of STC include slower colonic transit and fewer high-amplitude propagated contractions. It is a complex pathogenesis that involves the enteric nervous system, the interstitial cells of Cajal, and colonic smooth muscle, but it is still incompletely understood (4–7).
Decreased colonic motility is an important pathophysiological mechanism of STC. Recently, several studies have suggested that gut microbiota may be involved in the etiology of constipation. Disturbance of stool microbiota has been found in many patients with constipation (8, 9). Furthermore, Vandeputte et al. found that stool consistency is associated with the richness and composition of gut microbiota as well as enterotypes and bacterial growth rates (10). Recent advances in sequencing technology have profoundly affected the field of microbiology. Quantitative metagenomics analysis has been used successfully to study the pathogenesis of many chronic diseases (11, 12). However, to date, no study has used this approach to analyze STC.
In this study, to comprehensively catalog the gut microbiome features of constipation, we conducted a quantitative metagenomics study in 118 adults, including STC and healthy controls.
Methods
Study Participants
A total of 66 patients with STC and 52 healthy controls from September 2015 to December 2019 were enrolled in this study from the Shanghai Tenth People's Hospital. Inclusion criteria: Ages eligible for study: 18 years and older; body mass index: 18–24 kg/m2; and STC as defined by patients with a colonic transit test (CTT) of >48 h (13, 14). This clinical trial was registered at ClinicalTrials.gov (NCT02395484). The clinical diagnosis and fecal samples of all the individuals were obtained from the hospitals (Table 1).
Sample Collection and DNA Extraction
Fecal samples were collected from the recruited subjects from these 118 participants. All the samples were then frozen immediately and stored at −80°C until DNA extraction. DNA was extracted from each stool sample using the commercial QIAamp DNA Stool Mini Kit (Qiagen, Valencia, California, USA).
Deoxyribonucleic Acid Library Construction and Sequencing
The metagenomic DNA libraries were constructed with 2 μg genome DNA according to the instruction of the manufacturer (Illumina, California, USA), with an average of 350 bp insert size. The quality of all the libraries was evaluated using the Agilent 2100 Bioanalyzer with a DNA 1000 LabChip Kit.
Illumina Hiseq Sequencing
The Illumina Hiseq platform was employed to sequence the 118 samples, thereby obtaining a total of 1.6 Tbp sequencing data. Illumina raw reads were subjected to the following treatments: (1) reads with more than 3 ambiguous N bases were removed; (2) reads with less than 60% of high quality bases (Phred score ≥ 20) were deleted; and (3) 3′ end of reads were trimmed to the first high quality base. The subsequent high quality reads were further mapped to human genome by SOAPaligner (version 2.21) and any hit associated with the reads and their mated reads was removed. After QC, trimming, and remove host contaminate, 44.76 ± 0.86 million clean reads per sample on average are reserved. Sample statistic information is given in Supplementary Table S1.
De novo Assembly of the Illumina Short Reads
SOAPdenovo (version 2.04), which is based on De Bruijn graph construction, was employed to assemble short reads with parameters “-M 3 -u -L 100 -d 1 -F.” k-mers, varying from 39 to 59 by 4, was tested for each sample (15). The resulting scaffolds were cut into contigs at ambiguous Ns and only contigs longer than 500 bp were saved. N50 was calculated for contigs of different k-mers and only the contigs of largest N50 assembly were attributed to a sample. All these contigs were applied for gene prediction by Meta-Gene Marker (version 3.25).
Taxonomic and Gene Profiling
Microbial composition at each taxonomic level was calculated using the MetaPhlAn2 program with default parameters. The program is available at https://bitbucket.org/biobakery/biobakery/wiki/humann2. Relative abundances of the genes were calculated with the procedure introduced in Qin et al. (12). When calculating the abundance of genes, the high quality reads from each sample were aligned against the gene catalog by using SOAPalign2.21 with parameters of “-r 2 -m 100 - × 1,000” and only the both paired-end reads, which could be mapped to a same gene, were accepted.
Gene Catalog, the Kyoto Encyclopedia of Genes and Genomes Database Annotation, and Pathway Profile
After removing redundancy by Collect DNA-HIT, genes were also annotated by the KEGG database. The KEGG Orthology (KO) profiling was calculated as the sum abundances of genes with the same KO number. The abundances of the KEGG pathway were calculated as the average abundances of all the KO under the pathway (16).
Statistical Methods
Characteristics of participant between two groups were compared by using the t-tests for continuous variables and the chi-squared tests for categorical variables. The richness and the β-diversity of the microbiota dataset were analyzed using Quantitative Insights Into Microbial Ecology (17). The nonparametric Wilcoxon signed-rank test was employed to analyze the statistical significance of the gene, the KO, Ortholog Groups, enzyme, and different taxonomic (phylum, genus, and species) levels between the STC and healthy control group. The relative abundance of these features was subjected to statistical analyses. For categorical metadata and enterotype comparisons, samples were pooled into bins and significant features were identified using the Fisher's exact test with multiple testing correction of p-values. Statistical analyses were performed using SAS software (version 9.4; SAS Institute, Cary, North Carolina, USA) and R software (version 3.6.3; R Foundation for Statistical Computing, Vienna, Austria) (18).
Results
Enrollment of the Patient
Phenotype information was obtained from patients with STC and healthy controls during the discovery stage (90 samples) and the validation stage (28 samples), as shown in Table 1. The data obtained from these 118 samples are shown in Supplementary Table S1.
