- 1Department of Cardiology, Beijing Hospital, National Center of Gerontology, Institute of Geriatric Medicine, Chinese Academy of Medical Sciences, Beijing, China
- 2Department of Pulmonary and Critical Care Medicine, Beijing Hospital, National Center of Gerontology, Institute of Geriatric Medicine, Chinese Academy of Medical Sciences, Beijing, China
- 3Information Center, Beijing Hospital, National Center of Gerontology, Institute of Geriatric Medicine, Chinese Academy of Medical Sciences, Beijing, China
- 4Beijing Institute of Geriatrics, Beijing Hospital, National Center of Gerontology, Institute of Geriatric Medicine, Chinese Academy of Medical Sciences, Beijing, China
Background: This study aimed to develop and validate an electronic frailty index (eFI) based on routine electronic health records (EHR) for older adult inpatients and to analyze the correlations between frailty and hospitalized events and costs.
Methods: We created an eFI from routine EHR and validated the effectiveness by the consistency of the comprehensive geriatric assessment-frailty index (CGA-FI) with an independent prospective cohort. Then, we analyzed the correlations between frailty and hospitalized events and costs by regressions.
Results: During the study period, 49,226 inpatients were included in the analysis, 42,821 (87.0%) of which had enough data to calculate an eFI. A strong correlation between the CGA-FI and eFI was shown in the validation cohort of 685 subjects (Pearson's r = 0.716, P < 0.001). The sensitivity and specificity for an eFI≥0.15, the upper tertile, to identify frailty, defined as a CGA-FI≥0.25, were 64.8 and 88.7%, respectively. After adjusting for age, sex, and operation, an eFI≥0.15 showed an independent association with long hospital stay (odds ratio [OR] = 2.889, P < 0.001) and death in hospital (OR = 19.97, P < 0.001). Moreover, eFI values (per 0.1) were positively associated with total costs (β = 0.453, P < 0.001), examination costs (β = 0.269, P < 0.001), treatment costs (β = 0.414, P < 0.001), nursing costs (β = 0.381, P < 0.001), pharmacy costs (β = 0.524, P < 0.001), and material costs (β = 0.578, P < 0.001) after adjusting aforementioned factors.
Conclusions: We successfully developed an effective eFI from routine EHR from a general hospital in China. Frailty is an independent risk factor for long hospital stay and death in hospital. As the degree of frailty increases, the hospitalized costs increase accordingly.
Introduction
As the population of older adults rises globally, the condition of frailty is gaining prominent attention (1). In China, the older adults aged 65 years and older has reached 190 million, accounting for 13.5% of the total population at the end of 2020 (2). Frailty describes a decline in physiological capacity across multiple organ systems, characterized by an increased vulnerability to stressors (3). The condition of frailty is associated not only with a myriad of adverse outcomes (4) but also with increased healthcare costs (5–16). However, there is no gold standard assessment instrument for frailty (17, 18). The most widely used instruments in clinical research are variations of the frailty phenotype (19) or frailty index (FI) based on the cumulative deficit model (20). Our previous study showed that the comprehensive geriatric assessment-FI (CGA-FI) may be an optimal assessment tool among five prevalent frailty measurements (21). Although numerous clinicians have embraced the concept of frailty, we are facing large challenges to make the translation from frailty research to clinical practice. The barriers include not only a lack of consensus among prevalent frailty measurements but also time and resource limitations in busy clinical environments.
To reduce the burden of frailty assessment on clinicians, Clegg et al. developed an electronic FI (eFI) using routine electronic health records (EHR) in UK primary care (22). Based on Clegg's eFI, Pajewski and his colleagues modeled another adapted eFI with more information on nursing and laboratory assessments using routine EHR in the US, which showed an independent predictive value for mortality, hospitalizations, emergency department visits, and injurious falls (23). Meanwhile, these time-saving assessments of frailty based on EHR have shown good consistency with traditional assessment methods, such as the Fried phenotype and CGA-FI (24, 25). However, there has been no relevant frailty research based on EHR in China, so we do not know whether an effective eFI can be developed from routine EHR in China.
Previous studies on frailty in inpatients have mainly focused on the population in a certain department or with a particular disease type, which cannot fully reflect conditions across all departments. Our previous research has found that the prevalence of frailty between medical and surgical departments was significantly different (21), but there has been no research that can show in detail the distribution of frailty in each department of a general hospital. Moreover, due to limitations in data acquisition, there is currently no study using EHR to analyze the relationship between frailty and hospitalized costs. Therefore, the effective utilization of EHR big data is particularly important, which can provide valuable information for clinical management.
This study aimed to develop and validate an eFI based on routine EHR for older adult inpatients and to analyze the correlations between frailty and hospitalized events and costs.
Materials and Methods
Study Design
We conducted a cohort study on frailty using 6-year EHR of older adult inpatients from a general hospital in Beijing, China. The hospital is public, owned by government, with great attention to geriatrics and gerontology. We used a two-step approach to develop and validate an eFI based on the routine EHR for older adult inpatients. First, we followed published guidance on creating a FI using the cumulative deficit model to create an eFI from routine EHR, which involved diagnosis, nursing assessment, and laboratory tests (26). Second, in a validation cohort, we tested whether the eFI identified similar people as the CGA-FI. Then, we analyzed the correlation between frailty and hospitalized events and costs using the routine EHR.
Population in the EHR
The patients included were at least 65 years of age in the routine EHR database from July 1, 2013 to September 30, 2019. There were 96,393 hospitalizations involving 51,824 older adult inpatients in the records. During the study period, 34,612 patients had only one hospitalization, while 17,212 patients had two or more hospitalizations in the records. To avoid duplication, we chose the last hospitalization for these patients with two or more hospitalizations in the records for analysis. Considering the meaninglessness of hospital stays that were extremely short (1 day or less) and special issues regarding hospital stays that were extremely long, we excluded 5% of the patients, which included 2,006 (3.9%, 2006/51824) patients with hospital stay ≤ one day and 592 (1.1%, 592/51824) patients with hospital stay > 66 days. Therefore, 49,226 inpatients were eligible (Figure 1).
Validation Cohort
For the validation exercise, we used a linked dataset on a prospective cohort of inpatients who had been assessed with the research standard CGA-FI. These participants were enrolled from September 2018 to February 2019 in the same hospital. The characteristics of the prospective cohort were described in detail in our previously published article (21). Among the 1,000 older adult patients in the cohort study, 703 participants were matched in the EHR database of 49,226 cases by hospitalized ID and admission time. There were 18 participants who did not have sufficient data to calculate the eFI. Finally, there were 685 older adult inpatients who had both the CGA-FI and eFI assessed. The CGA-FI was the result of the sum of all 48 variables' scores divided by 48. The threshold of 0.25 as the cut-off value of CGA-FI for identifying frailty. The details of all variables in CGA-FI are described in Supplementary Table 1.
Composition of the Electronic Frailty Index (eFI)
As shown in Supplementary Table 2, according to the core criteria of the Rockwood frailty index (26), we modeled our adapted eFI referring to the factors included in the eFI of Pajewski et al. (23). Forty-five variables were selected to construct the eFI, which included 20 items on the diagnosis of chronic diseases, 20 items on nursing assessments, and 5 items on laboratory tests.
