- 1College of Medicine, Mohammed bin Rashid University of Medicine and Health Sciences, Dubai, United Arab Emirates
- 2Clinical Sciences Department, College of Medicine, University of Sharjah, Sharjah, United Arab Emirates
- 3Ministry of Health and Prevention (MOHAP), Dubai, United Arab Emirates
- 4The Royal Hospital, Muscat, Oman
Background: Identifying clinical-features or a scoring-system to predict a benefit from hospital admission for patients with COVID-19 can be of great value for the decision-makers in the health sector. We aimed to identify differences in patients' demographic, clinical, laboratory, and radiological findings of COVID-19 positive cases to develop and validate a diagnostic-model predicting who will develop severe-form and who will need critical-care in the future.
Methods: In this observational retrospective study, COVID-19 positive cases (total 417) diagnosed in Al Kuwait Hospital, Dubai, UAE were recruited, and their prognosis in terms of admission to the hospital and the need for intensive care was reviewed until their tests turned negative. Patients were classified according to their clinical state into mild, moderate, severe, and critical. We retrieved all the baseline clinical data, laboratory, and radiological results and used them to identify parameters that can predict admission to the intensive care unit (ICU).
Results: Patients with ICU admission showed a distinct clinical, demographic as well as laboratory features when compared to patients who did not need ICU admission. This includes the elder age group, male gender, and presence of comorbidities like diabetes and history of hypertension. ROC and Precision-Recall curves showed that among all variables, D dimers (>1.5 mg/dl), Urea (>6.5 mmol/L), and Troponin (>13.5 ng/ml) could positively predict the admission to ICU in patients with COVID-19. On the other hand, decreased Lymphocyte count and albumin can predict admission to ICU in patients with COVID-19 with acceptable sensitivity (59.32, 95% CI [49.89–68.27]) and specificity (79.31, 95% CI [72.53–85.07]).
Conclusion: Using these three predictors with their cut of values can identify patients who are at risk of developing critical COVID-19 and might need aggressive intervention earlier in the course of the disease.
Background
The pandemic of (COVID-19), which began at the end of 2019, represents an international public health emergency (1). Most patients with this disease suffer from mild to moderate illnesses (2). However, a small percentage of those patients suffer from more severe illnesses that can rapidly progress into a more critical form. This includes ARDS and acute respiratory failure, in addition to metabolic acidosis, coagulopathies, and septic shock (3). Depending on patient characteristics and the studied population, ICU admission varies between 5 and 16% of the total number of patients (4). The widespread of the disease led to a rapid overwhelming of the public health system of different countries, including the intensive care units (5), with some countries reaching a critical care crisis (6).
The current pandemic increased the burden substantially on acute and critical care services exceeding existing hospital capacity around the world (7). Managing the expected surges in intensive care capacity requires focused intensive care abilities and requirements to minimize loss of life and maintain control (8). Due to the increasing numbers of recorded positive COVID-19 cases, the medical teams in the front line are in urgent need of a tool that helps their clinical judgment to identify the few that will progress to critical cases.
A stratification tool for non-severe COVID-19 patients at admission can direct the resources and control the spread more efficiently and persevere the health team's power (9). The use of prediction models for COVID-19 will support medical decision making but are still poorly reported (10). The decisions of easing the preventive measures in countries that passed the peak of transmission are complicated with the anticipation of a second wave that necessitates a sufficiently prepared action plan for handling cases on admission (11). Identifying clinical features or a scoring system to predict a benefit from hospital admission for patients with COVID-19 can be of great value for the decision-makers in the health sector.
Right now, there are few reliable, applicable, or useable clinical models or scoring systems to predict if a tested positive for COVID-19 should be admitted to the hospital or asked to stay home especially in regions like the middle east using local patients parameters for prediction (12). During the manuscript writing, a scoring system of COVID-19 (CSS) was suggested that could help clinicians to identify high-risk patients with poor prognosis (13). Another promising predictive tool PREDI-CO score was suggested to be useful in resource allocation and treatment prioritization during the COVID-19 pandemic (14). We aimed to identify differences in patients' demographic, clinical, laboratory, and radiological findings between mild, severe, and critical cases of COVID-19 positive cases to develop and validate a diagnostic model predicting who will develop severe form and who will need critical care throughout the course of the disease.
Materials and Methods
Patients Data Collection
In this observational retrospective study, COVID-19 positive patients (total 417) admitted between January to June 2020 were recruited from Al Kuwait Hospital, Dubai, UAE. Those patients are consecutive patients approaching the hospital for COVID-19 related symptoms and were enrolled with the following inclusion criteria: Adult patients (above 18 years) with COVID-19 (confirmed by nasopharyngeal polymerase chain reaction; PCR positive sample). Complete current and past medical history, along with their demographic data, a history of a recent travel or contact with a confirmed or suspected case were documented.
