- 1Department of Production Engineering, Jadavpur University, Kolkata, West Bengal, India
- 2Department of Mechanical Engineering, Vel Tech Rangarajan Dr. Sagunthala R&D Institute of Science and Technology, Avadi, India
- 3University Centre for Research & Development, Chandigarh University, Mohali, India
- 4Department of Machining, Assembly and Engineering Metrology, Faculty of Mechanical Engineering, VSB-Technical University of Ostrava, Ostrava, Czechia
Optimization of electrical discharge machining (EDM) processes is a critical issue due to complex material removal mechanism, presence of multiple input parameters and responses (outputs) and interactions among them and varying interest of different stakeholders with respect to relative importance assigned to the considered responses. Multi-criteria decision making (MCDM) techniques have become potent tools in solving parametric optimization problems of the EDM processes. In this paper, more than 130 research articles from SCOPUS database published during 2013–22 are reviewed extracting information with respect to experimental design plans employed, materials machined, dielectrics used, process parameters and responses considered and MCDM tools applied along with their integration with other mathematical techniques. A detailed analysis of those reviewed articles reveals that the past researchers have mostly preferred Taguchi’s L9 orthogonal array as the experimental design plan; EDM oil as the dielectric fluid; medium and high carbon steels as the work materials; peak current and pulse-on time as the input parameters; material removal rate, tool wear rate and surface roughness as the responses; and grey relational analysis as the MCDM tool during conducting and optimizing EDM operations. This review paper would act as a data repository to the future researchers in understanding the stochastic behaviour of EDM processes and providing guidance in setting the tentative operating levels of varying input parameters along with achievable response values. The extracted dataset can be treated as an input to any of the machine learning algorithms for subsequent development of appropriate prediction models. This review also outlines potential future research avenues, emphasizing advancements in EDM technology and the integration of innovative multi-criteria decision-making tools.
1 Introduction
The EDM, developed by two Soviet scientists B. Lazarenko and N. Lazarenko in 1943 while investigating the destructive effect of electrical discharges on removing material from conductive workpieces, is one the most popular and industrially-accepted non-traditional machining processes in the present-day manufacturing scenario. It is an electro-thermal process in which material removal takes place due to a series of continuous repetitive high-frequency controlled pulse discharges between the tool (cathode) and the workpiece being machined (anode) (Pandey and Shan, 2017). During the EDM operation, a small gap (0.005–0.05 mm) is always maintained between the tool and the workpiece which is responsible for formation of a plasma channel raising the temperature around 8,000°C–12000°C resulting in melting and vaporization of material to provide the final shape to the workpiece according to the tool geometry (Youssef and El-Hofy, 2020). Both the tool and workpiece are immersed in a dielectric medium (deionized water/kerosene/EDM oil) which basically helps in plasma formation, cools the machining zone and removes the molten material (debris) by flushing action. When sufficient voltage and current are applied to the tool and the workpiece, electrons break away from the tool and accelerate towards the workpiece, thereby hitting and breaking the conductive dielectric medium, causing creation of tiny craters and removal of material from the workpiece surface (Ho and Newman, 2003; Phan N. H. et al., 2022). The working principle of an EDM process is demonstrated in Figure 1.
Unlike the conventional machining processes, EDM is a non-contact spark erosion process which is capable of generating complex shapes with high dimensional accuracy and tolerance on most of the conductive and difficult-to-cut advanced engineering materials irrespective of their physical and mechanical properties. It can efficiently machine medium and high carbon steels, aluminium and titanium and their alloys, MMCs and hybrid MMCs, superalloys (Inconel, Monel, Nimonics, etc.), shape memory alloys, tungsten carbide, etc., which have found wide ranging applications in many of the die and mold making, automobile, aerospace, defense, electronics, nuclear and medical industries (Muthuramalingam and Mohan, 2014; Pramanik et al., 2020). With appropriate modifications in the machining setup, it can also machine non-conductive materials, such as ceramics and glasses and generate micro-features (like micro-holes, cavities, pockets, etc.) in the above-mentioned materials (Prakash et al., 2019; Kumar et al., 2020; Thangaraj et al., 2020; Pandey and Anas, 2022). It has several advantages, like no formation of mechanical stress, chatter, burr and vibration, higher flexibility and dimensional accuracy, economical operation, etc. But it also suffers from some major disadvantages, like low MRR, poor surface quality, high tool wear, not suitable for mass production, formation of recast layer, white layer and surface cracks, heat affected zone, etc. Although it has been experimented that proper selection of dielectric fluid and tool material can help in achieving higher MRR along with lower SR and TWR on some of the selected work materials, use of hydrocarbons as the dielectric medium, excessive noise, emission of toxic substances, formation of aerosol and unhealthy working environment hinder its real-time applications in many of the industries (Chakraborty et al., 2015; Liu et al., 2022). Research works have now been directed towards adoption of green or dry EDM process with minimum consumption of dielectric, use of deionized water as dielectric, minimum energy consumption, minimum emission of toxic substances, etc., leading to sustainable manufacturing in present-day Industry 4.0 scenario (Leão and Pashby, 2004; Singh et al., 2016; Gouda et al., 2021; Ming et al., 2021).
Based on the working principle, EDM processes can be broadly divided into die-sinking EDM, rotary EDM (instead of a stationary tool, it is rotated to have better MRR and surface quality), ultrasonic EDM (ultrasonic pulses are made to pass between the tool and the workpiece), cryogenically cooled EDM (the tool is constantly cooled applying cryogenic fluids), powder-mixed EDM (abrasive particles, e.g., silicon carbide, boron carbide, etc., are proportionally mixed with dielectric fluid), vibration-assisted EDM (the tool is vibrated for easy removal of eroded material due to flushing action of the dielectric medium), wire EDM (a thin strand of continuous wire made of tungsten, molybdenum, brass or copper is employed as the tool electrode) etc. (Huu Phan and Muthuramalingam, 2021). Among all these variants, die-sinking (sinker/conventional) EDM is most commonly used in almost all the industries, in which the electrode (tool) having a distinctive shape sink (penetrates) into the material (hence, its name is sinker) causing material removal.
Like all other non-traditional machining processes, the performance of conventional EDM process with respect to MRR, SR, TWR, WLT, SCD and different form errors is noticed to be significantly influenced by its various input parameters which can be broadly classified as electrical and non-electrical (Muthuramalingam and Mohan, 2015). The examples of electrical parameters are Ton, Toff, Ip, voltage, pulse duration, DF, resistance, capacitance, etc. On the other hand, type of the dielectric and its pressure, tool material, work material thickness, TL, Sg, etc., are the examples of non-electrical parameters. Several studies have already been conducted to explore the relationships of those EDM parameters on the responses and it has been realized that maximum machining efficiency can only be achieved when an EDM process is operated at the optimal settings of its various input parameters. Nonlinear relationship between the inputs and outputs, stochastic and complicated electrical discharge mechanism, involvement of multiple conflicting responses (higher MRR versus lower SR, higher efficiency versus lower energy consumption, etc.) and varying opinions of the stakeholders (machine operators, process engineers and end users) regarding relative importance of the process characteristics make parametric optimization of an EDM process a complicated task. Occasionally, opinions of the machine operators/technical experts are sought and machining data handbooks are consulted for optimizing the performance of an EDM process which may sometimes lead to near or sub-optimal solutions. To resolve this issue, applications of some sound and systematic multi-objective optimization tools are highly recommended. In this direction, several MCDM techniques have been appeared as potent tools in identifying the optimal settings of varying EDM parameters leading to attainment of the most desired response values. They are mathematically quite simple and easy to implement helping the concerned engineers in optimizing the processes. Kalita et al. (2022) recently reviewed the applications of various MCDM techniques for solving parametric optimization problems of many of the non-traditional machining processes and identified GRA and TOPSIS as the two most popular tools employed for the said purpose. A separate literature review of the MCDM applications for optimizing only EDM processes along with identification of the experimental design plans employed, work materials machined, dielectrics used, process parameters considered along with their operational settings, measured response values and MCDM tools deployed is really scarce. This review paper attempts to bridge this gap while analyzing the contents of more than 130 research articles published during 2013–22 in the reputed international journals available in the SCOPUS database and identifies how different MCDM techniques have been employed for solving parametric optimization problems of standalone EDM processes. It would act as a data repository to help the process engineers in singling out the tentative settings of different EDM parameters for attaining the desired responses while relieving them from conducting pilot experiment trials. It would also help in identifying the most appropriate experimental design plan to be deployed for a given EDM application with known number of process parameters and their operating levels. The extracted information with respect to parametric settings and measured responses can be utilized as the training dataset in any of the machine learning algorithms to develop the corresponding predictive models. This paper is structured as follows: Section 2 provides the framework for the literature review and a brief overview of MCDM techniques is presented in Section 3. Applications of different MCDM tools for parametric optimization of EDM processes are shown in Section 4 through succinct tabular forms. The derived results are analyzed in Section 5 and Section 6 concludes the paper along with future research directions.
