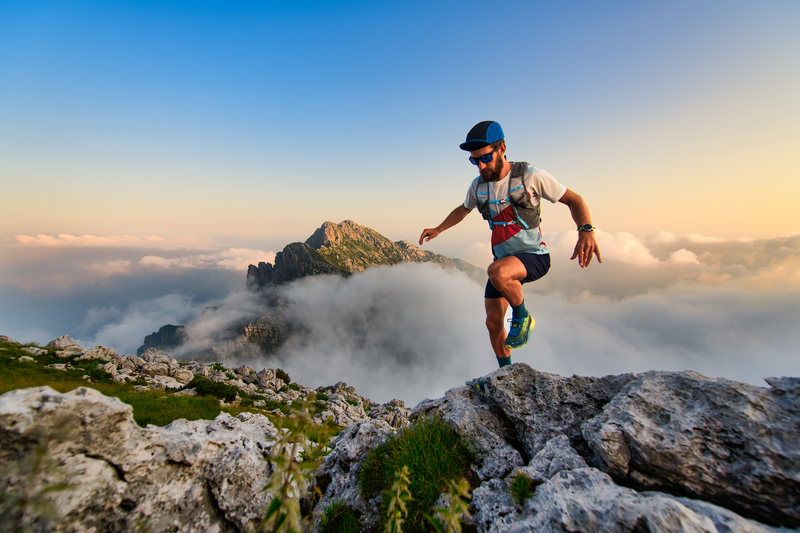
94% of researchers rate our articles as excellent or good
Learn more about the work of our research integrity team to safeguard the quality of each article we publish.
Find out more
ORIGINAL RESEARCH article
Front. Med. , 07 September 2021
Sec. Translational Medicine
Volume 8 - 2021 | https://doi.org/10.3389/fmed.2021.738687
Objectives: Uncovering the genetic basis of COVID-19 may shed insight into its pathogenesis and help to improve treatment measures. We aimed to investigate the host genetic variants associated with COVID-19.
Methods: The summary result of a COVID-19 GWAS (9,373 hospitalized COVID-19 cases and 1,197,256 controls) was obtained from the COVID-19 Host Genetic Initiative GWAS meta-analyses. We tested colocalization of the GWAS signals of COVID-19 with expression and methylation quantitative traits loci (eQTL and mQTL, respectively) using the summary data-based Mendelian randomization (SMR) analysis. Four eQTL and two mQTL datasets were utilized in the SMR analysis, including CAGE blood eQTL data (n = 2,765), GTEx v7 blood (n = 338) and lung (n = 278) eQTL data, Geuvadis lymphoblastoid cells eQTL data, LBC-BSGS blood mQTL data (n = 1,980), and Hannon blood mQTL summary data (n = 1,175). We conducted a transcriptome-wide association study (TWAS) on COVID-19 with precomputed prediction models of GTEx v8 eQTL in lung and blood using S-PrediXcan.
Results: Our SMR analyses identified seven protein-coding genes (TYK2, IFNAR2, OAS1, OAS3, XCR1, CCR5, and MAPT) associated with COVID-19, including two novel risk genes, CCR5 and tau-encoding MAPT. The TWAS revealed four genes for COVID-19 (CXCR6, CCR5, CCR9, and PIGN), including two novel risk genes, CCR5 and PIGN.
Conclusion: Our study highlighted the functional relevance of some known genome-wide risk genes of COVID-19 and revealed novel genes contributing to differential outcomes of COVID-19 disease.
Severe acute respiratory syndrome coronavirus 2 (SARS-CoV-2) and resultant COVID-19 have created a public health crisis worldwide. The majority of infected persons are either affected mildly or stay asymptomatic. It was reported that ~10–20% of people with COVID-19 infection need hospitalization (1). Hypertension, obesity, and diabetes are among the common comorbidities of hospitalized patients (2). Patients with older age or medical complications tend to have severe symptoms. However, some young and seemingly healthy individuals may also have serious outcomes from the virus infection. As the symptoms, severity, and prognosis of the disease are highly variable, host genetics may influence human's susceptibility to COVID-19, in a similar manner as it was noted for other infectious diseases (3).
