- 1Department of Family Medicine, Chang Gung Memorial Hospital, Linkou Branch, Taoyuan City, Taiwan
- 2Department of Gynecology and Obstetrics, Chang Gung Memorial Hospital, Taoyuan City, Taiwan
- 3Division of General Internal Medicine and Geriatrics, Department of Internal Medicine, Chang Gung Memorial Hospital, Taoyuan City, Taiwan
- 4Department of Family Medicine and Occupation Medicine, MacKay Memorial Hospital, Taipei City, Taiwan
- 5Department of Nursing, and Management, MacKay Junior College of Medicine, Taipei City, Taiwan
- 6Evidence-Based Medicine Center, Taizhou Hospital of Zhejiang Province Affiliated to Wenzhou Medical University, Zhejiang, China
- 7College of Medicine, Chang Gung University, Taoyuan City, Taiwan
Background: This study aimed to quantify the proportion of participants with chronic kidney disease (CKD) and associated metabolic risk factors in a middle-aged and elderly population in Guishan District, Taoyuan City, Taiwan.
Methods: This cross-sectional study enrolled residents aged 50–90 years living in one community. All participants received a standardized personal interview, including a structured questionnaire, anthropometric measurements, and blood samples collected for laboratory testing. CKD was defined as the presence of kidney damage (urine albumin-creatinine ratio ≥30 mg/g) or estimated glomerular filtration rate (eGFR) < 60 mL/min/1.73 m2. Multiple logistic regression models were used to evaluate the risk factors associated with CKD.
Results: A total of 400 participants were enrolled. The overall proportion of participants with CKD was 20.5% (95% confidence interval [CI]: 16.54–24.46%). The proportions of participants with CKD among those aged 50–64, 65–74, and 75 years and over were 17.7, 18.8, and 35.7%, respectively (p = 0.01). Multiple logistic regression model revealed that elevated blood pressure (odds ratio [OR] = 2.23, 95% CI: 1.16–4.30), hyperglycemia (OR = 2.87, 95% CI: 1.64–5.00), hyperuricemia (OR = 1.38, 95% CI: 1.14–1.69), and metabolic syndrome (OR = 2.30, 95% CI: 1.31–4.06) were significantly associated with CKD.
Conclusions: The prevalence of CKD in the study population was high. Hypertension, hyperglycemia, hyperuricemia, and metabolic syndrome are significantly associated with CKD in a middle-aged and elderly population in Taiwan.
Introduction
Taiwan has had the highest prevalence of end-stage renal disease (ESRD) worldwide for more than a decade (1). Besides in Taiwan, the increase in ESRD populations is also of great concern for the United States because ESRD expenditures are gradually consuming greater proportions of the healthcare budget (2). The prevalence of chronic kidney disease (CKD) is higher in older adults, reaching 37.2% in older patients (aged ≥ 65 years), while the prevalence of CKD is 11.93% in adults of all ages (age ≥ 20 years) in Taiwan (3). Treatments for CKD have the greatest effect on slowing the rate of disease progression when started early. CKD can be divided into five stages based on the appearance of kidney damage or estimated glomerular filtration rate (eGFR) < 60 mL/min/1.73 m2. Unfortunately, most patients are unaware of their disorders until they are in later stages (3).
Metabolic syndrome is another global health concern with a rising prevalence. It is defined as a cluster of disorders and risk factors for cardiovascular disease, including abdominal obesity, hyperglycemia (including impaired glucose tolerance and diagnosed diabetes), dyslipidemia, and elevated blood pressure. Many studies have demonstrated a strong association between metabolic syndrome or metabolic components and the risk for subsequent development of type 2 diabetes (4–8), cardiovascular disease (9–13), hyperuricemia, gout (14–16), and CKD (17–21). These factors interact as both cause and effect, and the multiple mechanisms involved in the development of CKD in patients with metabolic syndrome have not been well-established.
This study aimed to quantify the proportion of participants with CKD and associated risk factors, especially metabolic syndrome, in a middle-aged and elderly population in Taiwan. Understanding the proportion of subjects with CKD and important risk factors may lead to early detection of CKD and prevention of ESRD, cardiovascular disease, and reduce associated mortality.
Materials and Methods
Study Design and Participants
This community-based, cross-sectional study enrolled 400 volunteer residents aged 50 years and over who lived in Guishan District, Taoyuan City, Taiwan, between January 2014 and October 2014. We recruited these volunteers at gatherings in towns such as temples and community centers. Each participant received a standardized personal interview, including a structured questionnaire, anthropometric measurements, and collection of blood samples, on a single day.
Data Collection
Data were collected from participants using a structured questionnaire requesting information on smoking habits, physical exercise habits, medical history, and current medications. Height and weight were measured to the nearest 0.1 kg and 0.1 cm using an automatic scale. Body mass index (BMI) was calculated as the ratio of weight to height in meters squared (kg/m2). Waist circumference was measured at the midpoint between the lower border of the rib cage and the upper iliac crest on the mid-axillary line. Blood pressure was determined using an automatic sphygmomanometer on the right upper arm after at least 15 min of rest. Venous blood samples were collected after overnight fasting for at least 12 h. All blood samples were stored in a refrigerator at 4°C and analyzed at the clinical laboratory of Linkou Chang Gung Memorial Hospital, which was certified by the College of American Pathologists. Serum and urine creatinine levels were measured using the isotope dilution mass spectrometry (IDMS) traceable colorimetric method. Urinary albumin levels were measured using a turbidimetric immunoassay. Urine specimens were obtained in the morning and scheduled to avoid contamination by menstrual blood. The study protocol was approved by Chang-Gung Medical Foundation Institutional Review Board (102-2304B), and all participants provided signed informed consent before enrollment.
