- 1Neurodegenerative Diseases Research Group, Korea Brain Research Institute, Daegu, South Korea
- 2Department of Convergence Medicine, Pusan National University School of Medicine, Yangsan, South Korea
- 3Department of Life Sciences, Yeungnam University, Gyeongsan, South Korea
Proteomics has become an important field in molecular sciences, as it provides valuable information on the identity, expression levels, and modification of proteins. For example, cancer proteomics unraveled key information in mechanistic studies on tumor growth and metastasis, which has contributed to the identification of clinically applicable biomarkers as well as therapeutic targets. Several cancer proteome databases have been established and are being shared worldwide. Importantly, the integration of proteomics studies with other omics is providing extensive data related to molecular mechanisms and target modulators. These data may be analyzed and processed through bioinformatic pipelines to obtain useful information. The purpose of this review is to provide an overview of cancer proteomics and recent advances in proteomic techniques. In particular, we aim to offer insights into current proteomics studies of brain cancer, in which proteomic applications are in a relatively early stage. This review covers applications of proteomics from the discovery of biomarkers to the characterization of molecular mechanisms through advances in technology. Moreover, it addresses global trends in proteomics approaches for translational research. As a core method in translational research, the continued development of this field is expected to provide valuable information at a scale beyond that previously seen.
Introduction
Proteomics is the study of the entire set of proteins expressed in a cell, tissue, or individual (1, 2). With the advent of Mass spectrometry (MS)-based protein analysis technology, large-scale protein analysis has now become widely used (3–5). Proteomics involves a wide range of processes such as protein expression profiling, protein modifications, protein-protein interactions, protein structure, and protein function (6, 7). The results obtained from such tasks can be used to decipher disease processes, provide diagnosis and prognosis of diseases, aid in drug development, and deliver the basis for biological discovery (8–11). With the development of proteomics technology and its application to various diseases, especially cancer, significant progress has been made in identifying clinically applicable biomarkers and new therapeutic targets (12–14).
The proteomics approach has become popular in cancer studies. Proteomics-based technologies have enabled the identification of potential biomarkers and protein expression patterns that can be used to assess tumor prognosis, prediction, tumor classification, and to identify potential responders for specific therapies. This information can be obtained from different types of samples and be used to develop cancer therapeutics (15–18). In addition, in order to understand the basic biology of cancer, proteomics techniques have been utilized to understand how the signaling pathways in tumor cells are altered, improving our understanding of how to target various pathways for cancer therapy (19–22). As a result, cancer proteome databases have been created, and massive data sets have been collected and integrated with cancer molecular biology data, as well as clinical information.
Over recent years, multi-Omics approaches using patient samples have been used worldwide in translational research. A multi-Omics analysis is a comprehensive and integrated analysis of combined data generated from various omics approaches, including proteomics, genomics, transcriptomics, and metabolomics (23). Multi-Omics analyses can produce large-scale datasets compared to a single analysis, and provides valuable information on the pathophysiology of diseases caused by complex events, thereby making a significant contribution to the diagnosis of diseases and the development of treatments (24–27). Therefore, the continual use of Omics approaches that aggregates multiple Omics data sets will likely have a significant impact on translational research, including in cancer biology, and will likely be the basis for the study of various diseases going forward.
In this paper, we will provide an overview of different cancer proteomics approaches. We will also discuss the use of proteomics technology in a variety of cancers and global trends in the proteomics approaches mentioned above in translational research across the characterization of molecular mechanisms.
General Analytical Strategies of Proteomics
Analytical platforms for proteomics have been developed to identify the entire set of proteins in organisms and to uncover qualitative and quantitative protein variations upon diverse environmental changes. In addition, comprehensive research on proteins has become possible by building an amino acid sequence database on the composition of proteins (1). Generally, a proteomic analysis consists of the following steps: (1) protein extraction, (2) protease digestion, (3) peptide fractionation, and (4) LC-MS analysis (Figure 1). Initially, proteins are extracted and purified from tissue or cell lysates by centrifugation and filtration. Then, the protein mixture is typically separated by two-dimensional gel electrophoresis to reduce sample complexity. Total proteins can be identified by LC-MS analysis of their peptides, which are produced by enzymatic (usually tryptic) digestion, and the data are interpreted using a proteome database (1, 28–31).
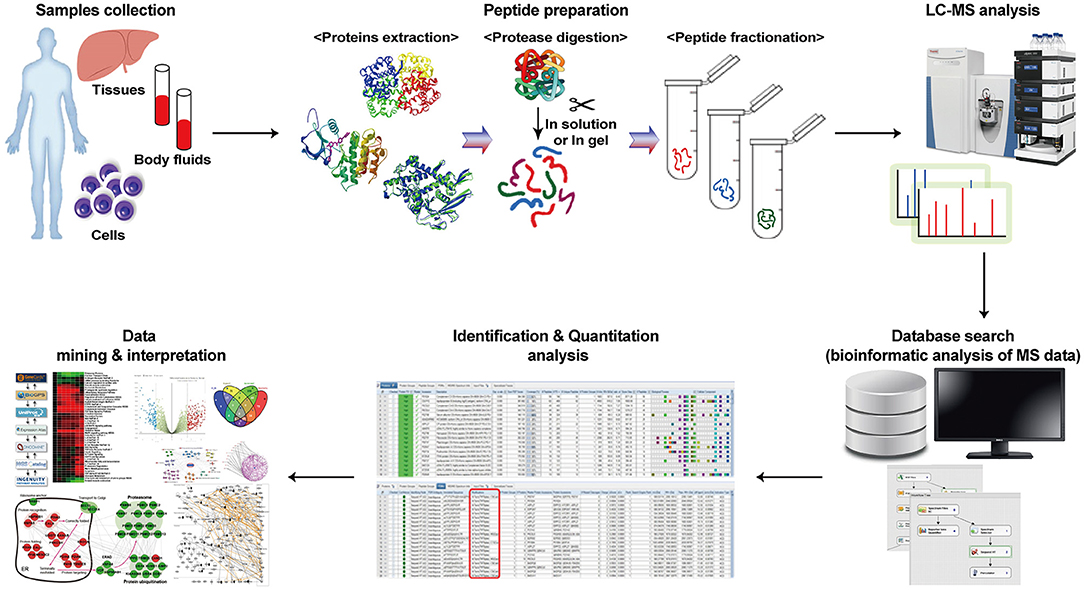
Figure 1. Workflow of the proteomics investigation. Proteomics exhibit many proteins by peptide preparation, analysis using mass spectrometry, and interpretation of peptide data through existing databases.
An attractive part of the proteomics field is its ability to reveal novel biomarkers of diseases. For example, as cancer progresses, changes in protein profiles and differences in protein distribution both in tissues and body fluids such as blood can be examined through quantitative analysis. Proteomics enable the simultaneous qualitative and quantitative profiling of numerous proteins. Liquid chromatography-mass spectrometry (LC/MS) is a key technique that obtains high-resolution spectra of mixed peptides, allowing the discovery of sensitive and specific biomarkers associated with cancer (31). The high-throughput technologies based on this technique enable semi-quantitative and quantitative analyses (32). For the quantitative study of proteins, label-based and label-free approaches are widely used in clinical research (Table 1). Label-based quantitation strategies allow the quantitative and qualitative analysis of proteins in a sample. The methods consist in using stable isotope labeling of compound markers such as amino acids to tag proteins or peptides. The samples containing the tagged proteins are then compared with control proteins tagged with isotope-free markers. These methods have the advantage of minimizing disparities between individually handled samples (33–35). However, proteins may be partially labeled and the reagents are expensive. In proteomics, the common labeling methods are SILAC, ICAT, TMT, and iTRAC. SILAC has the least experimental variability because it is a metabolic labeling method; the isotope reagent is used in the initial step of sample preparation (i.e., cell culture) and the labeled proteins are generated through metabolic processes (33). ICAT is a chemical labeling technique that uses a reagent consisting of a functional group that targets cysteinyl residues, a deuterium atom-based linker region, and a biotin group for protein purification. Sample complexity is significantly reduced through affinity-based extraction of labeled proteins (36). Isobaric labeling methods using TMT and iTRAC need tandem MS techniques. Labeling reagents containing reporter ions are produced under tandem MS, and their amount is proportional to that of tagged peptides, resulting in the quantitation of proteins (34, 35). In contrast to label-based strategies, label-free quantitation approaches using MRM or SWATH are straightforward without labeling steps, which is suitable for large-scale studies. Label-free proteins are quantified based on the signal intensities or spectral counts of peptides unique to them, which are obtained from the MS analysis. The recent development of high-resolution mass spectrometers has led to advances in label-free quantitation for proteomics. Label-free quantitation is easy to use, yields highly reproducible results in biochemical experiments, and is reliable when many statistical verifications are required (37–40). The amount of protein can also be analyzed using antibody arrays such as the ELISA. This is a semi-quantitative and quantitative analysis in which capture antibodies are immobilized on a solid surface such as a nitrocellulose membrane, glass slide, silicone, or beads. Then, the interaction between the antibody and its target protein is detected. However, it is not a discovery-oriented method, and it is limited to the detection of usable and compatible proteins (41).
Qualitative and quantitative protein analyses are very important for understanding biological phenomena and discovering molecular biomarkers of diseases. To diagnose cancer and other diseases using proteomics, minute quantitative and state alterations in the expression of specific proteins need to be detected. Since protein profiles may differ between patients, accurate tools for sample selection, analysis methods and data interpretation need be established to identify relevant protein alterations. Early cancer diagnosis and the discovery of novel potential biomarkers require advances in statistical analyses along with technologies capable of detecting and tracking small protein alterations with high accuracy, reproducibility, and analytical throughput.
Application of Proteomics in Cancers
Cancer involves aberrant cell proliferation, in which the cell cycle of the normal cell becomes dysregulated due to a variety of genetic alterations. Cancer can occur in any tissue of the body and is characterized by its ability to invade or spread to other tissues and organs (42–44). In particular, malignant tumors not only grow rapidly and metastasize to various other tissues but can also develop resistance to the drugs used in treatment, thereby threatening the life of patients (45–47). Proteomics has emerged as an important research tool for exploring the biological changes in cancer. Based on proteomics technology, key information such as protein targets and signaling pathways related to the growth and metastasis of cancer cells have been identified.