The cohort was divided into two subgroups, the training group to identify predictors of ICU admission (n = 128) who were admitted between January and February and the validation group (n = 289) who were admitted in March till June 2020. The study was approved by the Ministry of Health and Prevention (MOHAP) Research Ethics Committee number (MOHAP/DXB-REC/MMM/NO.44/2020). The main presenting symptoms were enlisted, including [fever, cough, fatigue, anorexia, shortness of breath (SOB), sputum production, myalgias, headache, confusion, rhinorrhea, sore throat, hemoptysis, vomiting, diarrhea, nausea, anosmia, and ageusia]. Risk factors for severe illness were examined, including old age, cardiovascular diseases (CVD), diabetes mellitus (DM), hypertension (HTN), prior stroke and or transient ischemic attack, cancer, chronic lung disease, and chronic kidney disease (CKD).
Patients Classification
Patients were classified according to “Clinical Management of Critically Ill COVID-19 Patients” guidelines (Version 1- April 15, 2020) issued by MOHAP (6). Accordingly, patients were classified into mild illness, pneumonia, and severe pneumonia (fever or suspected respiratory infection, plus one of the following: respiratory rate >30 breaths/min, severe respiratory distress, and SpO2 ≤ 93% on room air). Severe cases that needed oxygen therapy with no response to titrated oxygen therapy will require ICU treatment.
Criteria for ICU Admission
All patients were managed by same protocol and were evaluated by the same intensivists available during their admission period. Admission criteria for ICU followed the hospital policy ABH/CLN/033/V02, 2017, revised 2020. All physicians followed the same protocol. Severity at the time of admission (as per the criteria already defined): Severe disease: 9/39, and Critical disease: 30/39.
Blood and Radiological Tests
Laboratory tests were retrieved that include (1) complete blood count, including neutrophil count lymphocyte count, heamoglobin; Hb, white cell count; WCC, and platelets count, (2) coagulation profile, including interenational normalized ratio; INR, Prothrombin time; PT, (3) electrolytes, including sodium; Na and potassium; K, (4) renal function tests, including urea, creatinine, and estimated glomerular filtration rate; eGFR, (5) liver function tests, including total serum bilirubin, alanine aminotransferase; ALT, aspartate aminotransferase; AST, alkaline phosphatase; ALP, and albumin, (6) inflammatory markers, including C-reactive protein; CRP, D-dimers, lactate dehydrogenase; LDH, procalcitonin and ferritin. For risk of severe cases, the presence of lymphopenia, neutrophilia, high ALT/AST, high LDH, high CRP, high ferritin, high d-dimer, and high pro-calcitonin, above the age and gender-matched references were used as indicators of risk. Admission chest X-Ray (presence of bilateral air consolidation), and computerized tomography (CT) scan (presence of bilateral peripheral ground-glass opacities) were documented.
Statistical Analysis
For all statistical analyses and tests, SPSS was used (Released 2019. IBM SPSS Statistics for Windows, Version 26.0. Armonk, NY: IBM Corp). The Chi-Square Test of Independence was used to examine the association between categorical variables while student's t-test was used for continuous variables groups means comparison.
Patients were grouped into those that didn't need ICU and those who needed it. ROC Curve, Precision-Recall Curve, and Overall model quality options in SPSS were used to identify predictors of ICU admission and their cutoff values.
The normality test for all groups was done by Shapiro-Wilk tests using SPSS, and sig. of all independent variables> 0.05 means that all groups were normally distributed.
Results
Patients Needed ICU Were Older Men With Less Contact History
Training cohorts (n = 128) were divided into those who needed ICU (n = 39, 30.5%) “ICU” and those who didn't need ICU (n = 89, 69.5%) “No ICU.” Patients who needed ICU were older (57 ± 13 years old) than No ICU patients (44 ± 15 years old) (p = 0.0001). 36 (92.3%) of the ICU group were males compared to 66 (74.2%) in the No ICU group (p = 0.019). Documented contact history with a positive case of COVID-19 was more prevalent in the No ICU group (n = 26, 29.2%) compared to (n = 3, 7.7%) in ICU patients, as shown in Table 1. There was no difference in their BMI (28.42–27.49) or Duration of illness before approaching the hospital (5–7 ± 3 days).
Patients Needed ICU Presented More With SOB and Fever
Patients needed ICU admission presented more SOB (n = 29, 74.4%) than No ICU (n = 33, 37.1%), P < 0.001 and with fever (n = 30, 76.9%) compared to No ICU (n = 53, 59.6%), p = 0.05), as shown in Table 2.
Patients Needed ICU Showed Distinct Laboratory Findings
Patients needed ICU admission showed a higher Neutrophil count, WCC, Urea, creatinine, AST, CRP, D Dimer, LDH, Ferritin, and Troponin but lower Hb, eGFR, and albumin compared with No ICU patients, as shown in Table 3.
Patients Needed ICU Showed More CXR and Less CT Scan Opacities
Patients needed ICU admission showed more frequency of consolidations identified by chest X-Ray (n = 32, 82.1%) than No ICU group (n = 43, 57.3%), p = 0.001. Bilateral peripheral ground-glass opacities (GGO) documented by chest CT scan was higher in No ICU patients (n = 64, 71.9%) compared to (n = 23, 59.0%) in ICU patients, as shown in Table 4.