2 Framework for the literature review
As mentioned earlier, the main aim of this paper is to critically review the applications of various MCDM tools leading to parametric optimization of standalone EDM processes. Keeping this objective in mind, the SCOPUS database has been exhaustively searched with the keyword “Optimization of EDM process using MCDM methods” (Optimization AND EDM process AND MCDM techniques) in the title, keywords and abstract with identification of more than 250 research articles published during the stipulated time duration of 2013–2022 (last 10 years). To keep the number of papers to be reviewed into a manageable quantity, those articles published during the last 10 years are only considered here. An initial screening has then been performed to exclude those articles published in conference proceedings and as book chapters. Although there are different variants of EDM process, like rotary EDM, vibration-assisted EDM, powder-mixed EDM, ultrasonic EDM, electrical discharge milling, etc., this review paper only considers optimization of die-sinking EDM (conventional EDM) using varying MCDM tools. Finally, the contents of 137 research articles are analyzed with subsequent extraction of the relevant information with respect to experimental design plan employed, work material machined, dielectric utilized, process parameters considered along with their operating levels, response values measured, MCDM technique deployed and other mathematical tools considered for criteria weight measurement/comparison purposes. The analyzed results are presented in succinct tabular forms to help the fellow researchers/readers in diverse dimensions, like consideration of the appropriate experimental design plan depending on the number of input parameters and their levels, suitable dielectric to be utilized, tentative operating levels of EDM parameters, achievable response values, selection of suitable criteria weight measurement technique and deployment as the training dataset in any of the machine learning algorithms to develop the corresponding prediction tools.
3 MCDM techniques
The MCDM techniques are those mathematical tools employed for identification of the most apposite alternative from a pool of candidate solutions in presence of multiple conflicting attributes/criteria (Chakraborty et al., 2023). There are several different types of MCDM techniques, like WSM (Miller and Starr, 1969), WPM (Miller and Starr, 1969), AHP (Saaty, 1980), MOORA (Brauers et al., 2008), TOPSIS (Behzadian et al., 2012), VIKOR (Opricovic and Tzeng, 2004), PROMETHEE (Brans and Vincke, 1985), COPRAS (Kaklauskas et al., 2006), ELECTRE (Roy and Vincke, 1981), PSI (Maniya and Bhatt, 2010), EDAS (Keshavarz et al., 2015), CODAS (Ghorabaee et al., 2016), MARCOS (Stević et al., 2020), DEAR (Liao and Chen, 2002), GRA (Kuo et al., 2008) etc., having their own merits and demerits. The basic input to any of the MCDM methods is a decision matrix consisting of a set of feasible alternatives whose performance needs to be evaluated based on multiple conflicting criteria. Most of these techniques are mathematically easy to understand and computationally uncomplicated to implement. Due to their simplicity, they have widely been acknowledged by the researchers to solve parametric optimization problems of many of the machining processes where the experiment trials having different combinations of the input parameters are treated as the alternatives and measured responses as the evaluation criteria. Based on the derived performance scores, all the experimental trials can be ranked from the best to the worst with identification of the optimal intermix of input parameters leading to subsequent attainment of the compromised response values. Although in most of the MCDM methods, equal importance (weight) has usually been assigned to the considered responses to avoid mathematical complexity, but depending on the preference of different stakeholders, relative weights measured using different subjective and objective techniques, like AHP, EM, PCA, WPCA, CRITIC, etc., have also been occasionally integrated with MCDM tools to derive more practical solutions.
4 Optimization of EDM processes using MCDM methods
4.1 AHP method
The AHP method, developed by Saaty (Saaty, 1980), is based on the principle of pair-wise comparison to compute the performance score of each of the alternatives under consideration. Using a 1-9 scale, the relative importance of each pair of criteria is first compared to determine their weights. Subsequently, the performance of the alternatives is again pair-wise evaluated against each of the criteria. The relative performance of the alternatives and criteria weights are finally aggregated together to calculate the overall scores of all the alternatives leading to their rankings. It is a subjective MCDM method, heavily relying on the individual judgments of the participating decision makers. These pair-wise comparisons can only be accepted when the resulting consistency ratios are observed to be less than 0.10. Table 1 shows the applications of AHP method for optimizing EDM processes. While performing EDM operation on Al 6061 alloy, Okponyia and Oke (Okponyia and Oke, 2020) proposed a novel approach while integrating present worth method, fuzzy theory and AHP to determine the optimal values of the considered input parameters and responses. The present worth method when applied as a diagnostic tool identified the said EDM operation as healthy and observed Ip as the most significant input parameter influencing the responses. Using AHP method, Sidhu et al. (2021) first determined the relative weights of RS, MRR and SR as 0.5815, 0.3090 and 0.1095 respectively and then pair-wise compared all the nine experiments against each of the responses (criteria) to identify trial number 4 (Ton = 45 μs, Toff = 15 μs, Ip = 8 A and graphite tool material) as the optimal choice during EDM of Al MMC with different tool materials.
4.2 MOORA method
It is one of the most computationally simple MCDM methods, in which the sum of the normalized performance scores of the non-beneficial criteria (smaller-the-better type) is subtracted from that of the normalized performance scores of the beneficial criteria (larger-the-better type) to obtain the overall scores of all the alternatives (Brauers et al., 2008). Sometimes, these performance scores are multiplied by the corresponding criteria weights to derive more pragmatic solutions. The applications of MOORA method considered for determination of the optimal parametric intermixes of EDM processes are enlisted in Table 2. While optimizing an EDM process, Paul et al. (2019) presented the application of a hybridized approach in the form of MOORA-PCA and contrasted its performance against conventional MOORA method. It was concluded that the proposed approach would provide better values of the considered responses as compared to standalone MOORA method. Kumar et al. (2022) developed a regression model correlating multi-performance characteristic index (determined based on MOORA method) and EDM parameters which was later optimized using GA. The most significant EDM parameters influencing MRR and TWR were also identified.
4.3 TOPSIS
The TOPSIS method (Behzadian et al., 2012) is based on calculation of the Euclidian distances of the considered alternatives from the ideal and the anti-ideal solutions and identification of the best alternative having the minimum distance from the ideal solution and maximum distance from the anti-ideal solution. Table 3 provides information of those EDM processes which have been optimized using this method. Singh et al. (2020) performed EDM operation on Inconel 718 material and optimized the process while considering different criteria weight measurement schemes, like SDV, MW, EM and AHP in fuzzy decision-making environment. It was noticed that almost all the criteria weight measurement methods coupled with TOPSIS would identify the same parametric combination as the optimal choice for the said EDM process. Based on the experimental data of EDM operation on mild steel using Cu-multi-walled carbon nanotube-coated 6061Al electrode, Mandal and Mondal (2021) employed MOPSO technique to search out the non-dominated solutions and developed the corresponding Pareto Frontier. The TOPSIS was later utilized to find out the most appropriate solution from the Pareto optimal set. The EDM operation of Ti-6Al-4V alloy was optimized by Sahu et al. (2022) employing a hybrid grey-TOPSIS-based QPSO technique. It was concluded that both MRR and MH were significantly affected by Ip, whereas, tool material was the most influential input parameter for TWR, SR and SCD. On the other hand, WLT and closeness coefficient calculated by TOPSIS were controlled by Ton.
4.4 VIKOR method
The VIKOR method, proposed by Opricovic and Tzeng (2004), is a compromise-based ranking approach, employed for solving MCDM problems having conflicting and non-commensurable criteria. It derives a compromise solution closest to the ideal solution and farthest from the anti-ideal solution based on an agreement established by the mutual concessions between the decision makers. The EDM processes have also been optimized using VIKOR method, as highlighted in Table 4. Using Taguchi’s L18 OA, Kumar J. et al. (2021) performed 18 EDM experiments on AZ-91 Mg alloy and endeavored to optimize the said process based on VIKOR method. In the later stage, fuzzy logic was integrated with VIKOR to predict the values of MRR and SR which were noticed to be quite close to the experimental observations.