Definitions of Measurement Cutoffs and Calculations
Chronic kidney disease (CKD) is defined as the presence of kidney damage (urine albumin-creatinine ratio ≥ 30 mg/g) or decreased renal function with an eGFR < 60 mL/min/1.73 m2. The eGFR was calculated using the 2009 Chronic Kidney Disease Epidemiology Collaboration (CKD-EPI) equation, as suggested by the Kidney Disease: Improving Global Outcomes 2012 clinical practice guideline.
2009 CKD-EPI equation:
eGFR = 141 × min (Scr /κ, 1)α × max(Scr /κ, 1)−1.209 × 0.993Age × 1.018 [if female] × 1.159 [if black] Scr is serum creatinine in mg/dL, κ is 0.7 for females and 0.9 for males, α is −0.329 for females and −0.411 for males, min indicates the minimum Scr/κ or 1, max indicates the maximum Scr/κ or 1 (22).
Metabolic syndrome was diagnosed when a participant had at least three of the following five medical conditions, as described by The Third Report of the National Cholesterol Education Program Expert Panel on Adult Treatment Panel Asian diagnostic criteria: (1) elevated blood pressure (systolic blood pressure ≥ 130 mm Hg or diastolic blood pressure ≥ 85 mm Hg, or drug treatment of previously diagnosed hypertension); (2) hyperglycemia (fasting plasma glucose ≥ 100 mg/dL, or established diagnosis of diabetes) (3) hypertriglyceridemia (serum triglyceride ≥ 150 mg/dL, or drug treatment of hypertriglyceridemia); (4) low high-density lipoprotein cholesterol (HDL-C) level (<40 mg/dL for men and <50 mg/dL for women); and (5) central obesity (≥90 cm for males and ≥80 cm for females).
BMI categories were defined as follows: (1) normal weight: BMI < 23 kg/m2; (2) overweight: BMI of 23 to <25 kg/m2; and (3) obesity: BMI ≥25 kg/m2, according to ranges established for Asian populations (23).
Statistical Analysis
Data are presented as mean ± standard deviation (SD) for continuous variables and number of participants (%) for categorical variables. Differences in the mean values of continuous variables were examined using an independent t-test and one-way analysis of variance. The chi-squared test and χ2-trend test were used for differences in proportions between categorical variables. The Mantel–Haenszel χ2 test was used to analyze stratified categorical data. Multiple logistic regression models were developed to investigate the association of five metabolic components of metabolic syndrome and CKD. Traditional factors known to be associated with metabolic factors and CKD were adjusted. We also selected the factors that showed significant differences in CKD and non-CKD participants in the current study as covariates in the regression models. All statistical analyses were performed using SPSS for Windows, SPSS version 27.0.1.0 (SPSS Inc., Chicago, IL). A p-value of <0.05 was considered significant.
Results
Proportions of Participants With Chronic Kidney Disease
As shown in Figure 1, the overall proportion of participants with CKD in the study population (age ≥ 50 years) was 20.5% (95% confidence interval [CI]: 16.54–24.46%). The oldest group had the highest proportion of participants with CKD, whether diagnosed by microalbuminuria, declined eGFR, or either one of them. More than one in three participants older than 75 years old (proportion: 35.7%, 95% CI: 23.16–48.26%) were diagnosed with CKD in this community-based study.
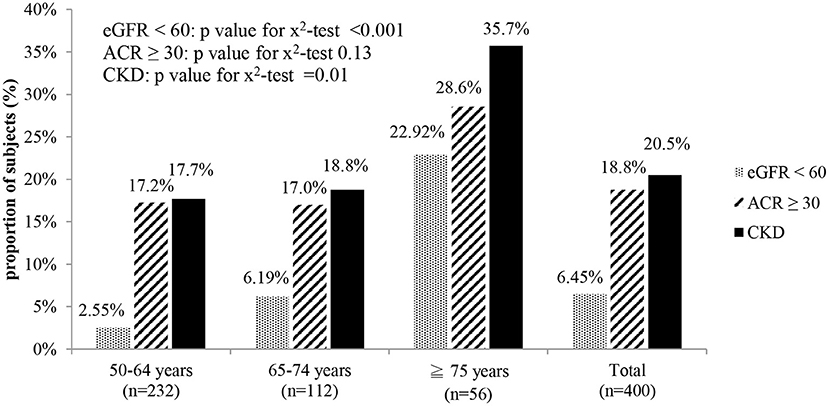
Figure 1. Proportion of subjects with CKD in different age groups. eGFR < 60: estimated glomerular filtration rate < 60 mL/min/1.73 m2. ACR ≥ 30: urine albumin creatinine ratio ≥ 30 mg/g. CKD: eGFR < 60 or ACR ≥ 30.