The need in elucidating the genetic drivers of the development of COVID-19 is urgent as it may allow novel insights into its pathogenesis. Host Genetic Initiative (HGI) is one of the global efforts to promote human genetic variance research of COVID-19 by platform building, analytical activities, and data sharing (4). Genome-wide association studies (GWASs) have been conducted worldwide to characterize gene variants defining the susceptibility and severity of the COVID-19. In particular, Severe Covid-19 GWAS Group has identified two loci associated with SARS-CoV-2 related respiratory failure, including the chr3p21.31 locus with multiple genes encoding chemokine receptors and the chr9q34.2 locus with the blood type gene ABO (5). Pairo-Castineira et al. also revealed a set of genetic variants enriched in COVID-19 patients admitted to intensive care units [6]. This set highlighted chr3p21.31, chr12q24.13 (OAS1, OAS2, and OAS3), chr19p13.2 (TYK2), chr19p13.3 (DPP9), and chr21q22.1 (IFNAR2) (6). These two studies provide valuable evidence for the genetic basis of COVID-19. Both of the studies analyzed the datasets collected by HGI at the early stages of the project.
To get a clear understanding of these GWAS outputs and gain more insight into the SARS-CoV-2 pathophysiology, we performed summary data-based Mendelian randomization (SMR) and transcriptome-wide association analyses. We prioritized the genes co-localized with the COVID-19 GWAS hits and mapped additional involved genes. The resultant list of genes suggests potential therapeutic targets for symptomatic COVID-19.
The summary result of a COVID-19 GWAS (9,373 hospitalized COVID-19 cases and 1,197,256 controls, excluding 23andMe) was obtained from the COVID-19 Host Genetic Initiative (HGI) GWAS meta-analyses round 5 (Release Date: January 18, 2021) (4). All the participants were of European origins. Ethical approval had been obtained in all original studies. A more detailed description of the datasets is provided in the Supplementary File.
FUMA was used to map SNPs to genes and identify genomic regions independent of linkage disequilibrium (LD) (7). All genes located closer than 10 kb of each variant were mapped. Independent significant SNPs (IndSigSNPs) were extracted, according to criteria of significance at the genome level (P ≤ 5.0 × 10−8) and of independence (r2 < 0.6). For each group of IndSigSNPs, lead SNPs were identified when they were in LD with each other at r2 < 0.1 within a 500 Kb window. The merging of lead SNPs into genomic risk loci was performed when they were located at a distance of <500 kb from each other. Clumping was carried out according to the European 1,000 Genomes Project phase 3 reference panel, with the entire MHC locus being merged into one region (chr6:25-35Mb).
Colocalization of GWAS signal with expression and methylation quantitative traits loci (eQTL and mQTL, respectively) was performed in a framework of the SMR v1.03 (8). In this, the GWAS summary result and eQTL data were used at the gene level to associate its expression level with a trait of interest. We utilized four eQTL and two mQTL datasets, including CAGE blood eQTL data (n = 2,765) (9), GTEx v7 blood (n = 338) and lung (n = 278) eQTL data (10), Geuvadis lymphoblastoid cells eQTL data (n = 373) (11), LBC-BSGS blood mQTL data (n = 1,980) (12), and Hannon blood mQTL summary data (n = 1,175) (13). Bonferroni procedure was employed to adjust P-values for multiple testing. Pleiotropic effects were sorted from the LD artifacts using the test for non-significant heterogeneity (PHEIDI > 0.01) which is embedded in the SMR analysis workflow.