Cancer Growth
As fundamental role of cancer therapeutics is inhibition of aberrant cell growth, proteomics-based approaches can play a decisive role in discovering specific biomarkers for growth of cancer. Recently, isobaric labeling TMT proteomics has been used to study hepatitis B virus related hepatocellular carcinoma (HCC) using patient samples with liver tumors and adjacent healthy tissue. Phosphoproteomic approaches elucidated that PYCR2 and ADH1A are related to metabolic reprogramming in HCC, phosphorylation of ALDOA promotes glycolysis and proliferation in CTNNB1-mutated HCC cells (48). This study has provided mechanistic insight on how to develop effective therapies for the clinical treatment of HCC. Genetically engineered mouse models and primary pancreatic epithelial cells have been developed to perform transcriptomics, proteomics, and metabolic analysis in pancreatic cancer. The study shows that LKB1 in primary pancreatic epithelial cells regulates pathways associated with glycolysis, serine metabolism, and DNA methylation using TMT labeling proteomics and proteomic dataset, suggesting that these may regulate the growth of pancreatic cancer cells. Furthermore, it found mechanism that loss of LKB1 and the activation of KRAS seen in oncogenicity is facilitated with the mTOR-dependent pathway (49). These findings comprehend biological mechanism as regulating the growth of cancer and highlight novel proteomics approaches to analyzing genes, proteins, and metabolites for discovering new cancer therapeutics.
An understanding of the tumor and stromal compartments is vital for understanding the growth that occurs in cancer. In tumor tissues, cancer cells interact with the microenvironment, including cancer-associated fibroblast (CAF). Through the proteomics approach, a major metabolic modulator of CAF has been identified. Using a label-free proteomic workflow, the differences in protein expression between the tumor and the stromal compartment were elucidated and confirmed that NNMT expression was increased in omental metastases of patients. The expression of NNMT in the stroma regulates histone methylation and subsequent transcriptional changes. These are critical for the CAF phenotype, enhancing migration and proliferation in ovarian cancer. In support of these findings, it was also shown that inhibiting NNMT expression in CAFs suppressed tumor growth in in vivo experiments, demonstrating the advantages that proteomics can be used to determine the disease phenotypes (50). These studies, by identifying proteins and pathways related to cancer growth and its environment, provide not only the potential to develop effective therapies but also bioinformatic resources to aid basic research.
Metastasis
The diversity of different cancers and the metastasis that occurs during cancer progression are severe obstacles for the successful development of therapeutics (51–53). In particular, metastasis is the most common characteristic of malignant cancers; however, the precise mechanism by which the metastatic cascade occurs is not clearly defined. Recently, several proteomics studies have been performed in an attempt to uncover the cause of the increased metastasis seen in cancer. In one such study, using several Omics like transcriptomics, proteomics, and phospho-proteomics to examine a patient-derived xenograft mouse model, TMT labeling analysis revealed that an increase in stress hormone levels during breast cancer progression was found to cause an increase in the activity of the glucocorticoid receptor (GR) at metastatic regions ultimately reducing the survival rate. Furthermore, it was found that the increased GR activity was implicated in the activation of multiple processes in metastasis and in the elevated expression levels of the kinase ROR1, both of which correlated with reduced survival. In support of these findings, the depletion of ROR1 reduced metastatic growth and extended the survival rate in preclinical models (54).
Lignitto et al. detected increased expression of Bach1, a pro-metastatic transcription factor, via a multi-Omics analysis of the transcriptome and proteome. In lung adenocarcinoma, the loss of keap1 and subsequent Nrf2 activation-induced metastasis through the accumulation of Bach1, and this process was related to a reduction in the survival rate of patients with lung cancer in a heme oxygenase-1-dependent manner. Nrf2 was shown to suppress the Fbxo22-mediated degradation of Bach1 in a heme oxygenase-1 dependent manner, suggesting that inhibition of heme oxygenase-1 could be an effective therapeutic strategy for preventing lung cancer metastasis (55). Such an integrated approach with TMT proteomics analysis defines the role of target molecules in cancer metastasis, providing valid information for diagnosis, prognosis, and therapies.
Drug Resistance
Cancer can recur despite treatments such as surgery and chemotherapy, suggesting that recurrent cancer contains cells that are resistant to anti-cancer drugs (56, 57). The proteomics approach can be employed to identify the characteristics of drug-resistant cancer cells and discover targets that can overcome drug resistance that develops during anti-cancer treatment. Several reports have shown that cells that survive treatment with anti-cancer agents in cancers such as breast, pancreatic, and lung cancers exhibit specific protein expression and molecular mechanisms, correlated with the poor survival rate of patients (58–60). These studies may provide the possibility to maximize the effect of chemotherapy using additional drugs that control key proteins involved in drug resistance.
The characteristics of drug-resistant cancer cells are related to stemness in development, progression, recurrence, and metastasis (61, 62). Cancer stem cells (CSCs) isolated from breast cancer cell lines exhibit drug resistance, and proteomic analyses of these cells suggest new specific markers and therapeutic targets for CSCs (63, 64). Raffel et al. suggested that the importance of targeting leukemic stem cells as the reason for the poor clinical outcomes after treatment for acute myeloid leukemia is due to chemotherapy-resistant cells. Hematopoietic stem/progenitor cells and leukemic stem cell population analysis revealed that IL3RA and CD99 could be markers of leukemic stem cells (65). In addition, pancreatic cancer with a poor prognosis and CSCs are characterized by changes in carbon metabolism and lipid metabolism. Proteomic analysis revealed that the proteins with the highest increase in CSCs were associated with carbon metabolism, and the inhibition of fatty acid synthesis reduced CSC viability, implying a key metabolic pathway regulating CSCs (66). The activity of drug-resistant cancer cells or CSCs interferes with the treatment process, and cancers in which these cells exist are classified as intractable cancers that cannot be treated with conventional anti-cancer drugs. Therefore, for an optimal cancer therapy, it is necessary to identify specific proteins in these cells and identify new diagnostic and therapeutic targets. Table 2 shows a summary of the biomarkers that have been identified in various cancers using proteomics.
Therefore, molecular-level studies for cancer treatment applying proteomics are being conducted, and various methods have been proposed. Among them, immunotherapy has become the preferred alternative treatment to a great extent. Unlike chemotherapy and anti-cancer drugs that directly target cancer cells, immunotherapy activates immune cells to induce attacks on cancer cells to eliminate them and controls the tumor microenvironment to enhance the anti-cancer therapeutic effect (67–69). To monitor cancer therapy and prognosis using immunotherapy, it is necessary to utilize appropriate biomarkers. Protein profiling of cancer patients receiving immunotherapy indicates a response to immunotherapy and survival, suggesting the potential of proteomics approaches to discover prognostic biomarkers (70, 71). Moreover, it can be applied to the treatment of cancer patients by providing molecular information on factors causing resistance to immunotherapy (72). These studies suggest that proteomics analysis can be commonly used for cancer patient biomarkers and can help enhance the immunotherapy response. Table 3 organizes the biomarkers and features found using the proteomics approach to immunotherapy.
Proteomics Approach in Brain Cancer
Glioblastoma multiforme (GBM) is a very aggressive primary brain tumor that presents as heterogeneous malignant types with poor prognosis, high tumor invasiveness, and rapid relapse or progression, resulting in disability during therapy (76–78). Thus, it is urgently needed to identify biomarkers to accurately measure drug response in patients with GBM. In this regard, proteomics analyses have successfully identified alterations in protein expression patterns in GBM.
For example, clinical tissue specimens from patients with high-grade gliomas (glioblastoma) and lower grade gliomas (astrocytoma) have been analyzed with high-resolution iTRAQ labeling quantitative proteomics approach to examine changes in the expression of nuclear proteins. An integrative analysis of both proteomics and transcriptomics data showed that YBX1 is expressed in tumor tissue, and it acts as a regulator of tumor invasion (79). The role of CDH18 in glioma carcinogenesis and its progression examined using proteomic analysis based on their group cohort database. The iTRAQ-based quantitative analysis showed that CDH18 was downregulated in tumor tissue from patients with glioma, and its downstream target, UQCRC2, was down-regulated in tumor tissue from patients with glioma compared to healthy tissue (80). These studies, therefore, defined a target protein for the development of new therapeutic strategies for the treatment of gliomas through proteomic analysis.
Various types of cancers contain a subpopulation known as cancer stem cells that have characteristics similar to normal stem cells, such as self-renewal and the ability to differentiate (81–83). GBM tumors are reportedly composed of heterogeneous types of cells, including a population of stem cell-like cells. The presence of these cells may represent an important therapeutic target because they can cause tumor growth and relapse during therapy (84, 85). To compare the secreted proteins from GBM-derived neural stem (GNS) cells, known cancer stem cells, and normal NSCs, Okawa et al. used a SILAC quantitative proteomics approach that use stable isotope labeling. This study showed that CD9 is enriched in GNS cells (86), indicating that protein and pathways that distinguish GNS cells from NSCs may have value as new biomarkers or candidate therapeutic targets in GBM.
Glioblastoma/astrocytoma can be diagnosed with biomarkers from liquid biopsies, such as plasma, cerebrospinal fluid, and urine, using proteomics. Biomarker candidates for glioblastoma have been found in plasma obtained from glioblastoma patients using SWATH-LC-MS/MS quantitative analysis. Because of this proteomics analysis, eight biomarker candidates for GBM were identified, and among these, LRG1, CRP, and C9 concentrations in plasma were positively correlated with GBM tumor size (87). In addition, Ni et al. have reported the discovery of biomarkers in a model of GBM in which C6 cells are injected into the brain of Wistar rats. They used MRM label-free proteomics to analyze the proteins present in urine over time post-cell injection and successfully identified 109 proteins that changed over time prior to any tumors being visible by MRI (88). Several proteomics studies have discovered biomarkers through the proteomics analysis of cerebrospinal fluid derived from patients with various types of brain tumors such as medulloblastoma (89), pediatric brain tumors (90), CNS lymphoma (91–93), and glioblastoma (94). Therefore, the biomarkers for brain tumors have been identified through proteomics, and the organization of that information led to the recent development of a monitoring system for multiple glioblastomas.
Recently, a glioblastoma phase II clinical trial using personalized immunotherapy failed to prolong patient survival. Despite this, Erhart et al. used a novel approach combining MS-based TMT quantitative proteomics and miRNA sequencing plus RT-qPCR to search for molecules that were related to treatment failure (73). In this way, target proteins and molecules causing therapeutic failure were discovered and analyzed to validate the potential of the novel approach. This strategy could be useful in identifying the factors associated with the failure of other cancer therapies and may pose the basis for the development of new therapies, including cancer immunotherapy.
In a rat xenograft model of human glioblastoma, a survival benefit was observed compared to the untreated control when animals were treated with bacterial carriers that can migrate to the tumor zone where they can induce apoptosis via hypoxia-induced expression of the p53 tumor-suppressor in the presence of the pro-apoptotic drug. The proteomics analysis in non-responders using LC-MS/MS revealed the presence of competing mechanisms being pro-apoptotic in the synapse in parallel with drug resistance (95). The proteomics approaches allow predicting a patient's prognosis by observing alterations in proteins that act as survival factors after cancer therapies.