Clinical Characteristics of the Study Population
A total of 400 participants were categorized into three different age groups: 50–64 years old (n = 232), 65–74 years old (n = 112), and ≥75 years (n = 56). The ratio of waist circumference to height (WC/height), diastolic blood pressure, total cholesterol, low-density lipoprotein cholesterol (LDL-C), alanine aminotransferase (ALT), creatinine, and eGFR were significantly different between the age subgroups (Table 1). We also compared demographic, anthropometric, and clinical characteristics between the CKD and the non-CKD groups and found that age, waist circumference, the ratio of waist circumference to height, systolic blood pressure, diastolic blood pressure, fasting glucose, triglyceride, HDL-C, LDL-C, the ratio of triglyceride to HDL-C, and uric acid were the factors significantly associated with CKD (Table 1).
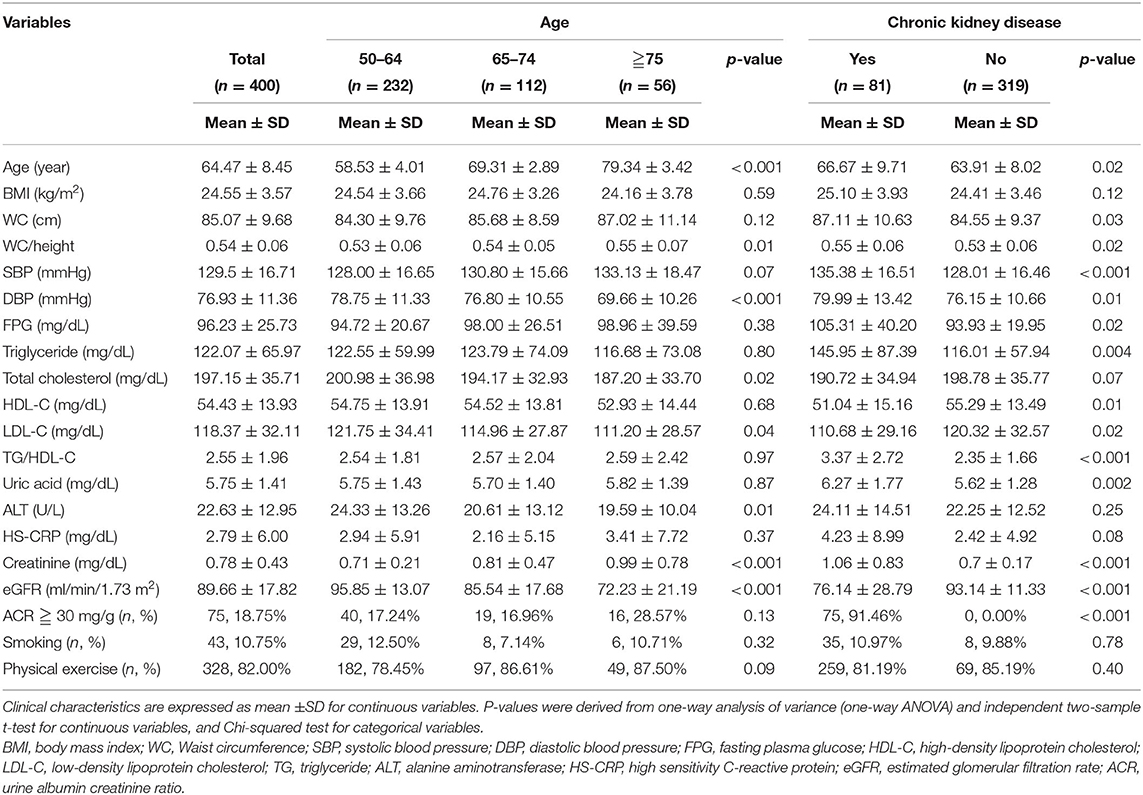
Table 1. Demographic, anthropometric, and biochemical characteristics of screened subjects with and without chronic kidney disease.
Associations Between Metabolic Syndrome and Chronic Kidney Disease
Table 2 shows the associations between the five components of metabolic syndrome and CKD. Elevated blood pressure, hyperglycemia, hypertriglyceridemia, and low HDL-C were significantly associated with an increased age-specific prevalence of CKD, while central obesity showed a similar trend but without statistical significance.
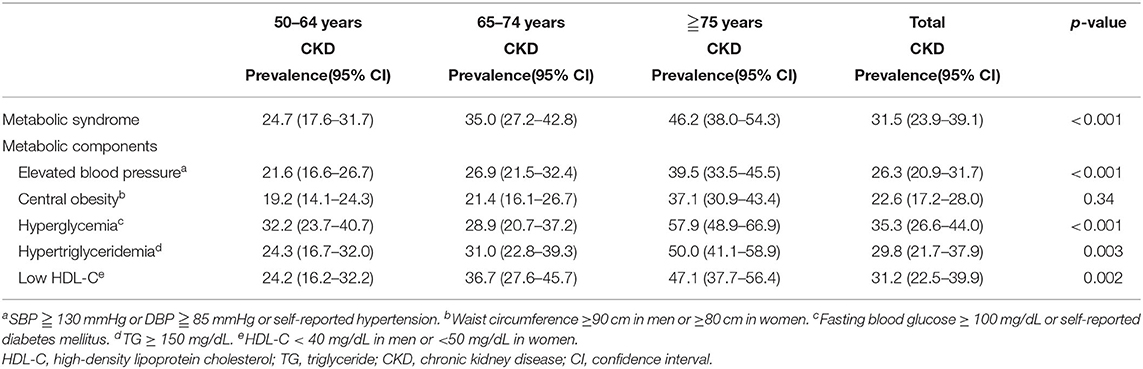
Table 2. Associations between metabolic risk factors and chronic kidney disease in subjects by age group.