These 20 items on the diagnosis of chronic diseases covered a range of systems, included hypertension, heart failure, myocardial infarction, atrial fibrillation/atrial flutter, peripheral arterial disease, venous thromboembolism, chronic lung disease, peptic ulcer, chronic kidney disease, diabetes, thyroid dysfunction, stroke, Parkinson's disease/parkinsonism, dementia, anxiety, depression, osteoporosis, arthritis, spondylosis/disc disorders, and malignancy. The international Classification of Diseases-tenth version (ICD-10) codes for each diagnosis were determined by three clinicians after discussion and according to the global standard for diagnostic health information from the World Health Organization website (https://icd.who.int/browse10/2010/en#/) (Supplementary Table 3).
These 20 items from the nursing assessments on the day of admission included the Barthel Index (containing 10 items: feeding, bathing, grooming, dressing, bowels, bladder, toilet use, transfers, mobility) (27), visual impairment, hearing impairment, insomnia, consciousness statement, constipation, appetite, pressure ulcer, BMI, heart rate, and blood pressure. The detailed cut-off values are shown in Supplementary Table 2.
Given the importance of laboratory tests for frailty assessment (28, 29), we included 5 routine laboratory items for constructing the eFI. Based on our previous study (21), we found that hemoglobin, albumin, sodium, and D-dimer were independently associated with frailty. Meanwhile, qualitative analysis of urine protein was widely tested and was useful to reflect renal function. Therefore, we selected these abovementioned 5 items from the routine laboratory tests. Usually, the blood and urine specimens were tested within 24 h after admission for inpatients. If a patient had more than one result for the same item, we chose the first result to construct the eFI. The detailed cut-off values are shown in Supplementary Table 2.
The eFI included a total of 45 factors and was calculated as the unweighted sum of the score for each factor, divided by the total number of non-missing items. There were no missing values regarding the diagnosis for all inpatients. Moreover, we did not want an absence of the Barthel Index, which contained 10 items and necessarily implied functional status. Therefore, these abovementioned 30 items in the eFI were guaranteed. We additionally excluded individuals who did not have at least 6 of the 10 items on the nursing assessments (except Barthel Index) or at least 3 of the 5 items on the laboratory measurements. Finally, we required ≥39 non-missing items, which were consistent with recommendations for constructing the FI (26). The missing data elements from the routine EHR in calculating the eFI are described in detail in Supplementary Table 4. The upper tertile of the eFI scores (eFI ≥ 0.15) was defined as the cut-off value to indicate frailty.
Hospitalized Events and Costs
We defined hospitalized events as long hospital stay (>14 days) and death in hospital. Hospitalized costs included total costs, examination costs, treatment costs, nursing costs, pharmacy costs, and material costs. The total cost was the sum of all payments to the hospital for this hospitalization. Examination costs meant the payment for all examinations, such as laboratory measurements and imaging examinations. Treatment costs referred to payments for treatments, mainly by doctors and therapists, such as operation fees and consultation fees. Nursing costs referred to payments for all nursing care. Pharmacy costs referred to payments for all medications used during this hospitalization. Material costs referred to the payment for all medical consumable materials, of which surgical and interventional consumables accounted for a large proportion. We calculated hospitalized costs in United States dollars using the average exchange rate from 2013 to 2019 (1 USD = 6.46 CNY) for international comparisons.
Statistical Analysis
Descriptive statistics were compared between the groups using chi-square tests for categorical variables and t-tests or Mann-Whitney U for continuous variables. Pearson's correlation coefficient was used to describe the association between the continuous versions of the eFI and the CGA-FI. Additionally, a receiver operating characteristic (ROC) curve was calculated to estimate the area under the curve (AUC) for the eFI in relation to frailty, which was defined as CGA-FI≥0.25. We examined the association between frailty (eFI≥0.15) and hospitalized events using multivariate logistic regression, adjusting for age, sex, and operation [any surgery or intervention treatment in this hospitalization). Considering the influence of departments on hospitalization events, we divided 30 clinical departments into five groups (1) cardiology department; (2) internal medicine departments except cardiology; (3) orthopedics department; (4) surgical departments except orthopedics; (5) emergency department and intensive care units (ICUs)] and assessed the prevalence of frailty in different departments. We conducted a separate analysis for each subgroup. The results of the logistic regression models are presented as odds ratios (ORs) and 95% confidence intervals (95% CIs).
To investigate the impact of the eFI on hospitalized costs, generalized linear regression models (gamma-distributed and log-linked) adjusted for age, sex, and operation were used for all patients and each subgroup. For patients with two or more hospitalizations in the records, we chose the last hospitalization for analysis. The eFI values were multiplied by 10 to give equal 0.1 increments in the generalized linear regression models. The use of a gamma-distributed generalized linear model with a log-transformed link function has been shown to be a good method to estimate health-care cost distributions that are generally right-skewed (30). The results of the generalized linear regression models were presented as the β and 95% CIs for eFI values (per 0.1). A P < 0.05 was considered statistically significant.
We performed sensitivity analyses to assess the robustness of the eFI as an independent associated factor of hospitalized events and costs. For patients with two or more hospitalizations (17,212 out of 51,824 patients, 33.2%), we used the first hospitalization records instead of the last hospitalization records. We also excluded 5% patients with extremely short hospital stay (3.2%, 1,0656/51,824) or extremely long hospital stay (1.8%, 958/51,824). According to the method of constructing the eFI, 87.6% (43,116/49,210) of patients had sufficient data to calculate the eFI. For comparison with the original results, we still used 0.15 as the cut-off point of the eFI to identify frailty. Other statistical methods in the sensitivity analysis were the same as in the original analysis.
All analyzes were performed using the R software program, version 3.5.3.
Results
Demographic and Clinical Characteristics
During the study period, 49,226 older adult inpatients from the EHR database were included (Figure 1). There were 50.5% males and the average age was 74.8 ± 6.9 years old. According to the method of constructing the eFI, there were 42,821 (87.0%) patients with sufficient data to calculate the eFI and 6,405 (13.0%) patients with insufficient data. The two groups had similar ages (74.8 ± 7.0 vs. 75.1 ± 6.7 years) and proportions of males (50.5 vs. 50.7%). However, the hospital days (9 [6, 14] vs. 4 [3, 7] days; P < 0.001) and death in hospital (3.4 vs. 0.7%; P < 0.001) were significantly different in these two groups. Moreover, the hospitalized costs in patients with insufficient data were significantly lower than those in the other group (all P < 0.01). Detailed information on the entire population is shown in Table 1. The departments of ophthalmology (54.5%, 3491/6405), general surgery (20.0%, 1284/6405), and traditional Chinese medicine (7.9%, 506/6405) were the top three leading contributors to the medical records with insufficient data (Supplementary Table 5). Among the 42,821 patients, 33.8% had an eFI≥0.15, and the median eFI was 0.111 (Table 1). Figure 2 shows the prevalence of frailty in different departments. Females showed a significantly higher proportion of frailty than males (36.4 vs. 31.2%; P < 0.001).