4.5 PROMETHEE
This method was developed by Brans and Vincke (1985) and is an outranking-based approach able to provide a complete ranking of the alternatives under consideration. The performance of the alternatives is assessed based on pair-wise comparisons against each criterion utilizing preference functions which are subsequently aggregated using criteria weights to derive the corresponding net outranking flows considered for ranking of the alternatives. Table 5 enlists the applications of PROMTHEE method leading to optimization of EDM processes. While machining Nimonic C263 alloy using three different electrode materials (copper, tungsten and cooper-tungsten), Shastri and Mohanty (2021) proposed a hybrid approach combining PROMETHEE with CS algorithm to optimize an EDM process. Besides MRR, SR and TWR, two other important responses, i.e., SEC and N were also considered to provide sustainable machining environment. A confirmatory experiment was finally conducted which had shown 6.02% overall improvement for the considered responses at the achieved optimal combinations of the EDM parameters.
4.6 COPRAS method
The COPRAS (Kaklauskas et al., 2006) follows step-wise ranking and evaluation procedure of the alternatives with respect to their relative significance and utility degree while considering both the ideal and the anti-ideal solutions. It basically assumes direct and proportional dependences of the significance and utility degree of the alternatives in presence of multiple conflicting criteria. Based on a BBD plan, Shastri and Mohanty (2022) performed EDM operation on Nimonic C263 material using EDM oil as the dielectric medium. During experimentation, each of the four EDM parameters was set at three different levels, i.e., Ton (100, 200, 300 μs), Vg (50, 60, 70 V), Ip (3, 5, 7 A), DF (80, 85, 90%) and tool material (W, Cu-W, Cu) and the corresponding values of various responses were measured/calculated as SR = 13.05 μm, SCD = 0.0026 μm/μm, RLT = 8.112 mm, MH = 319.12 HV and MC = 73.310 INR. Finally, the EDM process was optimized using COPRAS method with identification of the corresponding input parameters as Ton = 100 μs, Vg = 60 V, Ip = 5 A, DF = 85% and tungsten tool material. A confirmatory experiment was also conducted to validate the derived results showing only 6.13% error between the predicted and actual solutions.
4.7 PSI method
The PSI, developed by Maniya and Bhatt (2010), is a simple MCDM method requiring no information regarding weights of the considered criteria. In this approach, the candidate alternatives are sorted from the best to the worst depending on their preference selection indexes. Using PSI method, an EDM operation on titanium alloy was optimized by Phan NH. et al. (2022) while performing 16 experiments based on Taguchi’s L16 OA. Nickel-coated aluminium was used as the electrode material and HD-1 oil was the dielectric medium. Each of the EDM parameters was set at four different levels, i.e., Ton (100, 500, 1,000, 1,500 μs), Vg (40, 45, 50, 55 V) and Ip (10, 20, 30, 40 A). Using high-precision electronic weighing balance, the MRR and TWR values were recorded as 0.096 mm3/min and 0.027 mm3/min respectively at the derived optimal parametric combination of the said EDM process.
4.8 EDAS method
With respect to mathematical modelling, EDAS method slightly differs from TOPSIS as it is based on calculation of the distances from the average solution rather than ideal and anti-ideal solutions (Keshavarz et al., 2015). In this method, the arithmetic mean of the performance values of the alternatives against each of the criteria is taken into account to compute the average solution. In case of rank reversal problems, it sometimes performs better than TOPSIS. The EDAS method was utilized by Ganesh et al. (2020) to optimize the EDM operation of Inconel 718 material using brass as the tool material and EDM oil as the dielectric. Using BBD plan, 13 experiments were performed at three different levels of Ton (100, 250, 400 μs), Toff (60, 105,150 μs) and Ip (8, 10,12 A) and their effects on MRR and TWR were studied. The corresponding MRR and TWR values were obtained as 1.14626 mm3/min and 0.12875 mm3/min respectively. The EDAS method was employed to search out the optimal combinations of the considered EDM process parameters as three different criteria weighting scenarios. It was later integrated with NSGA-II and GP techniques. The NSGA-II was adopted to develop the corresponding Pareto front and GP metamodeling technique was applied to correlate the responses with EDM parameters. It was noticed that the developed GP-based metamodels would have better prediction accuracy for both the responses as compared to the conventional polynomial regression models.
4.9 CODAS method
In CODAS method (Ghorabaee et al., 2016), the candidate alternatives are evaluated and subsequently ranked based on their two relative distance measures (Euclidean and Taxicab) from the anti-ideal solution. The best alternative should have the maximum distance from the anti-ideal solution. When the performance of any two alternatives cannot be compared according to the Euclidean distance, the Taxicab distance is then considered as the secondary measure. Pandiyan et al. (2022) employed Taguchi’s L27 OA plan to study the effects of Ton, Vg and Ip on MRR, TWR, CIR and CYL while performing EDM operation on AA6061-T6 alloy using a copper electrode and EDM oil as the dielectric fluid. During experimentation, each of the EDM parameters was varied at three different levels, i.e., Ton (50, 75, 100 μs), Vg (30, 35,40 V) and Ip (9, 12, 15 A). The corresponding weights of MRR, TWR, CIR and CYL were estimated as 0.164, 0.128, 0.402 and 0.306 respectively using EM. Finally, the EDM process was optimized applying CODAS method with identification of the optimal settings of the input parameters as Ton = 100 μs, Vg = 40 V and Ip = 9 A, providing the measured values of MRR, TWR, CIR and CYL as 0.168 g/min, 0.017 g/min, 0.003 mm and 0.046 mm respectively.
4.10 MARCOS method
The MARCOS (Stević et al., 2020) is a recently developed MCDM tool, based on specifying the inherent relationship between the alternatives and some reference values. Usually, the ideal and the anti-ideal solutions are treated as the references. These relationships lead to the calculation of utility functions and a compromise ranking of the alternatives under consideration. The utility functions define the positions of all the alternatives with respect to ideal and anti-ideal solutions. The best alternative must be located nearest to the ideal solution and farthest from the anti-ideal solution. Treating Ip, Ton and Vg as the input parameters and MRR and SR as the responses, Biswal et al. (2022) applied MARCOS method for optimization of an EDM operation considering L9 OA as the design plan, deionized water as the dielectric and Al 6061-WC-B4C and Al 7175-WC-B4C as the work materials. It was interestingly noticed that there had been two different combinations of the EDM parameters for the two considered work materials. Finally, tensile strength, ultimate tensile strength and hardness values of the machined components were measured to validate the applicability of EDM process in machining of composite materials.
4.11 DEAR method
The DEAR method (Liao and Chen, 2002) is an easy to understand and implement tool and can be effectively integrated with Taguchi methodology to act as a multi-objective optimization technique to search out the most suitable intermix of input parameters of any of the machining processes. In this method, the alternatives are ranked based on the computed values of multi-response performance index. It has the inherent advantage of calculating the corresponding criterion weight considering the ratio of the criterion value for any alternative to the sum of criteria values for all the alternatives. Table 6 depicts the applications of Taguchi-DEAR method in optimizing EDM processes. Sameer et al. (2021) performed EDM operation on maraging steel treating Ip, DT and PF as the input parameters and MRR, SR and TWR as the responses and optimized the said process using Taguchi-DEAR method. In the similar direction, an EDM operation on titanium alloy was optimized by Phan et al. (2021) with identification of the optimal combination of EDM parameters as Ton = 1,000 μs, Vg = 55 V and Ip = 40 A which would yield the corresponding values of MRR, SR and TWR as 0.0139 g/min, 9.313 μm and 0.0089 g/min respectively.
4.12 GRA method
The GRA technique (Kuo et al., 2008) is based on the concept of grey theory, where it is assumed that any system in nature is neither white nor black, but it is mostly grey. A grey system deals with those problems with some known and some unknown information. It has proved itself as one of the most powerful tools in optimizing machining processes in presence of incomplete experimental dataset, measurement error and “larger-the-better” and “smaller-the-better” responses. In this method, the absolute difference between two data sequences is evaluated, while calculating the approximate grade of correlation existing between them. The basic objective of GRA is to transform multiple responses into a single performance measure (GRG) which finally helps in ranking of the considered alternatives (experimental trials). Its uncomplicated mathematical steps and independency of criteria weights make it a popular choice in optimizing diverse machining processes. In Table 7, details of the EDM operations are presented which have been optimized using GRA technique.
