Corrigendum: Evaluation of Wearable Technology in Dementia: A Systematic Review and Meta-Analysis
- 1Department of Anatomy and Neurobiology, Boston University Medical Center, Boston, MA, United States
- 2Department of Genetics and Genomic Sciences, Icahn School of Medicine at Mount Sinai, New York, NY, United States
- 3Division of Geriatric Medicine, Department of Medicine, Western University, London, ON, Canada
Background: The objective of this analysis was to systematically review studies employing wearable technology in patients with dementia by quantifying differences in digitally captured physiological endpoints.
Methods: This systematic review and meta-analysis was based on web searches of Cochrane Database, PsycInfo, Pubmed, Embase, and IEEE between October 25–31st, 2017. Observational studies providing physiological data measured by wearable technology on participants with dementia with a mean age ≥50. Data were extracted according to PRISMA guidelines and methodological quality assessed independently using Downs and Black criteria. Standardized mean differences between cases and controls were estimated using random-effects models.
Results: Forty-eight studies from 18,456 screened abstracts (Dementia: n = 2,516, Control: n = 1,224) met inclusion criteria for the systematic review. Nineteen of these studies were included in one or multiple meta-analyses (Dementia: n = 617, Control: n = 406). Participants with dementia demonstrated lower levels of daily activity (standardized mean difference (SMD), −1.60; 95% CI, −2.66 to −0.55), decreased sleep efficiency (SMD, −0.52; 95% CI, −0.89 to −0.16), and greater intradaily circadian variability (SMD, 0.46; 95% CI, 0.27 to 0.65) than controls, among other measures. Statistical between-study heterogeneity was observed, possibly due to variation in testing duration, device type or patient setting.
Conclusions and Relevance: Digitally captured data using wearable devices revealed that adults with dementia were less active, demonstrated increased fragmentation of their sleep-wake cycle and a loss of typical diurnal variation in circadian rhythm as compared to controls.
Introduction and Background
Dementia has been identified by the World Health Organization as a global priority for public health and social care in the twenty-first century (1). Advances in the molecular and genetic understanding of neurodegenerative disease has contributed to improved diagnostic paradigms and helped to foster a new era of personalized medicine for patients with dementia. This has coincided with the advancement in biological drug development for targeted therapies. These therapies have reflected the maturation in the scientific understanding of dementia that goes beyond raw measurement of cognitive performance. As a case in point a recent review of active clinical trials had shown that 14 biological treatments have targeted neuropsychiatric and behavioral symptoms as primary end-points. Challenges remain in capturing the heterogeneity of the clinical course experienced by individuals with dementia and translating these into meaningful end-points.
Technological advances using accelerometers, gyroscopes, and other motion detectors housed in mobile platforms may eventually present a cost-effective way to measure disease burden and deploy personalized treatments (2). Wearable devices that can continuously monitor physiological measures over extended periods, for example in the patient's home, provide unique information not attainable with traditional in-clinic monitoring and hold particular appeal in dementia populations (3). Advances in technology have made these devices increasingly affordable and user friendly but have been limited by methodological challenges. Specifically, their high resolution and sensitivity leaves them susceptible to noisy interference, complicated and time-consuming analytical techniques are required to derive clinically meaningful endpoints from the large amounts of data they produce, and the lack of standards has led to isolated “islands of expertise” (4).
The flexibility of wearable platforms has resulted in a variety of different uses including monitoring of gait, motion tracking, and sleep and circadian rhythm assessment (5). The ability to identify objective measurements of specific endpoints with respect to individual and group-wise subject performance, captured in real-time at various settings including at home, provides ecological validity that would otherwise be lost in laboratory settings. The main question that we had aimed to address was the potential for wearable devices to provide information on the behavioral and neuropsychiatric fluctuations inherent in the clinical course of dementia. The ability to accurately and objectively measure these fluctuations can provide researchers with viable digital surrogate end-points for use in clinical trials. We undertook a systematic review and meta-analysis to evaluate the utility of wearable technology in patients with dementia for the measurement of these neurophysiological parameters. The objective of this analysis was to systematically review studies employing wearable technology in patients with dementia by quantifying differences in digitally captured neurophysiological endpoints.
Literature Selection Criteria
Data Sources and Search Strategy
Five electronic databases were searched including Cochrane, EMBASE, PubMed, PsycInfo, and IEEE. Searches were performed for Cochrane, PsycInfo, and IEEE on October 31, 2017. A PubMed search was performed on October 25, 2017, and an Embase search on October 27, 2017. A combination of Medical Subject Headings and search terms were constructed by the authors (RP, AC, and JB) in collaboration with a librarian. The Search Terms provides an outline of the search strategy for PubMed only.
Search Terms. Systematic Review Search Strategy: PubMed
1. “Dementia”[Mesh]
2. dementia
3. (frontotemporal dementia)
4. (vascular dementia)
5. (Alzheimer* disease)
6. (Parkinson* disease)
7. (lewy body)
8. Creutzfeldt-Jakob
9. 1 or 2 or 3 or 4 or 5 or 6 or 7 or 8
10. “Technology”[Mesh]
11. (wearable device)
12. (assistive technology)
13. (wearable technology)
14. on-body
15. bracelet
16. GPS
17. actigraphy
18. accelerometer
19. (galvanic skin response)
20. biosensor
21. sensor
22. gyroscope
23. watch
24. necklace
25. harness
26. strap
27. patch
28. camera
29. chip
30. (step counter)
31. pouch
32. armband
33. node
34. 10 or 11 or 12 or 13 or 14 or 15 or 16 or 17 or 18 or 19 or 20 or 21 or 22 or 23 or 24 or 25 or 26 or 27 or 28 or 29 or 30 or 31 or 32 or 33
35. 9 and 3.
Types of Studies
We included observational studies reporting primary data in a peer-reviewed scientific journal. Studies had to include participants with a mean age ≥50 years and did not include any direct intervention (i.e., drug, vitamin, supplement, exercise, cognitive, or behavioral intervention). Studies published before 1970 or translated to English were excluded. Studies that did not provide descriptive statistics for a physiological outcome were excluded. Conference abstracts, review papers, case reports, letters, opinion pieces, editorials, article comments, or corrections were excluded.
Type of Exposure
We included all-cause dementia (any dementia subtype) as our exposure (6). Exact search terms for dementia subtypes included can be found in the search strategy (Search Terms). As we included studies from 1970 onwards, diagnostic criteria for the diagnosis of dementia differed between studies and is summarized for each study in Table 1.
