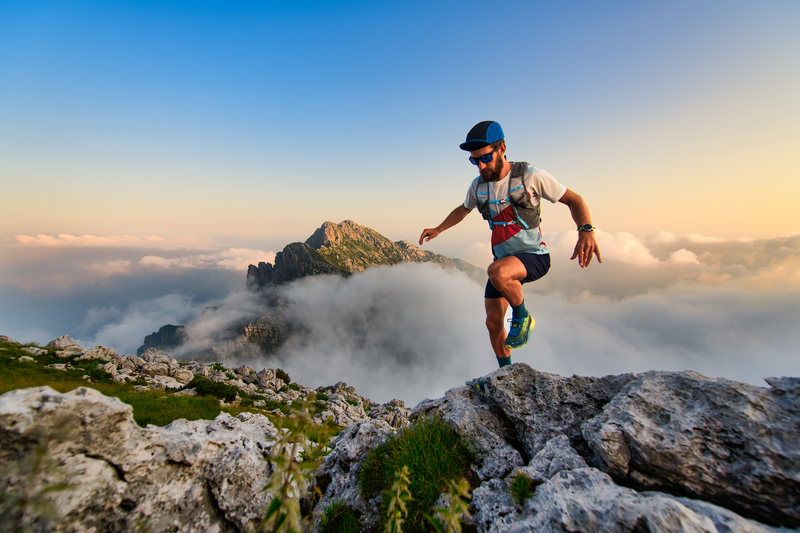
95% of researchers rate our articles as excellent or good
Learn more about the work of our research integrity team to safeguard the quality of each article we publish.
Find out more
SYSTEMATIC REVIEW article
Front. Med. , 14 January 2022
Sec. Pulmonary Medicine
Volume 8 - 2021 | https://doi.org/10.3389/fmed.2021.723635
Objective: Lung microbiota is increasingly implicated in multiple types of respiratory diseases. However, no study has drawn a consistent conclusion regarding the relationship between changes in the microbial community and lung diseases. This study verifies the association between microbiota level and lung diseases by performing a meta-analysis.
Methods: Literature databases, including PubMed, ISI Web of Science, Embase, Google Scholar, PMC, and CNKI, were used to collect related articles published before March 20, 2021. The standard mean deviation (SMD) and related 95% confidence intervals (CIs) were calculated using a random-effects model. Subgroup, sensitivity, and publication bias analyses were also conducted.
Results: Six studies, comprising 695 patients with lung diseases and 176 healthy individuals, were included in this meta-analysis. The results indicated that the microbiota level was higher in patients with lung diseases than in healthy individuals (SMD = 0.39, 95% CI = 0.22–0.55, I2 = 91.5%, P < 0.01). Subgroup analysis based on country demonstrated that the microbiota level was significantly higher in Chinese (SMD = 1.90, 95% CI = 0.87–2.93, I2 = 62.3%, P < 0.01) and Korean (SMD = 0.24, 95% CI = 0.13–0.35, I2 = 78.7%, P < 0.01) patients with lung diseases. The microbiota level of patients with idiopathic pulmonary fibrosis (IPF) (SMD = 1.40, 95% CI = 0.42–2.38, I2 = 97.3%, P = 0.005), chronic obstructive pulmonary disease (COPD) (SMD = 0.30, 95% CI = 0.09–0.50, I2 = 83.9%, P = 0.004), and asthma (SMD = 0.19, 95% CI = 0.06–0.32, I2 = 69.4%, P = 0.004) were significantly higher than those of the healthy group, whereas a lower microbiota level was found in patients with chronic hypersensitivity pneumonitis (CHP). The microbiota level significantly increased when the disease sample size was >50. Subgroup analysis based on different microbiota genera, indicated that Acinetobacter baumannii and Pseudomonas aeruginosa were significantly increased in COPD and asthma diseases.
Conclusion: We observed that patients with IPF, COPD, and asthma had a higher microbiota level, whereas patients with CHP had a lower microbiota level compared to the healthy individuals. The level of A. baumannii and P. aeruginosa were significantly higher in patients with COPD and asthma, and thus represented as potential microbiota markers in the diagnosis and treatment of lung diseases.
An abundance of microbiota lives both inside and outside the human body. Investigation of the microbiota has contributed dramatically to our understanding of their critical role in multiple human diseases, such as cancers, infectious diseases, and lung diseases (1–4). The “Human Microbiome Project” was launched in 2007 to understand the complexity of the human microbiome, which has also contributed to the understanding of pathogenesis in a wide range of human diseases (5). The human microbiota comprises bacteria, archaea, viruses, and eukaryotes. These microbiota communities affect human physiological functions, both in health and disease status, contributing to the enhancement or impairment of metabolic and immune functions (6). Alterations in the microbiota level and composition may lead to an ecological imbalance by decreasing the number of symbionts and increasing potentially dangerous pathogens (7).