Extracellular Vesicles as Brain Cancer Biomarkers
Cancer-derived extracellular vesicle (EV) containing DNA, RNA, protein, and lipids expand tumor aggressiveness delivering oncogenes into the circulatory system; thus, affecting the tumor microenvironment and body tissues in patients with cancer. However, cancer-derived EVs have the potential to be used to evaluate glioblastoma tumors as biomarkers (96–98). EVs from glioma expressing constitutively active epidermal EGFRvIII affecting the progression of GBM, including cell infiltration, angiogenesis and regulation of the tumor microenvironment, have been profiled. Using MS, label-free quantitative proteomic analysis in glioma cells, Choi et al. characterized the EVs, including their protein composition, and alterations in regulatory genes. EGFRvIII expressing cells were found to be richer in released extracellular exosomes compared to EGFRvIII-negative cells. The EVs from EGFRvIII expressing glioma cells reportedly contained pro-invasive proteins, such as CD44, CD147, and CD151 (99). This proteomics approach suggests that oncogenic changes in cancer cells can regulate the proteome and provide valuable information for the use of cancer biomarkers based on EVs.
EVs were isolated from the plasma of patients with glioma, and their proteome was analyzed with TMT labeling LC-MS/MS method to show that the EVs from patients with high-grade gliomas contained high levels of SDC1 (100). These data support the notion that high- and low-grade gliomas can be distinguished by examining the proteome of EVs isolated from the plasma of patients and, therefore, represent a useful marker for non-invasive diagnosis of glioma. In another study, a Cavitron ultrasonic surgical aspirator was used to isolate EVs from tumor tissues and fluid in patients with grade IV and grade II-III glioblastomas. Proteomics analysis of the EVs using label-free quantitative LC-MS/MS identified differentially enriched proteins. Among these, CCT2, CCT3, CCT5, CCT6A, CCT7, and TCP1 were increased in the EVs of glioblastoma patients; CCT6A was proposed as a biomarker, as it was associated with decreased survival (101). Therefore, cancer-derived EVs not only play a role in cancer pathology but could also be exploited as biomarker candidates to detect cancer.
Intriguingly, GBM proteomics provided multiple molecular targets. It seems to reflect the difference among sample type, sample state, pretreatment methods, and analytical methods. Table 4 summarizes the molecular targets of brain cancer related to diagnosis, prognosis, and therapy. Although the GBM biomarkers identified are looked diverse, they have been reported to be included in cancer-related pathways such as translation and receptor tyrosine kinase pathways (102, 103). Based on this, it is expected the biomarkers have the potential to function in a common pathway involved in the GBM development. Further Omics research will help reveal the exact protein components included in GBM developmental pathway. To discover reproducible and reliable molecular targets, multi-Omics analysis accompanying data integration must be conducted.
Construction of Cancer Databases
Databases have been created using data obtained from proteomics analyses of various types of cancer, and as a result, novel biomarkers and therapeutic targets have been proposed. The proteomics database is updated by collecting data on the proteome, including protein variations (CanProVar 2.0), extracellular matrix composition (MatrisomeDB), and differentially expressed proteins (dbDEPc 3.0) in cancer based on MS, providing resources for the study of various types of human cancer (104–106). Currently, numerous cancer research-oriented institutions around the world contribute to the integration of Omics data resulting in the construction of databases that can provide information to cancer researchers in a facile manner. The HUPO (Human Proteome Organization) focuses on the analysis of human tumors to identify specific signatures associated with multiple types of cancer and extend the genomic data format in the “Proteomics Standards Initiative” to support proteomics information. Similarly, The CPTAC (Clinical Proteomic Tumor Analysis Consortium) has conducted in-depth proteomics studies to publish key findings in several tumors (107–109), which are available through the CPTAC data portal. In addition, the proteomics data in CPTAC has been integrated with cBioportal, a useful information site for cancer genomics research, to facilitate the easy exploration and integrated analysis of proteomics data sets with clinical and genomic data (110). The LinkedOmics web application has three analytical modules that provide a platform for accessing, analyzing, and comparing cancer multi-omics data within and across cancer types. The database contains multi-omics and clinical data from The Cancer Genome Atlas (TCGA) program, and integrates MS-based global proteomics data generated by the CPTAC on selected TCGA tumor samples (26). CMPD is another database that integrates genomics and proteomics data sets. This database is used to address the complex biological properties of cancer, as it facilitates the identification of cancer-related mutated proteins that are encoded by mutated genes (111). Thus, to obtain more accurate information about cancer, some of the databases incorporate proteomics data into genomic-level studies, providing unified descriptions of cancer mutations at the DNA, RNA, and protein levels through subdivided databases. Various MS-based data sets can be used by researchers worldwide using a storage system as a database (112). In addition, a recently developed tool called ProteomicsDB can analyze various data sets of multiple cells, tissues, and organs by integrating proteomics data with other omics data from programs such as the Human Protein Atlas, which has the aim of mapping all the human proteins in cells (113). The proteomics database information that is available shows that it can be used for basic research, drug discovery, or decision making in the clinic. Studies have suggested that proteomics profiling can be used to investigate the biology of cancer, as well as to screen for and discover molecular biomarkers for the diagnosis, prognosis, and treatment of cancer. Table 5 presents the current proteomics databases.
Recent Trends in Proteomics
The human genome is very complex and is regulated at multiple levels so that genomic information can only be obtained with a variety of Omics methods but not with a single approach. Omics research, including genomics, transcriptomics, proteomics, metabolomics, and epigenomics, has advanced considerably through numerous technological advances and is now capable of providing information at various molecular levels, all of which have contributed significantly to our understanding of biological phenomena (25, 114, 115). In addition, it can provide key information in various diseases, which can then be effectively used in biological, medical, pharmaceutical, and industrial applications. However, since the information provided by each single Omics approach, such as at the gene (DNA or RNA), RNA, protein, and metabolite levels, there are limitations in obtaining comprehensive information on the genome. Each analysis will have variations that depend on the methodology, the equipment, and how the data is integrated, meaning that it is difficult to obtain reproducible, standardized results. The results of independent Omics studies are not sufficient to identify significant correlations in each of the high-level Omics analyses (23, 116–118). Clinically, when using an Omics analysis, there are no standard guidelines for defining a patient's clinical samples due to which they are not well-defined and classified, making it difficult to interpret the analytical results (119, 120). Thus, it has been proposed that a standardized clinical database that integrates the Omics analysis results from each patient needs to be created. To diagnose and treat each disease, researchers have made efforts to obtain new molecular information by reproducing analytical data, integrating databases, and standardizing each analysis step to allow for the production of identical analytical values anywhere in the world. In particular, the cancer research field has actively used Omics technologies, analyzing numerous different types of tumor samples, and using the data to develop new cancer treatments. Accordingly, a lot of information has been released through several consortiums.
The International Cancer Genome Consortium (ICGC) is an organization that provides a forum for the collaboration of cancer genome researchers in the fields of genomics and informatics, and that has systematically analyzed over 25,000 cancer genomes at the multi-omics level for 50 different cancer types (121, 122). These large-scale studies have collected whole genome and exome somatic mutation data from patients with, for example, breast, colorectal, pancreatic cancer and GBM as well as a repertoire of oncogenic mutations that enables the definition of clinically relevant types for prognosis and post-treatment management. They connect current genomic data with newly generated transcriptomic data by linking them with clinical and health information. These big data sets can establish specific criteria and methods to improve patient health, such as cancer prevention strategies, early disease detection, biomarker discovery, diagnosis, and prognosis. In addition, it provides multiple-omics data sources to enable the discovery of novel therapies for cancer patients in clinical trials (121).
The CPTAC aims to understand the molecular mechanisms behind the diversity of cancers using large-scale proteomics and proteogenomics (12). The member institutions have molecularly investigated blood, tumor tissues, and surrounding normal tissues of cancer patients at the gene and protein levels to find proteins that may promote cancer growth and become targets for treatment (123). All clinical information related to cancer patients is provided in the database, and the data are accessible (124). In addition, they have optimized proteomics-based technologies such as sample preparation, peptide extraction, chemical labeling, and MS, and provided experimental guidelines for MS, created new proteomics analyses to identify biomarkers in various cancer types, and provided access to radiology and histopathology data (images, etc.) (110, 125). Recently developed techniques enable the quantification of human proteins from a significantly small amount of sample. For example, Myers et al. developed a highly sensitive proteomics protocol using n-column TMT labeling and multiplexing, providing evidence for the post-transcriptional regulation of gene expression (126). The BASIL, a method for identifying phosphorylation and post-translational modification (PTMs) in relatively few cells lacking sensitivity in phosphoproteomic workflows, has been developed and verified using human pancreatic islet cells (127). These technological developments enable simple and effective quantitative multiplexed proteomics analysis of relatively small amounts of biological or clinical samples. Due to the lack of PTM signature databases, the analysis of signaling pathways generally regulated by post-translational modifications, such as phosphorylation analysis, has been performed using PTM data sets generated by MS at the gene-centric level. Accordingly, a freely available database of PTM signatures has been developed and compared to gene-centric methods in assessing signaling events in cancer cells treated with different agents, targeting signal transduction and cell cycle pathways (128).
The use of artificial intelligence (AI) or machine learning (ML) in combining a large amount of caner proteome and overcome data complexity from different data sources has been highlighted recently. AI can be implemented to create algorithms that increase their performance when certain types of resources or data are provided (129). Several studies have used AI tools to identify novel cancer biomarkers or predict cancer stages (130). Shen et al. used the Boruta algorithm to identify mutant genes involved in vascular invasion from TCGA, National Institute of Health, Medical Research, and AMC databases. A total of 10 genes were identified as vascular invasion-related mutations. Although it is yet to be confirmed whether this mutation can be used for clinical prediction, this study supports that ML can discriminate the gene mutation profile in hepatocellular carcinoma. Another example is connecting microscopy images and proteomics through ML (131). This study adopted a convolutional neural network algorithm to analyze histology from the Cancer Imaging Archive and proteomics datasets from CPTAC. Consequently, the histology-based prediction model accurately distinguishes renal cell carcinomas from normal samples; these predictions are strongly associated with a subset of protein markers. Future studies are required to determine whether this algorithm-based prediction is useful for other types of cancer. In addition, the application of AI to omics can further improve target profiling and integration in cancer and disease diagnosis and biomarker discovery.