Patients Needed ICU Required Multiple In-patient Treatments
More patients needed ICU admission were treated with Lopinavir-ritonavir (n = 32, 82.1%); p = 0.05, Favipiravir (n = 19, 48.7%); p = 0.024, intravenous (IV) AB(n = 38, 97.4%); P < 0.001, IV steroids (n = 32, 100%); P < 0.001, Tocilizumab (n = 26, 66.7%); P < 0.001, and Antifungal (n = 10, 25.6%); P < 0.001 than No ICU patients, as shown in Table 5.
Patients Needed ICU Showed a Higher Rate of Clinical Complications and Death
Patients needed ICU admission developed more complications like Acute cardiac injury, Acute kidney injury, acute liver injury, Acidosis, Ventilated, Acute Respiratory Distress Syndrome (ARDS), Septic shock with higher mortality when compared to No ICU patients, as shown in Table 6.
High D Dimer, Troponin, and Urea Can Positively Predict the Admission to ICU in Patients With COVID-19
Probabilistic forecasts for binary classification (ICU vs. NO ICU) predictive modeling using ROC Curves and Precision-Recall curves showed that among all variables D dimers (>1.5 mg/dl), Urea (>6.5 mmol/L), and Troponin >13.5 ng/ml) can positively predict the admission to ICU in patients with COVID-19. On the other hand, decreased Lymphocyte count and albumin can predict admission to ICU in patients with COVID-19, as shown in Table 7 and Figure 1.
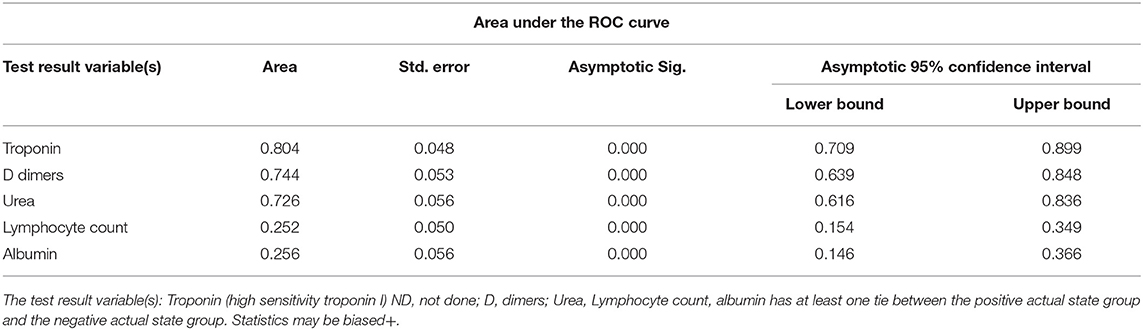
Table 7. Probabilistic forecasts for binary classification (ICU vs. NO ICU) predictive modeling using ROC Curves and Precision-Recall curves.
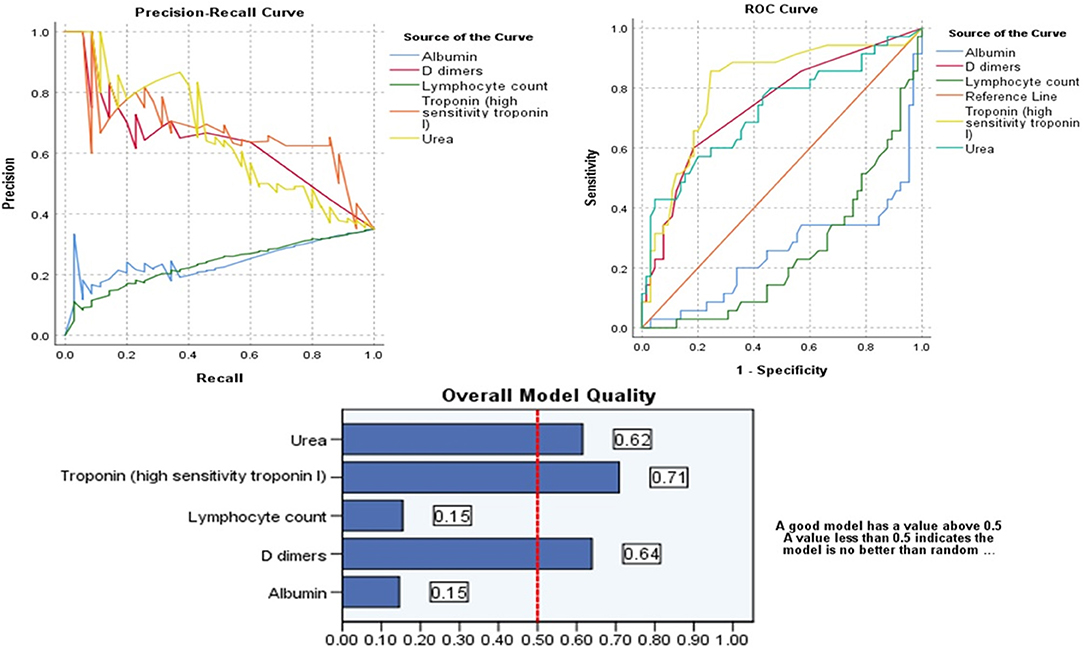
Figure 1. Probabilistic forecasts for binary classification (ICU vs. NO ICU) predictive modeling using ROC Curves, Precision-Recall curves of the significant parameters and Overall Model Quality.
