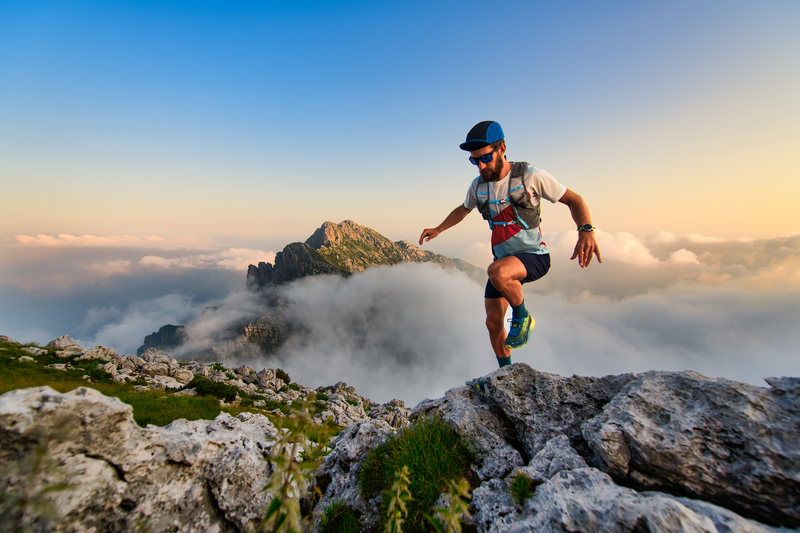
94% of researchers rate our articles as excellent or good
Learn more about the work of our research integrity team to safeguard the quality of each article we publish.
Find out more
ORIGINAL RESEARCH article
Front. Med. , 20 December 2021
Sec. Translational Medicine
Volume 8 - 2021 | https://doi.org/10.3389/fmed.2021.771608
Purpose: Machine Learning (ML) is rapidly growing in capability and is increasingly applied to model outcomes and complications in medicine. Surgical site infections (SSI) are a common post-operative complication in spinal surgery. This study aimed to develop and validate supervised ML algorithms for predicting the risk of SSI following minimally invasive transforaminal lumbar interbody fusion (MIS-TLIF).
Methods: This single-central retrospective study included a total of 705 cases between May 2012 and October 2019. Data of patients who underwent MIS-TLIF was extracted by the electronic medical record system. The patient's clinical characteristics, surgery-related parameters, and routine laboratory tests were collected. Stepwise logistic regression analyses were used to screen and identify potential predictors for SSI. Then, these factors were imported into six ML algorithms, including k-Nearest Neighbor (KNN), Decision Tree (DT), Support Vector Machine (SVM), Random Forest (RF), Multi-Layer Perceptron (MLP), and Naïve Bayes (NB), to develop a prediction model for predicting the risk of SSI following MIS-TLIF under Quadrant channel. During the training process, 10-fold cross-validation was used for validation. Indices like the area under the receiver operating characteristic (AUC), sensitivity, specificity, and accuracy (ACC) were reported to test the performance of ML models.
Results: Among the 705 patients, SSI occurred in 33 patients (4.68%). The stepwise logistic regression analyses showed that pre-operative glycated hemoglobin A1c (HbA1c), estimated blood loss (EBL), pre-operative albumin, body mass index (BMI), and age were potential predictors of SSI. In predicting SSI, six ML models posted an average AUC of 0.60–0.80 and an ACC of 0.80–0.95, with the NB model standing out, registering an average AUC and an ACC of 0.78 and 0.90. Then, the feature importance of the NB model was reported.
Conclusions: ML algorithms are impressive tools in clinical decision-making, which can achieve satisfactory prediction of SSI with the NB model performing the best. The NB model may help access the risk of SSI following MIS-TLIF and facilitate clinical decision-making. However, future external validation is needed.
Minimally invasive transforaminal lumbar interbody fusion (MIS-TLIF) is a classic minimally invasive operation for the treatment of lumbar degenerative diseases such as lumbar disc herniation, lumbar spinal stenosis, and lumbar spondylolisthesis. The operation uses channels to complete decompression and bone graft fusion and fixation. Compared with the traditional open TLIF, it better retains the paravertebral muscle structure, less intraoperative bleeding and faster post-operative recovery (1–3). It has become the mainstream operation scheme for minimally invasive fusion surgery at present.