Difference in Gut Microbiota Composition
Our analysis revealed that STC displayed significant differences between two groups (Figure 1). At the phylum level, the intestinal flora in patients with STC was enriched in Firmicutes, Actinobacteria, and Verrucomicrobia and in healthy individuals was enriched in Bacteroidetes, Euryarchaeota, Fusobacteria, and Synergistetes (Supplementary Table S2). The genera enriched in patients with STC included Alistipes, Parabacteroides, Subdoligranulum, and Ruminococcus and the genera enriched in healthy individuals were Bacteroides, Roseburia, Haemophilus, and Klebsiella (Supplementary Table S3). At the species level, patients with STC were enriched in Alistipes putredinis, Parabacteroides merdae, Odoribacter splanchnicus, and Eubacterium eligens and healthy individuals were enriched in Roseburia intestinalis, Haemophilus parainfluenzae, Megamonas unclassified, and Klebsiella pneumoniae (Supplementary Table S4). Therefore, the intestinal microbiome of patients with STC is significantly different from that of healthy individuals at the phylum, genus, and species level.
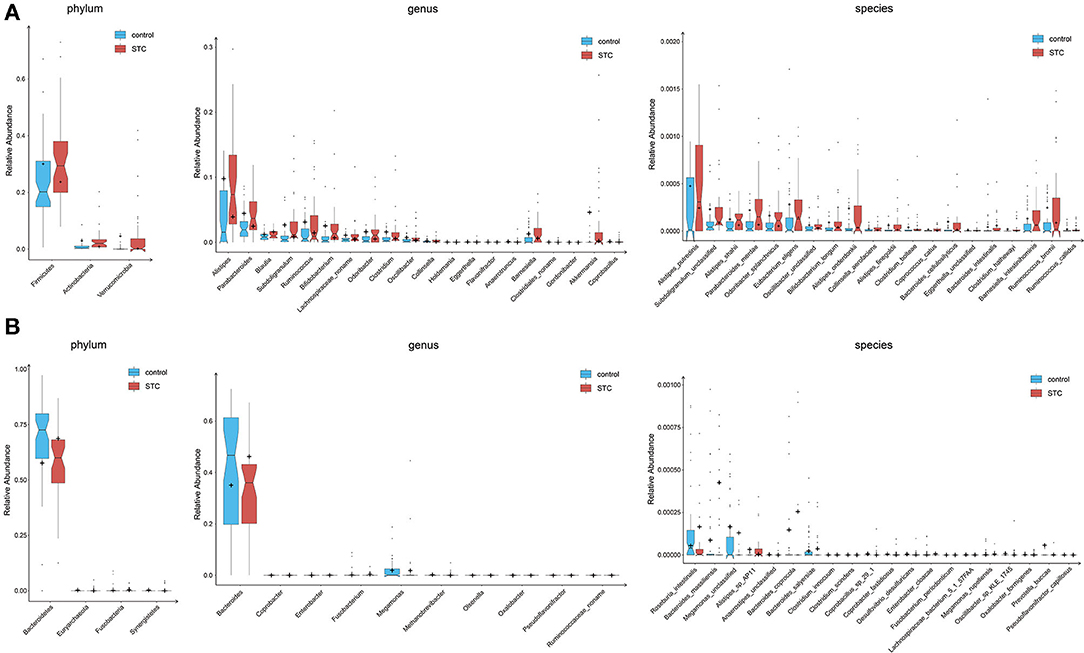
Figure 1. Differences of phylogenetic abundance between patients with slow transit constipation (STC) and healthy controls. The phylotypes were increased (A) or decreased (B) in patients with STC at the phylum, genus, and species levels. Red and blue indicate patients with STC and healthy controls, respectively. The phylogenetic abundance of phyla that had mean values <1% and that of genera and species that were <0.01% was excluded. After exclusion, the Wilcoxon rank-sum tests were applied to identify the differentially abundant phyla, genera, and species. Among these, the highest medians of the phylogenetic abundance in the enriched cohort were drawn as boxplots.
Detection of Enterotypes in Patients With STC
Enterotype is a characteristic stratification of the intestinal microbiome population. To determine whether the patients with STC have a unique enterotype, we subjected 90 samples from cohort 1 to enterotype analysis, as described previously (19). The results showed that all the samples clustered into 1 of 3 enterotypes. The Bacteroides and Prevotella enterotypes were identical to those reported earlier (6) and the third enterotype was distinguished by high levels of Alistipes and Eubacterium (Figure 2; Supplementary Figure S1). The heatmap of top 30 most abundant genera for each of these 3 enterotypes is shown in Supplementary Figure S2.
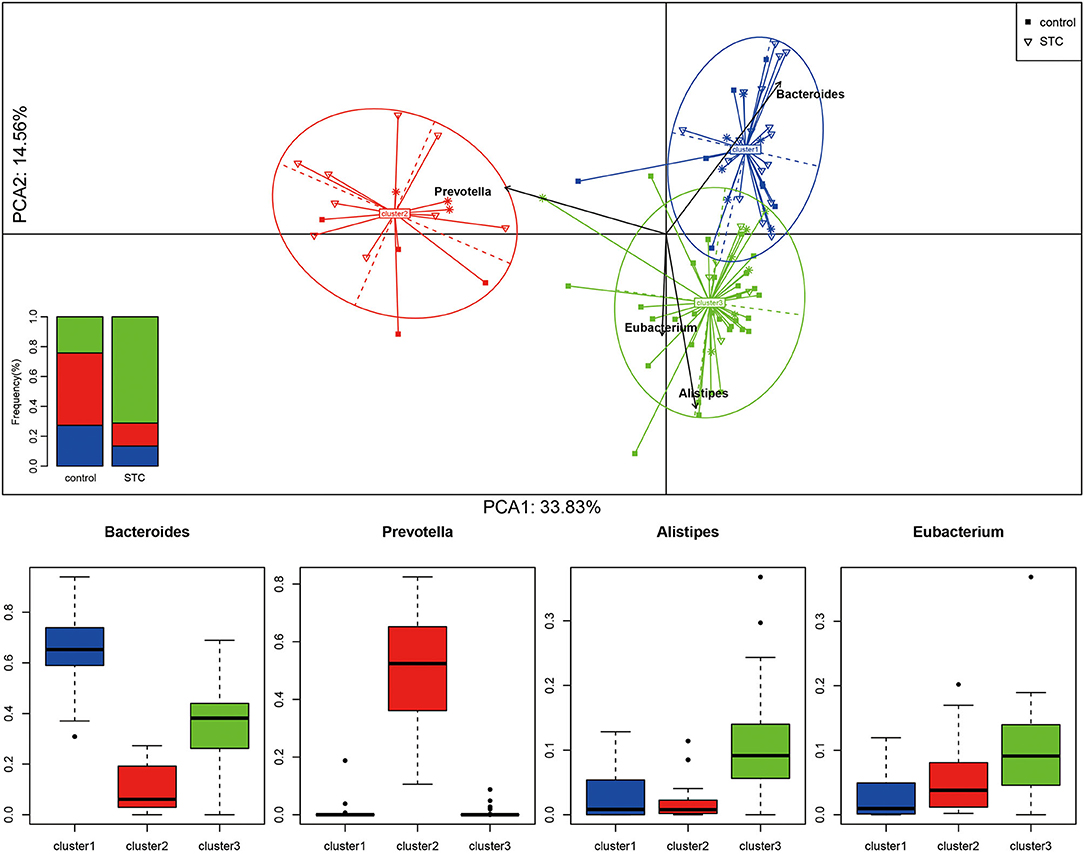
Figure 2. Sample clustering and classification for Enterotype analysis. According to the Jensen–Shannon distance of genus level, all the samples are clustered into the 3 types by the method of Manimozhiyan. Each of these 3 clusters are identifiable by the variation in the levels of 1 of 3 genera: Bacteroides (cluster 1), Prevotella (cluster 2), Alistipes and Ruminococcus (cluster 3). The histogram shows the distribution ratio of the control and STC groups in the 3 clusters.