The effects of metabolic syndrome on CKD were further examined using multiple logistic regression models (Table 3). Traditional factors known to be associated with metabolic factors and CKD were selected in the models, such as age, sex, and BMI categories. Additional factors showing significant differences in the CKD group in the preliminary analysis (Table 1) were also selected in the models, such as uric acid. After adjusting for age, sex, BMI categories, and uric acid, metabolic syndrome (yes vs. no, odds ratio [OR] = 2.30, 95% CI: 1.31–4.06) were independently significantly associated with CKD (Table 3 model A). Another model was used to examine each of the five components of metabolic syndrome. After adjusting for the above-mentioned confounding factors, elevated blood pressure (yes vs. no, OR = 2.23, 95% CI: 1.16–4.30) and hyperglycemia (yes vs. no, OR = 2.87, 95% CI: 1.64–5.00) were still the metabolic components significantly associated with CKD (Table 3 model B).
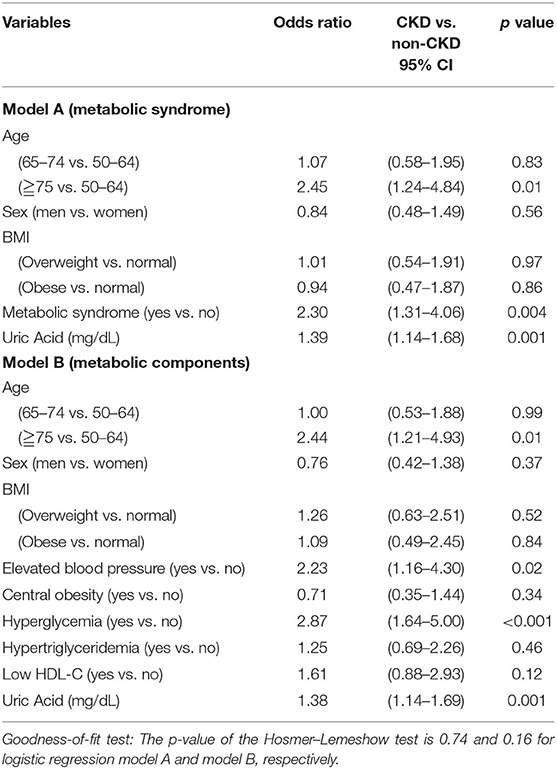
Table 3. Multiple logistic regressions: the effects of metabolic syndrome and metabolic components on the chronic kidney disease among screened population.
Discussion
In our study, hypertension, hyperglycemia, hyperuricemia, and metabolic syndrome were significantly associated with CKD in a middle-aged and older population in Taiwan. In several studies among different countries and races, metabolic syndrome has been identified as a risk factor for developing CKD (24–28). In Japan, Tozawa et al. (29) followed up 6,371 people without CKD or diabetes mellitus for 5 years and found that the relative risk of developing CKD was 1.86 (95% CI: 1.43–2.41, p < 0.0001) in those with metabolic syndrome after adjusting for age, sex, current cigarette smoking, and alcohol drinking habits. In the United States, Kurella et al. (30) enrolled 10,096 nondiabetic participants with 9 years of follow-up, likewise revealing that metabolic syndrome was independently associated with an increased risk for incident CKD in nondiabetic adults; the OR of incident CKD among participants with metabolic syndrome was 1.24 (95% CI, 1.01–1.51) after adjusting for the subsequent development of diabetes and hypertension. In the present study, metabolic syndrome also serves as an independent risk factor for the development of CKD (yes vs. no, OR = 2.43, 95% CI: 1.38–4.29) in a middle-aged and elderly population in Taiwan after adjusting for age, sex, BMI categories, and uric acid.
Each component of metabolic syndrome can cause renal damage; however, the components may not contribute equally to the risk of developing CKD (25, 31, 32). Many studies have further reported the gradient associations between CKD risk and the number of components of metabolic syndrome (29, 30, 32–34). The multiple mechanisms of renal damage caused by each metabolic syndrome component and their interactions with each other are not yet thoroughly understood. In the present study, elevated blood pressure and hyperglycemia were independent risk factors for CKD, while other components did not reach statistical significance after adjusting for confounding factors.
High-normal blood pressure is significantly associated with microalbuminuria when compared with optimal blood pressure, and the increase in urinary protein causes injury to tubular cells, leading to interstitial inflammation and fibrosis (35, 36). Previous studies have also revealed that elevated blood pressure, as a component of metabolic syndrome, is an independent risk factor for CKD development. Cao et al. (37) enrolled 11,274 participants and found that CKD risk was significantly greater (OR, 1.30; 95% CI: 1.03–1.63) in men with high-normal blood pressure than in those with optimal blood pressure. Song et al. (32) followed 75,468 urban workers for 2 years and found that the OR of metabolic syndrome-related to reduced eGFR was 1.43 (95% CI, 1.13–1.83). In addition, lower blood pressure targets (130/80 mmHg) are strongly associated with better renal outcomes (38). Thus, aggressive blood pressure control is suggested for the management of patients with metabolic syndrome and mild renal function decline to promote a better prognosis.