Although surgical site infections (SSI) following MIS-TLIF are lower than those following open TLIF, previous literature has reported that the incidence of SSI after MIS-TLIF is not uncommon as this procedure is widely used in the clinic (4, 5). Surprisingly, over 20% of the patients who were re-admitted within 30 days after operation were caused by SSI (6, 7). Meanwhile, the rise in the number of spinal surgeries results in an expanding number of SSI, which poses a critical challenge to patients and clinicians. At present, there are studies on the analysis of pre-operative and intraoperative risk factors for SSI after total spinal open surgery, but there is little literature investigating the risk factors for SSI following MIS-TLIF. Previously, most studies only described these risk factors as relative risks (RR) or odds ratios (OR) (7, 8), which are not sufficient to evaluate the risk of SSI following MIS-TLIF. Therefore, a comprehensive prediction model combining risk factors is needed to assess SSI risk for patients. Identification of high-risk surgical populations might help target interventions to patients at high risk, reduce the risk of hospitalization, and improve the clinical outcome.
Machine learning (ML) is a form of artificial intelligence (AI) in which algorithms automatically learn and improve by identifying patterns and complex relationships, with the ultimate goal of making decisions using minimal human intervention (9–13). Through the good performance exhibited by ML algorithms in medical big data, we have the potential to obtain more superior prediction tools than traditional statistical modeling modalities under certain conditions for better prediction of the risk of SSI. It is frustrating that there are currently very few studies training ML algorithms to predict SSI risk. As such, this study aimed to develop and validate ML-based models using pre-operative and intraoperative variables to predict the risk of SSI following MIS-TLIF.
The study was approved by the institutional review board (IRB) of Taizhou Central Hospital and was performed following national and international guidelines. The ethics committee waived the requirement for informed consent due to the retrospective nature of the study. A total of 705 patients, referred from May 2012 to October 2019 to the Department of Orthopedics, Taizhou Central Hospital (Taizhou, China) for MIS-TLIF owing to lumbar spine diseases, were enrolled in this research. The selection criteria were as follows: (1) age ≥ 18 years; (2) diagnosis of lumbar degenerative disease, including lumbar disc herniation, lumbar spinal stenosis, lumbar spondylolisthesis, and lumbar instability; (3) undergoing primary single-or multi-segment MIS-TLIF. The exclusion criteria were as follows: (1) Patients were pre-operatively complicated by active infection in the spine or other parts of the body, cauda equina syndrome, spinal deformity, and tumors. (2) Emergency surgery.
All operations were done by 4 experienced spinal surgeons at our institution. The operation was performed using general anesthesia. C-arm X-ray fluoroscopy accurately located the lesion in the intervertebral space and the root of the upper and lower arches of the vertebrae. A longitudinal incision of ~2.0–3.0 cm of skin and deep fascia was made on the more symptomatic side of the patient along the previously marked pedicle line. The soft tissue between the longest muscle and the multifidus interval was bluntly separated until the vertebral plate was exposed. The Quadrant channel (Medtronic Inc. USA) was placed in the appropriate position after gradual expansion of the cannula, the free arm and cold light source were attached and secured to fully expose the articular eminence, the vertebral plate, and other important anatomic structures. The Quadrant channel was opened, the soft tissue was removed from the field of view under direct vision, and bipolar electrocoagulation was used to adequately stop the hemorrhage. The base diameter is enlarged depending on the reality and was generally dilated to 8–9 cm. The superior lamina and some articular processes were removed by nucleus pulposus forceps, the ligamentum flavum was cleaned, the dura was revealed, and the nerve roots were protected and freed. Further revealing the disc space, cutting through the annulus fibrosus, enucleating the nucleus pulposus, and using reamers and curettes to further clean the intervertebral space. A polyetheretherketone (PEEK) interbody graft (Capstone, Medtronic Sofamor Danek) was then inserted. Finally, with the help of the Sextant system (Medtronic Sofamor Danek), percutaneous lumbar pedicle screws were inserted bilaterally. A drainage tube was placed after washing, and then the layer was sutured and the incision was closed. The protocol for prevention of SSI in this central was described in more detail in Supplementary Material. Patients were followed for a minimum of 1 year after the index operation to monitor all complications and incidences of revision surgery. All complications were recorded by the available electronic medical system. The primary outcome of interest was the occurrence of SSI. The diagnosis of SSI was based on the CDC criteria (Center for Disease Control and Prevention) (14). The primary diagnostic criteria for SSI were as follows: (1) the surgical wound showed redness, swelling, heat, pain, wave sense or combined with abscess, and drooling, (2) Bacterial culture of wound exudate was positive, (3) Patients with re debridement surgery, positive intraoperative lavage fluid or tissue bacterial culture, and (4) Laboratory tests for blood routine, C-reactive protein, procalcitonin, as well as MRI examination or histopathological examination were performed to confirm SSI.