Differences in Microbial Gene and Pathway Expression Between Two Groups
The gut microbiota of patients with STC and healthy individuals included in this study differed greatly, not only at the phylum, genus, and species level, but also in terms of gene expression. Genes corresponding to the 859 KO entries were differentially expressed in these 2 groups: 609 genes were enriched in patients with STC and 250 genes were enriched in healthy controls (Supplementary Table S5). To determine the functional impact of these differences, we performed a pathway analysis and found that the most abundant KEGG orthologs in both the groups were those associated with fatty acid biosynthesis (Supplementary Figure S3). In the fatty acid biosynthesis pathway, 3-oxoacyl-(acyl-carrier-protein) synthase II [EC:2.3.1.179] (fabF, K09458), 3-hydroxyacyl-(acyl-carrier-protein) dehydratase [EC:4.2.1.59] (fabZ, K02372), enoyl-(acyl-carrier protein) reductase I [EC:1.3.1.9 1.3.1.10] (fabI, K00208), and long-chain acyl-CoA synthetase [EC:6.2.1.3] (K01897) were enriched in healthy controls, whereas acetyl-CoA carboxylase/biotin carboxylase 1 [EC:6.4.1.2] (K11262) and fatty acid synthase, bacteria type [EC:2.3.1] (K11533) were enriched in patients with STC (Supplementary Figure S4).
In the butanoate metabolism (map00650) pathway, succinate dehydrogenase/fumarate reductase, flavoprotein subunit [EC:1.3.5.1] (K00239) was enriched in healthy controls, while (R,R)-butanediol dehydrogenase/meso-butanediol dehydrogenase/diacetyl reductase [EC:1.1.1.4 1.1.1.-1.1.1.303] (K00004), succinate-semialdehyde dehydrogenase [EC:1.2.1.76] (K18119), acetate CoA/acetoacetate CoA-transferase alpha subunit [EC:2.8.3.8 2.8.3.9] (K01034), acetaldehyde dehydrogenase (acetylating) [EC:1.2.1.10] (K00132), 4-hydroxybutyrate dehydrogenase [EC:1.1.1.61] (K00043), 4-hydroxybutyrate CoA-transferase [EC:2.8.3.-] (K18122), 4-hydroxybutyryl-CoA dehydratase/vinylacetyl-CoA delta-isomerase [EC:4.2.1.120 5.3.3.3] (K14534), butyryl-CoA dehydrogenase [EC:1.3.8.1] (K00248), enoyl-CoA hydratase [EC:4.2.1.17] (K01692), glutaconate CoA-transferase, subunit A [EC:2.8.3.12] (K01039), 3-hydroxybutyryl-CoA dehydrogenase [EC:1.1.1.157] (K00074), acetyl-CoA C-acetyltransferase [EC:2.3.1.9] (K00626), and hydroxymethylglutaryl-CoA lyase [EC:4.1.3.4] (K01640) were enriched in patients with STC (Supplementary Figure S5).
In the methane metabolism (map00680) pathway, acetyl-CoA synthetase [EC:6.2.1.1] (K01895), phosphate acetyltransferase [EC:2.3.1.8] (K00625), malate dehydrogenase [EC:1.1.1.37] (K00024), and 2,3-bisphosphoglycerate-independent phosphoglycerate mutase [EC:5.4.2.12] (K15633) were enriched in healthy controls, whereas heterodisulfide reductase subunit A [EC:1.8.98.1] (K03388), 3-hexulose-6-phosphate synthase [EC:4.1.2.43] (K08093), (methyl-Co(III) methanol-specific corrinoid protein):coenzyme M methyltransferase [EC:2.1.1.246] (K14080), formylmethanofuran dehydrogenase subunit A [EC:1.2.7.12] (K00200), anaerobic carbon monoxide dehydrogenase, CODH/acetyl-CoA synthase (ACS) complex subunit alpha [EC:1.2.7.4] (K00192), acetyl-CoA decarbonylase/synthase, CODH/ACS complex subunit beta [EC:2.3.1.169] (K00193), 2-phosphosulfolactate phosphatase [EC:3.1.3.71] (K05979), and methanogen homoaconitase large subunit [EC:4.2.1.114] (K16792) were enriched in patients with STC (Supplementary Figure S6).