Putatively causal genes were prioritized by a TWAS procedure, which was conducted for the lung and the whole blood cells. The gene-level association results were calculated from GWAS summary statistics using S-PrediXcan (14, 15). We used precomputed prediction models of GTEx v8 (16) eQTL and LD references from http://predictdb.org/. Bonferroni procedure was employed to adjust P-values for multiple testing.
A total of six genomic loci were identified in the COVID-19 dataset, respectively (Table 1; Supplementary Figure 1, Table 1). The 3p21.31 locus contains the largest amount of association signals and genes (Supplementary Figure 2). A total of 20 genome-wide genes were detected for the COVID-19 GWAS (Table 1). These genes included LIMD1, SLC6A20, LZTFL1, CCR9, FYCO1, CXCR6, XCR1, CCR3, FLT1P1, CCR1, UQCRC2P1, CCR2, LRRC2, VSTM2A, ABO, OAS1, OAS3, OAS2, DPP9, and IFNAR2. Two of these genes, FLT1P1 and UQCRC2P1, are non-coding genes.
Functionally important genes for COVID-19 were prioritized by the SMR analysis using six eQTL and two mQTL datasets, which identified a total of 25 associations, involving seven protein-coding genes (TYK2, IFNAR2, OAS1, OAS3, XCR1, CCR5, and MAPT) and four non-coding genes (LRRC37A4P, IL10RB-AS1, MGC57346, and CCR5AS) (Table 2; Figure 1). Several genes were implicated by two or more datasets, including IFNAR2 (eQTL of CAGE blood, eQTL of Geuvadis lymphoblastoid cell, and mQTL of LBC-BSGS blood), OAS1 (eQTL of Geuvadis lymphoblastoid cell and mQTL of Hannon blood), and XCR1 (mQTL of Hannon blood and mQTL of mQTL of Hannon blood). Two protein-coding genes, CCR5 and MAPT, are novel susceptibility genes for COVID-19, which were implicated by mQTL of Hannon blood and mQTL of LBC-BSGS blood, respectively (Figure 2).
Figure 1. Summary data-based Mendelian randomization (SMR) analysis of COVID-19. Each horizontal dashed line denotes a genome-wide significance level adjusted by Bonferroni.
Figure 2. Two loci from the SMR analysis. (A): Top plot, gray dots represent the P-values for SNPs from the COVID-19 GWAS. Bottom plots, the mQTL P-values of SNPs from the Hannon study for the cg14546840 and cg17786516 probes tagging CCR5 and CCR5AS, respectively. Highlighted in red are the genes (CCR5 and CCR5AS) that passed the SMR and HEIDI tests. (B): Top plot, gray dots represent the P-values for SNPs from the COVID-19 GWAS. The bottom plot, the mQTL P-values of SNPs from the LBC-BSGS study for the cg02228913 probe tagging MAPT. Highlighted in red is the gene (MAPT) that passed the SMR and HEIDI tests.
To connect GWAS signals to tissue-specific gene expression values, the TWAS framework was used (14, 15). Inferences were made for known genetic variants in lung and whole blood tissues from the GTEx v8 expression dataset. We discovered two genes associated with the lung eQTL dataset (CXCR6 and CCR5) and two genes associated with the blood eQTL dataset (CCR9 and PIGN) (Table 3). Among these genes, CCR5 and PIGN were novel susceptibility genes for COVID-19.
Together, our SMR analysis and TWAS of COVID-19 identified a total of 14 genes associated with COVID-19, comprising seven genes implicated by the input or previous GWASs and seven novel genes (including three protein-coding genes, CCR5, MAPT, and PIGN).
Exploration of the host genetic factors contributing COVID-19 has being started as early as the first datasets became available, including ones collected in HGI. Here we present the result of our exploration of the differential susceptibility to COVID-19 in the latest HGI dataset, which we dissected using both SMR and transcriptome-wide analyses.