Hyperglycemia, including previously diagnosed diabetes and impaired fasting glucose, is another component of metabolic syndrome that is significantly associated with CKD in the present study. Increased glomerular filtration rate, also called hyperfiltration, is a proposed mechanism for renal injury in diabetes, which has been hypothesized to cause intra-glomerular hypertension leading to albuminuria and reduced glomerular filtration rate. Hyperfiltration also occurs in patients with impaired fasting glucose and can be used as a predictor of diabetic nephropathy (39–42).
Hypertriglyceridemia, low HDL-C levels, and central obesity were not significantly associated with CKD in the present study. Several other studies have reported similar results. Although metabolic syndrome itself is an independent risk factor associated with CKD, dyslipidemia (including both hypertriglyceridemia and low HDL-C level) is not significantly associated with the development of CKD (26, 29, 30, 33, 34, 43). Some studies have shown that hypertriglyceridemia or low HDL-C levels are only significantly associated with the development of CKD in patients with metabolic syndrome (32, 44). Regarding central obesity, a longitudinal study in China found that people with both central and peripheral obesity had higher risks of elevated urine albumin-creatinine ratio, even after adjusting for multiple factors (OR: 1.14, 95% CI: 1.07–1.21, p < 0.001) (45). However, there is no consensus on the role of central obesity in the development of CKD (33, 35, 44, 46, 47). Differences in race, large disparities in participants' ages, the definitions of CKD, and adjusted confounding factors in these studies might be other reasons for discrepancies between studies.
CKD and metabolic syndrome are both considered inflammatory diseases (48, 49). As a downstream marker of inflammation, C-reactive protein is widely used to represent inflammatory conditions. It has been associated with CKD in previous studies (50, 51). However, in our present study, although the mean high sensitive C-reactive protein level was higher in the CKD group, the difference was not statistically significant (Table 1). Further follow-up studies are needed to confirm this association.
The present study has several limitations. First, this was a cross-sectional study; thus, the causal relationship between CKD and the associated risk factors could not be evaluated and determined. Second, the number of participants in this study was relatively small, and all participants were recruited from only a single community. Third, volunteer bias may exist because participation is on a volunteer basis. Volunteer bias is defined as the bias that comes from the fact that a particular sample contains only those participants who volunteer to participate in the study. Those who participate and find the topic particularly interesting are more likely to volunteer, and the same is true of those who are expected to be evaluated on a positive level (52). In addition, we recruited volunteer participants to join our study at gatherings in towns such as temples and community centers. This means that people who require considerable assistance or frequent medical care have a lower possibility of being recruited. Volunteer bias could potentially influence the prevalence of CKD and the associated risk factors, and the association between CKD and associated risk factors in those who require substantial assistance or care might not be observed in the present study. Fourth, it was difficult to collect all the participants' first morning voids in this community-based study. This could lead to biased estimation for some participants' urine albumin-creatinine ratios. Finally, insulin resistance is a known risk factor for CKD, but this has not been discussed in this study, further studies are needed.
Conclusions
CKD is found to be significantly associated with older age, elevated uric acid level, and metabolic syndrome after adjusting for sex and BMI categories in a middle-aged and elderly population in Taiwan. Among the components of metabolic syndrome, elevated blood pressure and hyperglycemia are independently associated with the risk of CKD. For patients with metabolic syndrome, clinical interventions such as lifestyle modification, weight reduction, the use of medications to correct elevated blood pressure, hyperglycemia, dyslipidemia, and hyperuricemia may prevent or delay the progression of CKD.
Data Availability Statement
The original contributions presented in the study are included in the article/supplementary material, further inquiries can be directed to the corresponding author/s.
Ethics Statement
The studies involving human participants were reviewed and approved by Chang-Gung Medical Foundation Institutional Review Board. The patients/participants provided their written informed consent to participate in this study. Written informed consent was obtained from the individual(s) for the publication of any potentially identifiable images or data included in this article.
Author Contributions
Conceived and supervised the study: J-YC. Designed the study: T-HT and J-YC. Performed the study: M-CL and J-YC. Analyzed the data and interpreted the results: M-CL, I-JC, L-TH, Y-JC, M-TT, T-HT, and J-YC. Writing-original draft: M-CL. Writing-editing: M-CL, I-JC, L-TH, T-HT, and J-YC. All authors contributed to the article and approved the submitted version.
Funding
This study was supported by Chang Gung Memorial Hospital (grants CORPG3C0171~3C0172, CZRPG3C0053, CORPG3G0021, CORPG3G0022, and CORPG3G0023).
Conflict of Interest
The authors declare that the research was conducted in the absence of any commercial or financial relationships that could be construed as a potential conflict of interest.
Publisher's Note
All claims expressed in this article are solely those of the authors and do not necessarily represent those of their affiliated organizations, or those of the publisher, the editors and the reviewers. Any product that may be evaluated in this article, or claim that may be made by its manufacturer, is not guaranteed or endorsed by the publisher.
