- 1Centre for Bioengineering, Mechanical Engineering, University of Canterbury, Christchurch, New Zealand
- 2Department of Intensive Care, Christchurch Hospital, University of Otago Christchurch School of Medicine, Christchurch, New Zealand
- 3Department of Intensive Care, Erasme University Hospital, Brussels, Belgium
- 4GIGA In Silico Medicine, University of Liege, Liege, Belgium
Introduction
For intensive care medicine, significant advances in care have been limited in recent years. A wealth of randomized clinical trials and a wide range of new protocols have yielded a wealth of understanding but have not created the next-generation care hoped for. As a result, a “less is more” perspective is growing in the field. This opinion article looks to the field of economics for lessons in how we might consider changing perspective to develop and assess new approaches to care.
Current approaches to major forms of care in the intensive care unit (ICU) have stagnated, increasingly complex patients with multiple co-morbidities have resulted in increased use of resources, cost and risk of complications. Growing fear of iatrogenic complications has led to a “less is more” approach to new care (1–3). The major hurdle to overcome is the increase in negative outcomes, which seem to regularly arise with more aggressive approaches to care seen in a range of randomized clinical trials in the last two or more decades. This analysis takes an outside the box approach, with lessons from the field of Economics, to assess the situation and make some bold proposals, in the interest primarily of stimulating “creative tension” in the field as to considering the way forward.
Cost-Benefit Analysis, So-Called Fat-Tailed Risk, and Medicine
A great deal of medical care treatment, perhaps particularly in the intensive care unit (ICU), can be seen as decision making under uncertainty. An economist might broadly define this process as assessing the potential value of the investment now vs. alternative outcome risks in the future, and discounting the “cost” of the outcome accordingly. Discounting adjusts the costs and outcomes of spending or interventions to account for investment benefits in delaying some or all of the cost of a treatment (4). The underlying issue is to determine how much to invest now – cost-benefit analysis (CBA) in simple. Thus, the question of what choice to make should be based on the relative “cost” of mistakes and their discounted cost.
However, the efficacy of CBA has been recently questioned based on its use in climate change analysis, challenged by Weitzman's “Dismal Theorem” (5). Weitzman used this theorem to show how traditional CBA could break down in issues like climate change, where avoiding a catastrophic future would demand an infinite investment now. By analogy, in the ICU, the ultimate cost to the patient is death, which would necessitate infinite investment in care using traditional CBA. However, such infinite investment, despite the actual high costs of critical care, is not typically made. This outcome demonstrates the fragility of CBA in the face of catastrophic or “fat tailed” risk, which occurs when catastrophic events can occur with non-negligible likelihood, no matter how remote the chance. More succinctly, the tail of the probability (of occurrence) distribution is “fat” and has non-negligible likelihood.
So-called “fat-tailed risk” arises from the formal definition of the Dismal Theorem noting society has an infinite or undefinable expected loss from very high consequence, but relatively very low-probability events. The infinite cost makes it impossible to calculate a present value to invest now to avoid it and thus no discount rate can apply to make a decision between options. The opposite, given human behavior, also arises, where low consequence, higher probability events in future are overly discounted and assumed to have a much lower or negligible impact and cost today due to a range of factors (6, 7).
More specifically, decision making for clinical staff and family is difficult, when we do not understand and cannot calculate the risks and thus value of a given treatment, when death or an unlikely event associated with increased mortality is a possible, albeit unlikely outcome. More importantly, the opposite case can also hold, where the risk of death or a negative outcome is assumed to be lower than is actually the case, and so actions may not be taken, the result of which are harmful to the patient. These “poor” choices or difficult decisions are a result of underlying uncertainty in patient response to care and thus in outcome, and in particular of our inability to quantify them to more optimally guide decision making and risk assessment in medical care, and in critical care in particular.
This difficult compromise can lead to poor choices, in both economics, but also as posited here, in medical decision-making – all complicated by the underlying uncertainty of what might occur in future, and the inability to properly assess underlying risk of both very unlikely, and more likely, events.