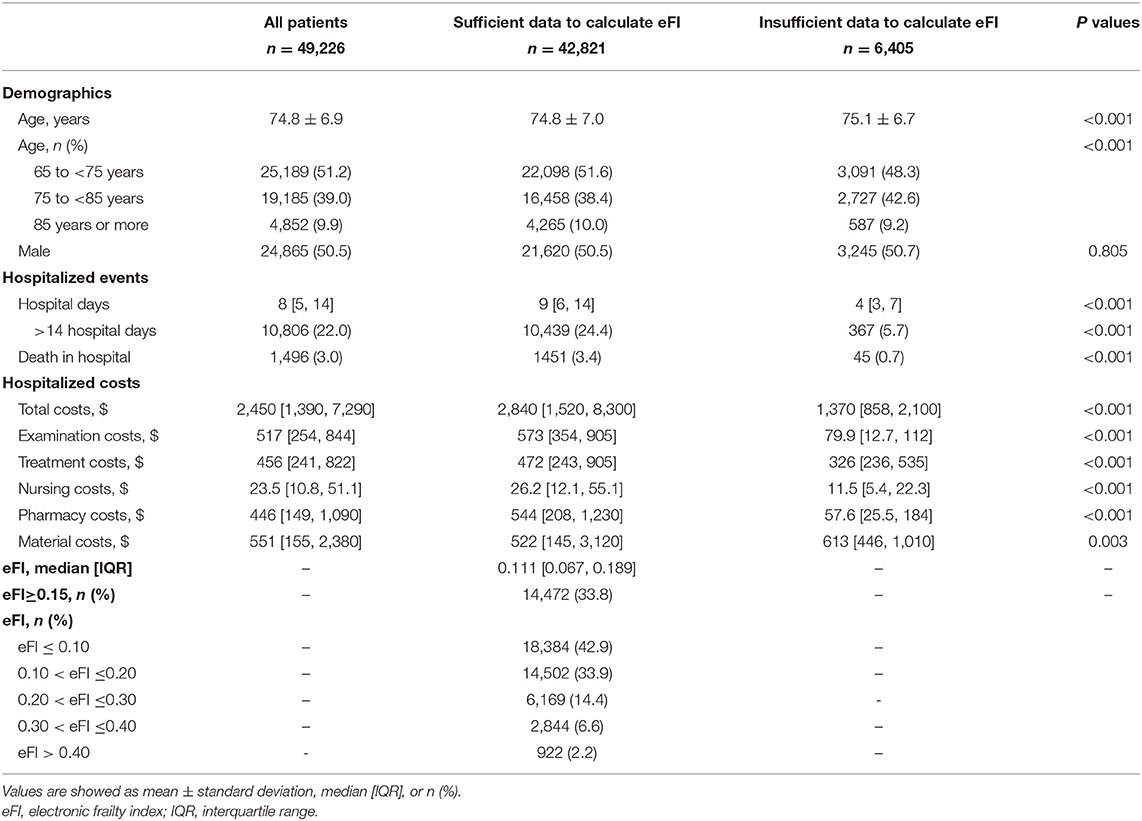
Table 1. Demographics and hospitalized events and costs of all patients stratified by whether or not the eFI could be calculated from the electronic health records.
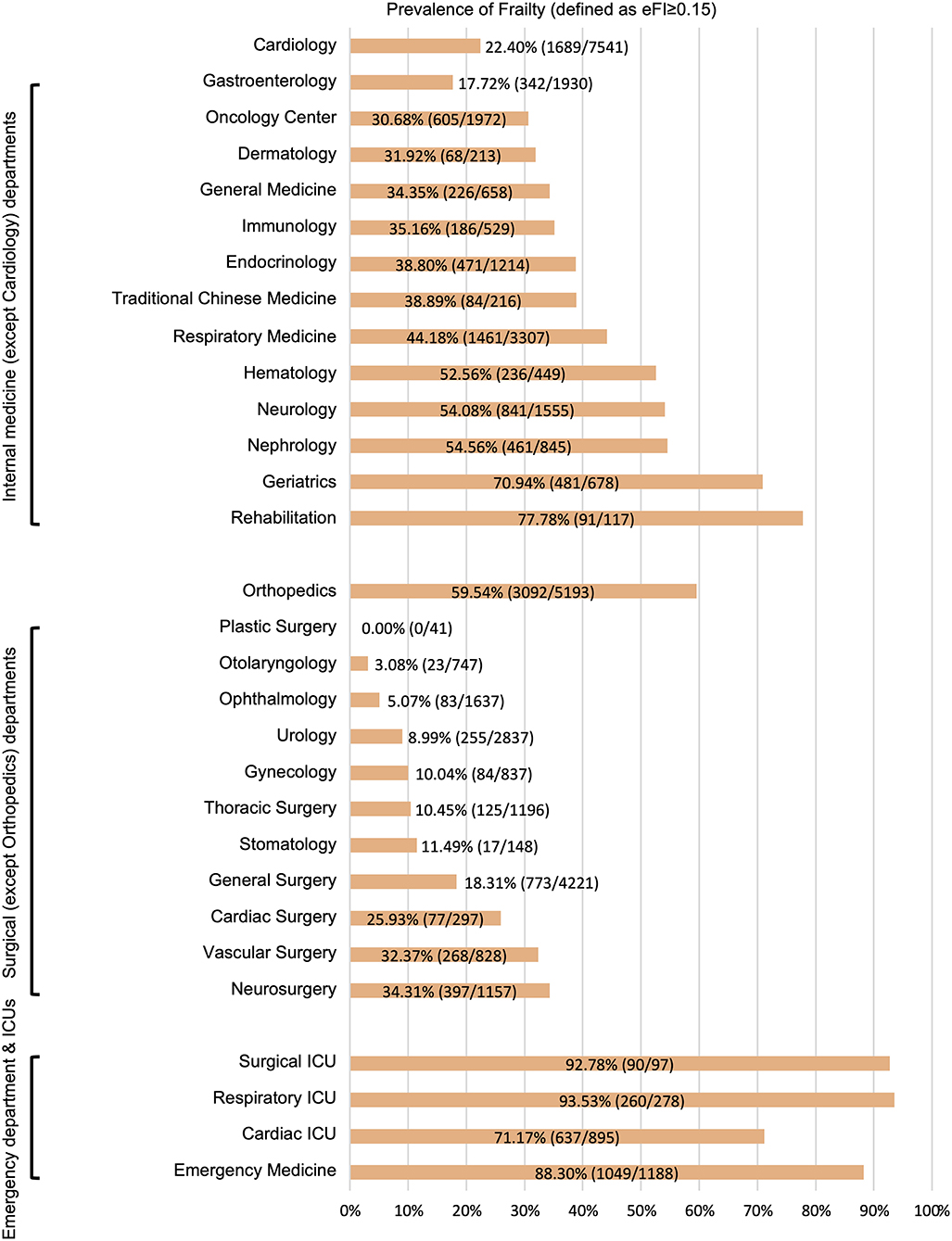
Figure 2. Prevalence of frailty in different departments. eFI, electronic frailty index; ICUs, intensive care units.
Correlation Between the eFI and CGA-FI
In the validation cohort, there were 685 participants who had both the eFI and CGA-FI assessed. The average age was 74.6 ± 6.7 years old, and 48.0% were males. The prevalence of frailty, defined as a CGA-FI ≥ 0.25, was 31.5%. The median CGA-FI was 0.198, while the median eFI was 0.102. Detailed information on all subjects is shown in Supplementary Table 6. A strong correlation is shown between the CGA-FI and eFI (Pearson's r = 0.716, P < 0.001) (Figure 3). The AUC of the ROC for the eFI to identify frailty in the subjects was 0.859. The sensitivity and specificity to identify frailty were 64.8 and 88.7%, respectively, for an eFI≥0.15 (Figure 4).