The personal and medical information of patients, including age, body mass index (BMI), smoking, alcohol, chronic heart failure (CHF), diabetes, COPD, stroke, rheumatic disease, and diagnosis. The pre-operative laboratory examinations, including blood routine examination, glycated hemoglobin A1c (HbA1c), liver function test, serum potassium, and serum calcium, were collected from the electronic medical record (EMR) system. Meanwhile, we recorded the surgery-related parameters, including operation time, intraoperative blood loss, surgical level, and the number of fusion segments in this investigation.
The statistical analyses were performed using SPSS for Windows software (ver. 26.0; SPSS Inc., Chicago, IL, USA). For continuous, normally distributed variables, means were calculated and compared using the independent student t-test; otherwise, the Mann–Whitney U-test was used for group comparisons. The chi-square test was used to analyze qualitative variables. Values of P < 0.05 were considered significant.
In this study, univariate and multivariate logistic regression tests were performed to identify the factors related to SSI following MIS-TLIF. Then, factors that were significant in both univariate and multivariate logistic regression were included and analyzed by stepwise regression. Next, the factors selected by stepwise regression are used as input variables to construct the machine learning (ML) models.
In its most basic sense, machine learning uses programmed algorithms that learn and optimize their operations by analyzing input data to make predictions within an acceptable range (15–18). In this study, 10-fold cross-validation was adopted, which means patients were randomly divided into a training set and a validation set at a ratio of 9:1 in each round. After 10 rounds, where all the patients are used for the test, the evaluation process ends. We developed five types of ML algorithms to model our data: k-Nearest Neighbor (KNN), Decision tree (DT), Support vector machine (SVM), Random Forest (RF), Multi-Layer Perceptron (MLP), Naïve Bayes (NB). When training the prediction model, we used 10-fold cross-validation (CV) on the training data to avoid overfitting and find the optimal hyperparameters. Then, Python software was used to further train the ML algorithms. To assess the performance of the ML algorithms, the area under the receiver operating characteristic (AUC) value, and sensitivity, specificity, and the whole dataset accuracy (ACC) of ML algorithms were reported. Of these, the ACC, sensitivity, and specificity were determined by the following formula:
In these formulas, TP: true positive; TN: true negative; FP: false positive; and FN: false negative. When we evaluated the performance of different ML algorithms, the closer the AUC was to 1, the better the classification performance of the model. In general, AUC > 0.7 indicated good performance of the model. We next determined the AUC values by comparing different ML algorithms, with the best performing algorithm as the final predictive model. Data analysis was performed using programs written in Python programming. The Python packages, including Scikit-learn 0.24.2 (19) and Python 3.8, are adopted for ML algorithms. A two-tailed P < 0.05 was deemed statistically significant. The flowchart of the study is presented in Figure 1.
Figure 1. Overall flowchart of whole process. The final prediction model was determined by the result of maximum AUC and ACC. MIS-TLIF, minimally invasive transforaminal lumbar interbody fusion; SSI, surgical site infection; EMR, electronic medical record; AIC, Akaike information criterion; AUC, area under the curve; ACC, accuracy; KNN, k-Nearest Neighbor; DT, Decision tree; SVM, Support vector machine; RF, Random Forest; MLP, Multi-Layer Perceptron; NB, Naïve bayes.
In this study, a total of 705 cases were included, 401 (56.9%) males and 304 (43.1%) females, mean (58.7 ± 7.2) years old. Among the 705 cases, SSI occurred in 33 (4.68%) cases, i.e., the SSI group; other cases were in the Non-SSI group. Among the SSI and Non-SSI groups, there were statistically significant differences in age (p < 0.001), BMI (p < 0.001), smoking (p = 0.002), diabetes (p < 0.001), ASA (p = 0.005), operation time (p < 0.001), estimated blood loss (EBL) (p < 0.001), number of fusion segments (p < 0.001), pre-operative HbA1c (p < 0.001), pre-operative albumin (p < 0.001), and pre-operative fibrinogen (p < 0.001). There were no significant differences in remaining parameters between the SSI and Non-SSI groups. The detailed results of the parameters are shown in Table 1.
In univariable analysis, age, BMI, smoking, diabetes, ASA, operation time, EBL, number of fusion segments, pre-operative HbA1c, pre-operative Alb, pre-operative glycated protein, and pre-operative fibrinogen were all significantly associated with the occurrence of SSI in the overall population (P < 0.05; Table 2). In multivariable logistic regression analysis, factors with statistical significance included age, BMI, operation time, EBL, pre-operative HbA1c, and pre-operative Alb (P < 0.05; Table 2). Stepwise logistic regression analyses identified the optimal subset of variables including age (OR 1.187, 95% CI 1.006–1.401), BMI (OR 1.445, 95% CI 1.005–2.076), pre-operative HbA1c (OR 35.390, 95% CI 21.215–40.124), EBL (OR 1.424, 95% CI 1.021–1.732), operation time (OR 1.041, 95% CI 1.003–1.080) (Table 3).