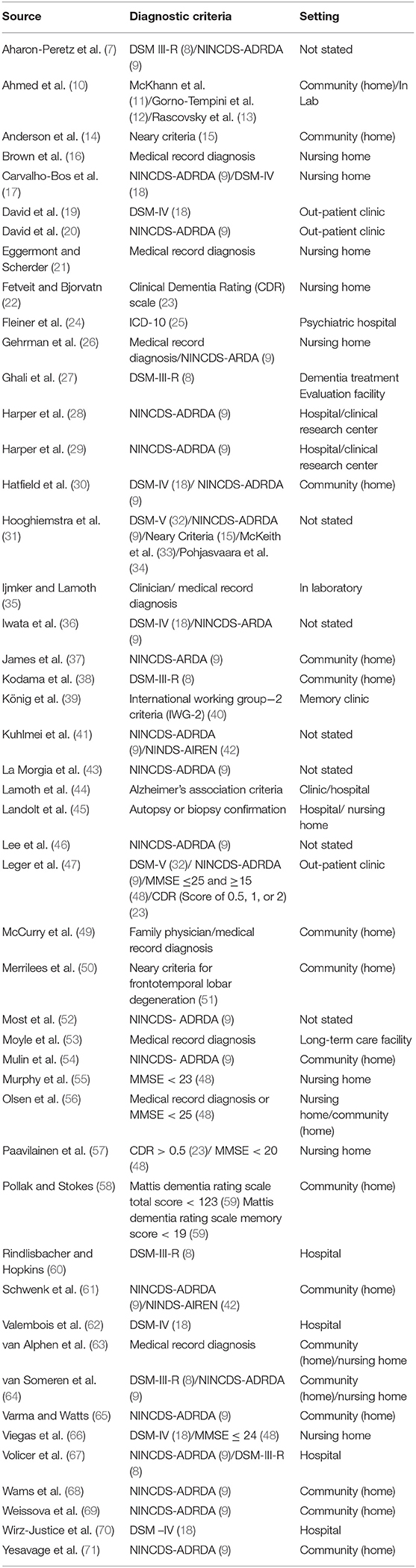
Table 1. Patient setting and diagnostic criteria for 48 observational studies testing wearable technology in participants with dementia.
Types of Outcome Measures
We included studies which provided physiological data as measured by wearable technology. Wearable technology was defined as a non-implantable, body-fixed sensor technology designed to monitor for >24 h and to not interfere with the wearer's normal activity (5, 72). By this definition, studies using finger-based pulse oximeters, blood pressure monitors, galvanic skin response sensors, functional near-infrared spectroscopy (fNIRS), and electroencephalograms (EEG) were excluded. Where studies included measurement devices other than a wearable device, only data from the wearable device was included in the final analysis.
Methods for Literature Secondary Screening
First Selection: Abstract Screening
Two authors (RP and AC) independently screened each record by title and abstract according to eligibility criteria. Eighteen thousand four hundred fifty-six abstracts were included in the initial screening process. There were 525 disagreements in abstract selection between the two reviewers. Conflicts were resolved by two additional authors (NS and JB) using the inclusion and exclusion criteria and definitions outlined in Figure 1.
Final Selection
Two hundred thirty articles were eligible for full text review. Two authors (RP and AC) independently determined eligibility of each article for inclusion. In cases of disagreement or conflict, senior authors (NS, JB, and KT) determined whether the study met eligibility criteria. Forty-eight articles were included in the final systematic review.
Data Collection
Data was extracted by three authors (JB, RP, and AC). Information extracted from each publication is provided in Table 6. To assess the methodological quality of included studies, we used the checklist provided by Downs and Black (73). A total quality score is provided for each study in Table 6 (maximum score = 32).
Statistical Analyses
Data analysis was performed using Stata/SE (StataCorp LP, Texas, Version 15). The age and number of included participants per study, as well as general study results are provided in Table 6. Initial synthesis of qualitative data revealed a number of common endpoints reported consistently by authors. Subsequent meta-analyses included only observational case-control or cross-sectional studies that presented data for these commonly reported endpoints (Table 7).
Meta-analyses were conducted using the standardized mean difference (Hedges' g). Hedges' g values ≤0.20, >0.20 but <0.80, and ≥0.80 were considered small, moderate, or large, respectively between controls and participants with dementia (74). For each single or combined effect size, a positive value indicated a higher mean value of that variable in participants with dementia than in healthy volunteers. Some publications contained two subgroups of dementia participants. A fixed effects meta-analysis was performed on the dementia subgroups within each of these studies to compute a composite effect size and variance (75). This composite effect was used in the across-study random effects analysis.
Across-study heterogeneity was investigated using the Cochran's Q-test and I2 statistic. Cochran's Q test was performed using the weighted method of moments method (75). Cochran's Q statistic was considered significant at p < 0.10. I2-values of 25, 50, and 75% were considered indicative of low, moderate, and high heterogeneity, respectively (76).
For each analysis, a funnel plot of standardized mean differences was constructed, and the risk of publication bias evaluated through funnel plot asymmetry and Egger tests. We acknowledge that many other factors including heterogeneity, differences in methodological quality, and selective reporting may produce funnel plot asymmetry (77).
The influence of each study on a meta-analysis estimate was investigated through influence analysis, where each individual study is omitted in turn and the meta-analysis re-estimated using a random effects model. For publications that included more than one subgroup of participants with dementia, the largest subgroup was included in the influence analysis.
There was no funding source for this study and the corresponding author had full access to all of the data in the study and had final responsibility for the decision to submit for publication.
Results
Systematic Review
Five database searches resulted in 18,456 retrieved abstracts after removal of duplicates (Figure 1). Two hundred thirty of these publications qualified for full-text screening after examination by title and abstract. Forty-eight studies qualified for inclusion in the final qualitative analysis and 19 of these publications qualified for inclusion in one or multiple meta-analyses (Dementia: n = 617, Control: n = 406) (Table 7).
Nineteen studies (39%) enrolled participants only with ad-related diagnoses. Table 2 describes the technical specifications of devices used in individual studies. Thirty-four studies (70%) tested participants using a wrist-worn actigraph. The average assigned duration of wear was 8.26 days (range: 6 min−28 days). Forty (83%) studies used accelerometry as the main measurement of activity. One study used an accelerometer with a gyroscope, while one further study used an accelerometer, gyroscope and magnetometer. Six studies (12%) used activity monitors which did not state the type of measurement modality.
Daily Activity
Of the 48 included studies, 23 (47%) groups reported outcome data on daily activity counts as measured by actigraphy. Qualitative analysis showed that activity counts were presented in a number of different ways (Table 3). Significant associations of activity counts with other measures, or differences in activity between individuals with dementia and control groups, were reported for the measures of daily activity (eight groups, 34%), peak daily activity (two groups, 8%), mean activity counts (five groups, 21%), daytime activity (five groups, 21%), night time activity (one group, 4%), number of immobile hours (one group, 4%), and activity patterns (three groups, 13%). Quantitative analysis demonstrated that participants with dementia had a significantly lower mean daytime activity counts compared to controls (mean difference, −1.60; 95% CI, −2.66 to −0.55) (Dementia: n = 210, Control: n = 136) (Figure 2I).