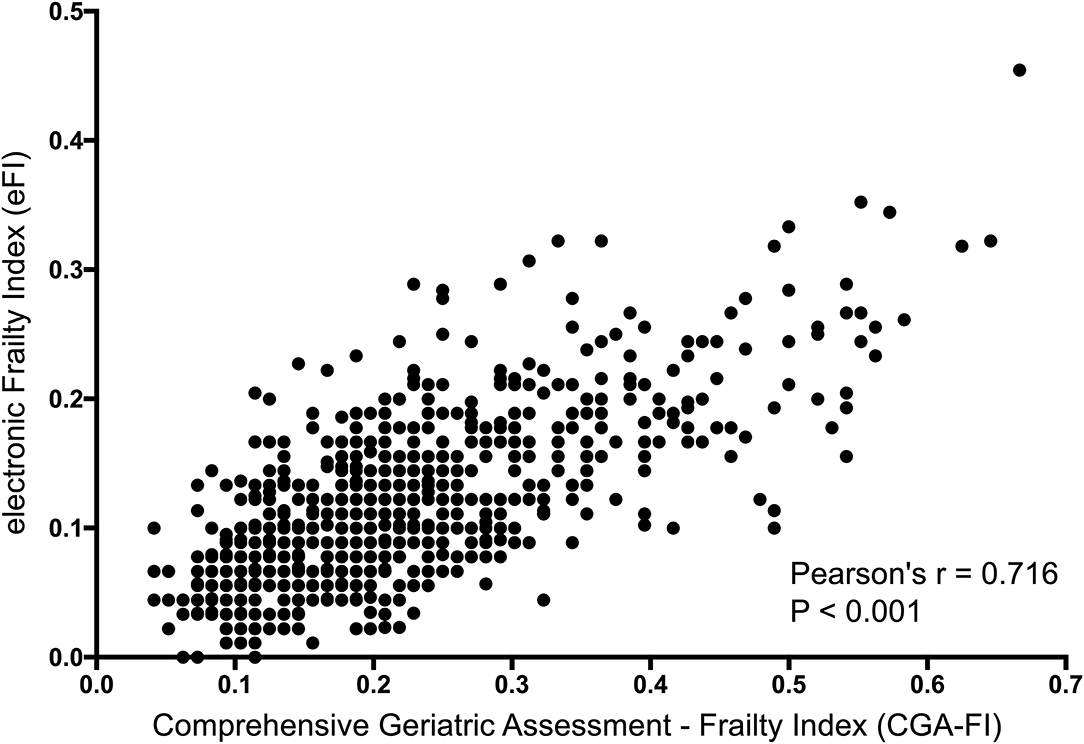
Figure 3. Correlation between the eFI and CGA-FI. eFI, electronic frailty index; CGA-FI, comprehensive geriatric assessment-frailty index.
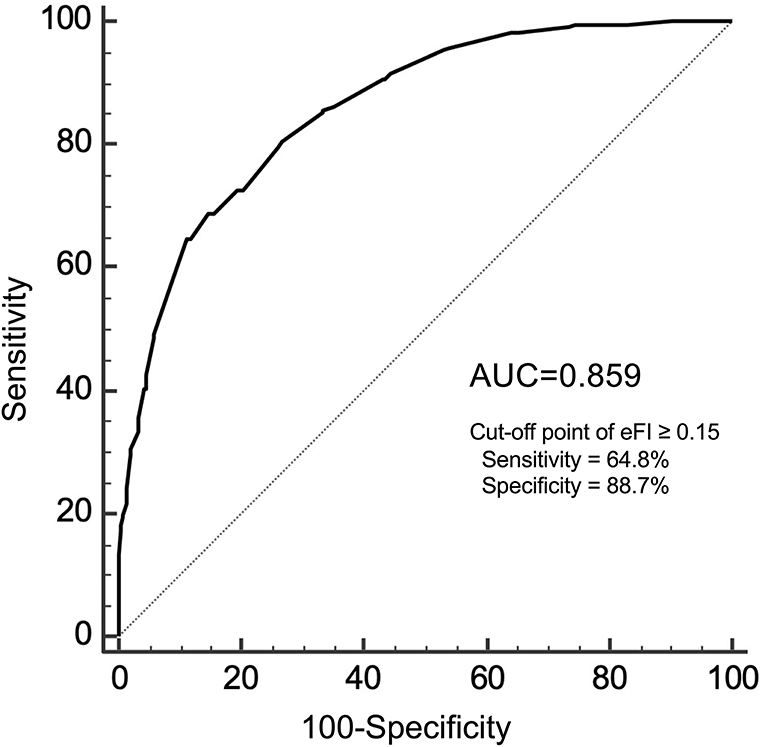
Figure 4. The ROC analysis for the eFI against CGA-FI ≥0.25 to identify frailty. ROC, receiver operating characteristic; eFI, electronic frailty index; CGA-FI, comprehensive geriatric assessment-frailty index; AUC, area under the curve.
Difference Between the Frail Group and Non-frail Group
We used 0.15 (tertile of eFI) as the cut-off value to indicate frailty in the 42,821 inpatients from the EHR database. Compared with the non-frail group, the frail group was significantly older (78.7 ± 7.1 vs. 72.8 ± 6.0 years; P < 0.001) and had a lower percentage of males (46.6 vs. 52.5%; P < 0.001). The department distributions and operations were clearly different between these two groups (both P < 0.001). The frail group had significantly longer hospital days (12 [8, 18] vs. 8 [5, 13] days, P < 0.001) and a higher mortality rate in the hospital (9.3 vs. 0.4%, P < 0.001) than the non-frail group. Meanwhile, the total hospitalized cost and each specific cost for the frail group were all significantly higher than those for the non-frail group (all P < 0.001) (Table 2).
Association of Frailty With Hospitalized Events and Costs
After adjusting for age, sex, and operation, an eFI≥0.15 showed independent correlations with long hospital stay (OR = 2.889, P < 0.001) and death in hospital (OR = 19.97, P < 0.001) for all older adult inpatients by multivariate logistic regression. In the subgroup analysis for different departments, the eFI was also independently associated with long hospital stay in all subgroups (ORs ranged from 1.392 to 4.750; all P < 0.001). The same results are presented for death in hospital (ORs ranged from 7.192 to 34.26; all P < 0.001) (Table 3).