Table 2. Univariate and multivariate logistic regression model analyses of SSI following MIS-TLIF in the whole data set.
Comparisons of the performance of prediction among the six ML algorithms models in the whole set are detailed in Figure 2. The result of the 10-fold CV of six ML algorithms in the whole set was demonstrated in Figure 3. It turned out that the NB model demonstrated the highest performance in predicting SSI, whose average AUC was 0.78, sensitivity 0.93, specificity 0.82, and accuracy 0.90 in whole sets. Accordingly, we chose the NB model as the final prediction model.
Figure 2. (A) ROC curve analysis of machine learning algorithms for predicting the risk of SSI following MIS-TLIF in total set. (B) Predictive performance comparison of the six types of machine learning algorithms in the total set. SSI, surgical site infection; MIS-TLIF, minimally invasive transforaminal lumbar interbody fusion; ROC, Receiver Operating Characteristic curve; KNN, k-Nearest Neighbor; DT, Decision tree; SVM, Support vector machine; RF, Random Forest; MLP, Multi-Layer Perceptron; NB, Naïve bayes.
Figure 3. ROC curve analysis of a 10-fold CV of six machine learning algorithms for predicting the risk of surgical site infection following minimally invasive transforaminal lumbar interbody fusion in total set. CV, cross validation; KNN, k-Nearest Neighbor; DT, Decision tree; SVM, Support vector machine; RF, Random Forest; MLP, Multi-Layer Perceptron; NB, Naïve bayes.
According to the indicators, the NB model is determined as the final prediction model. The relative importance of variables in the NB model was shown in Figure 4. The importance of high-ranking variables in the NB model was arranged as follows in descending order: pre-operative HbA1c, EBL, operation time, pre-operative Alb, BMI, and age.
Figure 4. The importance of the variables in the NB model model is in decreasing order as follows: Pre-operative HbA1c, EBL, operation time, pre-operative Alb, BMI, and age. HbA1c, glycated hemoglobin A1c; EBL, estimated blood loss; Alb, albumin; BMI, body mass index.
In this work, we developed and validated multiple ML algorithms to predict the risk of SSI following MIS-TLIF under the Quadrant channel. We found that the NB model provided improved predictive ability compared to other models. This study produced interesting findings concerning the added value of machine-learning methods, contrary to some of the wider literature on clinical prediction models. This ML-based model may potentially assist clinical decision-making when assessing and advising patients after MIS-TLIF under the Quadrant channel and when deciding on ongoing management.
MIS-TLIF is well-known to have many advantages compared to open TLIF in terms of reducing muscle injury, smaller incisions, and scarring, especially for elderly patients who cannot bear the trauma of traditional open TLIF surgery (20–22). In contrast, SSI after MIS-TLIF can cause additional prolonged antibiotic use, prolonged length of stay, increased risk of fusion failure, and even the need for re-invasive surgery or removal of the implant (23, 24), which not only increases the medical burden on patients and society but also causes increased mortality in patients.
With the continuous development of minimally invasive spine surgery, MIS-TLIF has gradually attracted the attention of spine surgeons. It has been reported that the incidence of SSI after lumbar interbody fusion is 2.4–5% (25–28). In this investigation, the incidence of SSI was 4.78, which was consistent with the previous report. Although risk factors associated with SSI after MIS-TLIF have been previously reported, little attention has been paid to the incidence of SSI following MIS-TLIF under the Quadrant channel. More importantly, we still lack a concise and reliable tool to identify high-risk groups and assist clinicians in their decision-making. Therefore, in this study, several ML-based models were constructed using AI techniques to predict the risk of SSI.
Many studies have shown that diabetes mellitus is an important risk factor for SSI after spine surgery (23, 29–31). Usually, glucose metabolism is closely related to the structure and pathophysiology of blood vessels. Disorders of glucose metabolism are inextricably linked to vascular structure and dysfunction, as has been demonstrated in many studies. And vascular pathological alterations cause local microcirculatory disorders leading to tissue ischemia and hypoxia, which provide a favorable environment for the growth of anaerobic bacteria and pose a potential risk for delayed post-operative wound healing and surgical site infection. Also, systemic immune deficiency caused by diabetes is the main reason for the occurrence of SSI. Therefore, surgeons usually strictly control patients' perioperative blood glucose and control pre-operative fasting glucose below 6.9 mmol/L before considering surgical treatment (32). Interestingly, glycosylated hemoglobin has also been identified as a risk factor for SSI. Chronic hyperglycemic state leads to cell-mediated immune and macrophage dysfunction, reduced overall immune function of the body, and diminished ability to respond to bacterial viruses. And the relative importance in our prediction model was ranked first. Therefore, we need to be more cautious about the perioperative glycemic management of patients.