Construction of a Model for Classifying Patients as Having STC Based on Intestinal Microbiome Biomarkers
On the basis of the microbial and gene expression characteristics identified as described above, we next sought to construct a model for distinguishing patients with STC from healthy individuals. First, we selected the 15 species with the most advantageous receiver operating characteristic (ROC) curve values from among the 59 species with differential abundance between the two groups (Table 2). The classification model constructed using these species as biomarkers had an Area Under Curve (AUC) of 88.65% in the discovery set and 78.65% in the validation set (Figure 3). Next, we constructed a classification model using the 10 differentially expressed KO markers with the most advantageous ROC curve values among the 859 differentially expressed KO biomarkers. This model had an AUC of 95.15% in the discovery set and 79.69% in the verification set (Table 3). Thus, the model constructed using KO biomarkers can adequately distinguish between patients with STC and healthy individuals.
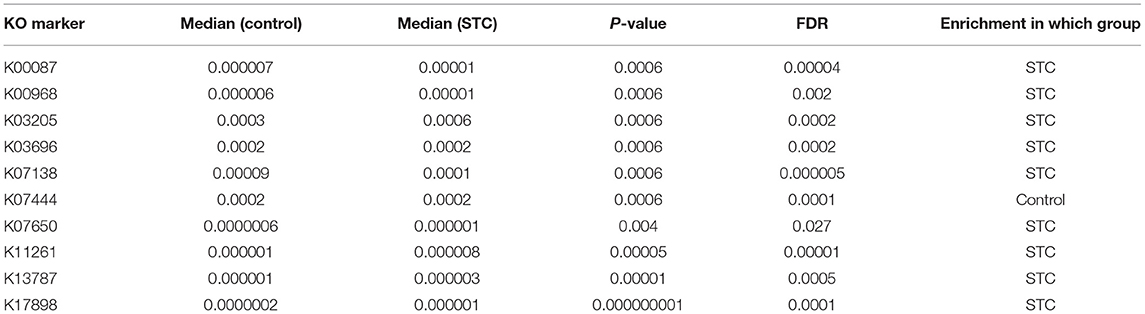
Table 2. The best 10 KEGG Ontology (KO) markers in the receiver operating characteristic (ROC) curve picked from the 859 differential KO markers.
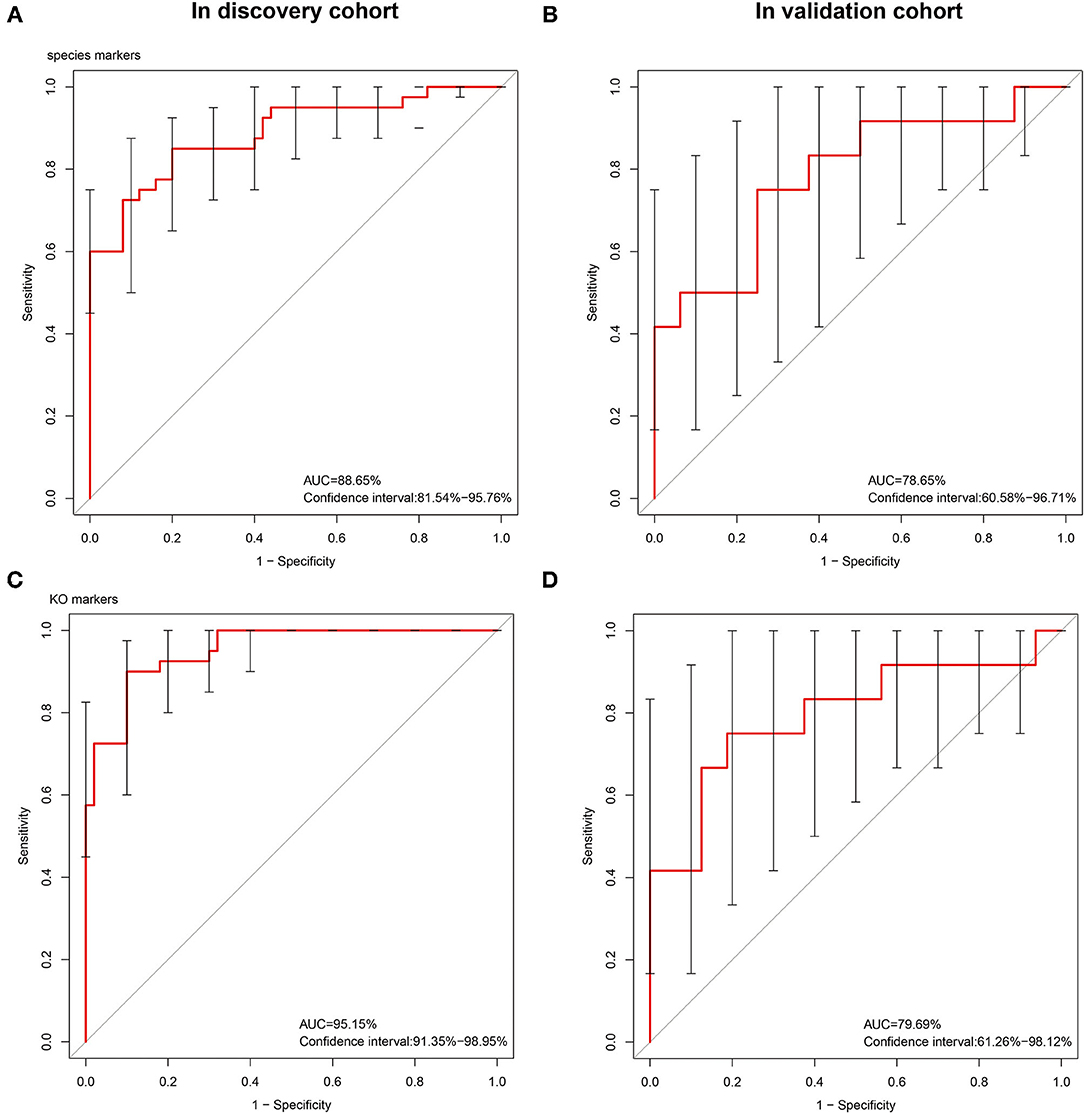
Figure 3. The receiver operating characteristic (ROC) curves of the sequenced reference species markers and the KEEG Ontology (KO) markers. The classification model constructed using 15 species as biomarkers had an AUC of 88.65% in the discovery set (A) and 78.65% (B) in the validation set. We constructed a classification model using the 10 differentially expressed KO markers with the most advantageous ROC curve values among the 859 differentially expressed KO biomarkers. This model had an AUC of 95.15% (C) in the discovery set and 79.69% (D) in the verification set.