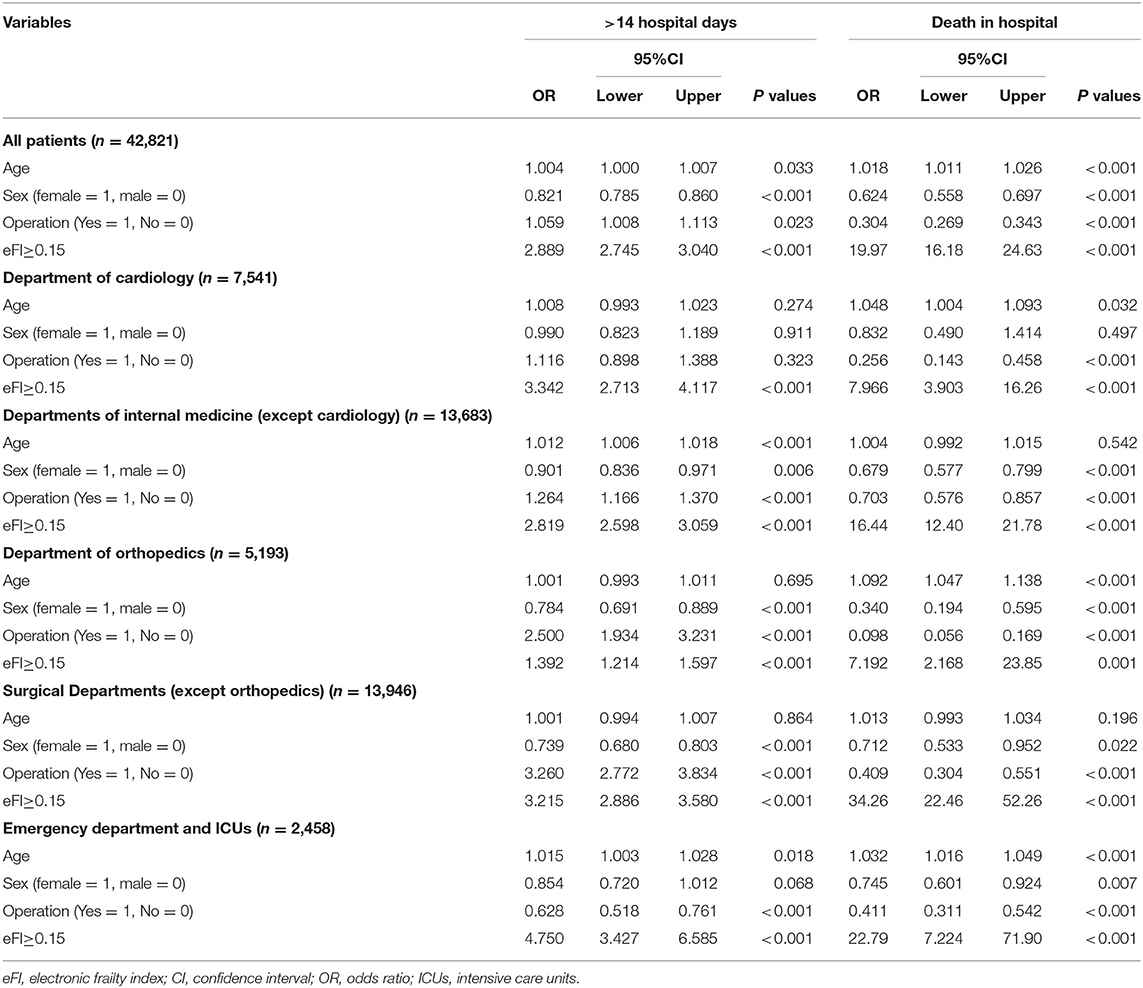
Table 3. Association between eFI and hospitalized events of elderly inpatients by logistic regression.
In this study, the hospitalized cost averaged of non-frail and frail group were US $2,290 (1,370, 6,520) and US $4,790 (2,060, 10,700), respectively (Table 1). After adjusting for age, sex, and operation, the eFI was positively associated with total costs (β = 0.453, P < 0.001), examination costs (β = 0.269, P < 0.001), treatment costs (β = 0.414, P < 0.001), nursing costs (β = 0.381, P < 0.001), pharmacy costs (β = 0.524, P < 0.001), and material costs (β = 0.578, P < 0.001) for all older adult inpatients. Subgroups of different departments showed similar correlations between the eFI and hospitalized costs (all P < 0.01) (Table 4).
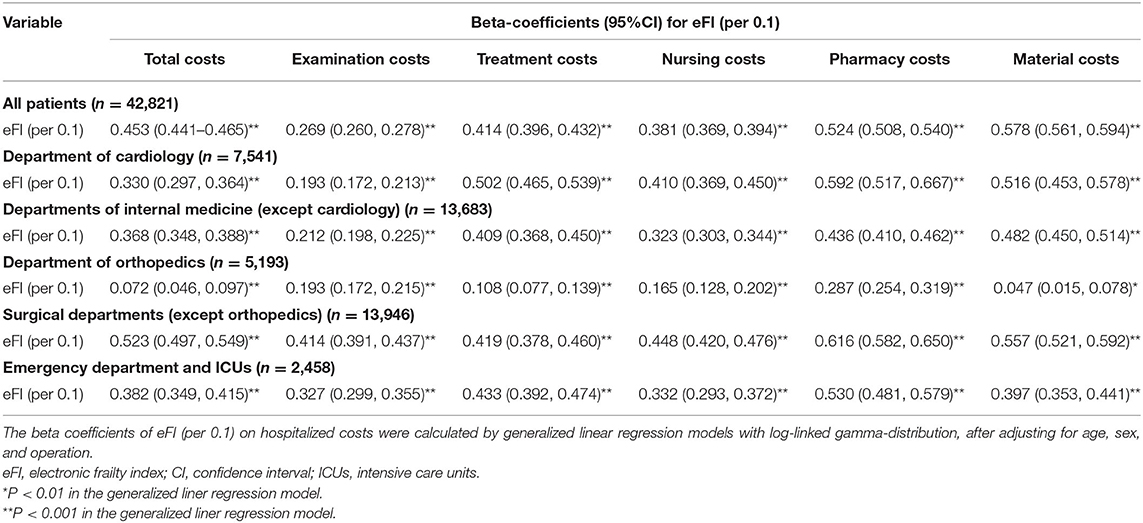
Table 4. Association between eFI and hospitalized costs of elderly inpatients by generalized liner regression models.
Sensitivity Analysis
When using the first hospitalization records instead of the last ones, the mortality in the hospital with sufficient data was 1.4%, which was lower than the 3.4% in the original data. There were 30.0% (12,934/43,116) of patients in the frail group, defined by an eFI≥0.15. All the trends between the frail group and the non-frail group were similar to those from the original analysis (Supplementary Table 7). After the sensitivity analysis, eFI≥0.15 remained an independent risk factor for long hospital stay and death in hospital (Supplementary Table 8). Moreover, the eFI was still independently associated with all kinds of increased hospitalized costs (Supplementary Table 9).
Discussion
To our knowledge, this is the first study on detecting frailty in older adult inpatients by routine EHR in China. Our study demonstrates that an effective eFI by routine EHR for older adult inpatients can be developed from a general hospital in China, and the eFI was validated by agreement with the CGA-FI. Although the prevalence of frailty varied obviously among different departments, frailty was an independent risk factor for hospitalized events in each subgroup of different departments. As the degree of frailty increased, the hospitalized costs for older adult inpatients increased accordingly.
Frailty Assessment by Routine EHR
In this study, we constructed an eFI to assess frailty using routine EHR. Our results showed a strong correlation between eFI and CGA-FI, which was consistent with the findings of Brundle et al. in the UK (25) and Abbasi et al. in Canada (31). Previous studies have shown that eFI could predict mid-term adverse events in older adult patients (22, 23). Our study showed that the eFI was independently associated with long hospital stay and death in hospital. Although the eFI opens a new direction to fill the gap between research and clinical application, several details should be considered in clinical practices based on our results.