In the present study, we found that high BMI is also a risk factor for SSI. In a large retrospective study by Onyekwelu et al. (33), BMI > 30 kg/m2 was found to be a high-risk factor for SSI after lumbar spinal fusion. The problems caused by obesity are present in almost all orthopedic surgeries, not only in spine surgery. Obesity can lead to endocrine disruption and insulin resistance, causing impaired glucose tolerance and even diabetes. On the other hand, obese patients may have a variety of underlying diseases that cause a decrease in the patient's immune capacity. Equally worrisome is the increased vascularity in the adipose tissue of obese patients and the even greater incidence of post-operative fat liquefaction, which also increases the risk of SSI.
Intriguingly, age was also identified as an independent risk factor in this study. However, we are also aware that this is a non-modifiable risk factor. Previous studies reported that the risk of SSI after spinal surgery was three times higher in patients aged ≥ 60 years than in the younger group (1, 27). Similar results were observed in the present study. Although it is still controversial whether advanced age is a risk factor for SSI, the current mainstream of scholars believe that elderly patients have reduced metabolism, degenerated immune function, and may be accompanied by numerous underlying diseases, which significantly reduce the reserve capacity of the organism and make it more sensitive to surgical trauma strikes. The ability of local tissues to resist infection as well as self-repair is weakened, increasing the risk of SSI following spine surgery.
Although univariate logistics regression analysis indicated a statistical difference in operation time and EBL between the two groups (P < 0.05), in multivariate logistic regression analysis, we found that EBL and operation time were identified as risk factors for SSI. It has been reported in the literature that prolonged operation time is a significant risk factor for SSI that cannot be ignored. Prolonged operation time leads to increased tissue stretching time, aggravating local ischemia and tissue inflammatory factor release, while prolonged surgical wound exposure leads to an increased possibility of direct blood entry of pathogenic bacteria from the wound. Whereas, the relationship between EBL and SSI has been debatable. According to previous literature, intraoperative blood transfusion >1,000 ml was reported as a risk factor for post-operative SSI in spine surgery and cardiac surgery (7, 27). Onyekwelu et al. (33) investigated 3,175 patients undergoing spine surgery and found that the incidence of post-operative SSI was 2.5% in patients with intraoperative bleeding <1,000 ml, and 3.5% in patients with intraoperative bleeding >1,000 ml. The incidence of SSI was 5.6% (p = 0.001) and was identified as an independent risk factor for SSI.
In the present study, we found that low serum albumin was an important risk factor for SSI. Serum albumin level is closely related to the nutritional status, liver and kidney function of patients. Hypoproteinemia will lead to immune suppression, local tissue edema, impaired microcirculation, and increased tissue fluid exudation, which will affect normal wound healing and create conditions for bacterial colonization. Previous literature has reported pre-operative hypocalcemia as a risk factor for post-operative spinal infection (30), and another interesting finding is that electrolyte variables such as blood calcium and potassium in this investigation were not statistically significantly different between the SSI and Non-SSI groups. Patients with toxic shock syndrome are usually found to suffer from hypocalcemia, which may be due to an increase in calcitonin secretion caused by the infection, resulting in lower blood calcium levels (34). Many previous studies have reported hypocalcemia in ~40% of patients with bacteremia (35). According to the inclusion and exclusion criteria of our study, none of the patients in the 2 groups had a significant active infection before surgery, so it is not difficult to explain why there was no statistically significant difference in pre-operative blood calcium between the 2 groups.
Compared with other research attempting to determine the risk of SSI following MIS-TLIF, our work has several advantages. First, a few studies focused on patients undergoing MIS-TLIF under the Quadrant channel. This may provide a reference for spine surgeons. Furthermore, while ML approaches have shown unparalleled prediction performance in MIS-TLIF under the Quadrant channel in recent reports, There is, however, little research in the available literature on applying ML algorithms to SSI following MIS-TLIF. As far as we know, this is the first study to develop a prediction model using ML algorithms for risk evaluation of SSI with easy-to-use clinical and laboratory data. Fortunately, our prediction model evidences good predictive performance of the model, which distinguishes itself from linear models adopted by previous research.
In this study, several limitations of this study require attention. First, the nature of a retrospective study might have introduced a selection bias. Second, the ML algorithm model we established, to some extent, was confined to one single center, which might restrict its generalizability pending further validation in real-world scenarios. Third, further independent external validation is required to confirm these findings. Finally, we collected as comprehensive a collection of SSI-related variables as possible, but there were still some important variables, such as methicillin-resistant Staphylococcus aureus (MRSA) carriage, that were not available in a timely manner. This could limit the generalizability of the study. Future research was needed to further look into this issue.