Analogies in ICU Medicine
One clear, highly debated analogy in intensive care unit (ICU) medicine arises from the debate around glycemic control (GC) (8). Beyond potential benefit and the high difficulty in providing safe, effective GC for all patients (9–11), the risk of hypoglycemia—a potentially catastrophic event in terms of outcome risk of death (12–16)—is offset by the risk of permissive hyperglycemia from higher targets (17) and associated glycemic variability (18–21), which have much lower perceived relative risks, but are also associated with increased mortality. Thus, economically, there is failure to control or invest in GC given the so-called fat-tailed risk of hypoglycemia, where no current investment seems able to ameliorate the problem, and the opposite case also holding, where the risk of death due to permissive poor GC increases the risk of death due to underestimating the risk (over discounting).
This approach would provide an acceptable trade-off if there was no possibility to obtain good control, safe from hypoglycemia. Equally, the much lower (relative) and highly discounted perceived risk of hyperglycemia for ICU patients accepted in this trade-off clashes with the actual, higher relative risk observed (22–26). In contrast, emerging personalized, model-based GC approaches can provide safe, effective control with reduced hypoglycemia (1–2% of patients) (27–29), and emerging glucose sensing technologies offer further safety increases (30).
However, in short, there remains a tendency to “invest nothing,” and thus to minimal GC, to avoid the (now manageable) fat-tailed catastrophic risk of hypoglycemia. Concomitantly, there is thus also the resulting tendency to accept relatively permissive levels of hyperglycemia by under-valuing and over-discounting its negative effects and increased risk of death. These choices and resulting outcome match well-known and increasingly well-accepted economic observations and human behaviors.
However, the “Dismal Theorem” is an “impossibility theorem” and it does not tell you what you should do instead (31). Relevant to the position made here, Weitzman was quoted: “We desperately need more information about what's going on in these tails. It's not the median values that are gonna kill us” (32). While he said this concerning climate change, it could be equally applied to many risks and choices made in ICU medicine, not just GC. More directly, if we could quantify uncertainty and variability in response to care, we could appropriately assess the risk, and choose, economically speaking, the right discount rate to assess whether (or not) to “invest” in more aggressive care.
The trade-off between these types of risk can be found in many core ICU therapies. Mechanical ventilation has the trade-off between the sudden impact of barotrauma or volutrauma vs. the “slow” damage of increased inspired oxygen settings when setting PEEP or tidal volume (33). Thus, quantifying the risk, via new metrics or personalized models, would allow the proper assessment of patient response and thus whether (or not) to provide more aggressive ventilation settings, which if done incorrectly increase the risk of cost, length of stay, and mortality. Fluid resuscitation therapy faces a similar contradiction between providing more input to support circulatory and cardiac function, and the ongoing risk of the therapy itself to patient outcome (34, 35). Quantifying responsiveness to fluid resuscitation therapy is a “holy grail” of ICU research, as quantifying the response would reduce the risk and make it manageable. In all cases, difficulty arises from the neither knowing, quantifying nor managing the risk of outlying, harmful patient responses to care due to inter- and intra- patient variability.
The Problem of Outliers, Variability and Risk
In general, many therapy approaches in randomized trials target median or mean behaviors, the central tendency of a cohort. However, they often fail to account for outliers or are unable to achieve these median goal targets for all or nearly all patients. Equally, those outlying events and patients can twist therapy targets due to their high risk of negative impact, such as hypoglycemia in GC. These outcomes suggest risk of behaviors for a therapy, rather than outcome, should be primary targets, particularly where fat-tailed risks can be quantified and managed.
Specifically, new approaches should seek to eliminate or directly manage outliers as they constitute the fat-tailed risk and are where both clinical and economical costs reside. This risk-based approach focuses less on achieving a target and more on minimizing any harm from care. It thus focuses on the knowing and managing the “tails” of the distribution of possible patient responses, rather than the central tendency or expected values, and in doing so might provide the means to develop therapies providing safe, effective care for virtually all patients. Overall, understanding and management of more immediate risks can allow better CBA and decision-making when it comes to evaluating interventions.
Figure 1 schematically shows how knowledge of potential variability in patient response can be used in a risk-based approach. When the distribution is unknown the response can be more or less variable, creating undesirable risk of a patient outcome response being too low (or too high) if the distribution is fat-tailed or wide. However, if the distribution is known, then care choices can be predicated on this knowledge, allowing a set threshold (5% in Figure 1) of risk in response to care. The analogy in the glycemic control case would be blood glucose below 4.0 mmol/L (72 mg/dL), associated with increasing risk.