Soepangkat et al. (2014), Dewangan et al. (2015b), Prabhu and Vinayagam (2015), Vijayanand and Ilangkumaran (2017) and Sharma et al. (2021) conducted EDM operations on various difficult-to-cut advanced engineering materials based on Taguchi’s OA plans and attempted to integrate GRA with fuzzy logic to frame simple “If-Then” clauses to depict the relationships between the calculated GRG values and different input parameters. It would also lead to identification of the optimal combinations of EDM parameters achieving the target response values. Treating Ton, Vg, DF and type of the tool material as the EDM parameters and MRR, SR, TWR, WLT, SCD and MH as the responses, Sahu and Mahapatra (2021) first compared the performance of the tool made of AlSi10 Mg over solid copper and graphite electrodes. A novel optimization technique, combining desirability-based GRA with FA, was later employed to optimize the said process. Finally, the response values at varying combinations of the EDM parameters were predicted using LSSVM while achieving satisfactory values of the root mean squared error.
4.13 Utility theory
Every product needs to be manufactured keeping in mind the need and expectation of the end users (customers). In general, utility can be defined as the usefulness of a specific product/process with reference to the customer expectations having its performance evaluated based on various objectives. The performance scores of a product/process are assessed with respect to each quality attribute and aggregated together to define a composite index (utility) (Kumar et al., 2000). Based on L18 OA design plan, Chandrashekarappa et al. (2021) machined HCHCr D2 steel material considering Ton, Ip and type of the tool material as the process variables and MRR, SR and TWR as the outputs. Graphite, copper and brass were used as the tool materials, distilled water and kerosene were utilized as the dielectric medium and the settings of Ton and Ip were maintained between 50 µs and 100 µs and 3 A and 9 A respectively. Weights of MRR, SR and TWR were estimated employing PCA and CRITIC methods. Both the optimization approaches, i.e., Taguchi-PCA-utility and Taguchi-CRITIC-utility had yielded the same combination of EDM parameters, i.e., distilled water as the dielectric, graphite as the tool material, Ip = 9 A and Ton = 50 µs as the optimal choice, providing the corresponding values of MRR, SR and TWR as 0.0632 g/min, 1.68 µm and 0.012 g/min respectively.
4.14 Multiple MCDM methods
It can be noticed from this literature review that some of the researchers have applied multiple MCDM methods for optimization of EDM processes. Those techniques have mainly been employed to validate the optimal parametric combination derived by one method against the other and it has been interestingly noticed that in most of the cases, they have provided the same intermix of EDM parameters. Table 8 shows applications of multiple MCDM methods considered for optimizing the EDM processes. From this table, it can be unveiled that the researchers (Das et al., 2018; Pradhan, 2018; Yuvaraj and Suresh, 2019; Kumar and Mondal, 2020; Kumar and Rai, 2020; Sahu and Mahapatra, 2020; Bhosale et al., 2021; Srinivasan et al., 2021b; Jana et al., 2021; Patnaik et al., 2022) have maximally preferred to employ both GRA and TOPSIS in identifying the optimal combinations of the EDM parameters. During machining of Si3N4-TiN ceramic material using an EDM process, Srinivasan et al. (2021b) applied both GRA and TOPSIS to achieve the same optimal intermix of EDM parameters as Ip = 10 A, Ton = 8 μs, Toff = 4 μs, PF = 20 kg/cm2 and Vs. = 32 V to achieve the measured response values as MRR = 0.00584 g/min, SR = 1.41 μm, TWR = 0.00118 g/min, PER = 0.0321 mm and PAR = 0.0411 mm. The corresponding regression equations correlating the considered responses and EDM parameters were also formulated which had been subsequently optimized using TLBO algorithm. Prabhakar et al. (2021) proposed the combined application of MOORA and ELECTRE methods to develop an integrated MCDM tool to optimize the EDM operation on titanium alloy. The influences of various input variables, like Ton, Toff and Ip on MRR, SR and TWR were investigated and it was concluded that an optimal intermix of EDM parameters as Ton = 60 µs, Toff = 8 µs and Ip = 7 A would provide the enhanced performance of the said EDM process.
5 Results and discussion
The main objective of this review paper is to explore the applications of various MCDM techniques as multi-objective optimization tools to determine the most appropriate combinations of input parameters for having enhanced machining performance of EDM processes. The shortlisted research works are extensively studied to extract the relevant information with respect to experimental design plans employed, dielectrics used, work materials machined, input parameters and responses considered along with their settings/measured values, MCDM tools deployed and their applications along with other mathematical techniques. The results of these analyses are graphically portrayed in Figures 2–4. It can be noticed from Figure 2A that 82.5% the past researches have preferred to conduct EDM experiments based on Taguchi methodology (i.e., L8, L9, L16, L18, L21, L25, L27 and L36) to examine the influences of different input parameters on the responses. This is because of its many advantageous features, like ability to provide robust design solutions with reduced experimental cost, inclusion of both qualitative and qualitative variables, easy mathematical steps, availability of user-friendly software, etc. Among various Taguchi’s OAs, L9 OA has been maximally utilized (39.4%), followed by L18 OA (13.9%) and L27 (13.1%) OA design plans. It is interestingly noticed that in the past studies, maximum number of EDM experiments has been carried out considering three parameters set at three different operating levels which have led to selection L9 OA as the most suitable design plan based on the computed degrees of freedom. Besides OAs, CCD (10.2%) and BBD (7.3%) have also been employed which would lead to development of the corresponding polynomial regression equations correlating EDM parameters and responses. Those equations have later been optimized using GA (2), NSGA-II (1), TLBO (2), MOPSO (1), QPSO (1), GP (1), FA (1) and CS (1) algorithms in the continuous solution space. The numerical value in the parenthesis indicates the number of occurrences of those techniques in the reviewed articles for EDM processes optimization.
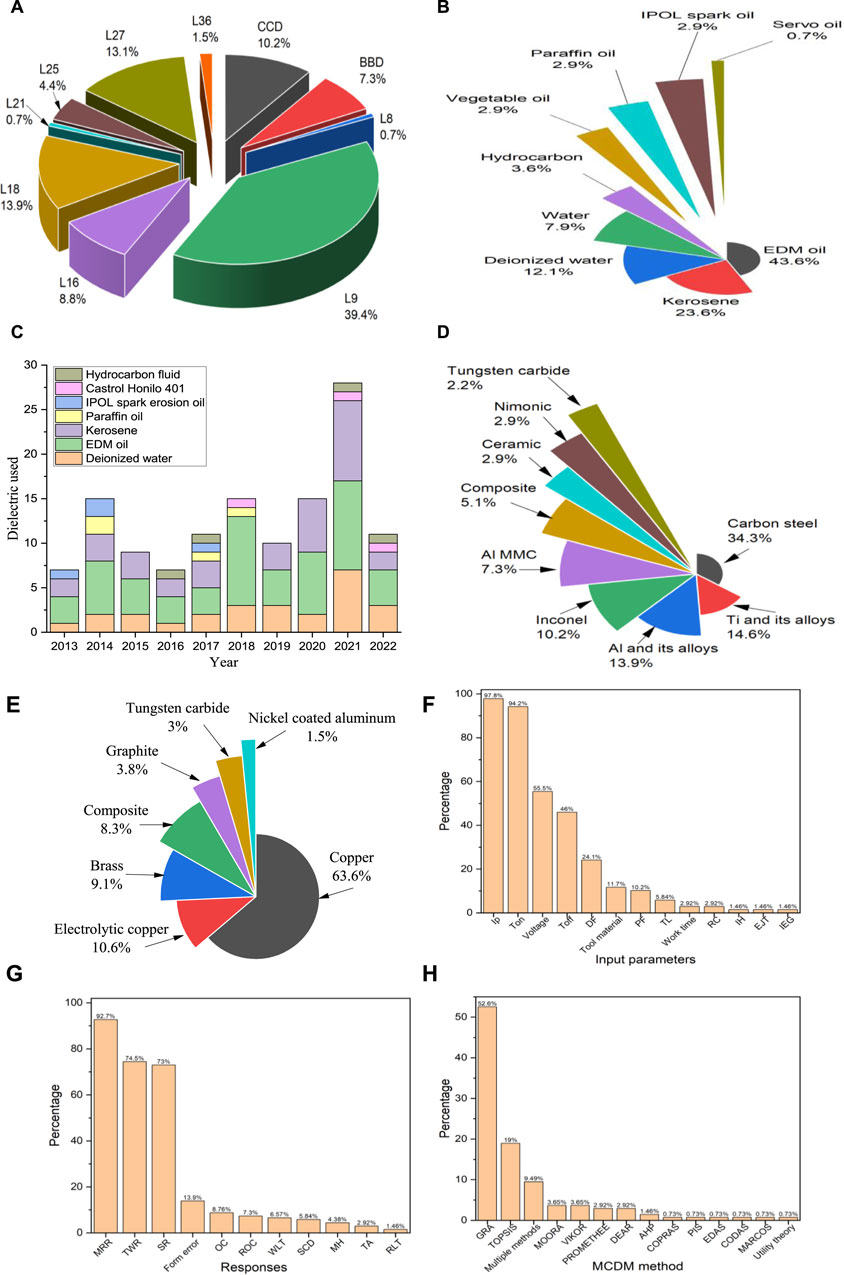
Figure 2. Graphical representation of the reviewed paper in terms of (A) design plan used (B) dielectric medium (C) trend of dielectric usage over the years (D) material machined (E) tool material (F) process parameter considered (G) response studied and (H) MCDM tools employed by the researchers.