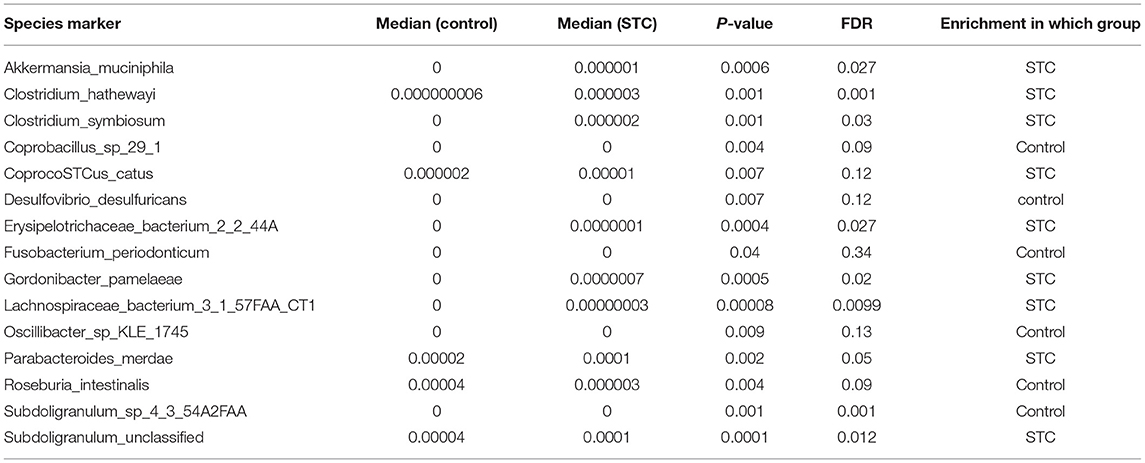
Table 3. The best 15 species markers in the ROC curve picked from the 59 differential species markers.
Discussion
In this study, we studied the gut microbiome in patients with STC using quantitative metagenomics. To the best of our knowledge, this is largest metagenomics study performed to date analyzing the characteristics of the gut microbiome in patients with STC. Our results show that the gut microbial characteristics of patients with STC differ from those of healthy controls, suggesting that gut microbiome composition may contribute to the development of STC.
We found that the intestinal flora of patients with constipation is more diverse than that of healthy individuals. In addition, we identified significant differences between the constipated and control groups at the phylum, genus, and species level. There was a statistically significant increase in the abundance of Actinobacteria, Firmicutes, and Verrucomicrobia and a statistically significant decrease in the abundance of Bacteroidetes, Euryarchaeota, Fusobacteria, and Synergistetes in the gut of patients with STC compared with the healthy control group.
However, the abundance of Roseburia intestinalis, a prominent butyrate-producing Firmicute that is reported to be the primary degrader of dietary fiber (20), was increased in the healthy group compared with the STC group. This is the first study to robustly demonstrate that Roseburia intestinalis abundance is increased in the gut microbiome of patients with STC compared with healthy controls using shotgun sequencing. The altered abundances of this species are a cause or a consequence of constipation that warrant further investigation. In the recent years, the role of Roseburia intestinalis has been revealed in more and more studies (21, 22). The administration of Roseburia intestinalis contributes to restoration of the gut microbiota, promoting colon repair, and the recovery of gastrointestinal function.
The findings from this study may provide a rationale for microbiome-based therapies and patient stratification in the clinical management of constipation. The molecular Operational Taxonomic Units markers identified the current cohort that need to be validated in larger, independent studies. Although the alterations that we noted in the microbiota could serve as biomarkers for disease diagnosis or severity stratification, animal experiments and in-vitro studies are needed to elucidate whether these markers are cause of the disease or simply the consequence of increased CTT leading to stool retention and altered fermentation parameters (23, 24). Further investigation into possible mechanisms, microbial-assisted diagnosis, and therapeutics holds great potential for the effective management of constipation.
We constructed classification algorithms (classifiers) to identify patients with STC using 3 types of biomarkers. Each of these 3 enterotypes are identifiable by the variation in the levels of 1 of 3 genera: Bacteroides (enterotype 1), Prevotella (enterotype 2), and the 3rd enterotypes were marked by Alistipes and Eubacterium (Figure 2; Supplementary Figure S1). These phylogenetic and functional differences among enterotypes, thus, reflect different combinations of microbial trophic chains with a probable impact on synergistic interrelations with the human hosts. The robustness and predictability of the enterotypes in different cohorts and at multiple phylogenetic and functional levels indicate that they are the result of well-balanced, defined microbial community compositions of which only a limited number exist across individuals (25, 26).
The results showed that all the samples clustered into 1 of 3 enterotypes. The Bacteroides and Prevotella enterotypes were identical to those reported earlier in other diseases (27–29) and the 3rd enterotype was distinguished by high levels of Alistipes and Eubacterium, which have never been reported, may be unique to patients with STC, and were different from other types of diseases.