To better understand the biomedical characteristics through cell analysis, research is being conducted at the single-cell level. Single-cell analysis has the advantage of identifying the unique characteristics of each cell. Various genome and transcriptome studies have conducted single-cell analysis at the DNA and RNA levels, and the need for proteomic studies at the single-cell level has also been discussed (132, 133). Proteins are fundamental units representing the phenotype and function of cells. Because the protein expression profile of each cell is different, the proteome of a single cell has the potential to not only identify detailed and specific protein expression patterns of a cell, but also understand the biological characteristics of each cell (134). Proteome analysis of bulk samples is used to obtain the proteome information of entire cells, which has limitations in obtaining cell-specific information due to factors such as cell heterogeneity (133, 134). Single-cell proteomics can obtain cell-specific information and identify changes that are specific to a certain cell type. Thus, protein changes can be classified in a more accurate and detailed cell-specific manner without being affected by the cell heterogeneity present in the analysis (135–137). However, for the analysis of single-cell proteomics, a reliable cell separation technique is required, and even when single cell isolation is achieved, the amount of expressed protein is small, hindering the analysis. Various methods have been proposed to overcome these limitations, including methods based on antibodies to quantify proteins at the single-cell level (138, 139). Recently, one of these methods succeeded in identifying more than 1,000 proteins from mouse embryonic stem cells at the single-cell level in an analysis based on mass spectrometry (140). Single-cell proteomics is at its infancy compared to single-cell genomics and transcriptomics, but its importance and necessity are increasing. In the future, with the continuous advancement of methods and technologies, our understanding of the biomedical characteristics of single cells will be extended to the protein level, allowing more direct and accurate information acquisition, including genetic information on prognosis, survival, and diagnosis, and novel biological discoveries in cancer.
Multi-Omics Approach
Cancer genomics and transcriptomics improve our understanding of cancer development and progression, and may lead to diagnosis, prediction, identification, and verification of cancer biomarkers for treatment (141, 142). Transcriptomics directly analyzes the RNA produced through transcriptional processes from genes contained in biological samples, including protein coding genes, mRNAs, small RNAs, and microRNAs (143). The analysis of the transcriptome in cancer samples is an important means of understanding how the expression of different genetic variants affects cancer. After the onset of cancer, the signaling pathways that play a key role in its biological activity, including progression and metastasis, are generally regulated by PTMs in cancer cells (144), in which components of the proteome and their networks are directly involved, performing key molecular functions in cells or tissues. The type of protein modification determines the function and activity of a protein. Protein modification occurs in various ways depending on the cancer cell cycle, pathological conditions, and microenvironment (145). Transcriptome data do not provide direct information on the proteome activity in vivo, thus limiting prognosis prediction and observations of drug responsiveness. On the other hand, proteomics-based protein modification measurements allow the analysis of signaling pathways in cancer cells, prognosis, and drug responsiveness. This means that not only transcriptome markers but also proteome markers are crucial for the establishment and validation of cancer targets and biomarkers. However, the genetic makeup differs between people, which hinders the identification of proteins with individual-specific sequence alterations caused by mutant genes and expressed in cancer. To address this, it would be useful to discover more reliable biomarkers and develop personalized precision medicine for cancer patients by analyzing the transcriptome sequencing data of different patients. Large-scale multi-Omics will allow us to identify the transcripts and proteins that are substantially expressed in vivo, explaining different molecular processes in more detail.
Recently, MS-based proteogenomic data obtained from various types of cancer using different methods have been presented, which can be used clinically to reach a deeper understanding of diseases, explain the relationship between a tumor's genome and the proteome, or to resolve tumor heterogeneity associated with clinical outcomes. In addition, several therapeutic alternatives have been proposed through multi-Omics analyses. For example, tumor tissues from diffuse gastric cancer (GC) patients from a young population have been analyzed by both a genomic analysis and a comprehensive proteomics analysis. The results identify the signaling pathways associated with somatic mutations in early-onset GC. This proteogenomic study has improved our understanding of cancer biology and patient stratification in GCs (146). Similarly, in a colon cancer cohort, a proteogenomic analysis has provided new therapeutic opportunities that target signaling proteins, metabolic enzymes, and tumor antigens. Comparisons of tumors and healthy tissues using proteomic and phosphoproteomic analyses have systematically identified colon cancer-associated proteins and phosphosites, suggesting that phosphorylation of retinoblastoma protein, an oncogenic driver, is a new therapeutic target. In addition, the association between reduced levels of CD8 T cells and increased glycolysis in tumors was investigated in this study, indicating that glycosylation is a potential target for overcoming tumor resistance to immune checkpoint blockade (123). Therefore, proteogenomic datasets could be a novel means for the discovery of new biological information and the development of therapeutics. MS-based shotgun proteomics has been used to analyze tumor tissues in patients with cancer and found that the genomic subtype converges with the proteomic subtype in prostate cancer (147). The integration of multi-Omics data such as genomics, epigenomics, or transcriptomics, in combination with proteomics, is more reliable and insightful for the identification of prognostic biomarkers than single Omics data. Table 6 summarizes the cancer biomarkers identified by the multi-Omics approaches and their characteristics.
In cooperation with the Baylor College of Medicine and the University of Washington Medical School, a proteogenomics database has been developed to explore the potential use of proteogenomics in cancer therapy (148). It has been proposed that these two types of data sets will be used to develop effective therapies and a complete understanding of tumor biology. The analysis of the gene sets obtained from multi-Omics, or other types of integrated studies have become condensed and integrated in order to facilitate the interpretation of multiple enrichment analysis, suggesting a way to obtain data, which is more accurate from cancer proteogenomic data (149). In addition, PepQuery, an integrated proteogenomic method, has been developed that is a quick and easy method for the proteomic validation of new genomic alterations. PepQuery is web-based, allowing for access to MS/MS spectra directly from cancer proteomic studies, and provides standalone program support for MS data (150). In the future, it is expected that, beyond proteomics, the application of proteogenomics in personalized medicine will lead to the development of patient-specific medicine.
The value of multi-Omics technology and datasets lies in the possibility of accurately extracting information to help understand patient-specific molecular complexities. The integration of multi-Omics datasets from cancer enables the large-scale omics analyses of cancer to identify the functional effects of genetic alterations, and to provide evidence for reasonable therapy options derived from tumor pathology. Tumor samples from treatment-naive patients with clear cell renal cell carcinoma were assessed using multi-Omics approaches, including genomic, transcriptomic, proteomic, and phosphoproteomic analyses, thereby confirming each molecular subgroup related to instability. The integration of proteogenomic measurements has been able to uniquely identify dysregulated proteins related to the numerous mechanisms of cells by genetic alterations (74). In addition, multi-Omics analysis was enable to classify protein expression patterns that changed in subtypes of endometrial cancer and suggested a method for maximizing the immunotherapeutic effect by targeting immune cells in these patients (75). Collaboration between PrecisionFDA and NCI-CPTAC identified mislabeled data and applied a process for accurate sample identification to multi-Omics studies so that correct data can be attributed to patients (151).
As such, cancer research using omics is further strengthened, and multi-omics data for translational research is being collected globally. With the discovery of new molecular mechanisms and molecular targets, in-depth multi-Omics analysis of cancer specimens has established networks, capabilities, and expertise at the genome, transcriptome, and proteome levels to improve our understanding of the molecular basis of cancer (152–155). These multi-omics analyses of the disease are taking place in the form of collaborative research worldwide.
Conclusion and Perspectives
Proteomics provides valuable information in several areas, including protein profiles, protein levels, sites of modification, and protein interactions in pathophysiological conditions. Because of this, cancer proteomics identified clinically applicable, novel biomarkers and therapeutic targets. The proteomics approach in cancer research has investigated molecular mechanisms and provided key information on cancer growth, metastasis, and therapy. Importantly, recent cancer proteome databases are established globally and can be freely accessed and used through the integration with bioinformatics. In this paper, we have reviewed the current state of proteomics in multiple cancers. In the case of cancer, the databases are well-organized; however, various diseases the organization of the database information is suboptimal compared to the cancer research database. To address this shortfall, systematic proteomics approaches should be carried out in a variety of diseases, and appropriate databases should be established to provide disease-related information.
Most Omics technologies aim to enhance cancer therapies, but multi-Omics has opened a new path for cancer-related translational research. As the principal information source for translation research, multi-Omics data are being collected in numerous ways. In the future, it will be possible to reverse the translational research to find molecular targets immediately from patients and to apply them to patients in the clinic using the information collected through the research (Figure 2).
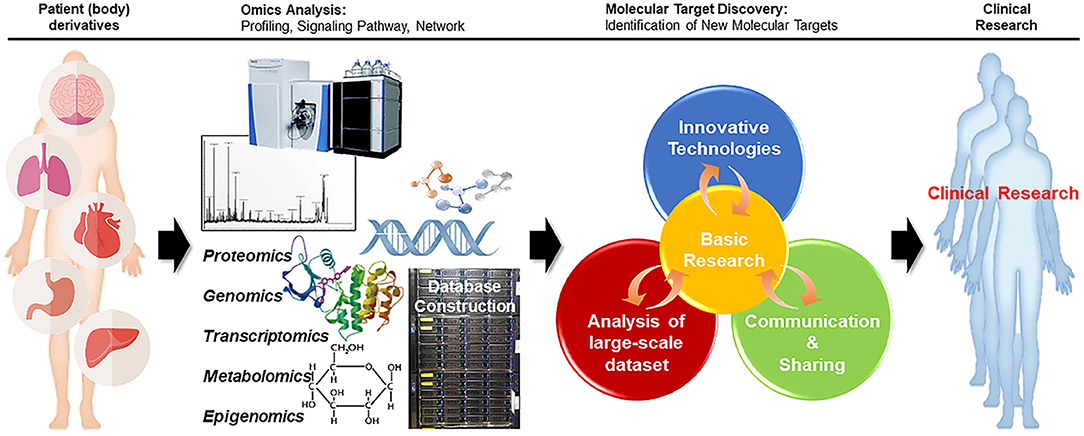
Figure 2. Reverse translational research strategy. In reverse translational research, in-depth multi-omics analysis of cancer specimens from patients can improve our understanding of the molecular basis of cancer, facilitating the discovery of new target molecules. Further clinical research with patients can aid in finding better approaches for the treatment of diseases.
The application of multi-Omics to translation research will take into account not only data collection, integration, and accumulation, but also other aspect including expertise, ethical understanding, communication, administration, and the ability to analyze, interpret, edit and share Omics data across areas. In order to achieve this deeper understanding of the interaction between each Omics data set will be needed.
Author Contributions
YK and JY conceived the idea and designed the study. H-SJ and SB searched the literature and examined the paper. PS contributed to the idea generation. YK, YS, MS, and JY wrote and revised the manuscript. All authors have read and approved the manuscript.
Funding
This research was supported by KBRI basic research program through the Korea Brain Research Institute funded by the Ministry of Science and ICT (21-BR-02-08) and by the 2020 Yeungnam University Research Grant (MS). This research was also supported by the National Research Foundation of Korea (NRF) grant funded by the Korea government (2020R1C1C1005500) and by the Dongil Culture and Scholarship Foundation.
Conflict of Interest
The authors declare that the research was conducted in the absence of any commercial or financial relationships that could be construed as a potential conflict of interest.
Publisher's Note
All claims expressed in this article are solely those of the authors and do not necessarily represent those of their affiliated organizations, or those of the publisher, the editors and the reviewers. Any product that may be evaluated in this article, or claim that may be made by its manufacturer, is not guaranteed or endorsed by the publisher.