Validation of the Three Predictors on a Larger Cohort of COVID-19 Patients
In order to validate the performance of these three markers in a larger cohort, we explored the Sensitivity and Specificity of using these parameters in the 289-validation cohort who attended the hospital between March and June 2020. Patients who showed D dimers (>1.5), Urea (>6.5 mmol/L), and Troponin >13.5 ng/ml were checked whether they would need ICU admission or not. Using the three markers gave low sensitivity (30.25%; 22.17–39.35%) but high specificity (93.10%; 88.26–96.39%) to predict ICU admission. Using any two of the three markers gave moderate sensitivity (59.32%, 49.89–68.27%) but high specificity (79.31%; 72.53–85.07%) to predict ICU admission. Using any one of the three markers gave high sensitivity (85.47%; 77.76–91.30%) but low specificity (45.98%; 38.41–53.68%) to predict ICU admission, as shown in Table 8.

Table 8. Validation of the three predictors on a larger cohort (n = 289) of COVID-19 patients suing to show different Sensitivity, Specificity, Positive Likelihood Ratio, and Negative Likelihood Ratio.
Discussion
Better understanding and identification of risk factors that might predispose for ICU admission might be essential for more active medical decision-making that might lead to optimal clinical practice to improve patients outcomes. Our results showed a significant difference in the pre-admission demographic, clinical, as well as laboratory characteristics of the ICU, admitted group when compared to non-admitted group.
Our study showed that patients needed ICU were older men with less contact history. Indeed, the association between ICU admission and elder age group was previously described in several reports that also showed the median age of critical/death groups to be higher than that of non-critical group groups (15–17). Older age and male sex were found to be independent risk factors for poor outcome of the illness in many reports (18). Specifically, older patients (>65 years) with comorbidities are at increased risk of death (19) where older age can independently predict the 60-day mortality after ICU admission (20). Such patients need careful observation and early intervention to prevent the potential development of severe COVID-19 (21).
On the other hand, disparities in the sex and gender observed in COVID-19 vulnerability was documented in many parts of the world (22). The majority of affected patients have been male who had more refractory disease and death (23). Gender differences in the COVID-19 outbreak should be taking into consideration in understanding the disease burden and dynamics of health emergency on individuals and communities (24). Male patients with heart injury, hyperglycemia, and high-dose corticosteroid were shown to have a high risk of death (21).
Our note that documented contact history with a positive case of COVID-19 was more prevalent in the No ICU group compared to ICU patients brings attention to the importance of the part of the asymptomatic carrier of the stories. Having contact with such asymptomatic carriers might be long enough to have more chances to present with the severe disease than the COVID-19 patients who came with known contact history as he will seek help earlier in the course of the disease. Asymptomatic carriers are prone to be mildly ill with the communicable period up to 3 weeks, and the communicated patients could develop severe illness (25).
Patients needed ICU admission presented more SOB and fever than No ICU patients. A systematic literature review and meta-analysis showed that among clinical manifestations, like fever, shortness of breath, or dyspnea, were associated with the progression of the disease (15). It is widely accepted that most of the COVID-19 patients will present with fever, cough, fatigue, and dyspnea, and fever is the most common symptom in patients with COVID-19 (26). Patients with severe COVID-19 are reported to have more silent hypoxemia, and coronavirus was suspected of having an idiosyncratic action on receptors involved in chemosensitivity to oxygen (27). Additionally, higher ferritin was higher in our cohort, which needed ICU admission compared to the No ICU group. Some reports document that high serum ferritin is a poor prognostic factor (28) as it represents a crucial mediator of immune dysregulation, contributing to the cytokine storm with fatal outcomes in COVID-19 (29).
Patients needed ICU admission showed a lower hemoglobin level compared with No ICU patients. So another explanation was linked to a hemoglobinopathy, hypoxia, and cell iron overload in COVID-19 patients due to direct SARS-CoV-2 interaction with hemoglobin molecule or hepcidin-mimetic action of a viral spike protein leading to an oxygen-deprived blood disease, with iron metabolism dysregulation (30). In a case study, recombinant human erythropoietin (rhEPO) was suggested to attenuate respiratory distress syndrome by enhancing leukocyte release from bone marrow and iron redistribution away from the intracellular virus (31).