Lungs are vital organs for gaseous exchange during respiration. For many years, the lungs have been thought to be a sterile environment (8). With advancements in molecular and biochemical techniques, many studies have reported that microbial imbalance, known as dysbiosis, contributes to the occurrence, development, and deterioration of lung diseases (9). A previous meta-analysis identified several taxa annotated for Rummeliibacillus sp. species, Deinococcus, Kurthia, Brevibacillus borstelensis, Caulobacter sp., Actinomyces graevenitzii, Rhodotorula mucilaginosa, and Mycobacterium tuberculosis, which were significantly abundant in tuberculosis (TB) cases. Other taxa, including Clostridiales, Tumebacillus ginsengisoli, Pelomonas aquatica, Propionibacterium acnes, and Haemophilus parahaemolyticus, were lower in patients with TB than in healthy individuals (10). However, since 2010, studies have also described seeing alterations of microbiota in other lung diseases, such as chronic obstructive pulmonary disease (COPD), idiopathic pulmonary fibrosis (IPF), and asthma indicating that the lung microbiota influenced both healthy and diseased subjects (11–13). COPD is the third leading cause of death worldwide (14). Whereas, IPF is a progressive lung disease that can lead to rapid death, and has an average survival rate of 2–3 years following diagnosis, mainly in older adults (15, 16). Although some studies had demonstrated a significant association between the lung microbiota and IPF, the key questions remained unanswered. Asthma kills ~1,000 people every day and affects over 330 million people worldwide (17). In addition, there is a growing interest in investigating microbiome interactions and identifying specific microbes or microbial products as potential new treatment targets (18).
Recently, pyrosequencing of the 16S ribosomal RNA (rRNA) gene amplicons from bronchoalveolar lavage specimens, bronchial brushings, and lung tissues from patients with lung diseases and healthy subjects demonstrated that various microorganisms were abnormally altered at the molecular level (19–23). However, assessment of the lung microbiota in the pathogenesis of these diseases has not yet been wholly and systematically conducted. Moreover, it is difficult to characterize the bacterial community in multiple lung diseases using conventional culture methods. There is also no scientific evidence or a clear consensus regarding this association from previous studies. Meta-analysis has the advantage of reducing errors by pooling a large amount of available data and providing more precise estimates. The purpose of this meta-analysis was to verify the association between microbiota level and lung diseases. Studies were included based on the inclusion and exclusion criteria, and data were extracted from these studies. Standard mean deviation (SMD) and related 95% confidence intervals (CIs) were calculated followed by sensitivity, publication bias assessment, and subgroup analyses.
This meta-analysis was guided by the Preferred Reporting Items for Systematic Reviews and Meta-Analysis (PRISMA) guidelines (24).
Two investigators independently performed a literature search using PubMed, ISI Web of Science, Embase, Google Scholar, PMC, and Chinese National Knowledge Infrastructure (CNKI), which was published before March 20, 2021. The publication language was limited to English and Chinese. Each database used a different search strategy. For example, in PubMed, the following MeSH terms and keywords were used: (“microbiota”[MeSH Terms] OR “microbiome”[MeSH Terms] OR “microbiota”[text word] OR “microbiome”[text word] OR “bacteria”[MeSH Terms] OR “bacteria”[textword]) AND (“lung diseases”[MeSH Terms] OR “lung diseases”[text word] OR “asthma”[MeSH Terms] OR “COPD”[MeSH Terms] OR “pneumonia”[MeSH Terms]). In addition, all irrelevant studies, such as case reports, comments, and review articles, were excluded. Relevant studies cited in review articles were also manually searched and included as eligible studies.
Two investigators independently read the titles and abstracts of the searched articles to determine whether they met the inclusion criteria. Both cross-sectional and longitudinal studies on the relationship between microbiota level and lung diseases were included. Studies were included in this meta-analysis if they met the following criteria: (1) included major types of lung diseases, such as COPD, asthma, pulmonary fibrosis, or pneumonitis; (2) the diagnosis of these diseases was clinically confirmed according to the disease guidelines; (3) the study included healthy individuals as a control group; (4) microbiota level was detected in subjects with lung diseases and the control group; (5) the number of case samples and control groups was provided; and (6) the study was published in Chinese or English. Articles were excluded if they: (1) were case reports, comments, animal or cell articles, or review articles; (2) duplicate articles; (3) insufficient data to allow for the extraction of microbiota expression level in patients and controls; and (4) articles not related to microbiota, microbiome, or bacteria.
Two investigators independently performed data extraction, which included the following information from each study: first author, year of publication, age of cases, ethnicity, the number of patients and controls, patient characteristics, sample type, microbiota detection methods, mean and standard deviation (SD) or standard error (SE) values of microbiota in both patients and control individuals. Any inconsistencies were resolved by other researchers until a consensus was reached.