References
1. Graves PR, Haystead TA. Molecular biologist's guide to proteomics. Microbiol Mol Biol Rev. (2002) 66:39–63. doi: 10.1128/MMBR.66.1.39-63.2002
2. Altelaar AF, Munoz J, Heck AJ. Next-generation proteomics: towards an integrative view of proteome dynamics. Nat Rev Genet. (2013) 14:35–48. doi: 10.1038/nrg3356
3. Callesen AK, Vach W, Jorgensen PE, Cold S, Tan Q, Depont Christensen R, et al. Combined experimental and statistical strategy for mass spectrometry based serum protein profiling for diagnosis of breast cancer: a case-control study. J Proteome Res. (2008) 7:1419–26. doi: 10.1021/pr7007576
4. Chu CS, Miller CA, Gieschen A, Fischer SM. Pathway-informed discovery and targeted proteomic workflows using mass spectrometry. Methods Mol Biol. (2017) 1550:199–221. doi: 10.1007/978-1-4939-6747-6_15
5. Mezger STP, Mingels AMA, Bekers O, Cillero-Pastor B, Heeren RMA. Trends in mass spectrometry imaging for cardiovascular diseases. Anal Bioanal Chem. (2019) 411:3709–20. doi: 10.1007/s00216-019-01780-8
6. Pandey A, Mann M. Proteomics to study genes and genomes. Nature. (2000) 405:837–46. doi: 10.1038/35015709
7. Hyung SJ, Ruotolo BT. Integrating mass spectrometry of intact protein complexes into structural proteomics. Proteomics. (2012) 12:1547–64. doi: 10.1002/pmic.201100520
8. Zaslavsky BY, Uversky VN, Chait A. Solvent interaction analysis as a proteomic approach to structure-based biomarker discovery and clinical diagnostics. Expert Rev Proteomics. (2016) 13:9–17. doi: 10.1586/14789450.2016.1116945
9. Lv LX, Yan R, Shi HY, Shi D, Fang DQ, Jiang HY, et al. Integrated transcriptomic and proteomic analysis of the bile stress response in probiotic Lactobacillus salivarius LI01. J Proteomics. (2017) 150:216–29. doi: 10.1016/j.jprot.2016.08.021
10. Goeminne LJE, Gevaert K, Clement L. Experimental design and data-analysis in label-free quantitative LC/MS proteomics: a tutorial with MSqRob. J Proteomics. (2018) 171:23–36. doi: 10.1016/j.jprot.2017.04.004
11. Prieto P, Jaen RI, Calle D, Gomez-Serrano M, Nunez E, Fernandez-Velasco M, et al. Interplay between post-translational cyclooxygenase-2 modifications and the metabolic and proteomic profile in a colorectal cancer cohort. World J Gastroenterol. (2019) 25:433–46. doi: 10.3748/wjg.v25.i4.433
12. Ellis MJ, Gillette M, Carr SA, Paulovich AG, Smith RD, Rodland KK, et al. Connecting genomic alterations to cancer biology with proteomics: the NCI Clinical Proteomic Tumor Analysis Consortium. Cancer Discov. (2013) 3:1108–12. doi: 10.1158/2159-8290.CD-13-0219
13. Faria SS, Morris CF, Silva AR, Fonseca MP, Forget P, Castro MS, et al. A timely shift from shotgun to targeted proteomics and how it can be groundbreaking for cancer research. Front Oncol. (2017) 7:13. doi: 10.3389/fonc.2017.00013
14. Lin YH, Eguez RV, Torralba MG, Singh H, Golusinski P, Golusinski W, et al. Self-assembled STrap for global proteomics and salivary biomarker discovery. J Proteome Res. (2019) 18:1907–15. doi: 10.1021/acs.jproteome.9b00037
15. Hanash S, Taguchi A. Application of proteomics to cancer early detection. Cancer J. (2011) 17:423–8. doi: 10.1097/PPO.0b013e3182383cab
16. Yadav M, Jhunjhunwala S, Phung QT, Lupardus P, Tanguay J, Bumbaca S, et al. Predicting immunogenic tumour mutations by combining mass spectrometry and exome sequencing. Nature. (2014) 515:572–6. doi: 10.1038/nature14001
17. Chen F, Chandrashekar DS, Varambally S, Creighton CJ. Pan-cancer molecular subtypes revealed by mass-spectrometry-based proteomic characterization of more than 500 human cancers. Nat Commun. (2019) 10:5679. doi: 10.1038/s41467-019-13528-0
18. Enroth S, Berggrund M, Lycke M, Broberg J, Lundberg M, Assarsson E, et al. High throughput proteomics identifies a high-accuracy 11 plasma protein biomarker signature for ovarian cancer. Commun Biol. (2019) 2:221. doi: 10.1038/s42003-019-0464-9
19. Posadas EM, Simpkins F, Liotta LA, MacDonald C, Kohn EC. Proteomic analysis for the early detection and rational treatment of cancer–realistic hope? Ann Oncol. (2005) 16:16–22. doi: 10.1093/annonc/mdi004
20. Nanjundan M, Byers LA, Carey MS, Siwak DR, Raso MG, Diao L, et al. Proteomic profiling identifies pathways dysregulated in non-small cell lung cancer and an inverse association of AMPK and adhesion pathways with recurrence. J Thorac Oncol. (2010) 5:1894–904. doi: 10.1097/JTO.0b013e3181f2a266
21. Shruthi BS, Vinodhkumar P, Selvamani. Proteomics: a new perspective for cancer. Adv Biomed Res. (2016) 5:67. doi: 10.4103/2277-9175.180636
22. Chang L, Ni J, Beretov J, Wasinger VC, Hao J, Bucci J, et al. Identification of protein biomarkers and signaling pathways associated with prostate cancer radioresistance using label-free LC-MS/MS proteomic approach. Sci Rep. (2017) 7:41834. doi: 10.1038/srep41834
23. Hasin Y, Seldin M, Lusis A. Multi-omics approaches to disease. Genome Biol. (2017) 18:83. doi: 10.1186/s13059-017-1215-1
24. Sun YV, Hu YJ. Integrative analysis of multi-omics data for discovery and functional studies of complex human diseases. Adv Genet. (2016) 93:147–90. doi: 10.1016/bs.adgen.2015.11.004
25. Karczewski KJ, Snyder MP. Integrative omics for health and disease. Nat Rev Genet. (2018) 19:299–310. doi: 10.1038/nrg.2018.4
26. Vasaikar SV, Straub P, Wang J, Zhang B. LinkedOmics: analyzing multi-omics data within and across 32 cancer types. Nucleic Acids Res. (2018) 46:D956–63. doi: 10.1093/nar/gkx1090
27. Xiao Y, Ma D, Zhao S, Suo C, Shi J, Xue MZ, et al. Multi-omics profiling reveals distinct microenvironment characterization and suggests immune escape mechanisms of triple-negative breast cancer. Clin Cancer Res. (2019) 25:5002–14. doi: 10.1158/1078-0432.CCR-18-3524
28. Aebersold R, Mann M. Mass spectrometry-based proteomics. Nature. (2003) 422:198–207. doi: 10.1038/nature01511
29. Garza S, Moini M. Analysis of complex protein mixtures with improved sequence coverage using (CE-MS/MS)n. Anal Chem. (2006) 78:7309–16. doi: 10.1021/ac0612269
30. Issaq H, Veenstra T. Two-dimensional polyacrylamide gel electrophoresis (2D-PAGE): advances and perspectives. Biotechniques. (2008) 44:697–700. doi: 10.2144/000112823
31. Angel TE, Aryal UK, Hengel SM, Baker ES, Kelly RT, Robinson EW, et al. Mass spectrometry-based proteomics: existing capabilities and future directions. Chem Soc Rev. (2012) 41:3912–28. doi: 10.1039/c2cs15331a
32. Kay RG, Galvin S, Larraufie P, Reimann F, Gribble FM. Liquid chromatography/mass spectrometry based detection and semi-quantitative analysis of INSL5 in human and murine tissues. Rapid Commun Mass Spectrom. (2017) 31:1963–73. doi: 10.1002/rcm.7978
33. Ong SE, Kratchmarova I, Mann M. Properties of 13C-substituted arginine in stable isotope labeling by amino acids in cell culture (SILAC). J Proteome Res. (2003) 2:173–81. doi: 10.1021/pr0255708
34. Thompson A, Schafer J, Kuhn K, Kienle S, Schwarz J, Schmidt G, et al. Tandem mass tags: a novel quantification strategy for comparative analysis of complex protein mixtures by MS/MS. Anal Chem. (2003) 75:1895–904. doi: 10.1021/ac0262560
35. DeSouza L, Diehl G, Rodrigues MJ, Guo J, Romaschin AD, Colgan TJ, et al. Search for cancer markers from endometrial tissues using differentially labeled tags iTRAQ and cICAT with multidimensional liquid chromatography and tandem mass spectrometry. J Proteome Res. (2005) 4:377–86. doi: 10.1021/pr049821j
36. Gygi SP, Rist B, Gerber SA, Turecek F, Gelb MH, Aebersold R. Quantitative analysis of complex protein mixtures using isotope-coded affinity tags. Nat Biotechnol. (1999) 17:994–9. doi: 10.1038/13690
37. Old WM, Meyer-Arendt K, Aveline-Wolf L, Pierce KG, Mendoza A, Sevinsky JR, et al. Comparison of label-free methods for quantifying human proteins by shotgun proteomics. Mol Cell Proteomics. (2005) 4:1487–502. doi: 10.1074/mcp.M500084-MCP200
38. Zhu W, Smith JW, Huang CM. Mass spectrometry-based label-free quantitative proteomics. J Biomed Biotechnol. (2010) 2010:840518. doi: 10.1155/2010/840518
39. Milac TI, Randolph TW, Wang P. Analyzing LC-MS/MS data by spectral count and ion abundance: two case studies. Stat Interface. (2012) 5:75–87. doi: 10.4310/SII.2012.v5.n1.a7
40. Cox J, Hein MY, Luber CA, Paron I, Nagaraj N, Mann M. Accurate proteome-wide label-free quantification by delayed normalization and maximal peptide ratio extraction, termed MaxLFQ. Mol Cell Proteomics. (2014) 13:2513–26. doi: 10.1074/mcp.M113.031591
41. Wilson JJ, Burgess R, Mao YQ, Luo S, Tang H, Jones VS, et al. Antibody arrays in biomarker discovery. Adv Clin Chem. (2015) 69:255–324. doi: 10.1016/bs.acc.2015.01.002
42. Armstrong PB, Armstrong MT. Intercellular invasion and the organizational stability of tissues: a role for fibronectin. Biochim Biophys Acta. (2000) 1470:O9–20. doi: 10.1016/S0304-419X(00)00003-2