Patients needed ICU admission showed a higher Neutrophil count and WCC compared with No ICU patients. Autopsy of COVID-19 patient's lungs showed infiltration of neutrophils with an aberrant neutrophil extracellular traps (NETs) formation that correlates with the clinical severity of COVID-19 (32). Enhanced neutrophil infiltration can induce necroinflammation that contributes to the higher mortality of COVID-19 in patients with underlying comorbidities (33). Recently, it was suggested that SARS-CoV2 could evade the innate immune response, causing uncontrolled NETs formation that leads to multi-organ failure (34).
Higher urea, creatine, and lower eGFR in ICU patients indicate the well-documented impact of COVID-19 on renal functions. Preexisting kidney disease on admission and/or the development of acute kidney injury (AKI) in patients with COVID-19 during hospitalization is high and is associated with in-hospital mortality (35). Usually, such AKI is resolved within 3 weeks after the onset of symptoms, but renal complications will lead specifically to higher mortality (36).
Patients needed ICU admission showed a higher AST and lowered albumin compared with No ICU patients that might indicate a hepatic injury. Digestive symptoms and liver injury have been reported during the course of the COVID-19 (37). It is well–known that patients with COVID-19 had liver comorbidities or reported abnormal levels of alanine aminotransferase and aspartate aminotransferase (AST) during disease progression (38). It is uncertain whether the COVID-19-related liver damage/dysfunction is caused by direct viral infection, as a consequence of the use of potentially hepatotoxic drugs, or as part of the multiple organ dysfunction in COVID-19 (39).
Patients needed ICU admission showed a higher C-reactive protein (CRP) and LDH compared with No ICU patients. This goes with the reports that found that the level of plasma CRP was positively correlated to the severity of COVID-19 pneumonia and can be useful as an earlier indicator for severe illness (40). In severe COVID-19 patients, CRP increased significantly before CT findings. Importantly, CRP, which was associated with disease development (41). Patients with positive RT-PCR had a significantly higher neutrophil count, CRP, lactate dehydrogenase (LDH), and Urea levels in serum (42). Serum LDH decline was shown to predict a favorable response to the treatment of COVID-19 infection (43).
Patients needed ICU admission showed more frequency of consolidations identified by chest X-Ray than the No ICU group. Bilateral peripheral GGO documented by chest CT scan was higher in No ICU patients compared to ICU patients. Chest CT is well-accepted as a standard method for the rapid diagnosis of COVID-19 (44). Typically COVID-19 pneumonia CT imaging abnormalities vary from focal unilateral to diffuse bilateral GGO that can be detected even in asymptomatic patients (45). Identification of GGO and a single lesion on the initial CT scan might suggest early-phase disease (46). So the lower incidence of GGO in the ICU group might indicate either it is not specific to severe cases or might indicate a false indication that the cases are not severe and might delay the actions needed.
Our results showed that patients who showed D dimers (>1.5 mg/dl), Urea (>6.5 mmol/L), and Troponin >13.5 ng/ml will have a higher chance of developing critical COVID-19 and will need ICU admission with higher complications and mortality. Using any two of the three markers gave moderate sensitivity (59.32%; 49.89–68.27%) but high specificity (79.31%; 72.53–85.07%) to predict ICU admission. High serum levels of D-Dimers and LDH in the absence of anticoagulation were associated with 1-month mortality among older inpatients with Covid-19 (47). Du et al. recently identified age ≥65 years, and cardiac troponin I ≥ 0.05 ng·mL-1 as two of four risk factors and predictors for mortality of COVID-19 pneumonia patients (48).
Using those bedside tests that can be done even outside the emergency department can save lives and resources by directing the service provider about the group of patients that might deteriorate and need admission to ICU. Directing such patients earlier to hospital with ICU might decrease the mortality and control the spread.
Conclusion
This demonstrated the accuracy of our approach in identifying factors that can predict the COVID 19 patient outcome. For that reason, the stratification of patients according to the parameters discovered by our model might provide a simple and efficient system for patients' risk stratification. This system might help clinicians and health care providers to deliver more efficient medical care for COVID 19 patients. Those factors, in addition to the fact that this report is the first report that uses in house patient cohort from UAE highlight the importance of implementation of such a method in the stratification of our own patients into high or low-risk groups for ICU transfer might be essential for more efficient use of our own resources and infrastructures available to deal with the COVID 19 outbreak.
Data Availability Statement
The original contributions presented in the study are included in the article/supplementary materials, further inquiries can be directed to the corresponding author/s.
Ethics Statement
The studies involving human participants were reviewed and approved by the Scientific Research Committee MOHAP/DXB-REC/MMM/NO.44/2020 and certify that the study was performed in accordance with the ethical standards as laid down in the 1964 Declaration of Helsinki and its later amendments ethical standards. Written informed consent for participation was not required for this study in accordance with the national legislation and the institutional requirements.
Author Contributions
All authors listed have made a substantial, direct and intellectual contribution to the work, and approved it for publication.
Conflict of Interest
The authors declare that the research was conducted in the absence of any commercial or financial relationships that could be construed as a potential conflict of interest.
Acknowledgments
We would like to thank all our patients for their patience. Also, we highly appreciate our colleagues and staff for their tremendous hard work during this crisis. In addition, we extend our gratitude to all staff at the Research Ethics Committee and the Information Technology Department for their help. This manuscript has been released as a pre-print at Research Square (49).