Genomic loci, as well as risk genes associated with the disease, were described. Notably, chromosome 3p21.31 with its chemokine receptor genes was highlighted as the peak for associations, along with chromosome 12q24.13 with the oligoadenylate synthase protein family gene cluster OAS1, OAS2, and OAS3. These enzymes activate RNAse L and degrade viral nucleic acids. The IFNAR2 gene (21q22.11 locus) encodes a subunit for interferons alpha and beta binding receptors. Notably, IFNAR2 is capable of producing soluble receptors, which binds and regulates endogenous production of type I IFNs (17, 18). This soluble IFNAR2 possesses both anti-proliferative and antiviral functions as well as therapeutic properties (19). In particular, in COVID-19, a protective role of IFNAR2 has been suggested (6, 20). Another region previously associated with COVID-19 was the blood group ABO locus at 9q34.2 [5].
Out of 18 protein-coding genes associated with COVID-19, twelve have been reported previously (5, 6), including ABO, CCR9, CXCR6, DPP9, FYCO1, IFNAR2, LZTFL1, OAS1, OAS2, OAS3, SLC6A20, and XCR1. The present analysis uncovered six novel risk genes contributing to severe COVID-19, including LIMD1, CCR3, CCR1, CCR2, LRRC2, and VSTM2A. A majority of these six genes are located in the 3p21.31 locus (CCR1, CCR2, CCR3, CCRL2, and LRRC2), while another gene VSTM2A maps to newly identified loci in the 7q31.1.
Our SMR analysis identified seven protein-coding genes significantly associated with predicted expression levels in the lung or the blood. Among these genes, two are novel, including CCR5 and MAPT. Our TWAS analysis identified four genes significantly associated with predicted expression levels in the lung or the blood. Two of these genes, CCR9 and CXCR6, were also found within the set of COVID-19 associated genes, while CCR5 and PIGN genes were novel. Together, our SMR and TWAS analyses identified three novel protein-coding genes for COVID-19, namely, CCR5, MAPT, and PIGN.
CCR5 is located in 3p21.31 and encodes chemokine receptors expressed in macrophages and T cells. In macrophages, CCR5 protein serves as a gateway for many viruses including HIV (21). Notably, some people lack functional CCR5 allele due to 31-bp deletion within its open reading frame, and resultant loss-of-the function. In both homo- and heterozygous individuals this deletion known as rs333 is a major determinant of the resistance to HIV. It is of interest that anti-CCR5 antibody leronlimab has been tried as a post-COVID-19 therapeutic molecule and shown to downregulate both inflammatory cytokine profile and the copy number of SARS-CoV2 RNA (22). In a recent study, the CCR5-Δ32 variant was found to be significantly less frequent in hospitalized COVID-19 than in healthy controls (P = 0.01, OR = 0.66, 95% CI = 0.49–0.88), with no homozygotes found among the patients, compared to 1% of the controls (23). In addition, CCR5 transcript was expressed among the patients at significantly higher levels than in the healthy non-deletion carriers (P = 0.01). Independent identification of CCR5 as overexpressed in hospitalized COVID-19 patients supports the validity of our TWAS analysis.
The MAPT gene encodes the microtubule-associated protein tau. Genetic variation within MAPT was reported to be associated with multiple neurodegenerative disorders, including Parkinson's disease and Alzheimer's disease (24–26). It was also a genome-wide risk gene for autoimmune diseases and some cardiometabolic traits, including body mass index, blood cell count, osteoarthritis (25, 27–29). In addition, the gene has been implicated in interstitial lung disease (30) and lung function (31). Recently, it was shown that the tau protein binds to SARS-CoV-2 S1 receptor-binding domain (RBD) with the implication that the heparin-binding site on the S1 protein participates in the aggregation of amyloid-like proteins and promotes neurodegeneration (32). In another study, amounts of tau protein in the neuronal-enriched extracellular vesicles of patients recovering from COVID-19 were larger than in historic controls (33). In 3D human brain organoids, SARS-CoV-2 preferably targets the neurons, where it changes the distribution of Tau from axons to soma, its hyperphosphorylation, the neurotoxic death (34). Taken together, these observations point that functional variation within the tau locus may indeed be relevant to COVID-19 and especially to its neurological sequelae.