The quality assessment of the studies included in this meta-analysis was conducted using the Newcastle–Ottawa Scale (NOS) (25), which could evaluate the risk of bias of all included case–control studies. The NOS assesses three domains: selection bias, group comparability, and cohort exposure. The total NOS score ranges from 0 to 9, with higher scores indicating better quality.
The mean and SD values of microbiota of the patients and control groups were extracted from the included studies. The SMD and related 95% CIs as the magnitude of the effect were calculated as the amount of the combined effect. Subgroup analysis was conducted based on country, disease difference, and case sample size > 50. Heterogeneity across studies was assessed using I2 statistics. I2-values of 25, 50, and 75% were assigned as low, moderate, and high degrees of heterogeneity, respectively. The pooled effect was determined using a random effect model when the I2 value was >75%. Otherwise, a fixed-effects model was used. A sensitivity analysis was performed to evaluate the influence of each study. Begg's and Egger's tests were used to assess the potential publication bias. All statistical analyses were conducted using Stata software version 12.0 (Stata Corp LP, TX, United States). Statistical significance was set at P < 0.05.
From the literature search, a total of 8,860 articles were retrieved from PubMed, ISI Web of Science, Embase, and other databases. All studies were screened by reading their titles, and 6,545 duplicates were excluded. After reading the abstracts, 2,183 studies were excluded because they were not related to the topic. We then carefully read the full text of the remaining 132 studies. Case reports, reviews, and molecular studies were excluded. Finally, we read the full text of 29 articles that met the inclusion criteria. Studies that did not provide clinical data were excluded. Therefore, we finally obtained six articles that met the inclusion criteria. A detailed schematic of the literature search is shown in Figure 1.
The characteristics of the included studies and the subjects were presented in Table 1. The NOS quality scores of the included studies ranged from 6 to 9 as shown in Table 1. Overall, the included studies comprised 871 individuals (695 patients and 176 healthy individuals), which were published between 2014 and 2020, and conducted in the United Kingdom, United States, Korea, and China. Among these articles, three studies included individuals of Caucasian ethnicity (19, 20, 22), while three studies included individuals of Asian ethnicity (21, 23, 26). The age of the case groups was 50–68 years. The sample size varied from 18 to 532 in the included studies. Moreover, they contained multiple comparisons. Therefore, we treated each group as an independent comparison. The lung microbiota samples were sourced from serum, lung tissue, sputum, and bronchoalveolar lavage fluid samples. The 16S rRNA gene sequence method was used in all included studies for microbiome screening. DNA was extracted from the microbiota, and quantitative reverse transcription-polymerase chain reaction (qRT-PCR) was used to detect the expression level of each microbiota. The subjects had COPD, asthma, IPF, or chronic hypersensitivity pneumonitis (CHP) diseases. In addition, three studies showed an association between bacterial species, such as Streptococcus pneumonia, Klebsiella pneumoniae, and Pseudomonas aeruginosa, and COPD (19, 23, 26). Two studies showed that several kinds of bacteria were related to IPF disease, including Haemophilus, Neisseria, and Streptococcus (20, 22). Yang et al. found that several types of bacteria had a potential relationship with asthma, for example, Acinetobacter baumannii, Enterobacter cloacae, P. aeruginosa, and Staphylococcus aureus among others (26). Prevotella, Streptococcus, Veillonella, Neisseria, and other bacteria were related to the occurrence and development of the CHP disease (20).
Fifty-two comparisons were performed to evaluate the microbiota level in subjects with lung diseases and control individuals, with a total of 871 subjects. Among these studies, a higher microbiota level was observed in subjects with lung diseases (SMD = 0.39, 95% CI = 0.22–0.55, I2= 91.5%, P < 0.01) (Figure 2). Heterogeneity analysis was performed across the included studies using Cochran Q and I2 tests. The results indicated significant heterogeneity across the included studies.
Figure 2. Forest plots of the association between microbiota expression level and patients with lung diseases. This is the overall analysis. For each study, the estimate of differences in mean microbiota level and its 95% confidence interval (95% CI) is plotted with a diamond. SMD, standard mean difference; Chi2, Chi-square statistic; df, degrees of freedom; I2, I-square heterogeneity statistic; IV, inverse variance; Z, Z-statistic.