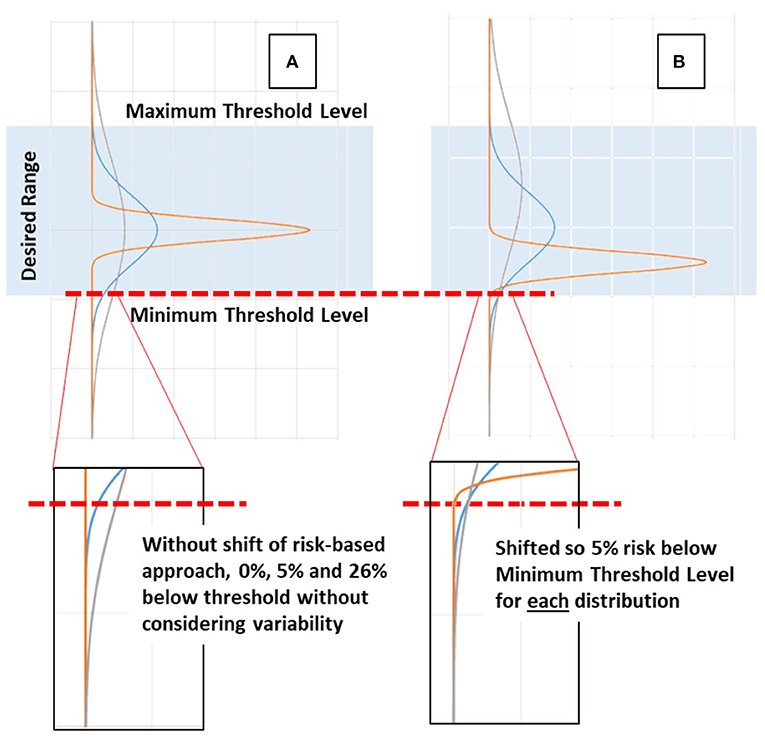
Figure 1. Schematic example of risk-based vs. typical approaches. (A) When distributions are unknown and median or central tendency values are targeted all distributions are centered together. A wide or fat-tailed distribution of response due to patient variability can create significant risk of being too low or too high compared to a desired range. (B) When the distribution and the tails in particular are known, then a set threshold of risk (5%) of being too low (or high) can be set and used to guide care, effectively shifting the distributions to a set lower bound in the example shown, and allowing the remainder of the distribution to fall where it may.
Given the focus on uncertainty in Weitzman's work and in CBA in general, as applied economically, there is significant strong analogy to the practice of medicine. Using GC as a “straw man” it is particularly applicable to the design and evaluation of new therapy approaches, where randomized trials are often confounded by the complexity, multiple factors, and uncertainty governing major outcomes in ICU patients and thus fail to deliver clear direction to the field (2, 3). Thus, the ability to quantify and directly manage patient variability and risk in care would provide a new way of approaching not only care, but how it is developed and evaluated.
Determining Variability and Managing Risk
Determining the distribution of risk or patient variability can be done using data, which is more and more available, both directly in statistical or stochastic models (36–38) or via a range of emerging artificial intelligence and/or deep learning methods (39). In fact, managing patient variability is an emerging field reflected in a range of recent research. Specifically in areas like new drug development methods, assessing differences between in response to care due to differences in race or sex, the use of a range of “omics” (e.g., proteomics, genomics) to better classify and target care, and in the broader use of “big data” and machine learning to everything from personalizing care to early warning alarms and other applications.
All of these approaches are emerging means to better classify patients and their potential needs, risks and variable responses (to care), sharing the common trait of bringing risk management into dosing and care. Doing so inherently creates increasingly personalized protocols. The act of personalizing care, whether done at the bedside or via algorithms and technology, is about managing patient-specific behaviors and responses to care outside the mean, median or central tendency, otherwise there would be no point to the effort. It is thus about giving all patients the best opportunity to respond like the expected target patient – inherently then, it is about risk reduction.
Once potential or actual variability and its risk is quantified in some way, it can be directly managed in care. Currently, only one glycemic control protocol takes such an approach in critical care, using a direct model-based approach to cohort variability to minimize hypoglycemia and provide consistent care across a range of cohorts (27). It shows the potential of this type of approach, vs. a targeted glycemia approach, and as data increases, greater resolution and personalization should be realized.