References
1. Wenham C, Smith J, Morgan R, Gender Group C-W. COVID-19: the gendered impacts of the outbreak. Lancet. (2020) 395:846–8. doi: 10.1016/S0140-6736(20)30526-2
2. Wu Z, McGoogan JM. Characteristics of and important lessons from the coronavirus disease 2019. (COVID-19) outbreak in china: summary of a report of 72314 cases from the chinese center for disease control and prevention. JAMA. (2020) 323:1239–42. doi: 10.1001/jama.2020.2648
3. Jin JM, Bai P, He W, Wu F, Liu XF, Han DM, et al. Gender differences in patients with covid-19: focus on severity and mortality. Front Public Health. (2020) 8:152. doi: 10.3389/fpubh.2020.00152
4. Grasselli G, Pesenti A, Cecconi M. Critical care utilization for the covid-19 outbreak in lombardy, italy: early experience and forecast during an emergency response. JAMA. (2020) 323:1545–6. doi: 10.1001/jama.2020.4031
5. Allenbach Y, Saadoun D, Maalouf G, Vieira M, Hellio A, Boddaert J, et al. Multivariable prediction model of intensive care unit transfer and death: a French prospective cohort study of COVID-19 patients. PLoS ONE. medRxiv. [Preprint]. (2020). doi: 10.1101/2020.05.04.20090118
6. Arabi YM, Murthy S, Webb S. COVID-19: a novel coronavirus and a novel challenge for critical care. Intensive Care Med. (2020) 46:833–6. doi: 10.1007/s00134-020-05955-1
7. Maves RC, Downar J, Dichter JR, Hick JL, Devereaux A, Geiling JA, et al. Triage of scarce critical care resources in COVID-19 an implementation guide for regional allocation: an expert panel report of the task force for mass critical care and the American college of chest physicians. Chest. (2020) 158:212–25. doi: 10.1016/j.chest.2020.03.063
8. Einav S, Hick JL, Hanfling D, Erstad BL, Toner ES, Branson RD, et al. Surge capacity logistics: care of the critically ill and injured during pandemics and disasters: CHEST consensus statement. Chest. (2014) 146(4 Suppl):e17S−43S. doi: 10.1378/chest.14-0734
9. Gong J, Ou J, Qiu X, Jie Y, Chen Y, Yuan L, et al. A tool to early predict severe 2019-novel coronavirus pneumonia (covid-19): a multicenter study using the risk nomogram in Wuhan and Guangdong, China. medRxiv. [Preprint]. (2020). doi: 10.1101/2020.03.17.20037515
10. Wynants L, Van Calster B, Bonten MMJ, Collins GS, Debray TPA, De Vos M, et al. Prediction models for diagnosis and prognosis of covid-19 infection: systematic review and critical appraisal. BMJ. (2020) 369:m1328. doi: 10.1101/2020.03.24.20041020
11. Ali I. COVID-19: are we ready for the second wave? Disaster Med Public Health Prep. (2020) 2020:1–3. doi: 10.1017/dmp.2020.149
12. Jiang X, Coffee M, Bari A, Wang J, Jiang X, Huang J, et al. Towards an artificial intelligence framework for data-driven prediction of coronavirus clinical severity. Comput Mater Continua. (2020) 63:537–51. doi: 10.32604/cmc.2020.010691
13. Shang Y, Liu T, Wei Y, Li J, Shao L, Liu M, et al. Scoring systems for predicting mortality for severe patients with COVID-19. EClinicalMedicine. (2020) 24:100426. doi: 10.1016/j.eclinm.2020.100426
14. Bartoletti M, Giannella M, Scudeller L, Tedeschi S, Rinaldi M, Bussini L, et al. Development and validation of a prediction model for severe respiratory failure in hospitalized patients with SARS-CoV-2 infection: a multicentre cohort study (PREDI-CO study). Clin Microbiol Infect. (2020) 26:1545–53. doi: 10.1016/j.cmi.2020.08.003
15. Zheng Z, Peng F, Xu B, Zhao J, Liu H, Peng J, et al. Risk factors of critical & mortal COVID-19 cases: a systematic literature review and meta-analysis. J Infect. (2020) 81:e16–25. doi: 10.1016/j.jinf.2020.04.021
16. Peng YD, Meng K, Guan HQ, Leng L, Zhu RR, Wang BY, et al. Clinical characteristics and outcomes of 112 cardiovascular disease patients infected by 2019-nCoV. Zhonghua Xin Xue Guan Bing Za Zhi. (2020) 48:E004. doi: 10.3760/cma.j.cn112148-20200220-00105
17. Tian S, Hu N, Lou J, Chen K, Kang X, Xiang Z, et al. Characteristics of COVID-19 infection in Beijing. J Infect. (2020) 80:401–6. doi: 10.1016/j.jinf.2020.02.018