Mutations in gene PIGN lead to well-characterized defects in the biosynthesis of glycosylphosphatidylinositol (GPI), an anchor that tether proteins to the extracellular face of eukaryotic plasma membranes (35). Notably, genome-scale CRISPR knockout screen of cells seeded with SARS-CoV-2 and three seasonal coronaviruses (HCoV-OC43, HCoV-NL63, and HCoV-229E) highlighted glycosylphosphatidylinositol biosynthesis as one of the key dependencies for these infectious agents in two independent studies (36, 37). In the mammalian plasma membranes, GPI-anchored proteins interact with glycosphingolipids, forming dynamic microdomains. When the transfer of synthesized GPI to proteins is defective, for the lack of a particular glycosphingolipid, a lactosylceramide, the GPI biosynthesis in the endoplasmic reticulum (ER) is severely suppressed (38). The metabolism of ceramides, and lactosylceramide, in particular, is severely disturbed in SARS-Cov-2 infection, with glucosylceramide synthase inhibitors displaying marked anti-SARS-CoV-2 effects (39). It seems that GPI-glucosylceramide equilibrium may be profoundly altered by SARS-CoV-2 replication and that these changes may contribute to COVID sequelae.
The strengths of this study include the use of the largest COVID-19 dataset available. Furthermore, we have diminished the potential population heterogeneity by limiting our analysis to individuals of European ancestry. Among the noticeable study limitations are that TWAS associations are considered to be noisy and that we have limited ourselves to testing only the genetic factors associated with COVID-19 risk, rather than taking into account the social and the environmental variables as well. As the findings from our study may be relevant to the European population only, uncovered associations certainly require further validation and detailed investigation.
In summary, our study highlighted some known and revealed some novel genes contributing to differential outcomes of COVID-19 disease.
Publicly available datasets were analyzed in this study. This data can be found here: https://www.covid19hg.org/results/.
FZ designed the study and performed the statistical analyses. FZ and AB contributed to data interpretation and wrote the manuscript. HC contributed to the data preparation. All the authors approved the final manuscript for submission and publication and agreed to be accountable for all aspects of the work.
The authors declare that the research was conducted in the absence of any commercial or financial relationships that could be construed as a potential conflict of interest.
All claims expressed in this article are solely those of the authors and do not necessarily represent those of their affiliated organizations, or those of the publisher, the editors and the reviewers. Any product that may be evaluated in this article, or claim that may be made by its manufacturer, is not guaranteed or endorsed by the publisher.
The authors thank all investigators and participants from the COVID-19 Host Genetics Initiative for sharing these data.