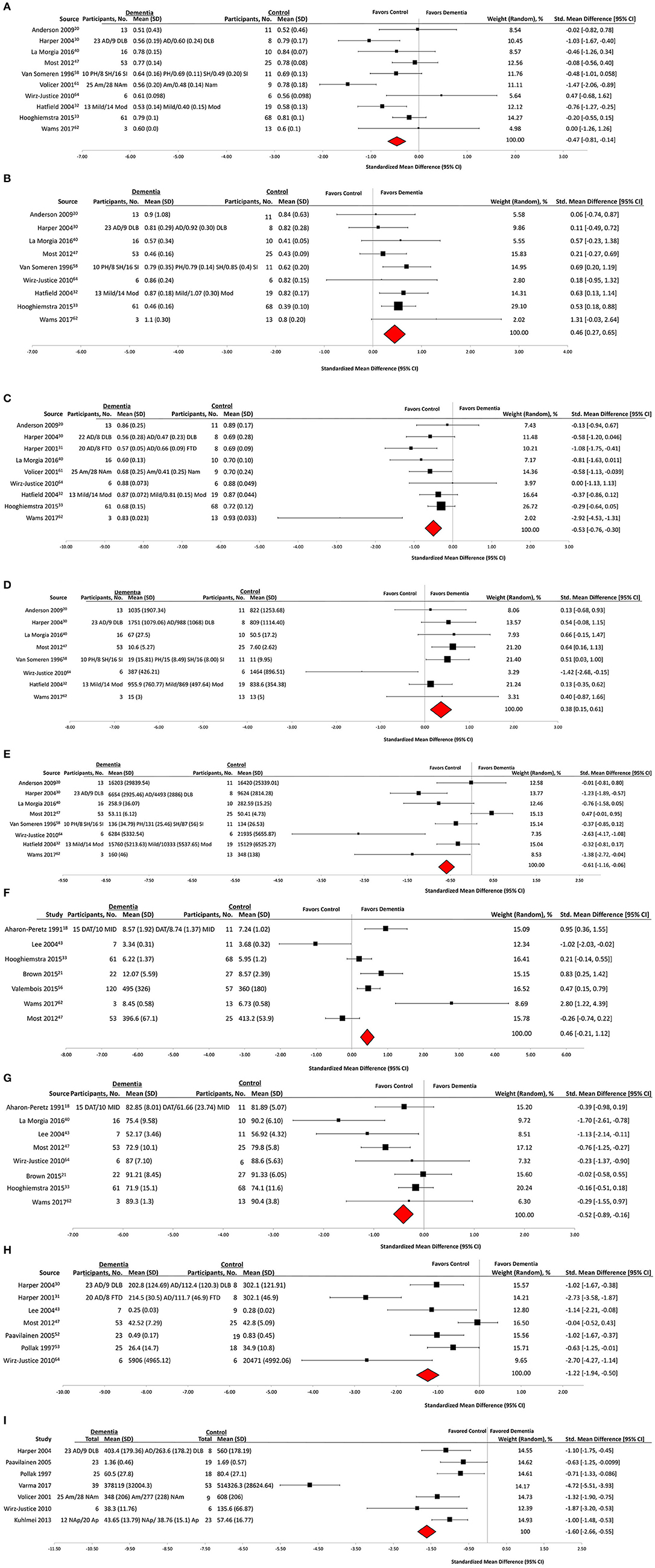
Figure 2. Actigraphy outcomes in observational case control studies of wearable technology. (A) Interdaily stability, (B) interdaily variability, (C) relative amplitude, (D) activity of least active 5 h, (E) Activity of most active 10 h, (F) total sleep time, (G) sleep efficiency, (H) amplitude, and (I) daytime activity.
Wearable Actigraphy for Sleep Derived Measures
Of the 48 included studies, 31 (64%) groups reported outcome data on sleep characteristics as measured by actigraphy (Table 4). Wake after sleep onset (WASO) was reported by 11 groups: six (55%) reported a significant association or difference in dementia subjects. Total sleep time (TST) was reported by 16 groups: nine (56%) reported a significant association or difference in dementia subjects. Sleep efficiency (SE) was reported by 12 groups: five (42%) reported a significant association or difference in dementia subjects. Participants with dementia had statistically significant lower mean sleep efficiency than controls (mean difference, −0.52; 95% CI, −0.89 to −0.16) (Dementia: n = 193, Control: n = 171) (Figure 2G), and no significant difference in mean total sleep time (mean difference, 0.46; 95% CI, −0.21 to 1.12) (Dementia: n = 291, Control: n = 212) (Figure 2F).
Non-parametric Measurements of Circadian Rhythm Using Wearable Devices
Sixteen (33%) of 48 studies reported non-parametric measurements of circadian rhythm (Table 4). Qualitative analysis revealed that intradaily variability (IV) was reported by 13 groups: eight (61%) reported an association or difference in dementia groups. Interdaily stability (IS) was reported by 14 groups: nine (64%) reported an association or difference in dementia subjects. Relative amplitude (RA) was reported by 12 groups: seven (58%) reported an association or difference in dementia subjects. Activity of most active 10 h (M10) was reported by nine groups: seven (77%) reported an association or difference in dementia subjects. Activity of least active 5 h (L5) was reported by eight groups: four (50%) reported an association or difference in dementia groups.
Participants with dementia had significantly lower mean values than controls on IS (mean difference, −0.47; 95% CI, −0.81 to −0·14) (Dementia: n = 298, Control: n = 180) (Figure 2A), RA (mean difference, −0.53; 95% CI, −0.76 to −0.30) (Dementia: n = 237, Control: n = 152) (Figure 2C), and M10 (mean difference, −0.61; 95% CI, −1.16 to −0.06) (Dementia: n = 184, Control: n = 103) (Figure 2E) outcomes. Participants with dementia had statistically significantly higher mean values than controls on IV (mean difference, 0.46; 95% CI, 0.27–0.65) (Dementia: n = 245, Control: n = 171) (Figure 2B) and L5 (mean difference, 0.38; 95% CI, 0.15–0.61) (Dementia: n = 184, Control: n = 103) (Figure 2D) outcomes.
Cosinor Analysis of Circadian Rhythm Using Wearable Devices
Nine (19%) out of the 48 groups reported a cosinor analysis of circadian rhythm. Qualitative analysis (Table 4) showed that midline estimating statistic of rhythm (mesor) was reported by five groups: two (40%) reported a significant association or difference in dementia subjects. Amplitude of the cosinor wave was reported by 10 groups: five (50%) reported an association or difference in dementia subjects. Acrophase was reported by eight groups: two (25%) reported an association or difference in dementia subjects. Quantitative analysis was only performed on the amplitude of the cosinor wave. It revealed that subjects with dementia had a significantly lower mean amplitude than controls (mean difference, −1.22; 95% CI, −1.94 to −0.50) (Dementia: n = 174, Control: n = 93) (Figure 2H).
Wearable Actigraphy for Gait Derived Measures
Of the 48 included studies six (12%) groups reported outcome data on actigraphy to measure posture and gait characteristics (Table 5). Qualitative analysis showed that all six (100%) reported an association or difference in dementia subjects. These studies each reported a different measure of gait or walking activity, and thus a meta-analysis was not possible.
Risk of Bias Within Studies
Average rating of methodological quality of included studies was 15·54 points (SD = 1·47). The median and mode were both 16 points, with a range of 12–18 (Table 6).
Meta-Analysis and Heterogeneity
Low between study heterogeneity (I2 < 50%) was observed for analyses of IV, RA, and L5 variables (Table 7). Moderate to high between study heterogeneity (I2 > 50%) was observed for analyses of IS, TST, amplitude, M10, SE, and daytime activity. Meta-regression or subgroup analyses were performed for all actigraphy measures with a moderate to high heterogeneity (I2 > 50%) which included IS, TST, Amplitude, M10, SE, and daytime activity. Type of dementia, mean age, study design and quality score were all investigated as explanatory variables. Subgroup analyses indicate that effect estimates vary markedly between dementia subtypes for variables M10 and SE, suggesting differences in dementia type between studies may account for some of the heterogeneity observed in meta-analyses of M10 and SE measurements.