18. Wang D, Yin Y, Hu C, Liu X, Zhang X, Zhou S, et al. Clinical course and outcome of 107 patients infected with the novel coronavirus, SARS-CoV-2, discharged from two hospitals in Wuhan, China. Crit Care. (2020) 24:188. doi: 10.1186/s13054-020-02895-6
19. Yang X, Yu Y, Xu J, Shu H, Xia J, Liu H, et al. Clinical course and outcomes of critically ill patients with SARS-CoV-2 pneumonia in Wuhan, China: a single-centered, retrospective, observational study. Lancet Respir Med. (2020) 8:475–81. doi: 10.1016/S2213-2600(20)30079-5
20. Xu J, Yang X, Yang L, Zou X, Wang Y, Wu Y, et al. Clinical course and predictors of 60-day mortality in 239 critically ill patients with COVID-19: a multicenter retrospective study from Wuhan, China. Crit Care. (2020) 24:394. doi: 10.1186/s13054-020-03098-9
21. Li X, Xu S, Yu M, Wang K, Tao Y, Zhou Y, et al. Risk factors for severity and mortality in adult COVID-19 inpatients in Wuhan. J Allergy Clin Immunol. (2020) 146:110–8. doi: 10.1016/j.jaci.2020.04.006
22. Gebhard C, Regitz-Zagrosek V, Neuhauser HK, Morgan R, Klein SL. Impact of sex and gender on COVID-19 outcomes in Europe. Biol Sex Differ. (2020) 11:29. doi: 10.1186/s13293-020-00304-9
23. Walter LA, McGregor AJ. Sex- and Gender-specific Observations and Implications for COVID-19. West J Emerg Med. (2020) 21:507–9. doi: 10.5811/westjem.2020.4.47536
24. Ambrosino I, Barbagelata E, Ortona E, Ruggieri A, Massiah G, Giannico OV, et al. Gender differences in patients with COVID-19: a narrative review. Monaldi Arch Chest Dis. (2020) 90:318–24. doi: 10.4081/monaldi.2020.1389
25. Hu Z, Song C, Xu C, Jin G, Chen Y, Xu X, et al. Clinical characteristics of 24 asymptomatic infections with COVID-19 screened among close contacts in Nanjing, China. Sci China Life Sci. (2020) 63:706–11. doi: 10.1007/s11427-020-1661-4
26. Hu Y, Sun J, Dai Z, Deng H, Li X, Huang Q, et al. Prevalence and severity of corona virus disease. 2019 (COVID-19): a systematic review and meta-analysis. J Clin Virol. (2020). 127:104371. doi: 10.1016/j.jcv.2020.104371
27. Tobin MJ, Laghi F, Jubran A. Why COVID-19 silent hypoxemia is baffling to physicians. Am J Respir Crit Care Med. (2020) 202:356–60. doi: 10.1164/rccm.202006-2157CP
28. Terpos E, Ntanasis-Stathopoulos I, Elalamy I, Kastritis E, Sergentanis TN, Politou M, et al. Hematological findings and complications of COVID-19. Am J Hematol. (2020) 95:834–47. doi: 10.1002/ajh.25829
29. Vargas-Vargas M, Cortés-Rojo C. Ferritin levels and COVID-19. Rev Panam Salud Publica. (2020) 44:e72. doi: 10.26633/RPSP.2020.72
30. Cavezzi A, Troiani E, Corrao S. COVID-19: hemoglobin, iron, and hypoxia beyond inflammation. a narrative review. Clin Pract. (2020) 10:1271. doi: 10.4081/cp.2020.1271
31. Hadadi A, Mortezazadeh M, Kolahdouzan K, Alavian G. Does recombinant human erythropoietin administration in critically ill COVID-19 patients have miraculous therapeutic effects? J Med Virol. (2020) 92:915–8. doi: 10.1002/jmv.25839
32. Barnes BJ, Adrover JM, Baxter-Stoltzfus A, Borczuk A, Cools-Lartigue J, Crawford JM, et al. Targeting potential drivers of COVID-19: Neutrophil extracellular traps. J Exp Med. (2020) 217:e20200652. doi: 10.1084/jem.20200652
33. Tomar B, Anders HJ, Desai J, Mulay SR. Neutrophils and neutrophil extracellular traps drive necroinflammation in COVID-19. Cells. (2020) 9:1383. doi: 10.3390/cells9061383
34. Thierry AR, Roch B. SARS-CoV2 may evade innate immune response, causing uncontrolled neutrophil extracellular traps formation and multi-organ failure. Clin Sci. (2020) 134:1295–300. doi: 10.1042/CS20200531
35. Cheng Y, Luo R, Wang K, Zhang M, Wang Z, Dong L, et al. Kidney disease is associated with in-hospital death of patients with COVID-19. Kidney Int. (2020) 97:829–38. doi: 10.1016/j.kint.2020.03.005
36. Pei G, Zhang Z, Peng J, Liu L, Zhang C, Yu C, et al. Renal involvement and early prognosis in patients with COVID-19 pneumonia. J Am Soc Nephrol. (2020) 31:1157–65. doi: 10.1681/ASN.2020030276