The Supplementary Material for this article can be found online at: https://www.frontiersin.org/articles/10.3389/fmed.2021.738687/full#supplementary-material
1. Richardson S, Hirsch JS, Narasimhan M. Clarification of mortality rate and data in abstract, results, and Table 2. JAMA. (2020) 323:2098. doi: 10.1001/jama.2020.7681
2. Richardson S, Hirsch JS, Narasimhan M, Crawford JM, McGinn T, Davidson KW, et al. Presenting characteristics, comorbidities, and outcomes among 5700 patients hospitalized with COVID-19 in the New York City Area. JAMA. (2020) 323:2052–9. doi: 10.1001/jama.2020.6775
3. Chapman SJ, Hill AV. Human genetic susceptibility to infectious disease. Nat Rev Genet. (2012) 13:175–88. doi: 10.1038/nrg3114
4. Initiative C-HG. The COVID-19 host genetics initiative, a global initiative to elucidate the role of host genetic factors in susceptibility and severity of the SARS-CoV-2 virus pandemic. Eur J Hum Genet. (2020) 28:715–8. doi: 10.1038/s41431-020-0636-6
5. Severe Covid GG, Ellinghaus D, Degenhardt F, Bujanda L, Buti M, Albillos A, et al. Genomewide association study of severe Covid-19 with respiratory failure. N Engl J Med. (2020) 383:1522–34. doi: 10.1056/NEJMoa2020283
6. Pairo-Castineira E, Clohisey S, Klaric L, Bretherick AD, Rawlik K, Pasko D, et al. Genetic mechanisms of critical illness in COVID-19. Nature. (2021) 591:92–8. doi: 10.1038/s41586-020-03065-y
7. Watanabe K, Taskesen E, van Bochoven A, Posthuma D. Functional mapping and annotation of genetic associations with FUMA. Nat Commun. (2017) 8:1826. doi: 10.1038/s41467-017-01261-5
8. Zhu Z, Zhang F, Hu H, Bakshi A, Robinson MR, Powell JE, et al. Integration of summary data from GWAS and eQTL studies predicts complex trait gene targets. Nat Genet. (2016) 48:481–7. doi: 10.1038/ng.3538
9. Lloyd-Jones LR, Holloway A, McRae A, Yang J, Small K, Zhao J, et al. The genetic architecture of gene expression in peripheral blood. Am J Hum Genet. (2017) 100:228–37. doi: 10.1016/j.ajhg.2016.12.008
10. Consortium GT, Laboratory DA, Coordinating Center -Analysis Working G, Statistical Methods groups-Analysis Working G, Enhancing Gg, Fund NIHC, et al. Genetic effects on gene expression across human tissues. Nature. (2017) 550:204–13. doi: 10.1038/nature24277
11. Lappalainen T, Sammeth M, Friedlander MR, t Hoen PA, Monlong J, Rivas MA, et al. Transcriptome and genome sequencing uncovers functional variation in humans. Nature. (2013) 501:506–11. doi: 10.1038/nature12531
12. Wu Y, Zeng J, Zhang F, Zhu Z, Qi T, Zheng Z, et al. Integrative analysis of omics summary data reveals putative mechanisms underlying complex traits. Nat Commun. (2018) 9:918. doi: 10.1038/s41467-018-03371-0
13. Hannon E, Gorrie-Stone TJ, Smart MC, Burrage J, Hughes A, Bao Y, et al. Leveraging DNA-methylation quantitative-trait loci to characterize the relationship between methylomic variation, gene expression, and complex traits. Am J Hum Genet. (2018) 103:654–65. doi: 10.1016/j.ajhg.2018.09.007
14. Gamazon ER, Wheeler HE, Shah KP, Mozaffari SV, Aquino-Michaels K, Carroll RJ, et al. A gene-based association method for mapping traits using reference transcriptome data. Nat Genet. (2015) 47:1091–8. doi: 10.1038/ng.3367
15. Barbeira AN, Dickinson SP, Bonazzola R, Zheng J, Wheeler HE, Torres JM, et al. Exploring the phenotypic consequences of tissue specific gene expression variation inferred from GWAS summary statistics. Nat Commun. (2018) 9:1825. doi: 10.1038/s41467-018-03621-1