Next, a subgroup analysis was conducted to explore potential heterogeneity. Country, sample size, type of microbiota, and type of lung disease were used as covariates to perform subgroup analysis using Stata software. Subgroup analysis based on country demonstrated that increased microbiota levels were significantly associated with lung disease occurrence in China (SMD = 1.90, 95% CI = 0.87–2.93, I2 = 62.3%, P < 0.01) and Korea (SMD = 0.24, 95% CI = 0.13–0.35, I2 = 78.7%, P < 0.01), as shown in Figure 3. However, there was no significant association between microbiota level and lung disease in the United Kingdom (SMD = 1.44, 95% CI = −0.11 to 2.98, I2 = 96.7%, P = 0.068) and the United States (SMD = −0.09, 95% CI = −0.23 to 0.05, I2 = 36.4%, P = 0.217). Subgroup analysis based on different lung diseases revealed that IPF patients showed a significant increase in their microbiota level compared to healthy individuals (SMD = 1.40, 95% CI = 0.42–2.38, I2 = 97.3%, P = 0.005), which was similar to that in patients with COPD (SMD = 0.30, 95% CI = 0.09–0.50, I2 = 83.9%, P = 0.004) and asthma (SMD = 0.19, 95% CI = 0.06–0.32, I2 = 69.4%, P = 0.004), as shown in Figure 4. However, a significant decrease in the microbiota level was observed in patients with CHP disease (SMD = −0.16, 95% CI = −0.31 to −0.01, P = 0.033), as shown in Figure 4. The effect of sample size on microbiota level in the disease and control groups was also explored. As shown in Figure 5, the microbiota level increased when the sample size was >50 (SMD = 0.38, 95% CI = 0.21–0.55, I2 = 92.4%, P < 0.01). However, no significant difference was found when the sample size was < 50 (SMD = 0.041, 95% CI = −0.43 to 1.25, I2 = 84.6%, P = 0.342).
Figure 3. Subgroup analysis of the association between microbiota expression level and patients with lung diseases based on different countries.
Figure 4. Subgroup analysis of the association between microbiota expression level and patients with lung diseases based on different lung diseases.
Figure 5. Subgroup analysis of the association between microbiota expression level and patients with lung diseases based on different sample sizes.
Finally, we performed a subgroup analysis based on the different types of microbiota. As shown in Figure 6, A. baumannii (SMD = 0.18, 95% CI = 0.05–0.31, I2 = 40.5%, P = 0.008) and P. aeruginosa (SMD = 0.28, 95% CI = 0.05–0.55, I2 = 80.9%, P = 0.017) showed a significant increase in the study performed by Yang et al. (26), corresponding with COPD and asthma. Although there was no significant difference for E. cloacae in COPD and asthma, there was an increasing trend of 0.20 SMD (P = 0.18). Furthermore, there was no significant relationship between E. cloacae, Haemophilus, Neisseria, S. aureus, and Streptococcus genera and lung diseases. We also conducted a subgroup analysis for A. baumannii, E. cloacae, P. aeruginosa, and S. aureus in patients with asthma and COPD. As shown in Supplementary Figure 1, A. baumannii level was significantly increased in patients with asthma (SMD = 0.15, 95% CI = 0.004–0.286, P = 0.044). However, there was no significant increase in P. aeruginosa level (SMD = 0.25, 95% CI = −0.044 to 0.541, P = 0.096). In addition, there was an increasing trend for A. baumannii (SMD = 0.21) and P. aeruginosa (SMD = 0.32) levels in patients with COPD (Supplementary Figure 2).
Figure 6. Subgroup analysis of the association between microbiota expression level and patients with lung diseases based on different microbiota genera.
In the present meta-analysis, sensitivity analysis and publication bias were also performed using the Stata software. As shown in Supplementary Figure 3, no comparisons fell outside the lower and upper limits. Begg's and Egger's tests demonstrated that there was a low risk of publication bias in this meta-analysis (Supplementary Figure 4).
Numerous investigations had indicated that the lung microbiota played multiple essential roles in lung diseases (27–31). However, previous studies had limitations in their characteristics to provide strong evidence, such as small sample size and lack of compliance with modern methodological research standards. Therefore, it is difficult to draw consistent conclusions from a single trial. Thus, we performed this meta-analysis to evaluate the relationship between microbiota level and lung diseases to provide a reliable evidence-based medical investigation for clinical treatment. Here, we retrieved all publicly available lung microbiome datasets and integrated the data from six related articles, comprising 871 individuals. The present meta-analysis was the first study to indicate that patients with COPD, IPF, and asthma had a higher level of lung microbiota compared to healthy individuals. A. baumannii and P. aeruginosa were more prevalent in patients with COPD and asthma. These findings were novel, and provided insights into the potential of microbial-targeting strategies for the treatment of COPD, asthma, and other lung diseases.
Decreased microbial diversity was associated with impairment of lung function. Furthermore, the variety of lung microbiota decreased with increasing age and disease severity in lung diseases (32, 33). However, previous studies had reported that the abundance of lung microbiota was higher in lung diseases than in healthy controls (22, 23, 26). Similarly, the present meta-analysis indicated that lung microbiota was higher in lung diseases, especially in COPD, IPF, and asthma, which was consistent with previous analyses. Older adults are more likely to suffer from lung disease than individuals who are at 25–44 years of age. Moreover, the mortality risk among older patients with chronic lung disease was elevated 100-fold compared to that in healthy individuals (34). However, the effects of global environmental and geographical alterations on the lung microbiome remained poorly understood (35). For example, the differences in the ecological and air particulate exposures, lifestyle characteristics, the accuracy of microbiota sequencing, or other factors, could lead to differences in the detection of microbiome (36, 37). However, large-scale samples are needed to provide strong evidence to illustrate the role of microbiota in the pathogenesis of lung diseases.