First, the cut-off value of the eFI for identifying frailty should be redefined according to the specific situation, instead of taking a generally accepted fixed value, such as 0.25 for the CGA-FI. Our study showed that the eFI was lower than the CGA-FI despite both having a strong correlation. The median CGA-FI was 0.198, while the median eFI was only 0.102 in the validation cohort. Therefore, applying cut-off value from CGA-FI to the eFI will underestimate the prevalence of the frail population. A similar study that simultaneously compared their own eFI and CGA-FI also showed that eFI was relatively lower than the CGA-FI for 85 participants in a Canadian primary care program (31). There were several possible reasons why our eFI values were lower than those of the CGA-FI. Our eFI was based on routine EHR from a single hospitalization rather than a summary of medical records across multiple visits. In a single hospitalization, the diagnoses seemingly not highly-related to the current treatments, such as depression or anxiety, may be ignored, especially in surgical departments. Moreover, the nurses might not pay enough attention to the conditions related to frailty during the admission assessment, which might have led to the neglect of some positive manifestations. Given that the frailty prevalence was 35.1% by the CGA-FI in our previous survey for older adult inpatients (21), it was more reasonable to use the tertile of eFI values (0.15) as the cut-off value for identifying frailty. Perhaps each center needs to define its own cut-off value for the eFI according to its own situation and research results.
Second, the timeliness of constructing the eFI should be considered in clinical practice. The routine EHR used to construct the eFI could be mainly collected within 24 h after admission in our study except for the diagnostic codes. Compared with the well-checked, ICD-10 coded discharge diagnosis, the uncoded admission diagnosis was relatively difficult to analyze. Therefore, we used the discharge diagnosis to calculate the eFI. If the admission diagnosis is improved by coding in the future, we would be able to obtain the eFI within 24 h after admission, which would be not only useful in follow-up of frail patients after discharge, but also in guiding to manage older adult inpatients in a targeted manner during hospitalization. In previous studies, the eFI was mainly developed in primary care settings (22, 23, 31). Compared with relatively complete hospitalization records, the cleaning of primary medical data is more complicated. It is difficult to obtain all information from one setting at one visit. There often needs to be 1 or 2 years of medical records to construct an eFI, which leads to a time lag in the guidance of clinical practice.
Third, the eFI should reflect the variability in frail conditions. Frailty is a dynamic condition, and individuals can fluctuate between states of severity of frailty (32). In previous studies, researchers have rarely paid attention to this point when constructing an eFI (22). This might also be due to the limitations of electronic medical data, which caused frailty assessment to rely too much on diagnostic codes (24). Pajewski et al. and Abbasi et al. improved the composition of the eFI by adding some laboratory tests and functional abilities (23, 31). We also agree with this improvement. Therefore, we added a 20-item set of nursing assessments and a 5-item set of laboratory tests to the factors of the eFI. A dynamic eFI during hospitalization can more effectively reflect the current condition of older adult inpatients, which would be more precisely guide clinical interventions.
The primary advantage of EHR data is its large volume, but the biggest challenge of EHR-based studies is the presence of missing data (33). Our study showed that 13.0% of patients had insufficient data to calculate the eFI, while Pajewski's study reported 29.6% (23), others did not report the details. We found that these 13% of patients had a significantly lower proportion of adverse events, which was consistent with the results of Pajewski's study (23). Our study showed that the department of ophthalmology contributed the majority of these patients (54.5%, 3491/6405), while the prevalence of frailty was only 5.07% in the department of ophthalmology. These results indicate that patients with insufficient data had a lower percentage of frailty. Therefore, the eFI can be applied to the vast majority of patients with a need for frailty assessment. Meanwhile, we should consider the tolerance for missing data when we select the factors for constructing an eFI.
Prevalence of Frailty Among Different Departments
We found that there were significant differences in the prevalence of frailty among the different departments. Generally, emergency departments and ICUs had the highest percentage of frailty (average: 82.8%), followed by internal medicine departments (average: 34.1%), and surgical departments (average: 27.1%). This trend was consistent with the results of a recent multicenter survey on frailty in older adult inpatients in China (34).
In this study, we found that the prevalence of frailty in the department of orthopedics (59.5%) was significantly higher than that in other surgical departments and most internal medicine departments. This was mainly because the conditions for most orthopedic patients were braked by osteoarthritis or fractures, especially hip fractures. The activities of daily living assessments for these inpatients in this state could not reflect their daily conditions. We should keep this point in mind when we use the eFI to guide clinical practice for inpatients in department of orthopedics. In our study, the prevalence of frailty in department of cardiology was lower than that in most internal medicine departments. This result was consistent with results in our previous cohort study (21). Since non-emergency percutaneous coronary intervention is not an outpatient procedure in China, it is easy to be explained. However, we were surprised to find that the prevalence of frailty in the gastroenterology ward (17.7%) was the lowest among all internal medicine departments. This might have been caused by the large amount of endoscopic procedures that lead to the development of gastroenterology near surgical departments. For hospitals that do not overly rely on endoscopic procedures, the prevalence of frailty in the gastroenterology ward may be closer to the average level among internal medical departments. In short, due to the differences in disease characteristics and treatment models, the prevalence of frailty varied significantly among different departments.
Association Between Frailty and Hospitalized Costs
Our findings showed that the hospitalized cost of patients with frailty was significantly higher than that patients without frailty. The eFI was positively associated with costs after adjusting age, sex and operation. This trend exists in all kinds of hospitalized costs (examination, treatment, nursing, pharmacy and material costs).
Previous studies has also suggested that frailty was associated with healthcare expenditure, both in developed and developing countries (5, 8–11, 15, 16). Several small-sample studies have shown that frailty was associated with increased hospitalized costs for patients with colorectal surgery (6), cardiac surgery (13), or transcatheter aortic value implantation (35). In china, studies also showed similar results in annual healthcare costs (12, 14). However, one study showed that there was no significant difference in hospitalized costs between frail and non-frail groups with elective non-cardiac surgeries (36), which is contrary to our results. These inconsistencies might have been due to the small sample size.
To our knowledge, previous studies did not simultaneously analyze the correlation between hospitalized costs and frailty in different departments. Our results showed that the increases in hospitalized costs in the department of orthopedics were smaller than those in other subgroups, especially in terms of material costs. There are several possible reasons to explain this phenomenon. Firstly, the cost of medical consumable materials is a large part of the total hospitalized cost in the department of orthopedics, whether for frail or non-frail inpatients. Secondly, the cost of medical consumable materials in China depends not only on the patient's condition but also on the patient's own choice. For example, patients with low income or without medical insurance may be more likely to choose the less expensive of similar products. Thirdly, the frailty assessment was influenced by patient braking, which may have led to an overestimation of the degree of frailty in many patients with fracture. However, even so, hospitalized costs are positively related to the degree of frailty for older adult inpatients in the department of orthopedics, after adjusting for age, sex, and operation.
Overall, considering that in-hospital interventions on frailty have been effective for older adult inpatients to reverse the degree of frailty (37), timely recognition of frailty is important to guide interventions on frailty and even reduce hospitalized costs to some degree for older adult inpatients.