We developed and validated ML algorithms for individualized prediction of SSI following MIS-TLIF by utilizing readily available pre-operative variables. The ML-based prediction model can identify whether patients are at high risk of SSI and may assist the clinician in decision making. In the future, we hope to enlarge the sample size in the following study and obtain more reliable achievements further.
The original contributions presented in the study are included in the article/Supplementary Material, further inquiries can be directed to the corresponding author/s.
The study was approved by an Institutional Ethics Committee at the Taizhou Central Hospital (Affiliated Hospital to Taizhou College). Considering that this work was a retrospective study: the Ethics Committee waived the requirement for informed consent from patients.
HW collected the data, analyzed the data, and drafted the manuscript. MY supervised the project, reviewed the manuscript, responsible for the whole project, designed the study, and supervised the study. BY, WL, TF, and QL conceived of the study, participated in its design and coordination, and helped to draft the manuscript. All authors read and approved the final manuscript.
The authors declare that the research was conducted in the absence of any commercial or financial relationships that could be construed as a potential conflict of interest.
All claims expressed in this article are solely those of the authors and do not necessarily represent those of their affiliated organizations, or those of the publisher, the editors and the reviewers. Any product that may be evaluated in this article, or claim that may be made by its manufacturer, is not guaranteed or endorsed by the publisher.
The Supplementary Material for this article can be found online at: https://www.frontiersin.org/articles/10.3389/fmed.2021.771608/full#supplementary-material
BMI, body mass index; COPD, chronic obstructive pulmoriary disease; CHF, chronic heart failure; ASA, American Society of Anesthesiologists; EBL, estimated blood loss; HbA1c, glycated hemoglobin A1c; Alb, albumin; RBC, red blood cells; WBC, white blood cells; PT, prothrombin time; APTT, activated partial thromboplastin time; Fib, fibrinogen; MIS-TLIF, minimally invasive transforaminal lumbar interbody fusion; CV, cross-validation; KNN, k-Nearest Neighbor; DT, Decision tree; SVM, Support vector machine; RF, Random Forest; MLP, Multi-Layer Perceptron; NB, Naïve Bayes; ACC, accuracy; AUC, area under the receiver operating characteristic curve; CV, cross-validation; TP, true positive; TN, true negative; FP, false positive; FN, false negative; OR, odds ratio; CI, confidence interval; ML, machine learning; AI, artificial intelligence; EMR, electronic medical record; MRSA, methicillin-resistant Staphylococcus aureus.
1. Lee W-c, Park J-Y, Kim KH, Kuh SU, Chin DK, Kim KS, Cho YE: Minimally invasive transforaminal lumbar interbody fusion in multilevel: comparison with conventional transforaminal interbody fusion. World Neurosurg. (2016) 85:236–43. doi: 10.1016/j.wneu.2015.09.009
2. Park Y, Ha JW, Lee YT, Sung NY. Minimally invasive transforaminal lumbar interbody fusion for spondylolisthesis and degenerative spondylosis: 5-year results. Clin Orthopaedics Relat Res. (2014) 472:1813–23. doi: 10.1007/s11999-013-3241-y
3. Park Y, Seok S-O, Lee S-B, Ha J-W. Minimally invasive lumbar spinal fusion is more effective than open fusion: a meta-analysis. Yonsei Med J. (2018) 59:524–38. doi: 10.3349/ymj.2018.59.4.524
4. Kulkarni AG, Patel RS, Dutta S. Does minimally invasive spine surgery minimize surgical site infections? Asian Spine J. (2016) 10:1000. doi: 10.4184/asj.2016.10.6.1000
5. O'Toole JE, Eichholz KM, Fessler RG. Surgical site infection rates after minimally invasive spinal surgery. J Neurosurg Spine. (2009) 11:471–6. doi: 10.3171/2009.5.SPINE08633
6. Akins PT, Harris J, Alvarez JL, Chen Y, Paxton EW, Bernbeck J, et al. Risk factors associated with 30-day readmissions after instrumented spine surgery in 14,939 patients. Spine. (2015) 40:1022–32. doi: 10.1097/BRS.0000000000000916