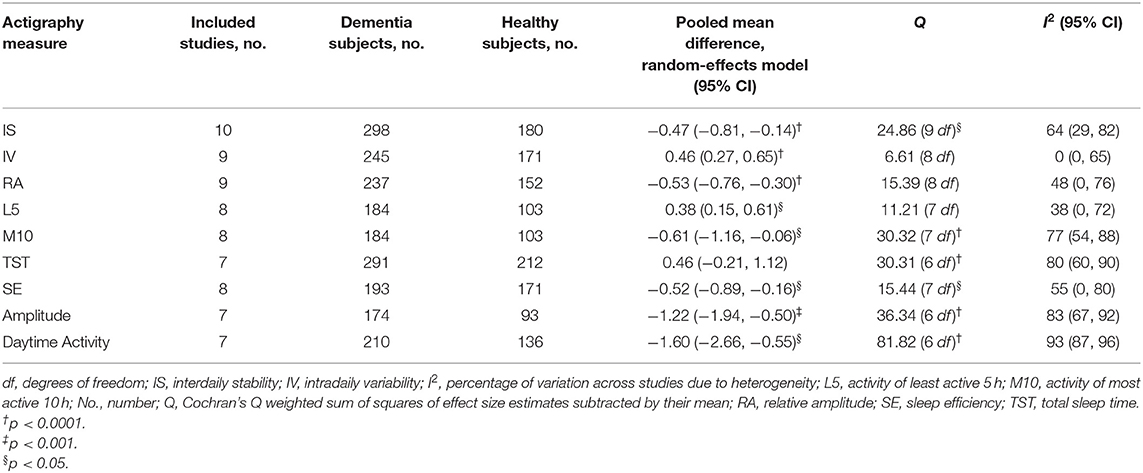
Table 7. Combined effect estimates and heterogeneity for actigraphy outcomes between dementia and control samples.
Risk of Publication Bias Across Studies for Meta-Analysis
Funnel plots for each variable investigated using random effects meta-analysis are provided in Figure 3. These plots were constructed with a measure of study size on the x-axis and a measure of effect size on the y-axis. Dashed lines represent the pseudo 95 and 99.7% confidence limits about the effect estimate (solid line). Funnel plot asymmetry was observed for all but two variables (IV and RA), and significant Egger tests observed for M10 (p = 0.0057) and amplitude variables (p = 0.0078), suggesting evidence of publication bias for these measurements.
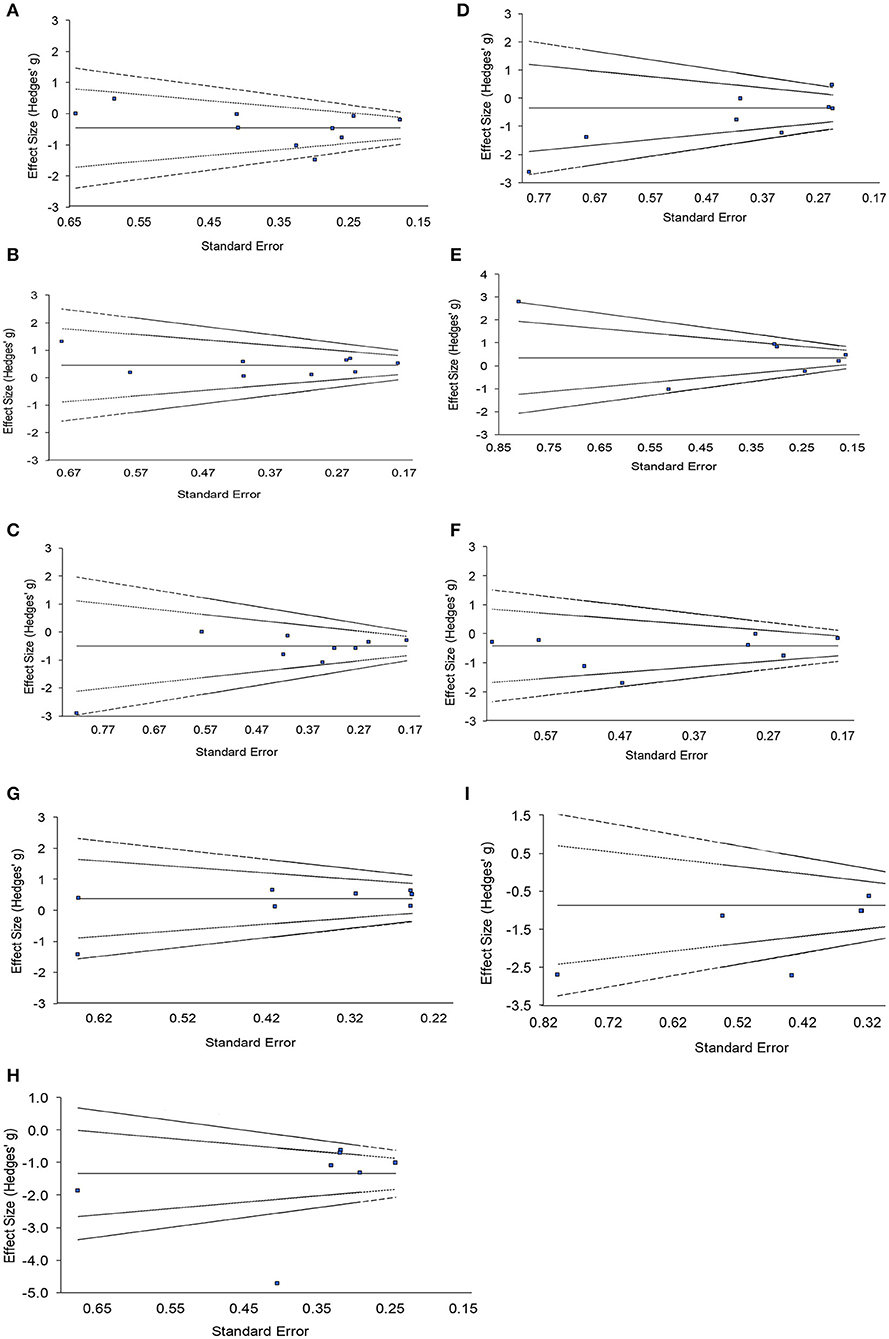
Figure 3. Funnel plots with pseudo 95 and 99.7% confidence intervals assessing publication bias of included studies for nine actigraphy measures. (A) Interdaily stability, (B) intradaily variability, (C) relative amplitude, (D) activity of most active 10h, (E) total sleep time, (F) sleep efficiency, (G) activity of least active 5h, (H) daytime activity, (I) amplitude.
Investigation of Influential Studies
The impact of each study on a meta-analysis estimate was investigated through influence analysis. Influence analysis shows that meta-analysis estimates are generally robust (Figure 4), excluding meta-analysis of daytime activity, where the pooled estimate decreases in magnitude markedly and precision of the estimate improves with exclusion of Varma and Watts (65). Even with exclusion of this influential study, the pooled estimate remains significant and shows the same direction of effect as in the full meta-analysis.