Acinetobacter baumannii is a gram-negative bacterium, which was once considered harmless. Recently, A. baumannii had become one of the most important pathogens that poses the greatest threat to human health in clinical treatment (38). A. baumannii frequently causes a series of lung infections, which may lead to a high mortality rate in patients with lung disease (39). Previous researches had shown that A. baumannii infection induced the production of pro-inflammatory cytokines, such as TNF-α, type I IFN, and IL-1β, which mediated lung immune response and led to cell death (40–43). P. aeruginosa is the leading cause of a decline in lung function and has a high prevalence rate in several types of lung infections (44, 45). P. aeruginosa attaches to different solid surfaces and forms biofilms to enable the bacteria to resist the host's innate immune system and antibiotic treatment (46). P. aeruginosa is commonly found in patients with IPF and asthma (47–51). In intubated patients, P. aeruginosa was also found to exacerbate COPD and contribute to tissue damage (33, 52). In addition, it has five secretory systems that secrete various toxins and hydrolytic enzymes to attack the host (53, 54). It can also induce acute host inflammatory responses (55). The role of A. baumannii and P. aeruginosa in the development of lung diseases, including asthma, COPD, and IPF, remains unclear, and more tangible evidence needs to be extracted. The complexity of the lung microbiota, its genetic and metabolic properties, and manipulation as a marker for the potential treatment of lung diseases need to be studied further.
The present meta-analysis had several limitations. First, only six studies were eligible for the pooled analysis, which may have influenced the interpretation and clinical application of outcomes. Second, IPF, COPD, and asthma were simultaneously included in the meta-analysis. However, there may be a specific microbiota in each of the different lung diseases. Third, we could not perform a pooled analysis based on the various stages of the disease due to insufficient data. Finally, the detection of 16S rRNA was not continuous, therefore we could not obtain a clear consensus on the changes in the microbial community during the occurrence or progression of the disease.
This meta-analysis indicated that patients with IPF, COPD, and asthma had a higher microbiota level than healthy individuals. Moreover, A. baumannii and P. aeruginosa were detected at a higher level in patients with COPD and asthma, which constituted a potential microbiota signature of the diagnosis, and treatment of these lung diseases. However, the interpretation of these results is limited by the small number of included studies and the sample size. Therefore, more studies with a rigorous methodology and larger sample sizes, including multicenter and multi-ethnic subjects, need to be performed.
The raw data supporting the conclusions of this article will be made available by the authors, without undue reservation.
LC, QW, and LZ designed the study and performed the meta-analysis. LC, QW, and WG wrote the draft and revised the manuscript. WG performed the literature search. CS and LZ extracted the data and processed the raw data. All authors contributed to the article and approved the submitted manuscript.
This work was supported by the Fundamental Research Funds for the Heilongjiang Provincial Universities, Scientific Research Fund of Harbin Medical University-Daqing (2018XN-23).
The authors declare that the research was conducted in the absence of any commercial or financial relationships that could be construed as a potential conflict of interest.
All claims expressed in this article are solely those of the authors and do not necessarily represent those of their affiliated organizations, or those of the publisher, the editors and the reviewers. Any product that may be evaluated in this article, or claim that may be made by its manufacturer, is not guaranteed or endorsed by the publisher.
The Supplementary Material for this article can be found online at: https://www.frontiersin.org/articles/10.3389/fmed.2021.723635/full#supplementary-material
1. Khan AA, Sirsat AT, Singh H, Cash P. Microbiota and cancer: current understanding and mechanistic implications. Clin Transl Oncol. (2021) 13:1–10. doi: 10.1007/s12094-021-02690-x