Figure 2B provide information with respect to different types of dielectrics used by the past researchers. EDM oil (EDM-30, EDM SAE 40, EDM SE0501, EDM SAE 450, D323, HD-1, HD-11, etc.) has been the mostly utilized dielectric (43.6%), followed by deionized water (12.1%) and plain water (7.9%). To achieve sustainable and dry machining environment while avoiding the perilous effects of EDM oil, it is noticed that deionized water and plain water have emerged out as user-friendly and less hazardous dielectric fluids during EDM operations (Figure 2C). The EDM process is extremely suitable to generate complex shape geometries in many of the hard-to-cut engineering materials having higher strength-to-weight ratio. Figure 2D shows the applications of EDM processes in machining of some of the major work materials, such as medium and high carbon steels (34.3%) (making of shafts, crankshafts, axles, gears, couplings, forgings, etc.), titanium and its alloys (14.6%) (used in airplanes, missiles and rockets), aluminium and its alloys (13.9%) (used in electric and electronic industries, making of automotive and aerospace body structures, solar equipment), Inconel (10.2%) (making of propeller blades, propulsion motors, wire rope, sheathing for underwater communication cables), aluminium MMCs (7.3%) (huge applications in defence, aerospace, automotive and aviation industries), other composites (5.1%) (used in chemical, paper, oil and gas industries, water treatment plants), ceramics (2.9%) (making of bio-implants, body armours, spark plugs, fiber optics, race car brakes, chemical sensors), Nimonic (2.9%) (for gas turbine components, nuclear boiler tube supports, automobile exhaust valves) and tungsten carbide (2.2%) (mainly used as cutting tool material for various machining operations). Other work materials, like magnesium and its alloys, Zircaloy, Monel, brass, copper and Nitinol have also been occasionally machined using EDM processes, but are not shown in Figure 2D due to their least number of occurrences (≤2) in the reviewed articles.
Figure 2E illustrates the trend of tool material usage in EDM research. Copper stands out as the most commonly used tool material, being featured in 63.6% papers. This prevalence is likely due to copper’s excellent electrical conductivity and thermal properties, making it an effective electrode material for the EDM process. Electrolytic copper was used in 10.6% papers, is a refined version of copper that usually offers higher purity and, consequently, better performance in EDM. Brass was also used in 9.1% papers, is also a favorable choice due to its good machining properties and cost-effectiveness. Composite tool materials, used in 8.3% papers, indicate an interest in exploring the benefits of composite electrodes, such as improved wear resistance or specialized machining capabilities, which can be tailored by combining different materials. Graphite, though less frequently used, appearing in only 3.8% papers, offers advantages such as higher melting points and the ability to achieve finer finishes. Its lower popularity could be due to its brittleness and the challenges associated with handling and machining graphite electrodes. Tungsten carbide, mentioned in 3% papers, is known for its hardness and high wear resistance, making it suitable for precision machining, although its use is less due to higher material costs. Nickel-coated aluminum found use only in 1.5% papers, suggesting that it may be a relatively new area of exploration in EDM tool materials, possibly offering a combination of the lightweight properties of aluminum with the superior surface characteristics of a nickel coating.
The performance of EDM processes with respect to MRR, SR (average surface roughness value, Ra), TWR, form errors (flatness, squareness, CYL, CIR, PAR and PER), OC, ROC, etc., is noticed to be significantly affected by the considered input parameters. Thus, it is highly recommended to operate the EDM processes while setting those parameters at their optimal levels. The major input parameters taken into account by the past researchers during EDM of diverse work materials are exhibited in Figure 2F which reveals that Ip has been the most important parameter (97.8%), followed by Ton (94.2%), voltage (55.5%), Toff (46%), DF (24.1%), type of the tool material (11.7%), PF (10.2%) etc. On the other hand, Figure 2G provides information about some of the most important responses measured to evaluate the machining performance of EDM processes. MRR has been maximally considered (92.7%), which has been followed by TWR (74.5%), SR (73%), form errors (13.9%), OC (8.76%), ROC (7.3%), WLT (6.57%), SCD (5.84%), MH (4.38%), TA (2.92%) and RLT (1.46%) according to their descending importance to represent performance of the EDM processes. In all the reviewed articles, the value of SR has mainly been measured in terms of Ra (average surface roughness). Besides Ra, other roughness parameters, like root mean square roughness (Rq), skewness of surface profile (Rsk), kurtosis of surface profile (Rku), mean width of roughness profile (Rsm) and ten-point surface roughness (Rz) have also been occasionally considered to represent surface quality of the machined components. But, the correlations between those roughness parameters have never been evaluated. There are also some unimportant EDM parameters, like C, GC, Sg, RC, DT, etc., and responses, such as PFE, fractal dimension, RS, SEC, N, MC, taper ratio, PE, TC, etc., considered by the past researchers in their experimental studies which are not included while developing Figures 2F, G due to their least number of occurrences in the reviewed articles.
Figure 2H depicts the quantum of different MCDM tools deployed for optimization of EDM processes in the reviewed research articles. The GRA technique has found maximum applications (52.6%), followed by TOPSIS (9%), MOORA (3.65%), VIKOR (3.65%), PROMETHEE (2.92%) and DEAR (2.92%) methods. Other MCDM methods, such as AHP, COPRAS, PIS, EDAS, CODAS, MARCOS and utility theory have also been occasionally utilized for the same purpose. The high popularity of GRA is perhaps due to its simplicity and efficiency in handling uncertainty and incomplete data. GRA integrates smoothly with the Taguchi method and simplifies multivariate analysis by converting multiple performance measures into a single composite score. Further, GRA does not require the weighting of criteria which reduces the computational load for researchers. Although the researchers have preferred to assign equal importance to the responses under consideration during optimization of EDM processes using different MCDM tools mainly to reduce the computation complexity, several subjective techniques, like AHP (13) and Simo’s weighting method (1) and objective techniques, like PCA (7), EM (4), SDV (1), WPCA (1) and CRITIC (1) have been employed for estimating the relative importance of different responses. To deal with uncertainty involved during allotting criteria weights, fuzzy set theory (4) has also been combined with MCDM methods. Integration of fuzzy logic with MCDM methods (7) has helped the researchers to develop the corresponding “If’-Then” rules to explore the relationships between EDM parameters and responses.
Although the operating level of each of the EDM parameters largely depends on the type of the work material and feature to be machined, interaction between the work and tool materials and manufacturer and model of a particular EDM setup; based on the reviewed articles, an attempt is put forward in Figure 3 to portray the settings of four main EDM parameters, i.e., Ton, Toff, Ip and Vg, as considered by various past researchers, in the form of box plots. It is interesting to notice that those settings are widely varying, leading to large number of outliers towards higher levels of all the considered parameters. For Ton parameter, the lower and upper whisker values of the developed box plot are 0.5 μs and 463 μs respectively; while the corresponding mean and median values are 163 μs and 100 μs respectively (excluding the outliers). Similarly, the lower and upper whiskers, mean and median values for Toff, Ip and Vg can be obtained. It can be noted that for Toff, the mean of the box plot is well above the upper whisker indicating that a few studies have considered extreme high values for Toff during experimentation as compared to bulk of other studies. Thus, the mean value of the process parameter range in such cases is not a true representation of Toff considered by the past research community. Thus, it can be concluded from Figure 3 that the future researchers should attempt to set the corresponding values of Ton, Toff, Ip and Vg as 100 μs, 20 μs, 10 A and 50 V (all median values) respectively for having the best performance of an EDM process. It would eventually relieve the operators to conduct pilot runs or rely on trial-and-error approach for having the idea regarding the initial settings of different EDM parameters prior to any real-time experiment, thereby saving machining cost and time.