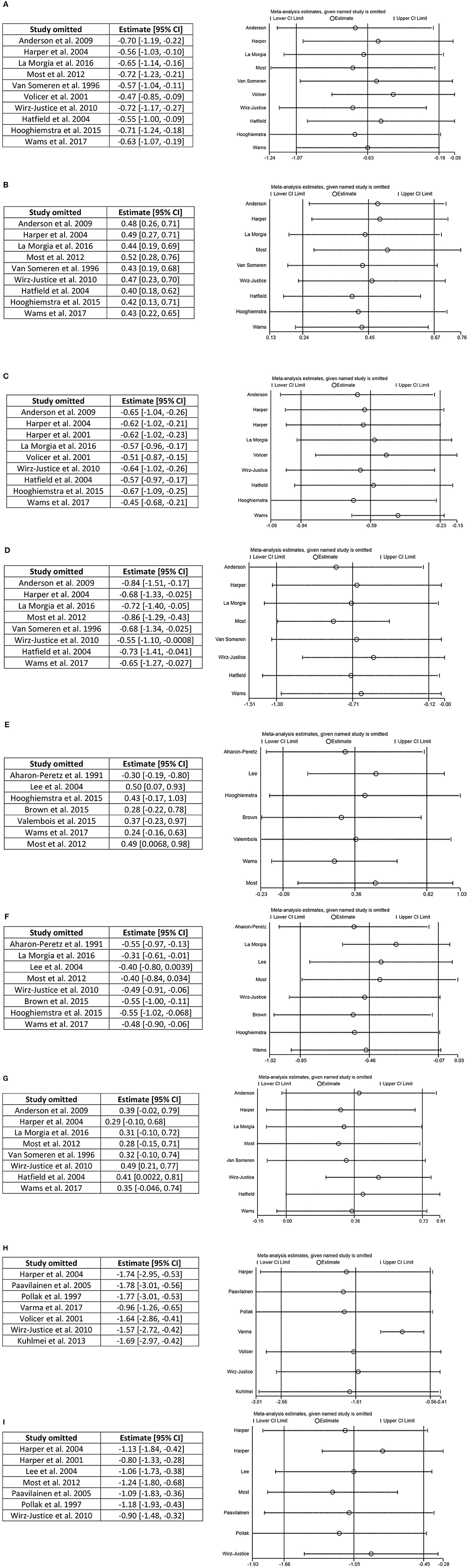
Figure 4. Influence analysis. (A) Interdaily stability, (B) intradaily variability, (C) relative amplitude, (D) activity of most active 10 h, (E) total sleep time, (F) sleep efficiency, (G) activity of least active 5 h, (H) daytime activity, and (I) amplitude.
Discussion
From our systematic review of the literature we found 48 articles which met our inclusion criteria of wearable technology use in patients with dementia for the measurement of physiological parameters. Wearable devices were utilized most extensively to measure circadian rhythm, measurement of the sleep wake cycle and daily activity. In the studies which were analyzed using forest plots, groups of participants with dementia were less active then controls, had a difference in their sleep wake cycle and showed differences in their circadian rhythms when compared to control groups. To our knowledge, this study is the first systematic review and meta-analysis of wearable device testing in participants with dementia.
Wearable Devices to Measure Sleep and Circadian Rhythm
The use of actigraphy to measure sleep was the most commonly reported outcome. Participants with dementia demonstrated reduced sleep efficiency as compared to controls. There was also a significant difference between individuals with dementia and controls on non-parametric measures of circadian rhythm including IV, IS, and RA, however it should be noted that for some measures the combined effects were substantially weighted by the results of Hooghiemstra et al. (31). Meta-analysis of the amplitude measure of circadian rhythm cosinor analysis also demonstrated a moderate but statistically significant difference between groups. Again, a high level of heterogeneity between studies was observed for this outcome measure. Despite evidence of the utility of wearable actigraphy in sleep monitoring, consistent outcome measures and methods of analyzing sleep data and circadian rhythm have not been universally agreed upon (2). In order for actigraphy to become routinely used in clinical and drug treatment trials, consistent outcome measures are needed and, as shown in this meta-analysis, may provide a useful endpoint for patients with dementia.
Wearable Devices and Daily Activity
When using wearable devices to measure daily activity, those with dementia had significantly lower daily activity counts than controls. This effect was demonstrated despite across-study variation in methods of calculating daytime activity including peak activity counts, mean activity, and daily activity. A meta-analysis of studies measuring daily activity showed that subjects with dementia demonstrate significantly less daily activity as compared to controls. Four groups reported no differences in nocturnal activity between subjects with dementia and controls. It should be noted that two of these studies did not recruit a control group, but instead compared participants with dementia to their caregivers [McCurry et al. (49) and Merrilees et al. (50)]. Physical activity has been examined in longitudinal studies and found to be associated with both development of dementia as well as disease progression (78). There is increasing evidence that physical activity and exercise as part of multi-domain interventions holds benefit for patients with dementia (79). However, as demonstrated in this review, definitions of physical activity differ significantly between studies and daily activity counts measured by wearable devices are not definite indicators of beneficial exercise, but merely of movement. Some researchers have attempted to quantify daily activity counts into variables such as energy expenditure, and this measure was also reduced in participants with dementia as compared to controls (55). With the growing availability of consumer wrist worn devices for movement and activity tracking, the use of daily activity measurements provides a potential novel end point for large scale clinical trials in dementia.
Wearable Devices and Gait
Analysis of gait behavior was studied by six groups. Significant differences between controls and those with dementia were reported by all groups for multiple aspects of the gait cycle and behavior. However, due to the variation in reported outcomes, a quantitative analysis could not be performed and conclusions regarding the use of wearable devices for the study of gait could not be reliably made. It is important to note that gait speed and walking speed were reported as significantly different in subjects with dementia when compared to controls, while cadence and step variance were not. Lower gait speed in particular has been shown in numerous longitudinal studies to correlate with increased fall risk in older adults (80). Further work to replicate these findings in subjects with dementia is warranted.
Limitations
The main limitation of the meta-analysis was the between-study heterogeneity (Table 7). Given differences in characteristics of study design such as duration of testing, wearable device type, and diagnosis, statistical heterogeneity was expected between publications included in each meta-analysis. Despite this, effect size comparisons between healthy volunteers and participants with dementia were generally consistent in direction between studies. Methodological considerations specifically for actigraphy testing in dementia have been more thoroughly addressed in a clinical review (81). Also, all papers included in this review corresponded to definitions of both all cause dementia and wearable devices which were agreed upon by the author group. As a result, studies which did not conform to these definitions have been excluded and the effect these may have had on the analysis cannot be quantified. Lastly not all devices used have been compared to gold—standard clinical testing and their methods of measurement may differ and therefore their reported differences should be interpreted with caution.
Conclusions and Implications
In conclusion this systematic review and meta-analysis has shown that the wearable devices studied demonstrate differences in those with dementia when compared to controls. Specifically, it provides evidence that wearable devices demonstrate a utility in measuring levels of activity, changes in circadian rhythm, and changes in the sleep wake cycle. Included studies were limited by their heterogeneity, the lack of classification of dementia sub-type and stage, as well as the lack of confirmatory clinical trials. Further work is warranted to correlate these findings with clinical changes which may represent surrogate digital end-points such as the neuro-psychiatric manifestations associated with circadian rhythm changes and the loss of mobility associated with decreased activity.
Data Availability Statement
All datasets generated for this study are included in the article/Supplementary Material.
Author Contributions
JB: concept and design. JB, AC, and RP: acquisition, analysis, or interpretation of data, and drafting of the manuscript. JB, AC, RP, NK, and KT: critical revision of the manuscript for important intellectual content, administrative, technical, or material support. AC: statistical analysis. KT: obtained funding. JB and KT: supervision. AC had full access to all of the data in the study and takes responsibility for the integrity of the data and the accuracy of the data analysis. All authors contributed to the article and approved the submitted version.
Conflict of Interest
The authors declare that the research was conducted in the absence of any commercial or financial relationships that could be construed as a potential conflict of interest.
Acknowledgments
We thank Alissa Link, Education and Information Services Librarian, for her assistance in the construction of database search terms.