Hence, it is important to quantify variability for use in managing patient care to reduce the risk of outlier responses and to thus create ever narrower ranges of overall patient response to care. However, risk reduction is often a secondary outcome at best in many studies, with the primary focus being performance to desired clinical outcomes. If the future of ICU care is on personalizing care, and thus on directly managing and reducing risk, then it might be time to consider risk quantification, management and reduction as the primary goal in developing new approaches to care.
Surely if the risk of care can be managed and reduced, particularly in current care, then the increased personalization of care might help overcome a current stagnation in improving outcomes (and cost).
A Bold Proposition?
As a result, this opinion and commentary puts forward two main propositions:
• Greater emphasis should be put on risk-based dosing, rather than on target-based dosing to minimize the likelihood of both fat-tailed and perceived lesser risks. In GC, dosing on risk to simultaneously minimize the relative risks of both hypoglycemia and permissive hyperglycemia. In short, dosing to minimize outliers rather than achieve a desired specific target or outcome.
• There needs to be far greater weight in clinical research put on evidence quantifying relative risks of choices in core ICU therapies, where large randomized trials on overall outcomes struggle given all the other uncertainties and complexity in the ICU patient (3).
More succinctly, when developing and evaluating protocols or approaches, like policy levers and choices in economics, risk-based and optimized decision making should be of concern over targeted median values and outcome focused approaches. This risk-based approach is not today's care, but should be tomorrow's.
Discussion
The field of intensive care medicine faces significant challenges from increasing demographics and complexity of patients. However, it has seen far less significant advances in care compared to the level and intensity of research directed at the core problems it faces in care. This outside the box analysis examines the issue and through a set of “bold proposals” suggests the need for much greater quantification and direct management of risk in clinical care, treating outliers rather than seeking targets for the “middle.” It thus suggests re-examining how clinical evidence is viewed and redesigning how clinical trial goals are designed in an effort to address issues with variability and risk, which are currently under-served or ignored.
Author Contributions
JC developed the idea and led writing. GS, J-CP, JK, and TD provided additional input and contributions to the development and writing. All authors contributed to the article and approved the submitted version.
Funding
EU H2020 funding for the RISE project Digital Clones for Personalised Medicine (DPCM) grant no (#872488 – DCPM).
Conflict of Interest
The authors declare that the research was conducted in the absence of any commercial or financial relationships that could be construed as a potential conflict of interest.
References
1. Auriemma CL, Van den Berghe G, Halpern SD. Less is more in critical care is supported by evidence-based medicine. Intens Care Med. (2019) 45:1806–9. doi: 10.1007/s00134-019-05771-2
2. Vincent JL. We should abandon randomized controlled trials in the intensive care unit. Crit Care Med. (2010) 38(10 Suppl.):S534–8. doi: 10.1097/CCM.0b013e3181f208ac
3. Vincent JL. Improved survival in critically ill patients: are large RCTs more useful than personalized medicine? Intens Care Med. (2016) 42:1778–80. doi: 10.1007/s00134-016-4482-5
4. Torgerson DJ, Raftery J. Economic notes. Discounting. BMJ. (1999) 319:914–5. doi: 10.1136/bmj.319.7214.914
5. Weitzman ML. On modeling and interpreting the economics of catastrophic climate change. The review of economics and statistics. (2009) 91:1–19. doi: 10.1162/rest.91.1.1
6. Berkman ET, Kahn LE, Livingston JL. Valuation as a Mechanism of Self-Control and Ego Depletion, in Self-Regulation and Ego Control. Elsevier (2016). p. 255–79.