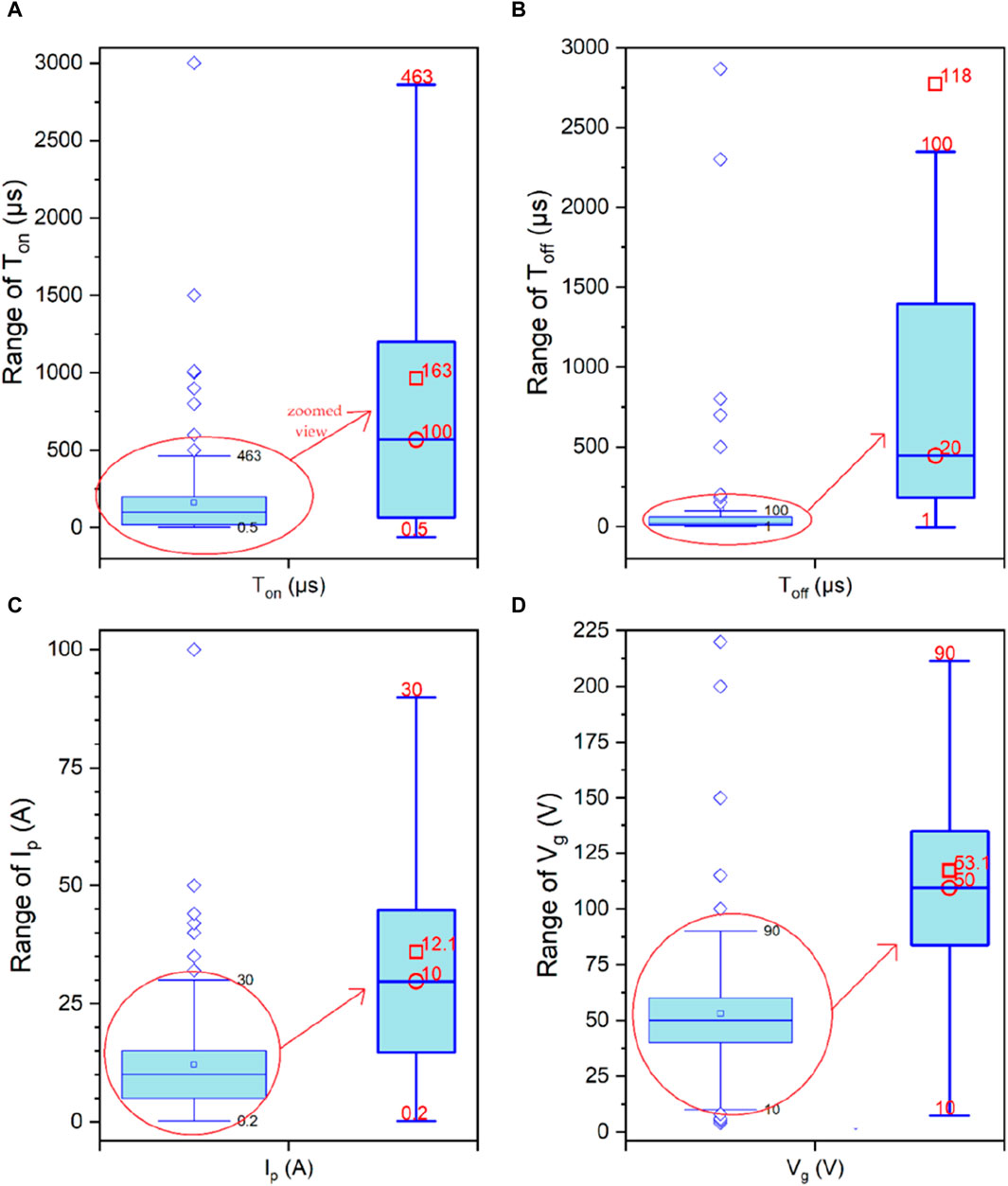
Figure 3. Ranges of main EDM parameters. (A) Pulse-on time (B) pulse-off time (C) paeak current (D) gap voltage.
In EDM process, different physical properties of the work material, machining time, material of the tool and operating levels of the input parameters under consideration significantly influence the achieved responses values. In Figure 4, values of three main responses, i.e., MRR, TWR and SR attained by the earlier researchers at the derived optimal intermixes of the considered EDM parameters are depicted in the form of box plots. While developing the box plots for MRR and TWR, their volumetric measured values are converted into corresponding gravimetric values and their unit is kept as mg/min. Those response values also widely vary depending on the type of the work material machined, tool material used and settings of the EDM parameters. For MRR, the values of lower and upper whiskers, mean and median are observed to be 0.001 mg/min, 357.332 mg/min, 157.710 mg/min and 35.706 mg/min respectively (excluding the outliers). On the other hand, those values for TWR are 0.002 mg/min, 23.294 mg/min, 16.000 mg/min and 1.361 mg/min respectively. In case of SR, lower and upper whiskers, mean and median values are obtained as 0.080 μm, 12.480 μm, 5.177 μm and 4.185 μm respectively. Thus, irrespective of the work and tool materials and input parameter settings, the achievable MRR, TWR and SR would be 35.706 mg/min, 1.361 mg/min and 4.185 μm respectively (considering their median values).
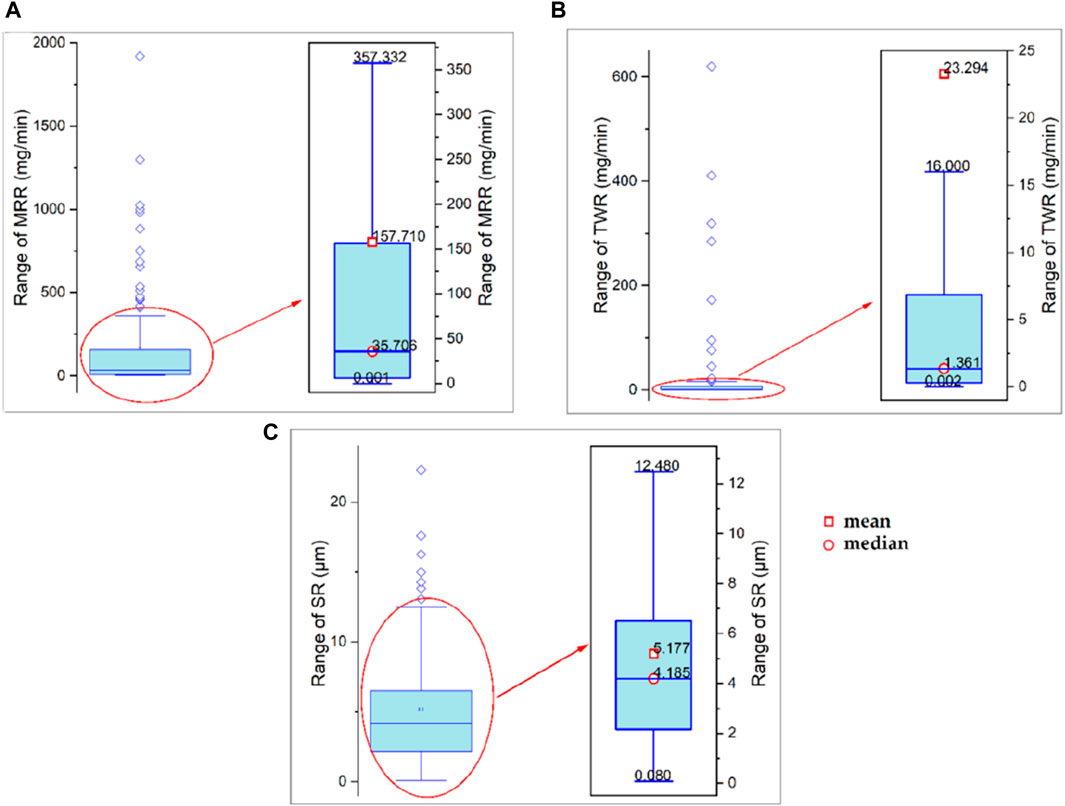
Figure 4. Ranges of main responses. (A) material removal rate (B) tool wear rate (C) surface roughness.
The co-citations of different keywords as considered in the reviewed articles with the focal keyword “Optimization of EDM process using MCDM methods” are plotted in Figure 5. From this figure, it can be interestingly unveiled that “Electrical discharges,” “Surface roughness,” “Material removal rate,” “Cutting tools,” “Multi-objective optimization,” “Wear of materials,” “Grey relational analysis,” “TOPSIS” and “Taguchi methods” are strongly related when different MCDM methods have been employed for optimizing the performance of EDM processes. Co-existence of other keywords, such as “Process parameters,” “Optimization,” “Tool wear rate,” “Electrodes,” “MCDM,” “L9 orthogonal arrays,” “Decision making,” “Current,” “Pulse-on time,” “Aluminium alloys,” “Titanium alloys” etc., also closely matches with the observations of this review paper.