References
1. Wu PC, Wu VC, Tarng DC. Chronic kidney disease in Taiwan's aging population: something far more than a distant ship's smoke on the horizon. J Formos Med Assoc. (2014) 113:890–1. doi: 10.1016/j.jfma.2014.03.013
2. Saran R, Robinson B, Abbott KC, Agodoa LY, Albertus P, Ayanian J, et al. US Renal Data System 2016 annual data report: epidemiology of kidney disease in the United States. Am J Kidney Dis. (2017) 69:A7–A8. doi: 10.1053/j.ajkd.2017.01.036
3. Wen CP, Cheng TYD, Tsai MK, Chang YC, Chan HT, Tsai SP, et al. All-cause mortality attributable to chronic kidney disease: a prospective cohort study based on 462 293 adults in Taiwan. Lancet. (2008) 371:2173–82. doi: 10.1016/S0140-6736(08)60952-6
4. Hanson RL, Imperatore G, Bennett PH, Knowler WC. Components of the “metabolic syndrome” and incidence of type 2 diabetes. Diabetes. (2002) 51:3120–7. doi: 10.2337/diabetes.51.10.3120
5. Resnick HE, Jones K, Ruotolo G, Jain AK, Henderson J, Lu W, et al. Insulin resistance, the metabolic syndrome, and risk of incident cardiovascular disease in nondiabetic american indians: the Strong Heart Study. Diabetes Care. (2003) 26:861–7. doi: 10.2337/diacare.26.3.861
6. Klein BE, Klein R, Lee KE. Components of the metabolic syndrome and risk of cardiovascular disease and diabetes in Beaver Dam. Diabetes Care. (2002) 25:1790–4. doi: 10.2337/diacare.25.10.1790
7. Sattar N, Gaw A, Scherbakova O, Ford I, O'Reilly DS, Haffner SM, et al. Metabolic syndrome with and without C-reactive protein as a predictor of coronary heart disease and diabetes in the West of Scotland Coronary Prevention Study. Circulation. (2003) 108:414–9. doi: 10.1161/01.CIR.0000080897.52664.94
8. Li W, Wang D, Wang X, Gong Y, Cao S, Yin X, et al. The association of metabolic syndrome components and diabetes mellitus: evidence from China National Stroke Screening and Prevention Project. BMC Public Health. (2019) 19:192. doi: 10.1186/s12889-019-6415-z
9. Sattar N, McConnachie A, Shaper AG, Blauw GJ, Buckley BM, de Craen AJ, et al. Can metabolic syndrome usefully predict cardiovascular disease and diabetes? Outcome data from two prospective studies. Lancet. (2008) 371:1927–35. doi: 10.1016/S0140-6736(08)60602-9
10. Ford ES. Risks for all-cause mortality, cardiovascular disease, and diabetes associated with the metabolic syndrome: a summary of the evidence. Diabetes Care. (2005) 28:1769–78. doi: 10.2337/diacare.28.7.1769
11. Galassi A, Reynolds K, He J. Metabolic syndrome and risk of cardiovascular disease: a meta-analysis. Am J Med. (2006) 119:812–9. doi: 10.1016/j.amjmed.2006.02.031
12. Gami AS, Witt BJ, Howard DE, Erwin PJ, Gami LA, Somers VK, et al. Metabolic syndrome and risk of incident cardiovascular events and death: a systematic review and meta-analysis of longitudinal studies. J Am Coll Cardiol. (2007) 49:403–14. doi: 10.1016/j.jacc.2006.09.032
13. Lee MY, Hsiao PJ, Huang JC, Hsu WH, Chen SC, Shin SJ. Association between metabolic syndrome and microvascular and macrovascular disease in type 2 diabetic mellitus. Am J Med Sci. (2018) 355:342–9. doi: 10.1016/j.amjms.2017.12.004
14. Choi HK, Ford ES. Prevalence of the metabolic syndrome in individuals with hyperuricemia. Am J Med. (2007) 120:442–7. doi: 10.1016/j.amjmed.2006.06.040
15. Choi HK, Ford ES, Li C, Curhan G. Prevalence of the metabolic syndrome in patients with gout: the Third National Health and Nutrition Examination Survey. Arthritis Rheum. (2007) 57:109–15. doi: 10.1002/art.22466
16. Liu JH, Ma QH, Xu Y, Chen X, Pan CW. Metabolic syndrome and 5-year incident hyperuricemia among older chinese adults: a community-based cohort study. Diabetes Metab Syndrome Obes Targets Therapy. (2020) 13:4191–200. doi: 10.2147/DMSO.S278542
17. Chen J, Muntner P, Hamm LL, Jones DW, Batuman V, Fonseca V, et al. The metabolic syndrome and chronic kidney disease in U.S. adults. Ann Intern Med. (2004) 140:167–74. doi: 10.7326/0003-4819-140-3-200402030-00007
18. Kittiskulnam P, Thokanit NS, Katavetin P, Susanthitaphong P, Srisawat N, Praditpornsilpa K, et al. The magnitude of obesity and metabolic syndrome among diabetic chronic kidney disease population: a nationwide study. PLoS One. (2018) 13:e0196332. doi: 10.1371/journal.pone.0196332
19. DeBoer MD, Filipp SL, Musani SK, Sims M, Okusa MD, Gurka M. Metabolic syndrome severity and risk of CKD and worsened GFR: the Jackson Heart study. Kidney blood Pressure Res. (2018) 43:555–67. doi: 10.1159/000488829
20. Xie K, Bao L, Jiang X, Ye Z, Bing J, Dong Y, et al. The association of metabolic syndrome components and chronic kidney disease in patients with hypertension. Lipids Health Dis. (2019) 18:229. doi: 10.1186/s12944-019-1121-5
21. Wu M, Shu Y, Wang L, Song L, Chen S, Liu Y, et al. Metabolic syndrome severity score and the progression of CKD. Eur J Clin Invest. (2021) :e13646. doi: 10.1111/eci.13646
22. Levey AS, Stevens LA, Schmid CH, Zhang YL, Castro AF 3rd, Feldman HI, et al. A new equation to estimate glomerular filtration rate. Ann Intern Med. (2009) 150:604–12. doi: 10.7326/0003-4819-150-9-200905050-00006
23. World Health Organization. The Asia-Pacific Perspective: Redefining Obesity and Its Treatment. Australia: Health Communications (2000).