37. Lee IC, Huo TI, Huang YH. Gastrointestinal and liver manifestations in patients with COVID-19. J Chin Med Assoc. (2020) 83:521–3. doi: 10.1097/JCMA.0000000000000319
38. Zhang C, Shi L, Wang F-S. Liver injury in COVID-19: management and challenges. Lancet Gastroenterol Hepatol. (2020) 5:428–30. doi: 10.1016/S2468-1253(20)30057-1
39. Feng G, Zheng KI, Yan QQ, Rios RS, Targher G, Byrne CD, et al. COVID-19 and liver dysfunction: current insights and emergent therapeutic strategies. J Clin Transl Hepatol. (2020) 8:18–24. doi: 10.14218/JCTH.2020.00018
40. Chen W, Zheng KI, Liu S, Yan Z, Xu C, Qiao Z. Plasma CRP level is positively associated with the severity of COVID-19. Ann Clin Microbiol Antimicrob. (2020) 19:18. doi: 10.1186/s12941-020-00362-2
41. Tan C, Huang Y, Shi F, Tan K, Ma Q, Chen Y, et al. C-reactive protein correlates with computed tomographic findings and predicts severe COVID-19 early. J Med Virol. (2020) 92:856–862. doi: 10.1002/jmv.25871
42. Mardani R, Ahmadi Vasmehjani A, Zali F, Gholami A, Mousavi Nasab SD, Kaghazian H, et al. Laboratory parameters in detection of covid-19 patients with positive rt-pcr; a diagnostic accuracy study. Arch Acad Emerg Med. (2020) 8:e43.
43. Yuan J, Zou R, Zeng L, Kou S, Lan J, Li X, et al. The correlation between viral clearance and biochemical outcomes of 94 COVID-19 infected discharged patients. Inflamm Res. (2020) 69:599–606. doi: 10.1007/s00011-020-01342-0
44. Li Y, Xia L. Coronavirus Disease (2019). (COVID-19): role of chest CT in DIAGNOSIS AND MANAGEMENT. AJR Am J Roentgenol. (2020) 214:1280–6. doi: 10.2214/AJR.20.22954
45. Shi H, Han X, Jiang N, Cao Y, Alwalid O, Gu J, et al. Radiological findings from 81 patients with COVID-19 pneumonia in Wuhan, China: a descriptive study. Lancet Infect Dis. (2020) 20:425–34. doi: 10.1016/S1473-3099(20)30086-4
46. Zhou S, Wang Y, Zhu T, Xia L. CT features of coronavirus disease 2019. (COVID-19) pneumonia in 62 patients in Wuhan, China. AJR Am J Roentgenol. (2020) 214:1287–94. doi: 10.2214/AJR.20.22975
47. Bousquet G, Falgarone G, Deutsch D, Derolez S, Lopez-Sublet M, Goudot FX, et al. ADL-dependency, D-Dimers, LDH and absence of anticoagulation are independently associated with one-month mortality in older inpatients with Covid-19. Aging. (2020) 12:11306–13. doi: 10.18632/aging.103583
48. Du RH, Liang LR, Yang CQ, Wang W, Cao TZ, Li M, et al. Predictors of mortality for patients with COVID-19 pneumonia caused by SARS-CoV-2: a prospective cohort study. Eur Respir J. (2020) 55:2000524. doi: 10.1183/13993003.00524-2020
Keywords: COVID-19, severe COVID-19 prediction, risk stratification, ICU - Intensive care unit, SARS-CoV-2
Citation: Hachim MY, Hachim IY, Naeem KB, Hannawi H, Salmi IA and Hannawi S (2020) D-dimer, Troponin, and Urea Level at Presentation With COVID-19 can Predict ICU Admission: A Single Centered Study. Front. Med. 7:585003. doi: 10.3389/fmed.2020.585003
Received: 19 July 2020; Accepted: 17 November 2020;
Published: 09 December 2020.
Edited by:
Ru-Ping Dai, Central South University, ChinaReviewed by:
Tommaso Tonetti, University of Bologna, ItalyAbele Donati, Marche Polytechnic University, Italy
Copyright © 2020 Hachim, Hachim, Naeem, Hannawi, Salmi and Hannawi. This is an open-access article distributed under the terms of the Creative Commons Attribution License (CC BY). The use, distribution or reproduction in other forums is permitted, provided the original author(s) and the copyright owner(s) are credited and that the original publication in this journal is cited, in accordance with accepted academic practice. No use, distribution or reproduction is permitted which does not comply with these terms.
*Correspondence: Suad Hannawi, c3VhZDFAYXVzZG9jdG9ycy5uZXQ=
†These authors have contributed equally to this work
‡ORCID: Mahmood Y. Hachim orcid.org/0000-0002-7096-0731
Ibrahim Y. Hachim orcid.org/0000-0001-6438-9455
Issa Al Salmi orcid.org/0000-0002-3443-5972
Suad Hannawi orcid.org/0000-0002-5689-6710