6 Conclusions and future directions
Based on the information extracted after comprehensively reviewing 137 research articles published during 2013–2022 and available in the SCOPUS database, the following conclusions can be drawn:
a) Keeping in mind the widespread applications of EDM processes in many of the modern-day industries to generate complex shape features on diverse difficult-to-cut work materials, their optimization using MCDM methods appears as a topic of immense interest among the research community.
b) The major advantage of MCDM-based optimization of EDM processes lies in the fact that in most of the cases, the derived optimal combinations of the input parameters would be among the conducted experimental runs, relieving the machinists to perform additional experiments.
c) Taguchi’s L9 OA plan has been maximally employed by the past researchers to carry out EDM experiments. On the other hand, EDM oil has been the most preferred dielectric fluid and medium and high carbon steels have been mostly machined using EDM processes. With respect to input and output parameters, peak current has been the most important EDM parameter and MRR has been maximally considered to characterize the performance of EDM processes.
d) Due to its uncomplicated computational steps and independency of criteria weighting technique, GRA has appeared to be the most popular multi-objective optimization tool to determine the optimal parametric combinations of EDM processes. On the other hand, the earlier researchers have preferred application of AHP to measure weights of different responses under consideration.
e) With respect to four most important EDM parameters, i.e., Ton, Toff, Ip and Vg, the future researchers are advised to conduct EDM experiments while setting their corresponding operating values at 100 μs, 20 μs, 10 A and 50 V respectively.
f) Irrespective of the work and tool materials and ranges of the input parameters, the achievable values of the three most important responses, i.e., MRR, TWR and SR would be 35.706 mg/min, 1.361 mg/min and 4.185 μm respectively.
g) It would act as a valuable data repository to explore the stochastic behaviour of EDM processes and guide the future researchers in setting the operating levels of the main input parameters, relieving them to conduct pilot experiments while saving experimental cost and time.
h) “Optimization of EDM process using MCDM methods” is strongly interlinked with “Electrical discharges,” “Surface roughness,” “Material removal rate,” “Cutting tools,” “Multi-objective optimization,” “Wear of materials,” “Grey relational analysis,” “TOPSIS” and “Taguchi methods.”
This review on the applications of MCDM techniques for parametric optimization of EDM processes proposes the following future research directions:
a) Applications of various metaheuristics for optimizing EDM processes may be explored.
b) Further review may be conducted on MCDM techniques and metaheuristics deployed to derive the optimal performance of other traditional as well as non-traditional machining processes.
c) The scope of other newly developed but yet to be popular MCDM tools, like combined compromise solution (CoCoSo), multi-attributive ideal-real comparative analysis (MAIRCA), multi-attributive border approximation area comparison (MABAC) etc., may be exploited to optimize EDM processes.
d) It is advised to estimate the relative importance of the responses using objective weighting methods, like CRITIC, method based on the removal effects of criteria (MEREC) etc., to derive more pragmatic solutions.
e) Integration of MCDM methods with fuzzy set, intuitionistic fuzzy set, hesitant fuzzy set, neutrosophic fuzzy set etc., is highly encouraged involving multiple decision makers to qualitatively evaluate significance of the responses in uncertain group decision making environment.
f) The extracted information with respect to process parameter settings and achieved response values may be treated as the inputs to any of the machine learning algorithms to design and develop the corresponding predictive models.
g) Future research should explore the integration of machine learning with MCDM techniques to enhance predictive accuracy and process optimization.
h) Additionally, investigating environmentally friendly EDM processes aligns with global sustainability goals, presenting a critical avenue for further studies.
The major limitations of this paper are consideration of only MCDM techniques for optimizing EDM processes as a review topic, exclusion of conference papers and book chapters from the scope of review, omitting the derived optimal values of EDM parameters from further analysis (due to lack of exact information) and dependency on only SCOPUS database.
Author contributions
DP: Conceptualization, Data curation, Formal Analysis, Investigation, Methodology, Validation, Writing–original draft, Writing–review and editing. KK: Conceptualization, Funding acquisition, Methodology, Visualization, Writing–review and editing. SC: Conceptualization, Methodology, Project administration, Resources, Supervision, Validation, Writing–original draft, Writing–review and editing. RČ: Conceptualization, Funding acquisition, Project administration, Resources, Writing–review and editing.
Funding
The author(s) declare financial support was received for the research, authorship, and/or publication of this article. The article has been done in connection with the project Students Grant Competition SP2024/087, “Specific Research of Sustainable Manufacturing Technologies” financed by the Ministry of Education, Youth and Sports and Faculty of Mechanical Engineering VŠB-TUO.
Conflict of interest
The authors declare that the research was conducted in the absence of any commercial or financial relationships that could be construed as a potential conflict of interest.
The author(s) declared that they were an editorial board member of Frontiers, at the time of submission. This had no impact on the peer review process and the final decision.
Publisher’s note
All claims expressed in this article are solely those of the authors and do not necessarily represent those of their affiliated organizations, or those of the publisher, the editors and the reviewers. Any product that may be evaluated in this article, or claim that may be made by its manufacturer, is not guaranteed or endorsed by the publisher.
References
Akgün, M. (2022). Performance analysis of electrode materials in electro discharge machining of monel K-500. Surf. Topogr. Metrology Prop. 10, 035026. doi:10.1088/2051-672x/ac8d19
Alagarsamy, S. V., Raveendran, P., and Ravichandran, M. (2021). Investigation of material removal rate and tool wear rate in spark erosion machining of Al-Fe-Si alloy composite using Taguchi coupled TOPSIS approach. Silicon 13, 2529–2543. doi:10.1007/s12633-020-00596-x
Anand, G., Satyanarayana, S., and Hussain, M. M. (2017). Optimization of process parameters in EDM with magnetic field using grey relational analysis with Taguchi technique. Mater. Today Proc. 4, 7723–7730. doi:10.1016/j.matpr.2017.07.107
Aravindan, N., Kumar, U. A., and Laxminarayana, P. (2018). Multi response optimisation of EDM parameters for micro channels machining of SS 316 with Taguchi-GRA. Mater. Today Proc. 5, 27028–27035. doi:10.1016/j.matpr.2018.09.006
Ay, M., Çaydaş, U., and Hasçalık, A. (2013). Optimization of micro-EDM drilling of Inconel 718 superalloy. Int. J. Adv. Manuf. Technol. 66, 1015–1023. doi:10.1007/s00170-012-4385-8
Behzadian, M., Otaghsara, S. K., Yazdani, M., and Ignatius, J. (2012). A state-of the-art survey of TOPSIS applications. Expert Syst. Appl. 39, 13051–13069. doi:10.1016/j.eswa.2012.05.056
Bhattacharjee, B., Biswas, N., Rai, R. N., Choudhuri, K., Saha, A., Das, A., et al. (2022). Stir cast Al-Cu-SiCp metal matrix composites: effect of SiC particles on the mechanical properties and optimization of EDM process parameters. J. Institution Eng. Ser. D, 601–610. doi:10.1007/s40033-022-00352-y
Bhosale, S. B., Bhowmik, S., and Ray, A. (2021). Evaluation of machinability and recast layer analysis of ferrous clay composite through electric discharge machining process. Arabian J. Sci. Eng. 47, 8523–8533. doi:10.1007/s13369-021-06337-5
Biswal, S., Sahoo, B. B., Jeet, S., Barua, A., Kumari, K., Naik, B., et al. (2022). Experimental investigation based on MCDM optimization of electrical discharge machined Al-WC-B4C hybrid composite using Taguchi-MARCOS method. Mater. Today Proc. 74, 587–594. doi:10.1016/j.matpr.2022.09.522
Bodukuri, A. K., and Kesha, E. (2021). Multi-attribute optimization of EDM process parameters for machining of SiC and B4C particle reinforced Al 6061 metal matrix composite adopting TOPSIS method. Int. J. Adv. Technol. Eng. Explor. 8, 735–752. doi:10.19101/ijatee.2021.874132
Brans, J. P., and Vincke, P. (1985). Note—a preference ranking organisation method: (the PROMETHEE method for multiple criteria decision-making). Manag. Sci. 31, 647–656. doi:10.1287/mnsc.31.6.647
Brauers, W. K. M., Zavadskas, E. K., Peldschus, F., and Turskis, Z. (2008). Multi-objective decision-making for road design. Transport 23, 183–193. doi:10.3846/1648-4142.2008.23.183-193
Chakraborty, S., Chatterjee, P., and Das, P. P. (2023) Multi-criteria decision making methods in manufacturing environment: models and applications. India: CRC Press: New York, NY.