24. Tanaka H, Shiohira Y, Uezu Y, Higa A, Iseki K. Metabolic syndrome and chronic kidney disease in Okinawa, Japan. Kidney Int. (2006) 69:369–74. doi: 10.1038/sj.ki.5000050
25. Iseki K. Metabolic syndrome and chronic kidney disease: a Japanese perspective on a worldwide problem. J Nephrol. (2008) 21:305–12.
26. Saito T, Mochizuki T, Uchida K, Tsuchiya K, Nitta K. Metabolic syndrome and risk of progression of chronic kidney disease: a single-center cohort study in Japan. Heart Vessels. (2013) 28:323–9. doi: 10.1007/s00380-012-0254-5
27. Boronat M, Bosch E, Lorenzo D, Quevedo V, López-Ríos L, Riaño M, et al. Prevalence and determinants of the metabolic syndrome among subjects with advanced nondiabetes-related chronic kidney disease in Gran Canaria, Spain. Ren Fail. (2016) 38:198–203. doi: 10.3109/0886022X.2015.1117904
28. Zomorrodian D, Khajavi-Rad A, Avan A, Ebrahimi M, Nematy M, Azarpazhooh MR, et al. Metabolic syndrome components as markers to prognosticate the risk of developing chronic kidney disease: evidence-based study with 6492 individuals. J Epidemiol Community Health. (2015) 69:594–8. doi: 10.1136/jech-2014-205160
29. Tozawa M, Iseki C, Tokashiki K, Chinen S, Kohagura K, Kinjo K, et al. Metabolic syndrome and risk of developing chronic kidney disease in Japanese adults. Hypertens Res. (2007) 30:937–43. doi: 10.1291/hypres.30.937
30. Kurella M, Lo JC, Chertow GM. Metabolic syndrome and the risk for chronic kidney disease among nondiabetic adults. J Am Soc Nephrol. (2005) 16:2134–40. doi: 10.1681/ASN.2005010106
31. Kuo CM, Chien WH, Shen HC, Hu YC, Chen YF, Tung TH. Clinical epidemiology of reduced kidney function among elderly male fishing and agricultural population in Taipei, Taiwan. Biomed Res Int. (2013) 2013:214128. doi: 10.1155/2013/214128
32. Song H, Wang X, Cai Q, Ding W, Huang S, Zhuo L. Association of metabolic syndrome with decreased glomerular filtration rate among 75,468 Chinese adults: a cross-sectional study. PLoS One. (2014) 9:e113450. doi: 10.1371/journal.pone.0113450
33. Chen J, Gu D, Chen CS, Wu X, Hamm LL, Muntner P, et al. Association between the metabolic syndrome and chronic kidney disease in Chinese adults. Nephrol Dial Transplant. (2007) 22:1100–6. doi: 10.1093/ndt/gfl759
34. Maleki A, Montazeri M, Rashidi N, Montazeri M, Yousefi-Abdolmaleki E. Metabolic syndrome and its components associated with chronic kidney disease. J Res Med Sci. (2015) 20:465–9. doi: 10.4103/1735-1995.163969
35. Knight EL, Kramer HM, Curhan GC. High-normal blood pressure and microalbuminuria. Am J Kidney Dis. (2003) 41:588–95. doi: 10.1053/ajkd.2003.50120
36. Eddy AA. Progression in chronic kidney disease. Adv Chronic Kidney Dis. (2005) 12:353–65. doi: 10.1053/j.ackd.2005.07.011
37. Cao X, Xie X, Xu G, Yuan H, Chen Z. [Cross-sectional study on high-normal blood pressure and chronic kidney disease in occupational physical examination population in Changsha]. Zhong Nan Da Xue Xue Bao Yi Xue Ban. (2014) 39:582–90. doi: 10.11817/j.issn.1672-7347.2014.06.007
38. Jafar TH, Stark PC, Schmid CH, Landa M, Maschio G, de Jong PE, et al. Progression of chronic kidney disease: the role of blood pressure control, proteinuria, and angiotensin-converting enzyme inhibition: a patient-level meta-analysis. Ann Intern Med. (2003) 139:244–52. doi: 10.7326/0003-4819-139-4-200308190-00006
39. Vervoort G, Veldman B, Berden JH, Smits P, Wetzels JF. Glomerular hyperfiltration in type 1 diabetes mellitus results from primary changes in proximal tubular sodium handling without changes in volume expansion. Eur J Clin Invest. (2005) 35:330–6. doi: 10.1111/j.1365-2362.2005.01497.x
40. Mogensen CE, Christensen CK. Predicting diabetic nephropathy in insulin-dependent patients. N Engl J Med. (1984) 311:89–93. doi: 10.1056/NEJM198407123110204