Some strong correlations occur between host properties and particular functions at the gene or module level (a module is a part of a pathway that is functionally tightly interconnected); we also attempted to identify bacterial biomarkers that may help in the diagnosis of STC by generating 10 different metagenomic shotgun markers. When using these biomarkers, we were able to distinguish patients with STC from normal patients with a moderate degree of certainty (AUC = 82.69). This is extremely important for the diagnosis and classification of constipation and serving the clinic through a cost-effective and time-saving technique is one of the purposes of this study.
The large sample size used in this study allowed us to focus on functional metagenomic data that are potentially related to prognosis and could be important for identifying adjuvant therapeutic targets that target functional pathways rather than specific species. Our analysis suggested that there are differences in the fatty acid biosynthesis, fatty acid degradation, butanoate metabolism, and methane metabolism pathways between STC and healthy microbiomes. Thus, modulating these metabolic pathways could influence the development of STC and related symptoms, providing new avenues for exploring the role of the gut microbiome in STC (30).
The most enriched orthologs in patients with STC were related to fatty acid, especially short-chain fatty acids (SCFAs), that play an important role in colonic motility. The intestinal microorganism ferment dietary fiber produces SCFAs, mainly acetic acid, propionic acid, and butyric acid. In the intestine, SCFAs not only act as nutrients for intestinal epithelial cells, but also serve to regulate intestinal pH, cell proliferation and differentiation, and gene expression (31, 32).
We also found that SCFAs have an important role in other diseases. Tan et al. reported low SCFAs in Parkinson's disease were significantly associated with poorer cognition. Lower butyrate levels correlated with worse postural instability-gait disorder scores (33). Liu et al. indicated that altered fecal microbiota might play vital roles in the pathogenesis of pediatric myasthenia gravis by reducing SCFAs. The microbial markers might serve as novel diagnostic methods for pediatric myasthenia gravis (34). Therefore, the intestinal microbiota in patients with STC may affect gut motility by altering host metabolism.
Metagenomics analysis showed that there was significant difference in the methane metabolism pathway between the two groups. This finding is particularly intriguing because one of the main clinical symptoms of patients with constipation is abdominal distension caused by excessive methane production. Compared with the healthy control group, methane metabolism pathways were significantly enriched in the STC group. Previous studies have shown that methane negatively affects intestinal motility, slowing colonic transit time (35, 36). This is the first study to focus on the correlation between methane and STC at the metagenomic level. On the basis of the above findings, we believe that methane quantification could be used to diagnose STC (37, 38).
There are several limitations of this study. First, we have collected for testing is natural feces, which are different from mucosal adherent bacteria. A cause–effect relationship between microbiome and constipation needs to be evaluated, especially for mucosal microbiome. Secondary, a thorough dietary survey was not performed prior to stool collection. Diet is a strong determinant of the microbial microenviroment. Constipated individuals are reported to consume fewer calories as well as lesser amount of protein and fat, but possibly increased amount of fibers and all of the above factors would affect the colonic microbiota and transit time. Thirdly, these diagnosis biomarkers should be tested through a larger clinical sample size and should be discussed to explore the application in future clinical diagnosis and treatment of STC. Fourth, we review the pros and cons of the current high-throughput methods. After isolating whole-genomic DNAs from a fecal sample, the high-throughput identification of 16S ribosomal DNA (rDNA) by Next Generation Sequencing (NGS) alone generates enough information for high-throughput identification of microbes constituting gut microbiota. NGS methods are the best approaches. However, this technology is far from becoming commonplace and affordable because of the high cost and infrastructure required for the analysis. In addition, this method cannot dynamically reflect the changes and effects of the intestinal flora and is gradually being replaced by metabolomics, transcriptomics, proteomics, bacterial culture, and fluorescence in situ hybridization (FISH), which facilitates the understanding the link between individual bacterial cells and their metabolic functions. Our results provide new avenues for the development of novel diagnostic tools and potential treatments. Furthermore, our findings suggest that alteration of the microbiome may lead to constipation by changing the levels of microbial-derived metabolites in the gut and that restoring balance to the disrupted microbiota could help to improve the clinical phenotype. Further experimental or preclinical studies are necessary to examine the role of the altered microbiota in the pathogenesis of colonic motility changes.
Conclusion
In this study, we have reviewed the association of gut microbiome composition with STC as well as its possible roles in the development of this disease. We revealed a novel link between the gut microbiome, host genome, and pathology of STC. This study will be a platform model of the microbiome studies to elucidate etiology of this disease, evidenced by the changes in genes, pathways, and various taxonomic levels. Discovery of the associated microbes of STC in the gut microbiome may help us to seek more intestinal microbial agents for this disease.
Data Availability Statement
The data presented in the study are deposited in the: https://www.ncbi.nlm.nih.gov/bioproject/779475 repository, accession number PRJNA779475.
Ethics Statement
The studies involving human participants were reviewed and approved by the Ethics Committee of Tenth Hospital of Tongji University. The patients/participants provided their written informed consent to participate in this study. Written informed consent was obtained from the individual(s) for the publication of any potentially identifiable images or data included in this article.
Author Contributions
HT, JC, CW, SZ, and QC conceived the method. ZZ, NQ, XL, and HQ designed the experiments. XL, SZ, CY, JC, and NL performed the experiments and implemented the method. ZZ, CW, HT, NQ, NL, and HQ assessed the data and planned the statistical analyses. HT, BY, JC, CY, and QC wrote and edited the manuscript. All authors contributed to the article and approved the submitted version of the manuscript.
Funding
This study was supported by the National Natural Science Foundation of China (No. 82100698) and the Clinical Research Plan of Shenkang Hospital Development Center (No. SHDC2020CR4026 and SHDC2020CR1030B).
Conflict of Interest
ZZ, CW, and NQ was employed by company Realbio Genomics Institute.
The remaining authors declare that the research was conducted in the absence of any commercial or financial relationships that could be construed as a potential conflict of interest.
Publisher's Note
All claims expressed in this article are solely those of the authors and do not necessarily represent those of their affiliated organizations, or those of the publisher, the editors and the reviewers. Any product that may be evaluated in this article, or claim that may be made by its manufacturer, is not guaranteed or endorsed by the publisher.