Supplementary Material
The Supplementary Material for this article can be found online at: https://www.frontiersin.org/articles/10.3389/fmed.2020.501104/full#supplementary-material
References
1. Alzheimer's Disease International (ADI). Dementia: a Public Health Priority. World Health Organization (2013).
2. Shelgikar AV, Anderson PF, Stephens MR. Sleep tracking, wearable technology, and opportunities for research and clinical care. Chest. (2016) 150:732–43. doi: 10.1016/j.chest.2016.04.016
3. Vasunilashorn S, Steinman BA, Liebig PS, Pynoos J. Aging in place: evolution of a research topic whose time has come. J Aging Res. (2012) 2012:1–6. doi: 10.1155/2012/120952
4. Espay AJ, Bonato P, Nahab F, Maetzler W, Horak F, Lang AE, et al. Technology in parkinson disease: challenges and opportunities. Mov Disord. (2017) 31:1272–82. doi: 10.1002/mds.26642
5. de Bruin ED, Hartmann A, Uebelhart D, Murer K, Zijlstra W. Wearable systems for monitoring mobility-related activities in older people: a systematic review. Clin Rehabil. (2008) 22:878–95. doi: 10.1177/0269215508090675
6. McCleery J, Cohen DA, Sharpley AL. Pharmacotherapies for sleep disturbances in dementia. Cochrane Database Syst Rev. (2016) 11:CD009178. doi: 10.1002/14651858.CD009178.pub3
7. Aharon-Peretz J, Masiah A, Pillar T, Epstein R, Tzischinsky O, Lavie P. Sleep-wake cycles in multi-infarct dementia and dementia of the Alzheimer type. Neurology. (1991) 41:1616–9. doi: 10.1212/WNL.41.10.1616
8. American Psychiatric Association. Diagnostic and Statistical Manual of Mental Disorders. 3rd ed, revised. Washington, DC (1987).
9. McKhann G, Drachman D, Folstein M, Katzman R, Price D, Stadlan EM. Report of the NINCDS-ADRDA work group under the auspices of department of health and human services task force on Alzheimer's disease. Neurology. (1984) 34:939–44. doi: 10.1212/WNL.34.7.939
10. Ahmed RM, Landin-Romero R, Collet TH, van der Klaauw AA, Devenney E, Henning E, et al. Energy expenditure in frontotemporal dementia: a behavioural and imaging study. Brain. (2017) 140:171–83. doi: 10.1093/brain/aww263
11. McKhann GM, Albert MS, Grossman M, Miller B, Dickson D, Trojanowski JQ, et al. Clinical and pathological diagnosis of frontotemporal dementia: report of the Work Group on Frontotemporal Dementia and Pick's Disease. Arch Neurol. (2001) 58:1803–9. doi: 10.1001/archneur.58.11.1803
12. Gorno-Tempini ML, Hillis AE, Weintraub S, Kertesz A, Mendez M, Cappa SF, et al. Classification of primary progressive aphasia and its variants. Neurology. (2011) 76:1006–14. doi: 10.1212/WNL.0b013e31821103e6
13. Rascovsky K, Hodges JR, Knopman D, Mendez MF, Kramer JH, Neuhaus J, et al. Sensitivity of revised diagnostic criteria for the behavioural variant of frontotemporal dementia. Brain. (2011) 134:2456–77. doi: 10.1093/brain/awr179
14. Anderson KN, Hatfield C, Kipps C, Hastings M, Hodges JR. Disrupted sleep and circadian patterns in frontotemporal dementia. Eur J Neurol. (2009) 16:317–23. doi: 10.1111/j.1468-1331.2008.02414.x
15. Neary D. Overview of frontotemporal dementias and the consensus applied. Dement Geriatr Cogn Disord. (1999) 10:6–9. doi: 10.1159/000051205
16. Brown DT, Westbury JL, Schüz B. Sleep and agitation in nursing home residents with and without dementia. Int Psychogeriatrics. (2015) 27:1945–55. doi: 10.1017/S1041610215001568
17. Carvalho-Bos SS, Riemersma-van der Lek RF, Waterhouse J, Reilly T, Van Someren EJ. Strong association of the rest – activity rhythm with well-being in demented elderly women. Am J Geriatr Psychiatry. (2007) 15:92–100. doi: 10.1097/01.JGP.0000236584.03432.dc
18. American Psychiatric Association. Diagnostic and Statistical Manual of Mental Disorders. 4th ed. Washington, DC (1994).
19. David R, Rivet A, Robert PH, Mailland V, Friedman L, Zeitzer JM, et al. Ambulatory actigraphy correlates with apathy in mild Alzheimer's disease. Dementia. (2010) 9:509–16. doi: 10.1177/1471301210381678
20. David R, Mulin E, Friedman L, Le Duff F, Cygankiewicz E, Deschaux O, et al. Decreased daytime motor activity associated with apathy in Alzheimer disease: an actigraphic study. Am J Geriatr Psychiatry. (2012) 20:806–14. doi: 10.1097/JGP.0b013e31823038af
21. Eggermont LHP, Scherder EJA. Ambulatory but sedentary : impact on cognition and the rest – activity rhythm in nursing home residents with dementia. J Gerontol B Psychol Sci Soc Sci. (2008) 63:279–87. doi: 10.1093/geronb/63.5.P279
22. Fetveit A, Bjorvatn B. Sleep duration during the 24-hour day is associated with the severity of dementia in nursing home patients. Int J Geriatr Psychiatry. (2006) 21:945–50. doi: 10.1002/gps.1587
23. Morris JC. The Clinical Dementia Rating (CDR): current version and scoring rules. Neurology. (1993) 43:2412–4. doi: 10.1212/WNL.43.11.2412-a
24. Fleiner T, Haussermann P, Mellone S, Zijlstra W. Sensor-based assessment of mobility-related behavior in dementia: feasibility and relevance in a hospital context. Int Psychogeriatr. (2016) 28:1687–94. doi: 10.1017/S1041610216001034
25. World Health Organization. The ICD-10 Classification of Mental and Behavioral Disorders: Clinical Descriptions and Diagnostic Guidelines. Geneva: World Health Organization (1997).
26. Gehrman P, Marler M, Martin JL, Shochat T, Corey-Bloom J, Ancoli-Israel S. The relationship between dementia severity and rest/activity circadian rhythms. Neuropsychiatr Dis Treat. (2005) 1:155–63. doi: 10.2147/nedt.1.2.155.61043
27. Ghali LM, Hopkins RW, Rindlisbacher P. Ghali. 1995 Temporal shifts.pdf. Int J Geriatr Psychiatry. (1995) 10:517–21. doi: 10.1002/gps.930100612
28. Harper DG, Stopa EG, McKee AC, Satlin A, Fish D, Volicer L. Dementia severity and lewy bodies affect circadian rhythms in Alzheimer disease. Neurobiol Aging. (2004) 25:771–81. doi: 10.1016/j.neurobiolaging.2003.04.009
29. Harper DG, Stopa EG, Mckee AC, Satlin A, Patricia C, Goldstein R, et al. Differential circadian rhythm disturbances in men with alzheimer disease and frontotemporal degeneration. Arch Gen Psychiatry. (2001) 58:353–60. doi: 10.1001/archpsyc.58.4.353
30. Hatfield CF, Herbert J, Van Someren EJW, Hodges JR, Hastings MH. Disrupted daily activity/rest cycles in relation to daily cortisol rhythms of home-dwelling patients with early Alzheimer's dementia. Brain. (2004) 127:1061–74. doi: 10.1093/brain/awh129
31. Hooghiemstra AM, Eggermont LHP, Scheltens P, Van Der Flier WM, Scherder EJA. The rest-activity rhythm and physical activity in early-onset dementia. Alzheimer Dis Assoc Disord. (2015) 29:45–9. doi: 10.1097/WAD.0000000000000037
32. American Psychiatric Association. Diagnostic and Statistical Manual of Mental Disorders. 5th ed. Washington, DC (2013).