7. Wakker PP. Explaining the characteristics of the power (CRRA) utility family. Health Econ. (2008) 17:1329–44. doi: 10.1002/hec.1331
8. Chase JG, Dickson JL. Traversing the valley of glycemic control despair. Crit Care. (2017) 21:237. doi: 10.1186/s13054-017-1824-9
9. Chase JG, Desaive T, Bohe J, Cnop M, De Block C, Gunst J, et al. Improving glycemic control in critically ill patients: personalized care to mimic the endocrine pancreas. Crit Care. (2018) 22:182. doi: 10.1186/s13054-018-2110-1
10. Chase JG, Preiser JC, Dickson JL, Pironet A, Chiew YS, Pretty CG, et al. Next-generation, personalised, model-based critical care medicine: a state-of-the art review of in silico virtual patient models, methods, and cohorts, and how to validation them. Biomed Eng Online. (2018) 17:24. doi: 10.1186/s12938-018-0455-y
11. Chase JG, Benyo B, Desaive T. Glycemic control in the intensive care unit: a control systems perspective. Ann Rev Control. (2019) 48:359–68. doi: 10.1016/j.arcontrol.2019.03.007
12. Bagshaw SM, Bellomo R, Jacka MJ, Egi M, Hart GK, George C. The impact of early hypoglycemia and blood glucose variability on outcome in critical illness. Crit Care. (2009) 13:R91. doi: 10.1186/cc7921
13. Finfer S, Liu B, Chittock DR, Norton R, Myburgh JA, McArthur C, et al. Hypoglycemia and risk of death in critically ill patients. N Engl J Med. (2012) 367:1108–18. doi: 10.1056/NEJMoa1204942
14. Penning S, Pretty C, Preiser JC, Shaw GM, Desaive T, Chase JG. Glucose control positively influences patient outcome: a retrospective study. J Crit Care. (2015) 30:455–9. doi: 10.1016/j.jcrc.2014.12.013
15. Egi M, Bellomo R, Stachowski E, French CJ, Hart GK, Taori G, et al. Hypoglycemia and outcome in critically ill patients. Mayo Clin Proc. (2010) 85:217–24. doi: 10.4065/mcp.2009.0394
16. Kalfon P, Le Manach Y, Ichai C, Brechot N, Cinotti R, Dequin PF, et al. Severe and multiple hypoglycemic episodes are associated with increased risk of death in ICU patients. Crit Care. (2015) 19:153. doi: 10.1186/s13054-015-0851-7
17. Moghissi ES, Korytkowski MT, DiNardo M, Einhorn D, Hellman R, Hirsch IB, et al. American Association of Clinical Endocrinologists and American Diabetes Association consensus statement on inpatient glycemic control. Diabetes Care. (2009) 32:1119–31. doi: 10.2337/dc09-9029
18. Krinsley JS, Preiser JC. Time in blood glucose range 70 to 140 mg/dl >80% is strongly associated with increased survival in non-diabetic critically ill adults. Crit Care. (2015) 19:179. doi: 10.1186/s13054-015-0908-7
19. Signal M, Le Compte A, Shaw GM, Chase JG. Glycemic levels in critically ill patients: are normoglycemia and low variability associated with improved outcomes? J Diabetes Sci Technol. (2012) 6:1030–7. doi: 10.1177/193229681200600506
20. Egi M, Bellomo R. Reducing glycemic variability in intensive care unit patients: a new therapeutic target? J Diabetes Sci Technol. (2009) 3:1302–8. doi: 10.1177/193229680900300610
21. Lanspa MJ, Krinsley JS, Hersh AM, Wilson EL, Holmen JR, Orme JF, et al. Percentage of time in range 70 to 139 mg/dL is associated with reduced mortality among critically ill patients receiving IV insulin infusion. Chest. (2019) 156:878–86. doi: 10.1016/j.chest.2019.05.016
22. Krinsley JS. Association between hyperglycemia and increased hospital mortality in a heterogeneous population of critically ill patients. Mayo Clin Proc. (2003) 78:1471–8. doi: 10.4065/78.12.1471
23. Falciglia M, Freyberg RW, Almenoff PL, D'Alessio DA, Render ML. Hyperglycemia-related mortality in critically ill patients varies with admission diagnosis. Crit Care Med. (2009) 37:3001–9. doi: 10.1097/CCM.0b013e3181b083f7
24. Finney SJ, Zekveld C, Elia A, Evans TW. Glucose control and mortality in critically ill patients. JAMA. (2003) 290:2041–7. doi: 10.1001/jama.290.15.2041
25. Jones KW, Cain AS, Mitchell JH, Millar RC, Rimmasch HL, French TK, et al. Hyperglycemia predicts mortality after CABG: postoperative hyperglycemia predicts dramatic increases in mortality after coronary artery bypass graft surgery. J Diabetes Comp. (2008) 22:365–70. doi: 10.1016/j.jdiacomp.2007.05.006