Limitations
There are three main limitations in this study. First, there was no follow-up data after discharge. We could not verify the long-term predictive value of the eFI for adverse events in the older adult inpatients because it is difficult to link our hospital EHR with national resident death records or EHR from other hospitals. Second, we used discharge but not admit diagnoses when building eFI. In our hospital, discharge diagnoses were inspected and coded in a variation of ICD-10 by specialists, which are the best source we can find representing the disease burden of patients at present. One of our targets in the future research is to build eFI based on the information gathered in first 24 h after admission, and to verify the value in prediction of outcomes. Third, despite its relatively large size, the EHR data in this study were from a single general hospital, which may not represent all hospitals in China. Multicenter studies with follow-up data are warranted in the future.
Conclusion
In summary, we successfully developed an effective eFI using routine EHR for older adult inpatients from a general hospital in China. With good agreement with the CGA-FI, the eFI can be a useful and convenient tool to clinicians for detecting frailty in older adult inpatients. The prevalence of frailty varied obviously among different departments, but frailty was an independent risk factor for long hospital stay and death in hospital. As the degree of frailty increased, the hospitalized costs for older adult inpatients increased accordingly.
Data Availability Statement
The raw data supporting the conclusions of this article will be made available by the authors, without undue reservation.
Ethics Statement
The studies involving human participants were reviewed and approved by the Ethics Committee of Beijing Hospital (No. 2019BJYYEC-131-01). The Ethics Committee waived the requirement of written informed consent for participation.
Author Contributions
Y-DL, JS, J-FY, and HW contributed conception and design of the study. Y-BX performed data collection, preparation, and cleansing. Y-DL and M-HD performed the statistical analysis and wrote the draft of the manuscript. All authors contributed to the article and approved the submitted version.
Funding
This work was supported by the Non-profit Central Research Institute Fund of Chinese Academy of Medical Sciences, China (No. 2019PT320013); the Beijing Municipal Science and Technology Commission, China (D181100000218003); and the CAMS Innovation Fund for Medical Sciences (No. 2018-I2M-1-002).
Conflict of Interest
The authors declare that the research was conducted in the absence of any commercial or financial relationships that could be construed as a potential conflict of interest.
Publisher's Note
All claims expressed in this article are solely those of the authors and do not necessarily represent those of their affiliated organizations, or those of the publisher, the editors and the reviewers. Any product that may be evaluated in this article, or claim that may be made by its manufacturer, is not guaranteed or endorsed by the publisher.
Acknowledgments
We sincerely appreciate Ms. Jing Zhang (1) Bodhi Lab., Beijing BeYes Technology Co. Ltd, Beijing, China; (2) Department of Biomedical Engineering, Tsinghua University, China) and Mr. Xiao-Da Lei (1. Bodhi Lab., Beijing BeYes Technology Co. Ltd, Beijing, China; 2. College of Software, Beihang University, China) for their professional support with data cleaning. Y-DL wants to give her sincere thanks to Mr. Rui Ma, Mr. Yi-Chao Li, and Ms. Qian-Rui Li for their friendly, genuine, and unpaid support for this work as her good friends.
Supplementary Material
The Supplementary Material for this article can be found online at: https://www.frontiersin.org/articles/10.3389/fmed.2021.731445/full#supplementary-material
References
1. Beard JR, Officer A, de Carvalho IA, Sadana R, Pot AM, Michel J-P, et al. The World report on ageing and health: a policy framework for healthy ageing. Lancet Lond Engl. (2016) 387:2145–54. doi: 10.1016/S0140-6736(15)00516-4
2. National Bureau of Statistics of China. Age Composition and Dependency Ratio of Population. Available online at: https://data.stats.gov.cn/english/easyquery.htm?cn=C01 (accessed December 31, 2020).
3. Clegg A, Young J, Iliffe S, Rikkert MO, Rockwood K. Frailty in elderly people. Lancet Lond Engl. (2013) 381:752–62. doi: 10.1016/S0140-6736(12)62167-9
4. Huang S-T, Tange C, Otsuka R, Nishita Y, Peng L-N, Hsiao F-Y, et al. Subtypes of physical frailty and their long-term outcomes: a longitudinal cohort study. J Cachexia Sarcopenia Muscle. (2020) 11:1223–31. doi: 10.1002/jcsm.12577
5. Ensrud KE, Kats AM, Schousboe JT, Taylor BC, Cawthon PM, Hillier TA, et al. Frailty Phenotype and Healthcare Costs and Utilization in Older Women. J Am Geriatr Soc. (2018) 66:1276–83. doi: 10.1111/jgs.15381
6. Robinson TN, Wu DS, Stiegmann GV, Moss M. Frailty predicts increased hospital and six-month healthcare cost following colorectal surgery in older adults. Am J Surg. (2011) 202:511–4. doi: 10.1016/j.amjsurg.2011.06.017
7. García-Nogueras I, Aranda-Reneo I, Peña-Longobardo LM, Oliva-Moreno J, Abizanda P. Use of health resources and healthcare costs associated with frailty: the FRADEA study. J Nutr Health Aging. (2017) 21:207–14. doi: 10.1007/s12603-016-0727-9
8. Peters LL, Burgerhof JGM, Boter H, Wild B, Buskens E, Slaets JPJ. Predictive validity of a frailty measure. (GFI) and a case complexity measure (IM-E-SA) on healthcare costs in an elderly population. J Psychosom Res. (2015) 79:404–11. doi: 10.1016/j.jpsychores.2015.09.015
9. Sirven N, Rapp T. The cost of frailty in France. Eur J Health Econ HEPAC Health Econ Prev Care. (2017) 18:243–53. doi: 10.1007/s10198-016-0772-7
10. Hajek A, Bock J-O, Saum K-U, Matschinger H, Brenner H, Holleczek B, et al. Frailty and healthcare costs-longitudinal results of a prospective cohort study. Age Ageing. (2018) 47:233–41. doi: 10.1093/ageing/afx157
11. Simpson KN, Seamon BA, Hand BN, Roldan CO, Taber DJ, Moran WP, et al. Effect of frailty on resource use and cost for Medicare patients. J Comp Eff Res. (2018) 7:817–25. doi: 10.2217/cer-2018-0029
12. Jin H-Y, Liu X, Xue Q-L, Chen S, Wu C. The Association between frailty and healthcare expenditure among Chinese older adults. J Am Med Dir Assoc. (2020) 21:780–5. doi: 10.1016/j.jamda.2020.03.008
13. Goldfarb M, Bendayan M, Rudski LG, Morin J-F, Langlois Y, Ma F, et al. Cost of cardiac surgery in frail compared with nonfrail older adults. Can J Cardiol. (2017) 33:1020–6. doi: 10.1016/j.cjca.2017.03.019