33. McKeith IG, Dickson DW, Lowe J, Emre M, O'Brien JT, et al. Diagnosis and management of dementia with Lewy bodies —third report of the DLB consortium. Neurology. (2005) 65:1863–72. doi: 10.1212/01.wnl.0000187889.17253.b1
34. Pohjasvaara T, Mäntylä R, Ylikoski R, Kaste M, Erkinjuntti T. Comparison of different clinical criteria (DSM-III, ADDTC, ICD-10, NINDS- AIREN, DSM-IV) for the diagnosis of vascular dementia. Stroke. (2000) 31:2952–7.
35. IJmker T, Lamoth CJC. Gait and cognition: the relationship between gait stability and variability with executive function in persons with and without dementia. Gait Posture. (2012) 35:126–30. doi: 10.1016/j.gaitpost.2011.08.022
36. Iwata A, Kowa H, Tsuji S. Monitoring daily life activity shows less activity among female dementia patients. Neurol Clin Neurosci. (2013) 1:91–5. doi: 10.1111/j.2049-4173.2013.00029.x
37. James BD, Boyle PA, Bennett DA, Buchman AS. Total daily activity measured with actigraphy and motor function in community-dwelling older persons with and without dementia. Alzheimer Dis Assoc Disord. (2012) 26:238–45. doi: 10.1097/WAD.0b013e31822fc3cb
38. Kodama A, Kume Y, Tsugaruya M, Ishikawa T. Deriving the reference value from the circadian motor active patterns in the “non-dementia” population, compared to the “dementia” population: what is the amount of physical activity conducive to the good circadian rhythm. Chronobiol Int. (2016) 33:1056–63. doi: 10.1080/07420528.2016.1196696
39. König A, Klaming L, Pijl M, Demeurraux A, David R, Robert P. Objective measurement of gait parameters in healthy and cognitively impaired elderly using the dual-task paradigm. Aging Clin Exp Res. (2017) 29:1181–9. doi: 10.1007/s40520-016-0703-6
40. Dubois B, Feldman HH, Jacova C, Hampel H, Molinuevo JL, Blennow K, et al. Advancing research diagnostic criteria for Alzheimer's disease: the IWG-2 criteria. Lancet Neurol. (2014) 13:614–29. doi: 10.1016/S1474-4422(14)70090-0
41. Kuhlmei A, Walther B, Becker T, Müller U, Nikolaus T. Actigraphic daytime activity is reduced in patients with cognitive impairment and apathy. Eur Psychiatry. (2013) 28:94–7. doi: 10.1016/j.eurpsy.2011.04.006
42. Román GC, Tatemichi TK, Erkinjuntti T, Cummings JL, Masdeu JC, Garcia JH, et al. Vascular Dementia Diagnostic criteria for research studies: Report of the NINDS-AIREN International Workshop. Neurology. (1993) 43:250–60. doi: 10.1212/WNL.43.2.250
43. La Morgia C, Ross-Cisneros FN, Koronyo Y, Hannibal J, Gallassi R, Cantalupo G, et al. Melanopsin retinal ganglion cell loss in Alzheimer disease. Ann Neurol. (2016) 79:90–109. doi: 10.1002/ana.24548
44. Lamoth CJ, Van Deudekom FJ, Van Campen JP, Appels BA, De Vries OJ, Pijnappels M. Gait stability and variability measures show effects of impaired cognition and dual tasking in frail people. J Neuroeng Rehabil. (2011) 8:2. doi: 10.1186/1743-0003-8-2
45. Landolt H, Glatzel M, Bla T, Achermann P, Roth C, Mathis J, et al. Sleep-wake disturbances in sporadic Creutzfeldt-Jakob disease. Neurology. (2006) 66:1418–24. doi: 10.1212/01.wnl.0000210445.16135.56
46. Lee JH, Friedland R, Whitehouse PJ, Woo JI. Rhythms of sleep-wake cycle and temperature in alzheimer ' s disease. J Neuropsychiatr. (2004) 16:192–8. doi: 10.1176/jnp.16.2.192
47. Leger D, Elbaz M, Dubois A, Rio S, Mezghiche H, Carita P, et al. Alzheimer's disease severity is not significantly associated with short sleep: survey by actigraphy on 208 mild and moderate alzheimer's disease patients. J Alzheimers Dis. (2016) 55:321–31. doi: 10.3233/JAD-160754
48. Folstein MF, Folstein SE, McHugh PR. “Mini-mental state”: a practical method for grading the cognitive state of patients for the clinician. J Psychiatr Res. (1975) 12:189–98.
49. McCurry SM, Pike KC, Vitiello MV, Logsdon RG, Teri L. Factors associated with concordance and variability of sleep quality in persons with Alzheimer's disease and their caregivers. Sleep. (2008) 31:741–8. doi: 10.1093/sleep/31.5.741
50. Merrilees J, Dowling GA, Hubbard E, Mastick J, Ketelle R, Miller BL. Characterization of apathy in persons wth frontotemporal dementia and the impact on family caregivers. Alzheimer Dis Assoc Disord. (2012) 00:1–6. doi: 10.1097/WAD.0b013e3182471c54
51. Neary D, Snowden JS, Gustafson L, Passant U, Stuss D, Black S, et al. Frontotemporal lobar degeneration—a consensus on clinical diagnostic criteria. Neurology. (1998) 51:1546–54. doi: 10.1212/WNL.51.6.1546
52. Most EIS, Aboudan S, Scheltens P, Van Someren EJW. Discrepancy between subjective and objective sleep disturbances in early- and moderate-stage alzheimer disease. Am J Geriatr Psychiatry. (2012) 20:460–7. doi: 10.1097/JGP.0b013e318252e3ff
53. Moyle W, Jones C, Murfield J, Draper B, Beattie E, Shum D, et al. Levels of physical activity and sleep patterns among older people with dementia living in long-term care facilities: A 24-h snapshot. Maturitas. (2017) 102:62–8. doi: 10.1016/j.maturitas.2017.05.015
54. Mulin E, Zeitzer JM, Friedman L, Le Duff F, Yesavage J, Robert PH, et al. Relationship between apathy and sleep disturbance in mild and moderate Alzheimer's disease: An actigraphic study. J Alzheimers Dis. (2011) 25:85–91. doi: 10.3233/JAD-2011-101701
55. Murphy J, Holmes J, Brooks C. Measurements of daily energy intake and total energy expenditure in people with dementia in care homes: the use of wearable technology. J Nutr Health Aging. (2017) 21:927–32. doi: 10.1007/s12603-017-0870-y
56. Olsen C, Pedersen I, Bergland A, Enders-Slegers M-J, Jøranson N, Calogiuri G, et al. Differences in quality of life in home-dwelling persons and nursing home residents with dementia – a cross-sectional study. BMC Geriatr. (2016) 16:137. doi: 10.1186/s12877-016-0312-4
57. Paavilainen P, Korhonen I, Lötjönen J, Cluitmans L, Jylhä M, Särelä A, et al. Circadian activity rhythm in demented and non-demented nursing-home residents measured by telemetric actigraphy. J Sleep Res. (2005) 14:61–8. doi: 10.1111/j.1365-2869.2004.00433.x
58. Pollak CP, Stokes PE. Circadian rest-activity rhythms in demented and nondemented older community residents and their caregivers. J Am Geriatr Soc. (1997) 45:446–52. doi: 10.1111/j.1532-5415.1997.tb05169.x
59. Mattis. Mental status examination for organic mental syndrome in the elderly patient. In: Karasu E, editor. Geriatric Psychiatry. New York, NY: Grune and Stratton (1976).