2. Miao R, Wan C. The relationship of gastric microbiota and Helicobacter pylori infection in pediatrics population. Helicobacter. (2020) 25:e12676. doi: 10.1111/hel.12676
3. Ricci A, Pagliuca A, Vermi M. The role of lung colonization in connective tissue disease-associated interstitial lung disease. Microorganisms. (2021) 9:932. doi: 10.3390/microorganisms9050932
4. Wang Z, Perrone F, Belluomini L, Mazzotta M, Bianconi M, Di Noia V, et al. Exploring the role of respiratory microbiome in lung cancer: a systematic review. Helicobacter. (2021) 164:103404. doi: 10.1016/j.critrevonc.2021.103404
6. Ogunrinola GA, Oyewale JO, Oshamika OO, Olasehinde GI. The human microbiome and its impacts on health. Int J Microbiol. (2020) 2020:8045646. doi: 10.1155/2020/8045646
7. Lemon KP, Armitage GC, Relman DA, Fischbach MA. Microbiota-targeted therapies: an ecological perspective. Sci Transl Med. (2012) 4:137rv5. doi: 10.1126/scitranslmed.3004183
8. Moffatt MF, Cookson WO. The lung microbiome in health and disease. Clin Med. (2017) 17:525–29. doi: 10.7861/clinmedicine.17-6-525
9. Hufnagl K, Pali-Scholl I, Roth-Walter F, Jensen-Jarolim E. Dysbiosis of the gut and lung microbiome has a role in asthma. Semin Immunopathol. (2020) 42:75–93. doi: 10.1007/s00281-019-00775-y
10. Hong BY, Paulson JN, Stine OC, Weinstock GM, Cervantes JL. Meta-analysis of the lung microbiota in pulmonary tuberculosis. Tuberculosis. (2018) 109:102–8. doi: 10.1016/j.tube.2018.02.006
11. Hilty M, Burke C, Pedro H, Cardenas P, Bush A, Bossley C, et al. Disordered microbial communities in asthmatic airways. PLoS One. (2010) 5:e8578. doi: 10.1371/journal.pone.0008578
12. Pragman AA, Kim HB, Reilly CS, Wendt C, Isaacson RE. The lung microbiome in moderate and severe chronic obstructive pulmonary disease. PLoS One. (2012) 7:e47305. doi: 10.1371/journal.pone.0047305
13. Willner D, Haynes MR, Furlan M, Schmieder R, Lim YW, Rainey PB, et al. Spatial distribution of microbial communities in the cystic fibrosis lung. ISME J. (2012) 6:471–4. doi: 10.1038/ismej.2011.104
14. Collaborators GBDCRD. Prevalence and attributable health burden of chronic respiratory diseases, 1990-2017: a systematic analysis for the Global Burden of Disease Study 2017. Lancet Respir Med. (2020) 8:585–96. doi: 10.1016/S2213-2600(20)30105-3
15. Raghu G, Collard HR, Egan JJ, Martinez FJ, Behr J, Brown KK, et al. An official ATS/ERS/JRS/ALAT statement: idiopathic pulmonary fibrosis: evidence-based guidelines for diagnosis and management. Am J Respir Crit Care Med. (2011) 183:788–824. doi: 10.1164/rccm.2009-040GL
16. Ley B, Collard HR, King TE Jr. Clinical course and prediction of survival in idiopathic pulmonary fibrosis. Am J Respir Crit Care Med. (2011) 183:431–40. doi: 10.1164/rccm.201006-0894CI
17. Hswen Y, Zhang A, Ventelou B. Estimation of asthma symptom onset using internet search queries: lag-time series analysis. JMIR Public Health Surveill. (2021) 7:e18593. doi: 10.2196/18593
18. Paudel KR, Dharwal V, Patel VK, Galvao I, Wadhwa R, Malyla V, et al. Role of lung microbiome in innate immune response associated with chronic lung diseases. Front Med. (2020) 7:554. doi: 10.3389/fmed.2020.00554
19. Cameron SJ, Lewis KE, Huws SA, Lin W, Hegarty MJ, Lewis PD, et al. Metagenomic sequencing of the chronic obstructive pulmonary disease upper bronchial tract microbiome reveals functional changes associated with disease severity. PLoS One. (2016) 11:e0149095. doi: 10.1371/journal.pone.0149095
20. Invernizzi R, Wu BG, Barnett J, Ghai P, Kingston S, Hewitt RJ, et al. The respiratory microbiome in chronic hypersensitivity pneumonitis is distinct from that of idiopathic pulmonary fibrosis. Am J Respir Crit Care Med. (2021) 203:339–47. doi: 10.1164/rccm.202002-0460OC
21. Kim HJ, Kim YS, Kim KH, Choi JP, Kim YK, Yun S, et al. The microbiome of the lung and its extracellular vesicles in nonsmokers, healthy smokers and COPD patients. Exp Mol Med. (2017) 49:e316. doi: 10.1038/emm.2017.7
22. Molyneaux PL, Cox MJ, Willis-Owen SA, Mallia P, Russell KE, Russell AM, et al. The role of bacteria in the pathogenesis and progression of idiopathic pulmonary fibrosis. Am J Respir Crit Care Med. (2014) 190:906–13. doi: 10.1164/rccm.201403-0541OC