14. Gao K, Li B-L, Yang L, Zhou D, Ding K-X, Yan J, et al. Cardiometabolic diseases, frailty, and healthcare utilization and expenditure in community-dwelling Chinese older adults. Sci Rep. (2021) 11:7776. doi: 10.1038/s41598-021-87444-z
15. Kim MJ, Jang SY, Cheong H-K, Oh I-H. Association of frailty with healthcare costs using claims data in korean older adults aged 66. J Nutr Health Aging. (2021) 25:653–9. doi: 10.1007/s12603-021-1612-8
16. Ambagtsheer RC, Moussa RK. Association of frailty with health service utilisation and health care expenditure in sub-Saharan Africa: evidence from Côte d'Ivoire. BMC Geriatr. (2021) 21:446. doi: 10.1186/s12877-021-02377-6
17. Rockwood K, Howlett SE. Fifteen years of progress in understanding frailty and health in aging. BMC Med. (2018) 16:220. doi: 10.1186/s12916-018-1223-3
18. Choi J-Y, Kim K-I, Choi Y, Ahn S-H, Kang E, Oh H-K, et al. Comparison of multidimensional frailty score, grip strength, and gait speed in older surgical patients. J Cachexia Sarcopenia Muscle. (2020) 11:432–40. doi: 10.1002/jcsm.12509
19. Fried LP, Tangen CM, Walston J, Newman AB, Hirsch C, Gottdiener J, et al. Frailty in older adults: evidence for a phenotype. J Gerontol A Biol Sci Med Sci. (2001) 56:M146–56. doi: 10.1093/gerona/56.3.M146
20. Jones DM, Song X, Rockwood K. Operationalizing a frailty index from a standardized comprehensive geriatric assessment. J Am Geriatr Soc. (2004) 52:1929–33. doi: 10.1111/j.1532-5415.2004.52521.x
21. Liang Y-D, Zhang Y-N, Li Y-M, Chen Y-H, Xu J-Y, Liu M, et al. Identification of Frailty and its risk factors in elderly hospitalized patients from different wards: a cross-sectional study in China. Clin Interv Aging. (2019) 14:2249–59. doi: 10.2147/CIA.S225149
22. Clegg A, Bates C, Young J, Ryan R, Nichols L, Ann Teale E, et al. Development and validation of an electronic frailty index using routine primary care electronic health record data. Age Ageing. (2016) 45:353–60. doi: 10.1093/ageing/afw039
23. Pajewski NM, Lenoir K, Wells BJ, Williamson JD, Callahan KE. Frailty screening using the electronic health record within a medicare accountable care organization. J Gerontol A Biol Sci Med Sci. (2019) 74:1771–7. doi: 10.1093/gerona/glz017
24. Gilbert T, Neuburger J, Kraindler J, Keeble E, Smith P, Ariti C, et al. Development and validation of a Hospital Frailty Risk Score focusing on older people in acute care settings using electronic hospital records: an observational study. Lancet Lond Engl. (2018) 391:1775–82. doi: 10.1016/S0140-6736(18)30668-8
25. Brundle C, Heaven A, Brown L, Teale E, Young J, West R, et al. Convergent validity of the electronic frailty index. Age Ageing. (2019) 48:152–6. doi: 10.1093/ageing/afy162
26. Searle SD, Mitnitski A, Gahbauer EA, Gill TM, Rockwood K. A standard procedure for creating a frailty index. BMC Geriatr. (2008) 8:24. doi: 10.1186/1471-2318-8-24
27. Mahoney FI, Barthel DW. Functional evaluation: the Barthel index. Md State Med J. (1965) 14:61–5. doi: 10.1037/t02366-000
28. Howlett SE, Rockwood MRH, Mitnitski A, Rockwood K. Standard laboratory tests to identify older adults at increased risk of death. BMC Med. (2014) 12:171. doi: 10.1186/s12916-014-0171-9
29. Mitnitski A, Collerton J, Martin-Ruiz C, Jagger C, von Zglinicki T, Rockwood K, et al. Age-related frailty and its association with biological markers of ageing. BMC Med. (2015) 13:161. doi: 10.1186/s12916-015-0400-x
30. Manning WG, Basu A, Mullahy J. Generalized modeling approaches to risk adjustment of skewed outcomes data. J Health Econ. (2005) 24:465–88. doi: 10.1016/j.jhealeco.2004.09.011
31. Abbasi M, Khera S, Dabravolskaj J, Vandermeer B, Theou O, Rolfson D, et al. A cross-sectional study examining convergent validity of a frailty index based on electronic medical records in a Canadian primary care program. BMC Geriatr. (2019) 19:109. doi: 10.1186/s12877-019-1119-x
32. Hoogendijk EO, Afilalo J, Ensrud KE, Kowal P, Onder G, Fried LP. Frailty: implications for clinical practice and public health. Lancet Lond Engl. (2019) 394:1365–75. doi: 10.1016/S0140-6736(19)31786-6
33. Goldstein BA, Navar AM, Pencina MJ, Ioannidis JPA. Opportunities and challenges in developing risk prediction models with electronic health records data: a systematic review. J Am Med Inform Assoc JAMIA. (2017) 24:198–208. doi: 10.1093/jamia/ocw042
34. Jiao J, Wang Y, Zhu C, Li F, Zhu M, Wen X, et al. Prevalence and associated factors for frailty among elder patients in China: a multicentre cross-sectional study. BMC Geriatr. (2020) 20:100. doi: 10.1186/s12877-020-1496-1
35. Patel JN, Ahmad M, Kim M, Banga S, Asche C, Barzallo M, et al. Relation of frailty to cost for patients undergoing transcatheter aortic valve implantation. Am J Cardiol. (2020) 125:469–74. doi: 10.1016/j.amjcard.2019.10.021
36. Zhu L-Y, Ji M-H, Zhang Y-F, Xia J-Y, Yang J-J. Frailty and short-term outcomes in elderly patients following elective noncardiac surgeries: a prospective cohort study. J Clin Anesth. (2020) 64:109820. doi: 10.1016/j.jclinane.2020.109820
37. Valenzuela PL, Morales JS, Castillo-García A, Mayordomo-Cava J, García-Hermoso A, Izquierdo M, et al. Effects of exercise interventions on the functional status of acutely hospitalised older adults: a systematic review and meta-analysis. Ageing Res Rev. (2020) 61:101076. doi: 10.1016/j.arr.2020.101076
Keywords: frailty, electronic health records-EHR, long hospital stay, mortality, hospitalized costs
Citation: Liang Y-D, Xie Y-B, Du M-H, Shi J, Yang J-F and Wang H (2021) Development and Validation of an Electronic Frailty Index Using Routine Electronic Health Records: An Observational Study From a General Hospital in China. Front. Med. 8:731445. doi: 10.3389/fmed.2021.731445
Received: 27 June 2021; Accepted: 02 September 2021;
Published: 28 September 2021.
Edited by:
Ming Yang, Sichuan University, ChinaReviewed by:
Lina Ma, Capital Medical University, ChinaSiti Setiati, University of Indonesia, Indonesia
Mario Ulises Pérez-Zepeda, Dalhousie University, Canada
Copyright © 2021 Liang, Xie, Du, Shi, Yang and Wang. This is an open-access article distributed under the terms of the Creative Commons Attribution License (CC BY). The use, distribution or reproduction in other forums is permitted, provided the original author(s) and the copyright owner(s) are credited and that the original publication in this journal is cited, in accordance with accepted academic practice. No use, distribution or reproduction is permitted which does not comply with these terms.
*Correspondence: Hua Wang, wh74220@aliyun.com; Jie-Fu Yang, yangjiefu2011@126.com
†These authors have contributed equally to this work and share first authorship