16. Consortium GT. The GTEx consortium atlas of genetic regulatory effects across human tissues. Science. (2020) 369:1318–30. doi: 10.1126/science.aaz1776
17. Shepardson KM, Larson K, Johns LL, Stanek K, Cho H, Wellham J, et al. IFNAR2 is required for anti-influenza immunity and alters susceptibility to post-influenza bacterial superinfections. Front Immunol. (2018) 9:2589. doi: 10.3389/fimmu.2018.02589
18. Hardy MP, Owczarek CM, Trajanovska S, Liu X, Kola I, Hertzog PJ. The soluble murine type I interferon receptor Ifnar-2 is present in serum, is independently regulated, and has both agonistic and antagonistic properties. Blood. (2001) 97:473–82. doi: 10.1182/blood.V97.2.473
19. Hurtado-Guerrero I, Hernaez B, Pinto-Medel MJ, Calonge E, Rodriguez-Bada JL, Urbaneja P, et al. Antiviral, immunomodulatory and antiproliferative activities of recombinant soluble IFNAR2 without IFN-ss mediation. J Clin Med. (2020) 9:959. doi: 10.3390/jcm9040959
20. Liu D, Yang J, Feng B, Lu W, Zhao C, Li L. Mendelian randomization analysis identified genes pleiotropically associated with the risk and prognosis of COVID-19. J Infect. (2021) 82:126–32. doi: 10.1016/j.jinf.2020.11.031
21. Alkhatib G, Combadiere C, Broder CC, Feng Y, Kennedy PE, Murphy PM, et al. CC CKR5: a RANTES, MIP-1alpha, MIP-1beta receptor as a fusion cofactor for macrophage-tropic HIV-1. Science. (1996) 272:1955–8. doi: 10.1126/science.272.5270.1955
22. Patterson BK, Seethamraju H, Dhody K, Corley MJ, Kazempour K, Lalezari J, et al. CCR5 inhibition in critical COVID-19 patients decreases inflammatory cytokines, increases CD8 T-cells, and decreases SARS-CoV2 RNA in plasma by day 14. Int J Infect Dis. (2020) 103:25–32. doi: 10.1016/j.ijid.2020.10.101
23. Cuesta-Llavona E, Gomez J, Albaiceta GM, Amado-Rodriguez L, Garcia-Clemente M, Gutierrez-Rodriguez J, et al. Variant-genetic and transcript-expression analysis showed a role for the chemokine-receptor CCR5 in COVID-19 severity. Int Immunopharmacol. (2021) 98:107825. doi: 10.1016/j.intimp.2021.107825
24. Chang D, Nalls MA, Hallgrimsdottir IB, Hunkapiller J, van der Brug M, Cai F, et al. A meta-analysis of genome-wide association studies identifies 17 new Parkinson's disease risk loci. Nat Genet. (2017) 49:1511–6. doi: 10.1038/ng.3955
25. Witoelar A, Jansen IE, Wang Y, Desikan RS, Gibbs JR, Blauwendraat C, et al. Genome-wide pleiotropy between parkinson disease and autoimmune diseases. JAMA Neurol. (2017) 74:780–92. doi: 10.1001/jamaneurol.2017.0469
26. Zhang CC, Zhu JX, Wan Y, Tan L, Wang HF, Yu JT, et al. Meta-analysis of the association between variants in MAPT and neurodegenerative diseases. Oncotarget. (2017) 8:44994–5007. doi: 10.18632/oncotarget.16690
27. Zhu Z, Guo Y, Shi H, Liu CL, Panganiban RA, Chung W, et al. Shared genetic and experimental links between obesity-related traits and asthma subtypes in UK Biobank. J Allergy Clin Immunol. (2020) 145:537–49. doi: 10.1016/j.jaci.2019.09.035
28. Tachmazidou I, Hatzikotoulas K, Southam L, Esparza-Gordillo J, Haberland V, Zheng J, et al. Identification of new therapeutic targets for osteoarthritis through genome-wide analyses of UK Biobank data. Nat Genet. (2019) 51:230–6. doi: 10.1038/s41588-018-0327-1
29. Chen MH, Raffield LM, Mousas A, Sakaue S, Huffman JE, Moscati A, et al. Trans-ethnic and ancestry-specific blood-cell genetics in 746,667 individuals from 5 global populations. Cell. (2020) 182:1198–213 e1114. doi: 10.1016/j.cell.2020.06.045