23. Wu D, Hou C, Li Y, Zhao Z, Liu J, Lu X, et al. Analysis of the bacterial community in chronic obstructive pulmonary disease sputum samples by denaturing gradient gel electrophoresis and real-time PCR. BMC Pulm Med. (2014) 14:179. doi: 10.1186/1471-2466-14-179
24. Moher D, Liberati A, Tetzlaff J, Altman DG, Group P. Preferred reporting items for systematic reviews and meta-analyses: the PRISMA statement. PLoS Med. (2009) 6:e1000097. doi: 10.1371/journal.pmed.1000097
25. Stang A. Critical evaluation of the Newcastle-Ottawa scale for the assessment of the quality of nonrandomized studies in meta-analyses. Eur J Epidemiol. (2010) 25:603–5. doi: 10.1007/s10654-010-9491-z
26. Yang J, Hong G, Kim YS, Seo H, Kim S, McDowell A, et al. Lung disease diagnostic model through igg sensitization to microbial extracellular vesicles. Allergy Asthma Immunol Res. (2020) 12:669–83. doi: 10.4168/aair.2020.12.4.669
27. Gupta S, Shariff M, Chaturvedi G, Sharma A, Goel N, Yadav M, et al. Comparative analysis of the alveolar microbiome in COPD, ECOPD, Sarcoidosis, and ILD patients to identify respiratory illnesses specific microbial signatures. Sci Rep. (2021) 11:3963. doi: 10.1038/s41598-021-83524-2
28. Losol P, Choi JP, Kim SH, Chang YS. The role of upper airway microbiome in the development of adult asthma. Immune Netw. (2021) 21:e19. doi: 10.4110/in.2021.21.e19
29. Ran Z, Liu J, Wang F, Xin C, Xiong B, Song Z. Pulmonary micro-ecological changes and potential microbial markers in lung cancer patients. Front Oncol. (2020) 10:576855. doi: 10.3389/fonc.2020.576855
30. Tiew PY, Jaggi TK, Chan LLY, Chotirmall SH. The airway microbiome in COPD, bronchiectasis and bronchiectasis-COPD overlap. Clin Respir J. (2021) 15:123–33. doi: 10.1111/crj.13294
31. Faner R, Sibila O, Agusti A, Bernasconi E, Chalmers JD, Huffnagle GB, et al. The microbiome in respiratory medicine: current challenges and future perspectives. Eur Respir J. (2017) 49:1602086. doi: 10.1183/13993003.02086-2016
32. Pittman JE, Wylie KM, Akers K, Storch GA, Hatch J, Quante J, et al. Association of antibiotics, airway microbiome, and inflammation in infants with cystic fibrosis. Ann Am Thorac Soc. (2017) 14:1548–55. doi: 10.1513/AnnalsATS.201702-121OC
33. Quinn RA, Adem S, Mills RH, Comstock W, DeRight Goldasich L, Humphrey G, et al. Neutrophilic proteolysis in the cystic fibrosis lung correlates with a pathogenic microbiome. Microbiome. (2019) 7:23. doi: 10.1186/s40168-019-0636-3
35. Mao Q, Jiang F, Yin R, Wang J, Xia W, Dong G, et al. Interplay between the lung microbiome and lung cancer. Cancer Lett. (2018) 415:40–8. doi: 10.1016/j.canlet.2017.11.036
36. Yu G, Gail MH, Consonni D, Carugno M, Humphrys M, Pesatori AC, et al. Characterizing human lung tissue microbiota and its relationship to epidemiological and clinical features. Genome Biol. (2016) 17:163. doi: 10.1186/s13059-016-1021-1
37. Anand S, Mande SS. Diet, microbiota and gut-lung connection. Front Microbiol. (2018) 9:2147. doi: 10.3389/fmicb.2018.02147
38. Tacconelli E, Carrara E, Savoldi A, Harbarth S, Mendelson M, Monnet DL, et al. Discovery, research, and development of new antibiotics: the WHO priority list of antibiotic-resistant bacteria and tuberculosis. Lancet Infect Dis. (2018) 18:318–27. doi: 10.1016/S1473-3099(17)30753-3
39. Wong D, Nielsen TB, Bonomo RA, Pantapalangkoor P, Luna B, Spellberg B. Clinical and pathophysiological overview of acinetobacter infections: a century of challenges. Clin Microbiol Rev. (2017) 30:409–47. doi: 10.1128/CMR.00058-16
40. Smani Y, Docobo-Perez F, McConnell MJ, Pachon J. Acinetobacter baumannii-induced lung cell death: role of inflammation, oxidative stress and cytosolic calcium. Microb Pathog. (2011) 50:224–32. doi: 10.1016/j.micpath.2011.01.008
41. Kang MJ, Jo SG, Kim DJ, Park JH. NLRP3 inflammasome mediates interleukin-1beta production in immune cells in response to Acinetobacter baumannii and contributes to pulmonary inflammation in mice. Immunology. (2017) 150:495–505. doi: 10.1111/imm.12704
42. Li Y, Guo X, Hu C, Du Y, Guo C, Di W, et al. Type I IFN operates pyroptosis and necroptosis during multidrug-resistant A. baumannii infection. Cell Death Differ. (2018) 25:1304–18. doi: 10.1038/s41418-017-0041-z
43. Rosales-Reyes R, Gayosso-Vazquez C, Fernandez-Vazquez JL, Jarillo-Quijada MD, Rivera-Benitez C, Santos-Preciado JI, et al. Virulence profiles and innate immune responses against highly lethal, multidrug-resistant nosocomial isolates of Acinetobacter baumannii from a tertiary care hospital in Mexico. PLoS One. (2017) 12:e0182899. doi: 10.1371/journal.pone.0182899