43. Hahn WC, Weinberg RA. Rules for making human tumor cells. N Engl J Med. (2002) 347:1593–603. doi: 10.1056/NEJMra021902
44. Soto AM, Sonnenschein C. The somatic mutation theory of cancer: growing problems with the paradigm? Bioessays. (2004) 26:1097–107. doi: 10.1002/bies.20087
45. Brabletz T, Jung A, Reu S, Porzner M, Hlubek F, Kunz-Schughart LA, et al. Variable beta-catenin expression in colorectal cancers indicates tumor progression driven by the tumor environment. Proc Natl Acad Sci U S A. (2001) 98:10356–61. doi: 10.1073/pnas.171610498
46. Gupta GP, Massague J. Cancer metastasis: building a framework. Cell. (2006) 127:679–95. doi: 10.1016/j.cell.2006.11.001
47. Lee SI, Kim DK, Seo EJ, Choi EJ, Kwon YW, Jang IH, et al. Role of Kruppel-like factor 4 in the maintenance of chemoresistance of anaplastic thyroid cancer. Thyroid. (2017) 27:1424–32. doi: 10.1089/thy.2016.0414
48. Gao Q, Zhu H, Dong L, Shi W, Chen R, Song Z, et al. Integrated proteogenomic characterization of HBV-related hepatocellular carcinoma. Cell. (2019) 179:1240. doi: 10.1016/j.cell.2019.10.038
49. Kottakis F, Nicolay BN, Roumane A, Karnik R, Gu H, Nagle JM, et al. LKB1 loss links serine metabolism to DNA methylation and tumorigenesis. Nature. (2016) 539:390–5. doi: 10.1038/nature20132
50. Eckert MA, Coscia F, Chryplewicz A, Chang JW, Hernandez KM, Pan S, et al. Proteomics reveals NNMT as a master metabolic regulator of cancer-associated fibroblasts. Nature. (2019) 569:723–8. doi: 10.1038/s41586-019-1173-8
51. Corso S, Migliore C, Ghiso E, De Rosa G, Comoglio PM, Giordano S. Silencing the MET oncogene leads to regression of experimental tumors and metastases. Oncogene. (2008) 27:684–93. doi: 10.1038/sj.onc.1210697
52. Cleary AS, Leonard TL, Gestl SA, Gunther EJ. Tumour cell heterogeneity maintained by cooperating subclones in Wnt-driven mammary cancers. Nature. (2014) 508:113–7. doi: 10.1038/nature13187
53. Koren S, Bentires-Alj M. Breast tumor heterogeneity: source of fitness, hurdle for therapy. Mol Cell. (2015) 60:537–46. doi: 10.1016/j.molcel.2015.10.031
54. Obradovic MMS, Hamelin B, Manevski N, Couto JP, Sethi A, Coissieux MM, et al. Glucocorticoids promote breast cancer metastasis. Nature. (2019) 567:540–4. doi: 10.1038/s41586-019-1019-4
55. Lignitto L, LeBoeuf SE, Homer H, Jiang S, Askenazi M, Karakousi TR, et al. Nrf2 activation promotes lung cancer metastasis by inhibiting the degradation of Bach1. Cell. (2019) 178:316–29 e318. doi: 10.1016/j.cell.2019.06.003
56. Kuczynski EA, Sargent DJ, Grothey A, Kerbel RS. Drug rechallenge and treatment beyond progression–implications for drug resistance. Nat Rev Clin Oncol. (2013) 10:571–87. doi: 10.1038/nrclinonc.2013.158
57. Wang X, Zhang H, Chen X. Drug resistance and combating drug resistance in cancer. Cancer Drug Resist. (2019) 2:141–60. doi: 10.20517/cdr.2019.10
58. Le Large TYS, El Hassouni B, Funel N, Kok B, Piersma SR, Pham TV, et al. Proteomic analysis of gemcitabine-resistant pancreatic cancer cells reveals that microtubule-associated protein 2 upregulation associates with taxane treatment. Ther Adv Med Oncol. (2019) 11:1758835919841233. doi: 10.1177/1758835919841233
59. Shenoy A, Belugali Nataraj N, Perry G, Loayza Puch F, Nagel R, Marin I, et al. Proteomic patterns associated with response to breast cancer neoadjuvant treatment. Mol Syst Biol. (2020) 16:e9443. doi: 10.15252/msb.20209443
60. Zhang X, Maity TK, Ross KE, Qi Y, Cultraro CM, Bahta M, et al. Alterations in the global proteome and phosphoproteome in third generation EGFR TKI resistance reveal drug targets to circumvent resistance. Cancer Res. (2021) 81:3051–66. doi: 10.1158/0008-5472.CAN-20-2435
61. Meacham CE, Morrison SJ. Tumour heterogeneity and cancer cell plasticity. Nature. (2013) 501:328–37. doi: 10.1038/nature12624
62. Phi LTH, Sari IN, Yang YG, Lee SH, Jun N, Kim KS, et al. Cancer stem cells (CSCs) in drug resistance and their therapeutic implications in cancer treatment. Stem Cells Int. (2018) 2018:5416923. doi: 10.1155/2018/5416923
63. Jeon SA, Kim DW, Lee DB, Cho JY. NEDD4 plays roles in the maintenance of breast cancer stem cell characteristics. Front Oncol. (2020) 10:1680. doi: 10.3389/fonc.2020.01680
64. Koh EY, You JE, Jung SH, Kim PH. Biological functions and identification of novel biomarker expressed on the surface of breast cancer-derived cancer stem cells via proteomic analysis. Mol Cells. (2020) 43:384–96. doi: 10.14348/molcells.2020.2230
65. Raffel S, Klimmeck D, Falcone M, Demir A, Pouya A, Zeisberger P, et al. Quantitative proteomics reveals specific metabolic features of acute myeloid leukemia stem cells. Blood. (2020) 136:1507–19. doi: 10.1182/blood.2019003654
66. Brandi J, Dando I, Pozza ED, Biondani G, Jenkins R, Elliott V, et al. Proteomic analysis of pancreatic cancer stem cells: functional role of fatty acid synthesis and mevalonate pathways. J Proteomics. (2017) 150:310–22. doi: 10.1016/j.jprot.2016.10.002
67. Riley RS, June CH, Langer R, Mitchell MJ. Delivery technologies for cancer immunotherapy. Nat Rev Drug Discov. (2019) 18:175–96. doi: 10.1038/s41573-018-0006-z
68. Murciano-Goroff YR, Warner AB, Wolchok JD. The future of cancer immunotherapy: microenvironment-targeting combinations. Cell Res. (2020) 30:507–19. doi: 10.1038/s41422-020-0337-2
69. Zhang Y, Zhang Z. The history and advances in cancer immunotherapy: understanding the characteristics of tumor-infiltrating immune cells and their therapeutic implications. Cell Mol Immunol. (2020) 17:807–21. doi: 10.1038/s41423-020-0488-6
70. Harel M, Ortenberg R, Varanasi SK, Mangalhara KC, Mardamshina M, Markovits E, et al. Proteomics of melanoma response to immunotherapy reveals mitochondrial dependence. Cell. (2019) 179:236–50 e218. doi: 10.1016/j.cell.2019.08.012
71. Chae YK, Kim WB, Davis AA, Park LC, Anker JF, Simon NI, et al. Mass spectrometry-based serum proteomic signature as a potential biomarker for survival in patients with non-small cell lung cancer receiving immunotherapy. Transl Lung Cancer Res. (2020) 9:1015–28. doi: 10.21037/tlcr-20-148
72. Peng DH, Rodriguez BL, Diao L, Chen L, Wang J, Byers LA, et al. Collagen promotes anti-PD-1/PD-L1 resistance in cancer through LAIR1-dependent CD8(+) T cell exhaustion. Nat Commun. (2020) 11:4520. doi: 10.1038/s41467-020-18298-8
73. Erhart F, Hackl M, Hahne H, Buchroithner J, Meng C, Klingenbrunner S, et al. Combined proteomics/miRNomics of dendritic cell immunotherapy-treated glioblastoma patients as a screening for survival-associated factors. NPJ Vaccines. (2020) 5:5. doi: 10.1038/s41541-019-0149-x
74. Clark DJ, Dhanasekaran SM, Petralia F, Pan J, Song X, Hu Y, et al. Integrated proteogenomic characterization of clear cell renal cell carcinoma. Cell. (2019) 179:964–83 e931. doi: 10.1016/j.cell.2019.10.007
75. Dou Y, Kawaler EA, Cui Zhou D, Gritsenko MA, Huang C, Blumenberg L, et al. Proteogenomic characterization of endometrial carcinoma. Cell. (2020) 180:729–48 e726. doi: 10.1016/j.cell.2020.01.026
76. Omuro A, DeAngelis LM. Glioblastoma and other malignant gliomas: a clinical review. J Am Med Assoc. (2013) 310:1842–50. doi: 10.1001/jama.2013.280319
77. Reifenberger G, Wirsching HG, Knobbe-Thomsen CB, Weller M. Advances in the molecular genetics of gliomas - implications for classification and therapy. Nat Rev Clin Oncol. (2017) 14:434–52. doi: 10.1038/nrclinonc.2016.204
78. Zhang BL, Dong FL, Guo TW, Gu XH, Huang LY, Gao DS. MiRNAs mediate GDNF-induced proliferation and migration of glioma cells. Cell Physiol Biochem. (2017) 44:1923–38. doi: 10.1159/000485883
79. Gupta MK, Polisetty RV, Sharma R, Ganesh RA, Gowda H, Purohit AK, et al. Altered transcriptional regulatory proteins in glioblastoma and YBX1 as a potential regulator of tumor invasion. Sci Rep. (2019) 9:10986. doi: 10.1038/s41598-019-47360-9
80. Bai YH, Zhan YB, Yu B, Wang WW, Wang L, Zhou JQ, et al. A novel tumor-suppressor, CDH18, inhibits glioma cell invasiveness via UQCRC2 and correlates with the prognosis of glioma patients. Cell Physiol Biochem. (2018) 48:1755–70. doi: 10.1159/000492317
81. Ginestier C, Hur MH, Charafe-Jauffret E, Monville F, Dutcher J, Brown M, et al. ALDH1 is a marker of normal and malignant human mammary stem cells and a predictor of poor clinical outcome. Cell Stem Cell. (2007) 1:555–67. doi: 10.1016/j.stem.2007.08.014
82. Prince ME, Sivanandan R, Kaczorowski A, Wolf GT, Kaplan MJ, Dalerba P, et al. Identification of a subpopulation of cells with cancer stem cell properties in head and neck squamous cell carcinoma. Proc Natl Acad Sci U S A. (2007) 104:973–8. doi: 10.1073/pnas.0610117104
84. Hemmati HD, Nakano I, Lazareff JA, Masterman-Smith M, Geschwind DH, Bronner-Fraser M, et al. Cancerous stem cells can arise from pediatric brain tumors. Proc Natl Acad Sci USA. (2003) 100:15178–83. doi: 10.1073/pnas.2036535100