41. Jin Y, Moriya T, Tanaka K, Matsubara M, Fujita Y. Glomerular hyperfiltration in non-proteinuric and non-hypertensive Japanese type 2 diabetic patients. Diabetes Res Clin Pract. (2006) 71:264–71. doi: 10.1016/j.diabres.2005.06.014
42. Rudberg S, Persson B, Dahlquist G. Increased glomerular filtration rate as a predictor of diabetic nephropathy–an 8-year prospective study. Kidney Int. (1992) 41:822–8. doi: 10.1038/ki.1992.126
43. Luk AO, So WY, Ma RC, Kong AP, Ozaki R, Ng VS, et al. Metabolic syndrome predicts new onset of chronic kidney disease in 5,829 patients with type 2 diabetes: a 5-year prospective analysis of the Hong Kong Diabetes Registry. Diabetes Care. (2008) 31:2357–61. doi: 10.2337/dc08-0971
44. Blaslov K, Bulum T, Duvnjak L. Waist-to-height ratio is independently associated with chronic kidney disease in overweight type 2 diabetic patients. Endocr Res. (2015) 40:194–8. doi: 10.3109/07435800.2014.987868
45. Qin S, Wang A, Gu S, Wang W, Gao Z, Tang X, et al. Association between obesity and urinary albumin-creatinine ratio in the middle-aged and elderly population of Southern and Northern China: a cross-sectional study. BMJ open. (2021) 11:e040214. doi: 10.1136/bmjopen-2020-040214
46. Nishikawa K, Takahashi K, Okutani T, Yamada R, Kinaga T, Matsumoto M, et al. Risk of chronic kidney disease in non-obese individuals with clustering of metabolic factors: a longitudinal study. Intern Med. (2015) 54:375–82. doi: 10.2169/internalmedicine.54.3092
47. Wang C, Liang K, Zhang X, Li C, Yang W, Ma Z, et al. Metabolic abnormalities, but not obesity, contribute to the mildly reduced eGFR in middle-aged and elderly Chinese. Int Urol Nephrol. (2014) 46:1793–9. doi: 10.1007/s11255-014-0797-8
48. Amdur RL, Feldman HI, Gupta J, Yang W, Kanetsky P, Shlipak M, et al. Inflammation and progression of CKD: the CRIC study. Clin J Am Soc Nephrol. (2016) 11:1546–56. doi: 10.2215/CJN.13121215
49. Reddy P, Lent-Schochet D, Ramakrishnan N, McLaughlin M, Jialal I. Metabolic syndrome is an inflammatory disorder: a conspiracy between adipose tissue and phagocytes. Clin Chim Acta. (2019) 496:35–44. doi: 10.1016/j.cca.2019.06.019
50. Shlipak MG, Fried LF, Crump C, Bleyer AJ, Manolio TA, Tracy RP, et al. Elevations of inflammatory and procoagulant biomarkers in elderly persons with renal insufficiency. Circulation. (2003) 107:87–92. doi: 10.1161/01.CIR.0000042700.48769.59
51. Stuveling EM, Hillege HL, Bakker SJ, Gans RO, De Jong PE, De Zeeuw D. C-reactive protein is associated with renal function abnormalities in a non-diabetic population. Kidney Int. (2003) 63:654–61. doi: 10.1046/j.1523-1755.2003.00762.x
Keywords: chronic kidney disease, prevalence, middle-aged and elderly, metabolic risk factors, metabolic syndrome
Citation: Lu M-C, Chen I-J, Hsu L-T, Chen Y-J, Tsou M-T, Tung T-H and Chen J-Y (2021) Metabolic Risk Factors Associated With Chronic Kidney Disease in a Middle-Aged and Elderly Taiwanese Population: A Cross-Sectional Study. Front. Med. 8:748037. doi: 10.3389/fmed.2021.748037
Received: 28 July 2021; Accepted: 25 October 2021;
Published: 16 November 2021.
Edited by:
Chia-Ter Chao, National Taiwan University Hospital Bei-Hu Branch, TaiwanReviewed by:
Dongwei Liu, First Affiliated Hospital of Zhengzhou University, ChinaGraham Jones, St Vincent's Hospital Sydney, Australia
Copyright © 2021 Lu, Chen, Hsu, Chen, Tsou, Tung and Chen. This is an open-access article distributed under the terms of the Creative Commons Attribution License (CC BY). The use, distribution or reproduction in other forums is permitted, provided the original author(s) and the copyright owner(s) are credited and that the original publication in this journal is cited, in accordance with accepted academic practice. No use, distribution or reproduction is permitted which does not comply with these terms.
*Correspondence: Jau-Yuan Chen, d2VsaW5zJiN4MDAwNDA7Y2dtaC5vcmcudHc=
†These authors have contributed equally to this work