7. Kim BD, Smith TR, Lim S, Cybulski GR, Kim JY. Predictors of unplanned readmission in patients undergoing lumbar decompression: multi-institutional analysis of 7016 patients. J Neurosurg Spine. (2014) 20:606–16. doi: 10.3171/2014.3.SPINE13699
8. Adogwa O, Elsamadicy AA, Han JL, Karikari IO, Cheng J, Bagley CA. 30-day readmission after spine surgery: an analysis of 1400 consecutive spine surgery patients. Spine. (2017) 42:520–4. doi: 10.1097/BRS.0000000000001779
9. Bai Z, Hu S, Wang Y, Deng W, Gu N, Zhao R, et al. Development of a machine learning model to predict the risk of late cardiogenic shock in patients with ST-segment elevation myocardial infarction. Ann Transl Med. (2021) 9:1162. doi: 10.21037/atm-21-2905
10. Hochman E, Feldman B, Weizman A, Krivoy A, Gur S, Barzilay E, et al. Development and validation of a machine learning-based postpartum depression prediction model: a nationwide cohort study. Depress Anxiety. (2021) 38:400–11. doi: 10.1002/da.23123
11. Pollock KG, Sekelj S, Johnston E, Sandler B, Hill NR, Ng FS, et al. Application of a machine learning algorithm for detection of atrial fibrillation in secondary care. Int J Cardiol Heart Vasc. (2020) 31:100674. doi: 10.1016/j.ijcha.2020.100674
12. Qin H, Hu X, Zhang J, Dai H, He Y, Zhao Z, et al. Machine-learning radiomics to predict early recurrence in perihilar cholangiocarcinoma after curative resection. Liver Int. (2021) 41:837–50. doi: 10.1111/liv.14763
13. Segal Z, Kalifa D, Radinsky K, Ehrenberg B, Elad G, Maor G, et al. Machine learning algorithm for early detection of end-stage renal disease. BMC Nephrol. (2020) 21:518. doi: 10.1186/s12882-020-02093-0
14. Mangram AJ, Horan TC, Pearson ML, Silver LC, Jarvis WR, Committee HICPA. Guideline for prevention of surgical site infection 1999. Infect Control Hosp Epidemiol. (1999) 20:247–80. doi: 10.1086/501620
15. Shin S, Austin PC, Ross HJ, Abdel-Qadir H, Freitas C, Tomlinson G, et al. Machine learning vs. conventional statistical models for predicting heart failure readmission and mortality. ESC Heart Fail. (2021) 8:106–15. doi: 10.1002/ehf2.13073
16. Siu BMK, Kwak GH, Ling L, Hui P. Predicting the need for intubation in the first 24 h after critical care admission using machine learning approaches. Sci Rep. (2020) 10:20931. doi: 10.1038/s41598-020-77893-3
17. Sofo L, Caprino P, Schena C, Sacchetti F, Potenza A, Ciociola A. New perspectives in the prediction of postoperative complications for high-risk ulcerative colitis patients: machine learning preliminary approach. Eur Rev Med Pharmacol Sci. (2020) 24:12781–7.
18. Tewari P, Kashdan E, Walsh C, Martin CM, Parnell AC, O'Leary JJ. Estimating the conditional probability of developing human papilloma virus related oropharyngeal cancer by combining machine learning and inverse Bayesian modelling. PLoS Comput Biol. (2021) 17:e1009289. doi: 10.1371/journal.pcbi.1009289
19. Pedregosa F, Varoquaux G, Gramfort A, Michel V, Thirion B, Grisel O, et al. Scikit-learn: machine learning in Python. J Mach Learn Res. (2011) 12:2825–30. Available online at: https://www.jmlr.org/papers/volume12/pedregosa11a/pedregosa11a.pdf?source=post_page
20. Kim J-S, Jung B, Lee S-H. Instrumented minimally invasive spinal-transforaminal lumbar interbody fusion (MIS-TLIF). Clin Spine Surg. (2018) 31:E302–9. doi: 10.1097/BSD.0b013e31827415cd
21. Lee KH, Yeo W, Soeharno H, Yue WM. Learning curve of a complex surgical technique: minimally invasive transforaminal lumbar interbody fusion (MIS TLIF). Clin Spine Surg. (2014) 27:E234–40. doi: 10.1097/BSD.0000000000000089
22. Tan JH, Liu G, Ng R, Kumar N, Wong H-K. Is MIS-TLIF superior to open TLIF in obese patients?: a systematic review and meta-analysis. Eur Spine J. (2018) 27:1877–86. doi: 10.1007/s00586-018-5630-0
23. Gum JL, Carreon LY, Stimac JD, Glassman SD. Predictors of oswestry disability index worsening after lumbar fusion. Orthopedics. (2013) 36:e478–83. doi: 10.3928/01477447-20130327-26
24. Hart RA, Cabalo A, Bess S, Akbarnia BA, Boachie-Adjei O, Burton D, et al. Comparison of patient and surgeon perceptions of adverse events after adult spinal deformity surgery. Spine. (2013) 38:732–6. doi: 10.1097/BRS.0b013e31827ae242