85. Pollard SM, Yoshikawa K, Clarke ID, Danovi D, Stricker S, Russell R, et al. Glioma stem cell lines expanded in adherent culture have tumor-specific phenotypes and are suitable for chemical and genetic screens. Cell Stem Cell. (2009) 4:568–80. doi: 10.1016/j.stem.2009.03.014
86. Okawa S, Gagrica S, Blin C, Ender C, Pollard SM, Krijgsveld J. Proteome and secretome characterization of glioblastoma-derived neural stem cells. Stem Cells. (2017) 35:967–80. doi: 10.1002/stem.2542
87. Miyauchi E, Furuta T, Ohtsuki S, Tachikawa M, Uchida Y, Sabit H, et al. Identification of blood biomarkers in glioblastoma by SWATH mass spectrometry and quantitative targeted absolute proteomics. PLoS ONE. (2018) 13:e0193799. doi: 10.1371/journal.pone.0193799
88. Ni Y, Zhang F, An M, Yin W, Gao Y. Early candidate biomarkers found from urine of glioblastoma multiforme rat before changes in MRI. Sci China Life Sci. (2018) 61:982–7. doi: 10.1007/s11427-017-9201-0
89. Rajagopal MU, Hathout Y, MacDonald TJ, Kieran MW, Gururangan S, Blaney SM, et al. Proteomic profiling of cerebrospinal fluid identifies prostaglandin D2 synthase as a putative biomarker for pediatric medulloblastoma: a pediatric brain tumor consortium study. Proteomics. (2011) 11:935–43. doi: 10.1002/pmic.201000198
90. Samuel N, Remke M, Rutka JT, Raught B, Malkin D. Proteomic analyses of CSF aimed at biomarker development for pediatric brain tumors. J Neurooncol. (2014) 118:225–38. doi: 10.1007/s11060-014-1432-3
91. Murase S, Saio M, Andoh H, Takenaka K, Shinoda J, Nishimura Y, et al. Diagnostic utility of CSF soluble CD27 for primary central nervous system lymphoma in immunocompetent patients. Neurol Res. (2000) 22:434–42. doi: 10.1080/01616412.2000.11740697
92. Roy S, Josephson SA, Fridlyand J, Karch J, Kadoch C, Karrim J, et al. Protein biomarker identification in the CSF of patients with CNS lymphoma. J Clin Oncol. (2008) 26:96–105. doi: 10.1200/JCO.2007.12.1053
93. Zetterberg H, Andreasson U, Blennow K. CSF antithrombin III and disruption of the blood-brain barrier. J Clin Oncol. (2009) 27:2302–3. doi: 10.1200/JCO.2008.19.8598
94. Shnaper S, Desbaillets I, Brown DA, Murat A, Migliavacca E, Schluep M, et al. Elevated levels of MIC-1/GDF15 in the cerebrospinal fluid of patients are associated with glioblastoma and worse outcome. Int J Cancer. (2009) 125:2624–30. doi: 10.1002/ijc.24639
95. Mehta N, Lyon JG, Patil K, Mokarram N, Kim C, Bellamkonda RV. Bacterial carriers for glioblastoma therapy. Mol Ther Oncolyt. (2017) 4:1–17. doi: 10.1016/j.omto.2016.12.003
96. Skog J, Wurdinger T, van Rijn S, Meijer DH, Gainche L, Sena-Esteves M, et al. Glioblastoma microvesicles transport RNA and proteins that promote tumour growth and provide diagnostic biomarkers. Nat Cell Biol. (2008) 10:1470–6. doi: 10.1038/ncb1800
97. Kucharzewska P, Christianson HC, Welch JE, Svensson KJ, Fredlund E, Ringner M, et al. Exosomes reflect the hypoxic status of glioma cells and mediate hypoxia-dependent activation of vascular cells during tumor development. Proc Natl Acad Sci USA. (2013) 110:7312–7. doi: 10.1073/pnas.1220998110
98. Kalluri R. The biology and function of exosomes in cancer. J Clin Invest. (2016) 126:1208–15. doi: 10.1172/JCI81135
99. Choi D, Montermini L, Kim DK, Meehan B, Roth FP, Rak J. The impact of oncogenic EGFRvIII on the proteome of extracellular vesicles released from glioblastoma cells. Mol Cell Proteomics. (2018) 17:1948–64. doi: 10.1074/mcp.RA118.000644
100. Indira Chandran V, Welinder C, Mansson AS, Offer S, Freyhult E, Pernemalm M, et al. Ultrasensitive immunoprofiling of plasma extracellular vesicles identifies syndecan-1 as a potential tool for minimally invasive diagnosis of glioma. Clin Cancer Res. (2019) 25:3115–27. doi: 10.1158/1078-0432.CCR-18-2946
101. Hallal S, Russell BP, Wei H, Lee MYT, Toon CW, Sy J, et al. Extracellular vesicles from neurosurgical aspirates identifies chaperonin containing TCP1 subunit 6A as a potential glioblastoma biomarker with prognostic significance. Proteomics. (2019) 19:e1800157. doi: 10.1002/pmic.201800157
102. Wang Y, Arribas-Layton M, Chen Y, Lykke-Andersen J, Sen GL. DDX6 orchestrates mammalian progenitor function through the mRNA degradation and translation pathways. Mol Cell. (2015) 60:118–30. doi: 10.1016/j.molcel.2015.08.014
103. Pearson JRD, Regad T. Targeting cellular pathways in glioblastoma multiforme. Signal Transduct Target Ther. (2017) 2:17040. doi: 10.1038/sigtrans.2017.40
104. Zhang M, Wang B, Xu J, Wang X, Xie L, Zhang B, et al. CanProVar 2.0: an updated database of human cancer proteome variation. J Proteome Res. (2017) 16:421–32. doi: 10.1021/acs.jproteome.6b00505
105. Yang Q, Zhang Y, Cui H, Chen L, Zhao Y, Lin Y, et al. dbDEPC 30: the database of differentially expressed proteins in human cancer with multi-level annotation and drug indication. Database. (2018) 2018:bay015. doi: 10.1093/database/bay015
106. Shao X, Taha IN, Clauser KR, Gao YT, Naba A. MatrisomeDB: the ECM-protein knowledge database. Nucleic Acids Res. (2020) 48:D1136–44. doi: 10.1093/nar/gkz849
107. Zhang B, Wang J, Wang X, Zhu J, Liu Q, Shi Z, et al. Proteogenomic characterization of human colon and rectal cancer. Nature. (2014) 513:382–7. doi: 10.1038/nature13438
108. Mertins P, Mani DR, Ruggles KV, Gillette MA, Clauser KR, Wang P, et al. Proteogenomics connects somatic mutations to signalling in breast cancer. Nature. (2016) 534:55–62. doi: 10.1038/nature18003
109. Zhang H, Liu T, Zhang Z, Payne SH, Zhang B, McDermott JE, et al. Integrated proteogenomic characterization of human high-grade serous ovarian cancer. Cell. (2016) 166:755–65. doi: 10.1016/j.cell.2016.05.069
110. Wu P, Heins ZJ, Muller JT, Katsnelson L, de Bruijn I, Abeshouse AA, et al. Integration and analysis of CPTAC proteomics data in the context of cancer genomics in the cBioPortal. Mol Cell Proteomics. (2019) 18:1893–8. doi: 10.1074/mcp.TIR119.001673
111. Huang PJ, Lee CC, Tan BC, Yeh YM, Julie Chu L, Chen TW, et al. CMPD: cancer mutant proteome database. Nucleic Acids Res 43(Database issue). (2015) D849–855. doi: 10.1093/nar/gku1182
112. Okuda S, Watanabe Y, Moriya Y, Kawano S, Yamamoto T, Matsumoto M, et al. jPOSTrepo: an international standard data repository for proteomes. Nucleic Acids Res. (2017) 45:D1107–11. doi: 10.1093/nar/gkw1080
113. Samaras P, Schmidt T, Frejno M, Gessulat S, Reinecke M, Jarzab A, et al. ProteomicsDB: a multi-omics and multi-organism resource for life science research. Nucleic Acids Res. (2020) 48:D1153–63. doi: 10.1093/nar/gkz974
114. Manzoni C, Kia DA, Vandrovcova J, Hardy J, Wood NW, Lewis PA, et al. Genome, transcriptome and proteome: the rise of omics data and their integration in biomedical sciences. Brief Bioinform. (2018) 19:286–302. doi: 10.1093/bib/bbw114
115. Song P, Kwon Y, Joo JY, Kim DG, Yoon JH. Secretomics to discover regulators in diseases. Int J Mol Sci. (2019) 20:163893. doi: 10.3390/ijms20163893
116. Kim M, Tagkopoulos I. Data integration and predictive modeling methods for multi-omics datasets. Mol Omics. (2018) 14:8–25. doi: 10.1039/C7MO00051K
117. Pinu FR, Beale DJ, Paten AM, Kouremenos K, Swarup S, Schirra HJ, et al. Systems biology and multi-omics integration: viewpoints from the metabolomics research community. Metabolites. (2019) 9:40076. doi: 10.3390/metabo9040076
118. Wu C, Zhou F, Ren J, Li X, Jiang Y, Ma S. A selective review of multi-level omics data integration using variable selection. High Throughput. (2019) 8:10004. doi: 10.3390/ht8010004
119. Beal J, Montagud A, Traynard P, Barillot E, Calzone L. Personalization of logical models with multi-omics data allows clinical stratification of patients. Front Physiol. (2018) 9:1965. doi: 10.3389/fphys.2018.01965
120. Kellogg RA, Dunn J, Snyder MP. Personal omics for precision health. Circ Res. (2018) 122:1169–71. doi: 10.1161/CIRCRESAHA.117.310909
121. International Cancer Genome C, Hudson TJ, Anderson W, Artez A, Barker AD, Bell C, et al. International network of cancer genome projects. Nature. (2010) 464:993–8. doi: 10.1038/nature08987
122. Zhang J, Baran J, Cros A, Guberman JM, Haider S, Hsu J, et al. International Cancer Genome Consortium Data Portal–a one-stop shop for cancer genomics data. Database. (2011) 2011:bar026. doi: 10.1093/database/bar026
123. Vasaikar S, Huang C, Wang X, Petyuk VA, Savage SR, Wen B, et al. Proteogenomic analysis of human colon cancer reveals new therapeutic opportunities. Cell. (2019) 177:1035–49 e1019. doi: 10.1016/j.cell.2019.03.030
124. Whiteaker JR, Halusa GN, Hoofnagle AN, Sharma V, MacLean B, Yan P, et al. CPTAC Assay Portal: a repository of targeted proteomic assays. Nat Methods. (2014) 11:703–4. doi: 10.1038/nmeth.3002