26. Whitcomb BW, Pradhan EK, Pittas AG, Roghmann MC, Perencevich EN. Impact of admission hyperglycemia on hospital mortality in various intensive care unit populations. Crit Care Med. (2005) 33:2772–7. doi: 10.1097/01.CCM.0000189741.44071.25
27. Stewart KW, Pretty CG, Tomlinson H, Thomas FL, Homlok J, Noemi SN, et al. Safety, efficacy and clinical generalization of the STAR protocol: a retrospective analysis. Ann Intens Care. (2016) 6:24. doi: 10.1186/s13613-016-0125-9
28. Dubois J, Van Herpe T, van Hooijdonk RT, Wouters R, Coart D, Wouters P, et al. Software-guided versus nurse-directed blood glucose control in critically ill patients: the LOGIC-2 multicenter randomized controlled clinical trial. Crit Care. (2017) 21:212. doi: 10.1186/s13054-017-1799-6
29. Blaha J, Kopecky P, Matias M, Hovorka R, Kunstyr J, Kotulak T, et al. Comparison of three protocols for tight glycemic control in cardiac surgery patients. Diabetes Care. (2009) 32:757–61. doi: 10.2337/dc08-1851
30. Preiser JC, Lheureux O, Thooft A, Brimioulle S, Goldstein J, Vincent JL. Near-continuous glucose monitoring makes glycemic control safer in ICU patients. Crit Care Med. (2018) 46:1224–9. doi: 10.1097/CCM.0000000000003157
31. Anthoff D, Tol RSJ. Climate policy under fat-tailed risk: an application of FUND. Ann Operat Res. (2014) 220:223–37. doi: 10.1007/s10479-013-1343-2
33. Major VJ, Chiew YS, Shaw GM, Chase JG. Biomedical engineer's guide to the clinical aspects of intensive care mechanical ventilation. Biomed Eng Online. (2018) 17:169. doi: 10.1186/s12938-018-0599-9
34. Desaive T, Horikawa O, Ortiz JP, Chase JG. Model-based management of cardiovascular failure: where medicine and control systems converge. Ann Rev Control. (2019) 48:383–91. doi: 10.1016/j.arcontrol.2019.05.003
35. Cecconi M, De Backer D, Antonelli M, Beale R, Bakker J, Hofer C, et al. Consensus on circulatory shock and hemodynamic monitoring. Task force of the European Society of Intensive Care Medicine. Intens Care Med. (2014) 40:1795–815. doi: 10.1007/s00134-014-3525-z
36. Le Compte AJ, Lee DS, Chase JG, Lin J, Lynn A, Shaw GM. Blood glucose prediction using stochastic modeling in neonatal intensive care. IEEE Trans Biomed Eng. (2010) 57:509–18. doi: 10.1109/TBME.2009.2035517
37. Lin J, Lee D, Chase JG, Shaw GM, Le Compte A, Lotz T, et al. Stochastic modelling of insulin sensitivity and adaptive glycemic control for critical care. Comput Methods Programs Biomed. (2008) 89:141–52. doi: 10.1016/j.cmpb.2007.04.006
38. Uyttendaele V, Knopp JL, Stewart KW, Desaive T, Benyó B, Szabo-Nemedi N, et al. A 3D insulin sensitivity prediction model enables more patient-specific prediction and model-based glycaemic control. Biomed Signal Process Control. (2018) 46:192–200. doi: 10.1016/j.bspc.2018.05.032
Keywords: decision-making, risk, cost-benefit analysis, risk-based protocol, risk-based assessment
Citation: Chase JG, Shaw GM, Preiser J-C, Knopp JL and Desaive T (2021) Risk-Based Care: Let's Think Outside the Box. Front. Med. 8:535244. doi: 10.3389/fmed.2021.535244
Received: 15 February 2020; Accepted: 22 January 2021;
Published: 25 February 2021.
Edited by:
César Aldecoa, Hospital Universitario Río Hortega, SpainReviewed by:
Lee S. Nguyen, Clinique Ambroise Paré, FranceCopyright © 2021 Chase, Shaw, Preiser, Knopp and Desaive. This is an open-access article distributed under the terms of the Creative Commons Attribution License (CC BY). The use, distribution or reproduction in other forums is permitted, provided the original author(s) and the copyright owner(s) are credited and that the original publication in this journal is cited, in accordance with accepted academic practice. No use, distribution or reproduction is permitted which does not comply with these terms.
*Correspondence: James Geoffrey Chase, Z2VvZmYuY2hhc2VAY2FudGVyYnVyeS5hYy5ueg==