44. Fuentes-Zacarias P, Arzate-Castaneda DA, Sosa-Gonzalez I, Villeda-Gabriel G, Morales-Mendez I, Osorio-Caballero M, et al. Pseudomonas aeruginosa induces spatio-temporal secretion of IL-1beta, TNFalpha, proMMP-9, and reduction of epithelial E-cadherin in human alveolar epithelial type II (A549) cells. Acta Biochim Pol. (2021) 68:207–15. doi: 10.18388/abp.2020_5509
45. Schick A, Kassen R. Rapid diversification of Pseudomonas aeruginosa in cystic fibrosis lung-like conditions. Proc Natl Acad Sci USA. (2018) 115:10714–19. doi: 10.1073/pnas.1721270115
46. Mulcahy LR, Isabella VM, Lewis K. Pseudomonas aeruginosa biofilms in disease. Microb Ecol. (2014) 68:1–12. doi: 10.1007/s00248-013-0297-x
47. Ding FX, Liu B, Zou WJ, Li QB, Tian DY, Fu Z. Correction: pseudomonas aeruginosa-derived exosomes ameliorates allergic reactions via inducing the Treg response in asthma. Pediatr Res. (2020) 88:822. doi: 10.1038/s41390-020-0823-8
48. Faure E, Kwong K, Nguyen D. Pseudomonas aeruginosa in Chronic Lung Infections: how to adapt within the host? Front Immunol. (2018) 9:2416. doi: 10.3389/fimmu.2018.02416
49. John A, Gozdzik-Spychalska J, Durda-Masny M, Czainski W, Pawlowska N, Wlizlo J, et al. Pseudomonas aeruginosa, the type of mutation, lung function, and nutritional status in adults with cystic fibrosis. Nutrition. (2021) 89:111221. doi: 10.1016/j.nut.2021.111221
50. Maurice NM, Bedi B, Sadikot RT. Pseudomonas aeruginosa biofilms: host response and clinical implications in lung infections. Am J Respir Cell Mol Biol. (2018) 58:428–39. doi: 10.1165/rcmb.2017-0321TR
51. Tuli JF, Ramezanpour M, Cooksley C, Psaltis AJ, Wormald PJ, Vreugde S. Association between mucosal barrier disruption by Pseudomonas aeruginosa exoproteins and asthma in patients with chronic rhinosinusitis. Allergy. (2021) 76:3459–69. doi: 10.1111/all.14959
52. Huang YJ, Kim E, Cox MJ, Brodie EL, Brown R, Wiener-Kronish JP, et al. A persistent and diverse airway microbiota present during chronic obstructive pulmonary disease exacerbations. OMICS. (2010) 14:9–59. doi: 10.1089/omi.2009.0100
53. Bleves S, Viarre V, Salacha R, Michel GP, Filloux A, Voulhoux R. Protein secretion systems in Pseudomonas aeruginosa: a wealth of pathogenic weapons. Int J Med Microbiol. (2010) 300:534–43. doi: 10.1016/j.ijmm.2010.08.005
54. Pena RT, Blasco L, Ambroa A, Gonzalez-Pedrajo B, Fernandez-Garcia L, Lopez M, et al. Relationship between quorum sensing and secretion systems. Front Microbiol. (2019) 10:1100. doi: 10.3389/fmicb.2019.01100
Keywords: lung microbiota, COPD, asthma, meta-analysis, IPF—idiopathic pulmonary fibrosis
Citation: Chai L, Wang Q, Si C, Gao W and Zhang L (2022) Potential Association Between Changes in Microbiota Level and Lung Diseases: A Meta-Analysis. Front. Med. 8:723635. doi: 10.3389/fmed.2021.723635
Received: 28 June 2021; Accepted: 14 December 2021;
Published: 14 January 2022.
Edited by:
Piero Valentini, Agostino Gemelli University Polyclinic (IRCCS), ItalyReviewed by:
Setegn Eshetie, University of Gondar, EthiopiaCopyright © 2022 Chai, Wang, Si, Gao and Zhang. This is an open-access article distributed under the terms of the Creative Commons Attribution License (CC BY). The use, distribution or reproduction in other forums is permitted, provided the original author(s) and the copyright owner(s) are credited and that the original publication in this journal is cited, in accordance with accepted academic practice. No use, distribution or reproduction is permitted which does not comply with these terms.
*Correspondence: Wenyan Gao, Z2Fvd2VueWFuMTk4N0AxMjYuY29t; Lun Zhang, emhhbmdsdW4zMDZAMTYzLmNvbQ==
†These authors have contributed equally to this work
Disclaimer: All claims expressed in this article are solely those of the authors and do not necessarily represent those of their affiliated organizations, or those of the publisher, the editors and the reviewers. Any product that may be evaluated in this article or claim that may be made by its manufacturer is not guaranteed or endorsed by the publisher.
Research integrity at Frontiers
Learn more about the work of our research integrity team to safeguard the quality of each article we publish.