Acknowledgments
The authors would like to thank Chao Ding and Xiaolong Ge for their helpful advice and discussion.
Supplementary Material
The Supplementary Material for this article can be found online at: https://www.frontiersin.org/articles/10.3389/fmed.2021.777961/full#supplementary-material
References
1. Emmanuel A, Cools M, Vandeplassche L, Kerstens R. Prucalopride improves bowel function and colonic transit time in patients with chronic constipation: an integrated analysis. Am J Gastroenterol. (2014) 109:887–94. doi: 10.1038/ajg.2014.74
2. Bharucha AE, Pemberton JH, Locke GR III. American gastroenterological association technical review on constipation. Gastroenterology. (2013) 144:218–38. doi: 10.1053/j.gastro.2012.10.028
3. Wald A, Scarpignato C, Kamm MA, Mueller-Lissner S, Helfrich I, Schuijt C, et al. The burden of constipation on quality of life: results of a multinational survey. Aliment Pharmacol Ther. (2007) 26:227–36. doi: 10.1111/j.1365-2036.2007.03376.x
4. Zoppi G, Cinquetti M, Luciano A, Benini A, Muner A, Bertazzoni Minelli E. The intestinal ecosystem in chronic functional constipation. Acta Paediatr. (1998) 87:836–41. doi: 10.1111/j.1651-2227.1998.tb01547.x
5. Khalif IL, Quigley EM, Konovitch EA, Maximova ID. Alterations in the colonic flora and intestinal permeability and evidence of immune activation in chronic constipation. Dig Liver Dis. (2005) 37:838–49. doi: 10.1016/j.dld.2005.06.008
6. Zhu L, Liu W, Alkhouri R, Baker RD, Bard JE, Quigley EM, et al. Structural changes in the gut microbiome of constipated patients. Physiol Genomics. (2014) 46:679–86. doi: 10.1152/physiolgenomics.00082.2014
7. Kang DW, DiBaise JK, Ilhan ZE, Crowell MD, Rideout JR, Caporaso JG, et al. Gut microbial and short-chain fatty acid profiles in adults with chronic constipation before and after treatment with lubiprostone. Anaerobe. (2015) 33:33–41. doi: 10.1016/j.anaerobe.2015.01.005
8. Huang LS, Kong C, Gao RY, Yan X, Yu HJ, Wen B, et al. Analysis of fecal microbiota in patients with functional constipation undergoing treatment with synbiotics. Eur J Clin Microbiol Infect Dis. (2018) 37:555–63. doi: 10.1007/s10096-017-3149-7
9. Huang L, Zhu Q, Qu X, Qin H. Microbial treatment in chronic constipation. Sci China Life Sci. (2018) 61:744–52. doi: 10.1007/s11427-017-9220-7
10. Vandeputte D, Falony G, Vieira-Silva S, Tito RY, Joossens M, Raes J. Stool consistency is strongly associated with gut microbiota richness and composition, enterotypes and bacterial growth rates. Gut. (2016) 65:57–62. doi: 10.1136/gutjnl-2015-309618
11. Le Chatelier E, Nielsen T, Qin J, Prifti E, Hildebrand F, Falony G, et al. Richness of human gut microbiome correlates with metabolic markers. Nature. (2013) 500:541–6. doi: 10.1038/nature12506
12. Qin N, Yang F, Li A, Prifti E, Chen Y, Shao L, et al. Alterations of the human gut microbiome in liver cirrhosis. Nature. (2014) 513:59–64. doi: 10.1038/nature13568
13. Metcalf AM, Phillips SF, Zinsmeister AR, MacCarty RL, Beart RW, Wolff BG. Simplified assessment of segmental colonic transit. Gastroenterology. (1987) 92:40–7. doi: 10.1016/0016-5085(87)90837-7
14. Southwell BR, Clarke MC, Sutcliffe J, Hutson JM. Colonic transit studies: normal values for adults and children with comparison of radiological and scintigraphic methods. Pediatr Surg Int. (2009) 25:559–72. doi: 10.1007/s00383-009-2387-x
15. Xie Y, Wu G, Tang J, Luo R, Patterson J, Liu S, et al. SOAPdenovo-Trans: de novo transcriptome assembly with short RNA-Seq reads. Bioinformatics. (2014) 30:1660–6. doi: 10.1093/bioinformatics/btu077
16. Li W, Godzik A. Cd-hit: a fast program for clustering and comparing large sets of protein or nucleotide sequences. Bioinformatics. (2006) 22:1658–9. doi: 10.1093/bioinformatics/btl158
17. Parameswaran P, Torres CI, Lee HS, Krajmalnik-Brown R, Rittmann BE. Syntrophic interactions among anode respiring bacteria (ARB) and Non-ARB in a biofilm anode: electron balances. Biotechnol Bioeng. (2009) 103:513–23. doi: 10.1002/bit.22267
18. Yu D, Yang Y, Long J, Xu W, Cai Q, Wu J, et al. Long-term diet quality and gut microbiome functionality: a prospective, shotgun metagenomic study among Urban Chinese Adults. Curr Dev Nutr. (2021) 5:nzab026. doi: 10.1093/cdn/nzab026
19. Arumugam M, Raes J, Pelletier E, Le Paslier D, Yamada T, Mende DR, et al. Enterotypes of the human gut microbiome. Nature. (2011) 473:174–80. doi: 10.1038/nature09944
20. Shahid S, Ramzan Z, Maurer AH, Parkman HP, Fisher RS. Chronic idiopathic constipation: more than a simple colonic transit disorder. J Clin Gastroenterol. (2012) 46:150–4. doi: 10.1097/MCG.0b013e318231fc64
21. Xu F, Cheng Y, Ruan G, Fan L, Tian Y, Xiao Z, et al. New pathway ameliorating ulcerative colitis: focus on Roseburia intestinalis and the gut-brain axis. Therap Adv Gastroenterol. (2021) 14:17562848211004469. doi: 10.1177/17562848211004469