60. Rindlisbacher P, & Hopkins RW. An investigation of the sundowning syndrome. Int J Geriatr Psychiatry. (1992) 7:15–23. doi: 10.1002/gps.930070104
61. Schwenk M, Hauer K, Zieschang T, Englert S, Mohler J, Najafi B. Sensor-derived physical activity parameters can predict future falls in people with dementia. Gerontology. (2014) 60:483–92. doi: 10.1159/000363136
62. Valembois L, Oasi C, Pariel S, Jarzebowski W, Lafuente-Lafuente C, Belmin J. Wrist actigraphy: a simple way to record motor activity in elderly patients with dementia and apathy or aberrant motor behavior. J Nutr Heal Aging. (2015) 19:759–64. doi: 10.1007/s12603-015-0530-z
63. Van Alphen HJM, Volkers KM, Blankevoort CG, Scherder EJA, Hortobágyi T, Van Heuvelen MJG. Older adults with dementia are sedentary for most of the day. PLoS ONE. (2016) 11:1–16. doi: 10.1371/journal.pone.0152457
64. van Someren EJ, Hagebeuk EE, Lijzenga C, Scheltens P, de Rooij SE, Jonker C, et al. Circadian rest-activity rhythm disturbances in Alzheimer's disease. Biol Psychiatry. (1996) 40:259–70. doi: 10.1016/0006-3223(95)00370-3
65. Varma VR, Watts A. Daily physical activity patterns during the early stage of Alzheimer's disease. J Alzheimers Dis. (2017) 55:659–67. doi: 10.3233/JAD-160582
66. Viegas SM, Richards KC, Beck CK, Lambert CW, O'Sullivan PS, Cole CS, et al. Predictors of daytime sleep of nursing home residents with dementia. J Am Psychiatr Nurses Assoc. (2006) 12:286–93. doi: 10.1177/1078390306295071
67. Volicer L, Harper DG, Manning BC, Goldstein R, Satlin A. Sundowning and circadian rhythms in alzheimer ' s disease. Am J Psychiatry. (2001) 158:704–11. doi: 10.1176/appi.ajp.158.5.704
68. Wams EJ, Wilcock GK, Foster RG, Wulff K. Sleep-wake patterns and cognition of older adults with amnestic mild cognitive impairment (aMCI): a comparison with cognitively healthy adults and moderate alzheimer's disease patients. Curr Alzheimer Res. (2017) 14:1030–41. doi: 10.2174/1567205014666170523095634
69. Weissová K, Bartoš A, Sládek M, Nováková M, Sumová A. Moderate changes in the circadian system of Alzheimer's disease patients detected in their home environment. PLoS ONE. (2016) 11:1–19. doi: 10.1371/journal.pone.0146200
70. Wirz-Justice A, Schröder CM, Gasio PF, Cajochen C, Savaskan E. The circadian rest-activity cycle in korsakoff psychosis. Am J Geriatr Psychiatry. (2010) 18:33–41. doi: 10.1097/JGP.0b013e3181b0467a
71. Yesavage JA, Friedman L, Kraemer HC, Noda A, Wicks D, Bliwise DL, et al. A follow-up study of actigraphic measures in home-residing Alzheimer's disease patients. J Geriatr Psychiatry Neurol. (1998) 11:7–10. doi: 10.1177/089198879801100103
72. Lukowicz P, Kirstein T, Tröster G. Wearable systems for health care applications. Methods Inf Med. (2004) 43:232–8. doi: 10.1055/s-0038-1633863
73. Downs SH, Black N. The feasibility of creating a checklist for the assessment of the methodological quality both of randomised and non-randomised studies of health care interventions. J Epidemiol Commun Health. (1998) 52:377–84. doi: 10.1136/jech.52.6.377
74. Cohen J. Statistical Power Analysis for the Behavioral Sciences. Routledge (1988). Available from: https://www.taylorfrancis.com/books/9780203771587 (accessed September 8, 2018).
75. Borenstein M, Hedges LV, Higgins JPT, Rothstein HR. A basic introduction to fixed-effect and random-effects models for meta-analysis. Res Synth Methods. (2010) 1:97–111. doi: 10.1002/jrsm.12
76. Higgins JP, Green S. Cochrane Handbook for Systematic Reviews of Interventions: Cochrane Book Series. Chichester: The Cochrane Collaboration and John Wiley & Sons Ltd. (2008). p. 1–649. doi: 10.1002/9780470712184
77. Ioannidis JPA. Perfect study, poor evidence: interpretation of biases preceding study design. Semin Hematol. (2008) 45:160–6. doi: 10.1053/j.seminhematol.2008.04.010
78. Rovio S, Kåreholt I, Viitanen M, Winblad B, Kivipelto M, Soininen NH, et al. Leisure-time physical activity at midlife and the risk of dementia and Alzheimer's disease. Lancet Neurol. (2005). 4:705–11. doi: 10.1016/S1474-4422(05)70198-8
79. Ngandu T, Lehtisalo J, Solomon A, Levälahti E, Ahtiluoto S, Antikainen R, et al. A 2 year multidomain intervention of diet, exercise, cognitive training, and vascular risk monitoring versus control to prevent cognitive decline in at-risk elderly people (FINGER): a randomised controlled trial. Lancet. (2015) 385:2255–63. doi: 10.1016/S0140-6736(15)60461-5
80. Peel NM, Kuys SS, Klein K. Gait speed as a measure in geriatric assessment in clinical settings: a systematic review. J Gerontol A Biol Sci Med Sci. (2013) 68:39–46. doi: 10.1093/gerona/gls174
Keywords: technology, geriatrics, cognition, sleep, wearable
Citation: Cote AC, Phelps RJ, Kabiri NS, Bhangu JS and Thomas KK (2021) Evaluation of Wearable Technology in Dementia: A Systematic Review and Meta-Analysis. Front. Med. 7:501104. doi: 10.3389/fmed.2020.501104
Received: 27 September 2019; Accepted: 30 November 2020;
Published: 11 January 2021.
Edited by:
Jon Irazusta, University of the Basque Country, SpainReviewed by:
Tomasz Grodzicki, Jagiellonian University Medical College, PolandJavier Santabarbara, University of Zaragoza, Spain
Copyright © 2021 Cote, Phelps, Kabiri, Bhangu and Thomas. This is an open-access article distributed under the terms of the Creative Commons Attribution License (CC BY). The use, distribution or reproduction in other forums is permitted, provided the original author(s) and the copyright owner(s) are credited and that the original publication in this journal is cited, in accordance with accepted academic practice. No use, distribution or reproduction is permitted which does not comply with these terms.
*Correspondence: Jaspreet S. Bhangu, amJoYW5ndUBidS5lZHU=
†These authors have contributed equally to this work