30. Fingerlin TE, Murphy E, Zhang W, Peljto AL, Brown KK, Steele MP, et al. Genome-wide association study identifies multiple susceptibility loci for pulmonary fibrosis. Nat Genet. (2013) 45:613–20. doi: 10.1038/ng.2609
31. Kichaev G, Bhatia G, Loh PR, Gazal S, Burch K, Freund MK, et al. Leveraging polygenic functional enrichment to improve GWAS power. Am J Hum Genet. (2019) 104:65–75. doi: 10.1016/j.ajhg.2018.11.008
32. Idrees D, Kumar V. SARS-CoV-2 spike protein interactions with amyloidogenic proteins: potential clues to neurodegeneration. Biochem Biophys Res Commun. (2021) 554:94–8. doi: 10.1016/j.bbrc.2021.03.100
33. Sun B, Tang N, Peluso MJ, Iyer NS, Torres L, Donatelli JL, et al. Characterization and biomarker analyses of post-COVID-19 complications and neurological manifestations. Cells. (2021) 10:386. doi: 10.3390/cells10020386
34. Ramani A, Muller L, Ostermann PN, Gabriel E, Abida-Islam P, Muller-Schiffmann A, et al. SARS-CoV-2 targets neurons of 3D human brain organoids. EMBO J. (2020) 39:e106230. doi: 10.15252/embj.2020106230
35. Nakagawa T, Taniguchi-Ikeda M, Murakami Y, Nakamura S, Motooka D, Emoto T, et al. A novel PIGN mutation and prenatal diagnosis of inherited glycosylphosphatidylinositol deficiency. Am J Med Genet A. (2016) 170A:183–88. doi: 10.1002/ajmg.a.37397
36. Schneider WM, Luna JM, Hoffmann HH, Sanchez-Rivera FJ, Leal AA, Ashbrook AW, et al. Genome-scale identification of SARS-CoV-2 and pan-coronavirus host factor networks. Cell. (2021) 184:120–32 e114. doi: 10.1016/j.cell.2020.12.006
37. Hoffmann HH, Sanchez-Rivera FJ, Schneider WM, Luna JM, Soto-Feliciano YM, Ashbrook AW, et al. Functional interrogation of a SARS-CoV-2 host protein interactome identifies unique and shared coronavirus host factors. Cell Host Microbe. (2020) 29:267–280.e5. doi: 10.1101/2020.09.11.291716
38. Wang Y, Maeda Y, Liu YS, Takada Y, Ninomiya A, Hirata T, et al. Cross-talks of glycosylphosphatidylinositol biosynthesis with glycosphingolipid biosynthesis and ER-associated degradation. Nat Commun. (2020) 11:860. doi: 10.1038/s41467-020-14678-2
Keywords: GWAS, COVID-19, TWAS, eQTL, mQTL
Citation: Baranova A, Cao H and Zhang F (2021) Unraveling Risk Genes of COVID-19 by Multi-Omics Integrative Analyses. Front. Med. 8:738687. doi: 10.3389/fmed.2021.738687
Received: 09 July 2021; Accepted: 17 August 2021;
Published: 07 September 2021.
Edited by:
Victoria Bunik, Lomonosov Moscow State University, RussiaReviewed by:
Guenter Raddatz, German Cancer Research Center (DKFZ), GermanyCopyright © 2021 Baranova, Cao and Zhang. This is an open-access article distributed under the terms of the Creative Commons Attribution License (CC BY). The use, distribution or reproduction in other forums is permitted, provided the original author(s) and the copyright owner(s) are credited and that the original publication in this journal is cited, in accordance with accepted academic practice. No use, distribution or reproduction is permitted which does not comply with these terms.
*Correspondence: Fuquan Zhang, emhhbmdmcUBuam11LmVkdS5jbg==
Disclaimer: All claims expressed in this article are solely those of the authors and do not necessarily represent those of their affiliated organizations, or those of the publisher, the editors and the reviewers. Any product that may be evaluated in this article or claim that may be made by its manufacturer is not guaranteed or endorsed by the publisher.
Research integrity at Frontiers
Learn more about the work of our research integrity team to safeguard the quality of each article we publish.