22. Qin Q, Yan S, Yang Y, Chen J, Li T, Gao X, et al. A metagenome-wide association study of the gut microbiome and metabolic syndrome. Front Microbiol. (2021) 12:682721. doi: 10.3389/fmicb.2021.682721
23. Hadizadeh F, Walter S, Belheouane M, Bonfiglio F, Heinsen FA, Andreasson A, et al. Stool frequency is associated with gut microbiota composition. Gut. (2017) 66:559–60. doi: 10.1136/gutjnl-2016-311935
24. Barbara G, Feinle-Bisset C, Ghoshal UC, Quigley EM, Santos J, Vanner S, et al. The intestinal microenvironment and functional gastrointestinal disorders. Gastroenterology. (2016) 2016:S0016–5085. doi: 10.1053/j.gastro.2016.02.028
25. Eckburg PB, Bik EM, Bernstein CN, Purdom E, Dethlefsen L, Sargent M, et al. Diversity of the human intestinal microbial flora Science. (2005) 308:1635–8. doi: 10.1126/science.1110591
26. Hayashi H, Sakamoto M, Benno Y. Phylogenetic analysis of the human gut microbiota using 16S rDNA clone libraries and strictly anaerobic culture-based methods. Microbiol Immunol. (2002) 46:535–48. doi: 10.1111/j.1348-0421.2002.tb02731.x
27. Brial F, Chilloux J, Nielsen T, Vieira-Silva S, Falony G, Andrikopoulos P, et al.Human and preclinical studies of the host-gut microbiome co-metabolite hippurate as a marker and mediator of metabolic health. Gut. (2021) 70:2105–14. doi: 10.1136/gutjnl-2020-323314
28. Lu J, Zhang L, Zhai Q, Zhao J, Zhang H, Lee YK, et al. Chinese gut microbiota and its associations with staple food type, ethnicity, and urbanization. NPJ Biofilms Microbiomes. (2021) 7:71. doi: 10.1038/s41522-021-00245-0
29. Seong E, Bose S, Han SY, Song EJ, Lee M, Nam YD, et al. Positive influence of gut microbiota on the effects of Korean red ginseng in metabolic syndrome: a randomized, double-blind, placebo-controlled clinical trial. EPMA J. (2021) 12:177–97. doi: 10.1007/s13167-021-00243-4
30. Vital M, Howe AC, Tiedje JM. Revealing the bacterial butyrate synthesis pathways by analyzing (meta)genomic data. mBio. (2014) 5:e00889. doi: 10.1128/mBio.00889-14
31. Bai J, Li Y, Zhang W, Fan M, Qian H, Zhang H, et al. Effects of cereal fibers on short-chain fatty acids in healthy subjects and patients: a meta-analysis of randomized clinical trials. Food Funct. (2021) 12:7040–53. doi: 10.1039/D1FO00858G
32. Kim MG, Jo K, Chang YB, Suh HJ, Hong KB. Changes in the gut microbiome after galacto-oligosaccharide administration in loperamide-induced constipation. J Pers Med. (2020) 10:161. doi: 10.3390/jpm10040161
33. Tan AH, Chong CW, Lim SY, Yap IKS, Teh CSJ, Loke MF, et al. Gut microbial ecosystem in parkinson disease: new clinicobiological insights from multi-omics. Ann Neurol. (2021) 89:546–59. doi: 10.1002/ana.25982
34. Liu P, Jiang Y, Gu S, Xue Y, Yang H, Li Y, et al. Metagenome-wide association study of gut microbiome revealed potential microbial marker set for diagnosis of pediatric myasthenia gravis. BMC Med. (2021) 19:159. doi: 10.1186/s12916-021-02034-0
35. Pimentel M, Saad RJ, Long MD, Rao SSC. ACG clinical guideline: small intestinal bacterial overgrowth. Am J Gastroenterol. (2020) 115:165–78. doi: 10.14309/ajg.0000000000000501
36. Attaluri A, Jackson M, Valestin J, Rao SS. Methanogenic flora is associated with altered colonic transit but not stool characteristics in constipation without IBS. Am J Gastroenterol. (2010) 105:1407–11. doi: 10.1038/ajg.2009.655
37. Pimentel M, Lin HC, Enayati P, van den Burg B, Lee HR, Chen JH, et al. Methane, a gas produced by enteric bacteria, slows intestinal transit and augments small intestinal contractile activity. Am J Physiol Gastrointest Liver Physiol. (2006) 290:G1089–95. doi: 10.1152/ajpgi.00574.2004
Keywords: slow transit constipation, gut microbiome, metagenomic analysis, pathogenesis, diagnostic, biomarker
Citation: Tian H, Ye C, Yang B, Cui J, Zheng Z, Wu C, Zhou S, Lv X, Qin N, Qin H, Li N and Chen Q (2022) Gut Metagenome as a Potential Diagnostic and Predictive Biomarker in Slow Transit Constipation. Front. Med. 8:777961. doi: 10.3389/fmed.2021.777961
Received: 16 September 2021; Accepted: 22 November 2021;
Published: 08 February 2022.
Edited by:
Yan-Dong Wang, Beijing University of Chemical Technology, ChinaCopyright © 2022 Tian, Ye, Yang, Cui, Zheng, Wu, Zhou, Lv, Qin, Qin, Li and Chen. This is an open-access article distributed under the terms of the Creative Commons Attribution License (CC BY). The use, distribution or reproduction in other forums is permitted, provided the original author(s) and the copyright owner(s) are credited and that the original publication in this journal is cited, in accordance with accepted academic practice. No use, distribution or reproduction is permitted which does not comply with these terms.
*Correspondence: Qiyi Chen, baiyi_nanjing@163.com
†These authors have contributed equally to this work