25. Lim S, Edelstein AI, Patel AA, Kim BD, Kim J. Risk factors for postoperative infections after single-level lumbar fusion surgery. Spine. (2018) 43:215–22. doi: 10.1097/BRS.0000000000000608
26. Liu J-M, Deng H-L, Chen X-Y, Zhou Y, Yang D, Duan M-S, et al. Risk factors for surgical site infection after posterior lumbar spinal surgery. Spine. (2018) 43:732–7. doi: 10.1097/BRS.0000000000002419
27. Sebastian A, Huddleston III P, Kakar S, Habermann E, Wagie A, Nassr A. Risk factors for surgical site infection after posterior cervical spine surgery: an analysis of 5,441 patients from the ACS NSQIP 2005–2012. Spine J. (2016) 16:504–9. doi: 10.1016/j.spinee.2015.12.009
28. Veeravagu A, Patil CG, Lad SP, Boakye M. Risk factors for postoperative spinal wound infections after spinal decompression and fusion surgeries. Spine. (2009) 34:1869–72. doi: 10.1097/BRS.0b013e3181adc989
29. Deng H, Chan AK, Ammanuel SG, Chan AY, Oh T, Skrehot HC, et al. Risk factors for deep surgical site infection following thoracolumbar spinal surgery. J Neurosurg Spine. (2019) 32:292–301. doi: 10.3171/2019.8.SPINE19479
30. Klemencsics I, Lazary A, Szoverfi Z, Bozsodi A, Eltes P, Varga PP. Risk factors for surgical site infection in elective routine degenerative lumbar surgeries. Spine J. (2016) 16:1377–83. doi: 10.1016/j.spinee.2016.08.018
31. Yao R, Zhou H, Choma TJ, Kwon BK, Street J. Surgical site infection in spine surgery: who is at risk? Global Spine J. (2018) 8(Suppl. 4):5S−30S. doi: 10.1177/2192568218799056
32. Olsen MA, Nepple JJ, Riew KD, Lenke LG, Bridwell KH, Mayfield J, et al. Risk factors for surgical site infection following orthopaedic spinal operations. JBJS. (2008) 90:62–9. doi: 10.2106/JBJS.F.01515
33. Onyekwelu I, Glassman SD, Asher AL, Shaffrey CI, Mummaneni PV, Carreon LY. Impact of obesity on complications and outcomes: a comparison of fusion and nonfusion lumbar spine surgery. J Neurosurg Spine. (2017) 26:158–62. doi: 10.3171/2016.7.SPINE16448
34. Aderka D, Schwartz D, Dan M, Levo Y. Bacteremic hypocalcemia: a comparison between the calcium levels of bacteremic and nonbacteremic patients with infection. Arch Intern Med. (1987) 147:232–6. doi: 10.1001/archinte.147.2.232
Keywords: surgical infection sites, machine learning, risk factors, minimally invasive transforaminal lumbar interbody fusion, prediction model
Citation: Wang H, Fan T, Yang B, Lin Q, Li W and Yang M (2021) Development and Internal Validation of Supervised Machine Learning Algorithms for Predicting the Risk of Surgical Site Infection Following Minimally Invasive Transforaminal Lumbar Interbody Fusion. Front. Med. 8:771608. doi: 10.3389/fmed.2021.771608
Received: 06 September 2021; Accepted: 30 November 2021;
Published: 20 December 2021.
Edited by:
Vadim Byvaltsev, Irkutsk State Medical University, RussiaReviewed by:
Sergey Mlyavykh, Privolzhsky Research Medical University (PIMU), RussiaCopyright © 2021 Wang, Fan, Yang, Lin, Li and Yang. This is an open-access article distributed under the terms of the Creative Commons Attribution License (CC BY). The use, distribution or reproduction in other forums is permitted, provided the original author(s) and the copyright owner(s) are credited and that the original publication in this journal is cited, in accordance with accepted academic practice. No use, distribution or reproduction is permitted which does not comply with these terms.
*Correspondence: Wenle Li, ZHJsbGVlQDE2My5jb20=; Mingyu Yang, NjE2MzUzMTA4QHFxLmNvbQ==
†These authors have contributed equally to this work and share first authorship
Disclaimer: All claims expressed in this article are solely those of the authors and do not necessarily represent those of their affiliated organizations, or those of the publisher, the editors and the reviewers. Any product that may be evaluated in this article or claim that may be made by its manufacturer is not guaranteed or endorsed by the publisher.
Research integrity at Frontiers
Learn more about the work of our research integrity team to safeguard the quality of each article we publish.