125. Mertins P, Tang LC, Krug K, Clark DJ, Gritsenko MA, Chen L, et al. Reproducible workflow for multiplexed deep-scale proteome and phosphoproteome analysis of tumor tissues by liquid chromatography-mass spectrometry. Nat Protoc. (2018) 13:1632–61. doi: 10.1038/s41596-018-0006-9
126. Myers SA, Rhoads A, Cocco AR, Peckner R, Haber AL, Schweitzer LD, et al. Streamlined protocol for deep proteomic profiling of FAC-sorted cells and its application to freshly isolated murine immune cells. Mol Cell Proteomics. (2019) 18:995–1009. doi: 10.1074/mcp.RA118.001259
127. Yi L, Tsai CF, Dirice E, Swensen AC, Chen J, Shi T, et al. Boosting to Amplify Signal with Isobaric Labeling (BASIL) strategy for comprehensive quantitative phosphoproteomic characterization of small populations of cells. Anal Chem. (2019) 91:5794–801. doi: 10.1021/acs.analchem.9b00024
128. Krug K, Mertins P, Zhang B, Hornbeck P, Raju R, Ahmad R, et al. A curated resource for phosphosite-specific signature analysis. Mol Cell Proteomics. (2019) 18:576–93. doi: 10.1074/mcp.TIR118.000943
129. LeCun Y, Bengio Y, Hinton G. Deep learning. Nature. (2015) 521:436–44. doi: 10.1038/nature14539
130. Shen J, Qi L, Zou Z, Du J, Kong W, Zhao L, et al. Identification of a novel gene signature for the prediction of recurrence in HCC patients by machine learning of genome-wide databases. Sci Rep. (2020) 10:4435. doi: 10.1038/s41598-020-61298-3
131. Azuaje F, Kim SY, Perez Hernandez D, Dittmar G. Connecting histopathology imaging and proteomics in kidney cancer through machine learning. J Clin Med. (2019) 8. doi: 10.3390/jcm8101535
132. Altschuler SJ, Wu LF. Cellular heterogeneity: do differences make a difference? Cell. (2010) 141:559–63. doi: 10.1016/j.cell.2010.04.033
133. Wang D, Bodovitz S. Single cell analysis: the new frontier in “omics.” Trends Biotechnol. (2010) 28:281–90. doi: 10.1016/j.tibtech.2010.03.002
134. Kelly RT. Single-cell proteomics: progress and prospects. Mol Cell Proteomics. (2020) 19:1739–48. doi: 10.1074/mcp.R120.002234
135. Evers TMJ, Hochane M, Tans SJ, Heeren RMA, Semrau S, Nemes P, et al. Deciphering metabolic heterogeneity by single-cell analysis. Anal Chem. (2019) 91:13314–23. doi: 10.1021/acs.analchem.9b02410
136. Slavov N. Single-cell protein analysis by mass spectrometry. Curr Opin Chem Biol. (2021) 60:1–9. doi: 10.1016/j.cbpa.2020.04.018
137. Specht H, Emmott E, Petelski AA, Huffman RG, Perlman DH, Serra M, et al. Single-cell proteomic and transcriptomic analysis of macrophage heterogeneity using SCoPE2. Genome Biol. (2021) 22:50. doi: 10.1186/s13059-021-02267-5
138. Hughes AJ, Spelke DP, Xu Z, Kang CC, Schaffer DV, Herr AE. Single-cell western blotting. Nat Methods. (2014) 11:749–55. doi: 10.1038/nmeth.2992
139. Lo CA, Kays I, Emran F, Lin TJ, Cvetkovska V, Chen BE. Quantification of protein levels in single living cells. Cell Rep. (2015) 13:2634–44. doi: 10.1016/j.celrep.2015.11.048
140. Budnik B, Levy E, Harmange G, Slavov N. SCoPE-MS: mass spectrometry of single mammalian cells quantifies proteome heterogeneity during cell differentiation. Genome Biol. (2018) 19:161. doi: 10.1186/s13059-018-1547-5
141. Zhang L, Xiao H, Zhou H, Santiago S, Lee JM, Garon EB, et al. Development of transcriptomic biomarker signature in human saliva to detect lung cancer. Cell Mol Life Sci. (2012) 69:3341–50. doi: 10.1007/s00018-012-1027-0
142. Yang W, Soares J, Greninger P, Edelman EJ, Lightfoot H, Forbes S, et al. Genomics of Drug Sensitivity in Cancer (GDSC): a resource for therapeutic biomarker discovery in cancer cells. Nucleic Acids Res. (2013) 14:D955–61. doi: 10.1093/nar/gks1111
143. Wang Z, Gerstein M, Snyder M. RNA-Seq: a revolutionary tool for transcriptomics. Nat Rev Genet. (2009) 10:57–63. doi: 10.1038/nrg2484
144. Chen L, Liu S, Tao Y. Regulating tumor suppressor genes: post-translational modifications. Signal Transduct Target Ther. (2020) 5:90. doi: 10.1038/s41392-020-0196-9
145. Doucet A, Butler GS, Rodriguez D, Prudova A, Overall CM. Metadegradomics: toward in vivo quantitative degradomics of proteolytic post-translational modifications of the cancer proteome. Mol Cell Proteomics. (2008) 7:1925–51. doi: 10.1074/mcp.R800012-MCP200
146. Mun DG, Bhin J, Kim S, Kim H, Jung JH, Jung Y, et al. Proteogenomic characterization of human early-onset gastric cancer. Cancer Cell. (2019) 35:111–24 e110. doi: 10.1016/j.ccell.2018.12.003
147. Sinha A, Huang V, Livingstone J, Wang J, Fox NS, Kurganovs N, et al. The proteogenomic landscape of curable prostate cancer. Cancer Cell. (2019) 35:414–27 e416. doi: 10.1016/j.ccell.2019.02.005
148. Zhang B, Whiteaker JR, Hoofnagle AN, Baird GS, Rodland KD, Paulovich AG. Clinical potential of mass spectrometry-based proteogenomics. Nat Rev Clin Oncol. (2019) 16:256–68. doi: 10.1038/s41571-018-0135-7
149. Savage SR, Shi Z, Liao Y, Zhang B. Graph algorithms for condensing and consolidating gene set analysis results. Mol Cell Proteomics. (2019) 18(8Suppl.1):S141–52. doi: 10.1074/mcp.TIR118.001263
150. Wen B, Wang X, Zhang B. PepQuery enables fast, accurate, and convenient proteomic validation of novel genomic alterations. Genome Res. (2019) 29:485–93. doi: 10.1101/gr.235028.118
151. Boja E, Tezak Z, Zhang B, Wang P, Johanson E, Hinton D, et al. Right data for right patient-a precisionFDA NCI-CPTAC multi-omics mislabeling challenge. Nat Med. (2018) 24:1301–2. doi: 10.1038/s41591-018-0180-x
152. Office IDAC, Committee IIDA. Analysis of five years of controlled access and data sharing compliance at the International Cancer Genome Consortium. Nat Genet. (2016) 48:224–5. doi: 10.1038/ng.3499
153. He Y, Mohamedali A, Huang C, Baker MS, Nice EC. Oncoproteomics: current status and future opportunities. Clin Chim Acta. (2019) 495:611–24. doi: 10.1016/j.cca.2019.06.006
154. Tong M, Yu C, Zhan D, Zhang M, Zhen B, Zhu W, et al. Molecular subtyping of cancer and nomination of kinase candidates for inhibition with phosphoproteomics: reanalysis of CPTAC ovarian cancer. EBioMedicine. (2019) 40:305–17. doi: 10.1016/j.ebiom.2018.12.039
155. Zhang J, Bajari R, Andric D, Gerthoffert F, Lepsa A, Nahal-Bose H, et al. The international cancer genome consortium data portal. Nat Biotechnol. (2019) 37:367–9. doi: 10.1038/s41587-019-0055-9
Glossary
LC-MS/MS, liquid chromatography-tandem mass spectrometry; SILAC, stable isotope labeling by with amino acids in cell culture; ICAT, isotope-coded affinity tag; TMT, tandem mass tag; iTRAQ, isobaric tags for relative and absolute quantitation; MRM, multiple reaction monitoring; SWATH, sequential window acquisition of all theoretical fragment ion spectra; ELISA, enzyme-linked immunosorbent assay; PYCR2, Pyrroline-5-Carboxylate Reductase 2; ADH1A, Alcohol dehydrogenase 1A; CTNNB1, Catenin Beta 1; ALDOA, Aldolase A; LKB1, Liver kinase B1; mTOR. mammalian target of rapamycin; NNMT, N-methyltransferase; ROR1, Receptor-tyrosine-kinase-like orphan receptor 1; Nrf2, nuclear factor erythroid 2–related factor 2; Bach1, BTB domain and CNC homolog 1; Keap1, Kelch-like ECH-associated protein 1; Fbxo22, F-box only protein 22; YBX1, Y-box binding protein 1; CDH18, Cadherin 18; UQCRC2, Ubiquinol-cytochrome c reductase core protein 2; miRNA, MicroRNA; EGFR, Epidermal growth factor receptor; SDC1, Syndecan-1; CCT2, Chaperonin containing TCP1 subunit 2; CCT6A, Chaperonin containing TCP1 subunit 6A; TCP1, T-Complex 1; LRG1, Leucine-Rich Alpha-2-Glycoprotein 1; CRP, C-reactive protein; C9, Complement component C9; CNS, Central nervous system; BASIL, Boosting to amplify signal with isobaric labeling.
Keywords: cancer, proteomics, multi-omics, biomarkers, translational research
Citation: Kwon YW, Jo H-S, Bae S, Seo Y, Song P, Song M and Yoon JH (2021) Application of Proteomics in Cancer: Recent Trends and Approaches for Biomarkers Discovery. Front. Med. 8:747333. doi: 10.3389/fmed.2021.747333
Received: 26 July 2021; Accepted: 26 August 2021;
Published: 22 September 2021.
Edited by:
Enrico Capobianco, University of Miami, United StatesReviewed by:
Canhua Huang, Sichuan University, ChinaBogang Wu, George Washington University, United States
Copyright © 2021 Kwon, Jo, Bae, Seo, Song, Song and Yoon. This is an open-access article distributed under the terms of the Creative Commons Attribution License (CC BY). The use, distribution or reproduction in other forums is permitted, provided the original author(s) and the copyright owner(s) are credited and that the original publication in this journal is cited, in accordance with accepted academic practice. No use, distribution or reproduction is permitted which does not comply with these terms.
*Correspondence: Minseok Song, bWluc2VvayYjeDAwMDQwO3l1LmFjLmty; Jong Hyuk Yoon, amh5b29uJiN4MDAwNDA7a2JyaS5yZS5rcg==