Chakraborty, S., Dey, V., and Ghosh, S. K. (2015). A review on the use of dielectric fluids and their effects in electrical discharge machining characteristics. Precis. Eng. 40, 1–6. doi:10.1016/j.precisioneng.2014.11.003
Chandrashekarappa, M. P. G., Kumar, S., Pimenov, D. Y., and Jagadish, G. K. (2021). Experimental analysis and optimization of EDM parameters on HcHcr steel in context with different electrodes and dielectric fluids using hybrid Taguchi-based PCA-utility and CRITIC-utility approaches. Metals 11, 419. doi:10.3390/met11030419
Chaudhury, P., and Samantaray, S. (2020). Multi-optimization of process parameters for machining of a non-conductive SiC ceramic composite by non-conventional machining method. Manuf. Rev. 7, 32. doi:10.1051/mfreview/2020027
Chauhan, N. K., Das, A. K., and Rajesha, S. (2018). Optimization of process parameters using grey relational analysis and taguchi method during micro-EDMing. Mater. Today Proc. 5, 27178–27184. doi:10.1016/j.matpr.2018.09.029
Das, P. P., Gupta, P., Das, S., Pradhan, B. B., and Chakraborty, S. (2018). Application of grey-fuzzy approach in parametric optimization of EDM process in machining of MDN 300 steel. Mater. Sci. Eng. 297, 012013. doi:10.1088/1757-899x/297/1/012013
Dastagiri, M., Rao, P. S., and Valli, P. M. (2018). Optimization of EDM process parameters by using heuristic approach. Mater. Today Proc. 5, 27036–27042. doi:10.1016/j.matpr.2018.09.007
Debnath, S., and Ghosh, S. (2021). Experimental investigation of Electro discharge machining process by AHP-MOORA technique. J. Industrial Eng. Decis. Mak. 2 (1), 1–7. doi:10.31181/jiedm200201001d
Dewan, P. R., Lepcha, L. P., Khaling, A. N., Prasad, N., and Rai, S. (2018). Experimental analysis and optimization of EDM process parameters. Mater. Sci. Eng. 377, 012220. doi:10.1088/1757-899x/377/1/012220
Dewangan, S., and Biswas, C. K. (2013). Optimisation of machining parameters using grey relation analysis for EDM with impulse flushing. Int. J. Mechatronics Manuf. Syst. 6, 144–158. doi:10.1504/ijmms.2013.053826
Dewangan, S., Gangopadhyay, S., and Biswas, C. K. (2015a). Study of surface integrity and dimensional accuracy in EDM using fuzzy TOPSIS and sensitivity analysis. Measurement 63, 364–376. doi:10.1016/j.measurement.2014.11.025
Dewangan, S., Gangopadhyay, S., and Biswas, C. K. (2015b). Multi-response optimization of surface integrity characteristics of EDM process using grey-fuzzy logic-based hybrid approach. Eng. Sci. Technol. Int. J. 18, 361–368. doi:10.1016/j.jestch.2015.01.009
Dey, A., Shrivastav, M., and Kumar, P. (2021). Optimum performance evaluation during machining of Al6061/cenosphere AMCs using TOPSIS and VIKOR based multi-criteria approach. Proc. Institution Mech. Eng. Part B J. Eng. Manuf. 235, 2174–2188. doi:10.1177/0954405420958770
Fatatit, A. Y., and Kalyon, A. (2021). Determination of multi-performance characteristics in electric discharge machining of DIN 1.2767 steel using grey relational analysis. Sci. J. Koya Univ. 9, 1–7. doi:10.14500/aro.10718
Gaikwad, S., Teli, S., and Gaikwad, L. (2014). Optimization of EDM parameters on machining Ti-6Al-4V with a core electrode using grey relational analysis. Int. J. Res. Aeronautical Mech. Eng. 2, 24–31.
Ganesh, N., Ghadai, R., Bhoi, A. K., Kalita, K., and Gao, X. Z. (2020). An intelligent predictive model-based multi-response optimization of EDM process. Comput. Model. Eng. Sci. 124, 459–476. doi:10.32604/cmes.2020.09645
Gangil, M., and Pradhan, M. K. (2018a). Optimization the machining parameters by using VIKOR method during EDM process of titanium alloy. Mater. Today Proc. 5, 7486–7495. doi:10.1016/j.matpr.2017.11.420
Gangil, M., and Pradhan, M. K. (2018b). Optimization of machining parameters of EDM for performance characteristics using RSM and GRA. J. Mech. Eng. Biomechanics 2, 27–33. doi:10.24243/jmeb/2.4.169
Ghorabaee, M. K., Zavadskas, E. K., Turskis, Z., and Antucheviene, J. (2016). A new combinative distance-based assessment (CODAS) method for multi-criteria decision-making. Econ. Comput. Econ. Cybern. Stud. Res. 50 (3), 25–44.
Gopalakrishnan, S., senthilvelan, T., and Ranganathan, S. (2013). Statistical optimization of EDM parameters on machining of aluminum hybrid metal matrix composite by applying Taguchi based grey analysis. J. Sci. Industrial Res. 72, 358–365.
Gouda, D., Panda, A., Nanda, B. K., Kumar, R., Sahoo, A. K., and Routara, B. C. (2021). Recently evaluated electrical discharge machining (EDM) process performances: a research perspective. Mater. Today Proc. 44, 2087–2092. doi:10.1016/j.matpr.2020.12.180
Gowthaman, S., Balamurugan, K., Kumar, P. M., Ali, S. A., Kumar, K. M., and Gopal, N. V. R. (2018). Electrical discharge machining studies on Monel-super alloy. Procedia Manuf. 20, 386–391. doi:10.1016/j.promfg.2018.02.056
Hanif, M., Ahmad, W., Hussain, S., Jahanzaib, M., and Shah, A. H. (2019). Investigating the effects of electric discharge machining parameters on material removal rate and surface roughness on AISI D2 steel using RSM-GRA integrated approach. Int. J. Adv. Manuf. Technol. 101, 1255–1265. doi:10.1007/s00170-018-3019-1
Hema, P., Naveena, K., and Chaitanya, Y. (2022). Parametric optimization of process parameters on performance characteristics using die-sinking EDM with deionized water and kerosene as dielectrics. Mater. Today Proc. 62, 655–664. doi:10.1016/j.matpr.2022.03.629
Ho, K. H., and Newman, S. T. (2003). State of the art electrical discharge machining (EDM). Int. J. Mach. Tools Manuf. 43, 1287–1300. doi:10.1016/s0890-6955(03)00162-7
Huo, J., Liu, S., Wang, Y., Muthuramalingam, T., and Pi, V. N. (2019). Influence of process factors on surface measures on electrical discharge machined stainless steel using TOPSIS. Mater. Res. Express 6, 086507. doi:10.1088/2053-1591/ab1ae0
Huu Phan, N., and Muthuramalingam, T. (2021). Multi criteria decision making of vibration assisted EDM process parameters on machining silicon steel using Taguchi-DEAR methodology. Silicon 13 (6), 1879–1885. doi:10.1007/s12633-020-00573-4
Jampana, V. N. R., Ramana Rao, P. S. V., and Kumar, A. (2022). Bi-objective optimization of process parameters in electric discharge machining of SS630 using grey relation analysis. J. Nanomater. 2022, 1–11. doi:10.1155/2022/1165750
Jana, A. K., Kumar, R. R., Mohanty, S. C., Rao, K. M., Shanker, V. G., and Reddy, A. Y. (2021). Parametric optimization of die sinking EDM in AISI D2 steel considering Canola oil as dielectric using TOPSIS and GRA. Mater. Sci. Eng. 1057, 012061. doi:10.1088/1757-899x/1057/1/012061
Kaklauskas, A., Zavadskas, E. K., Raslanas, S., Ginevicius, R., Komka, A., and Malinauskas, P. (2006). Selection of low e-windows in retrofit of public buildings by applying multiple criteria method COPRAS: a Lithuanian case. Energy Build. 38, 454–462. doi:10.1016/j.enbuild.2005.08.005
Kalita, K., Chakraborty, S., Ghadai, R. K., and Chakraborty, S. (2022). Parametric optimization of non-traditional machining processes using multi-criteria decision making techniques: literature review and future directions. Multiscale Multidiscip. Model. Exp. Des. 6, 1–40. doi:10.1007/s41939-022-00128-7
Karmiris-Obratański, P., Papazoglou, E. L., Leszczyńska-Madej, B., Karkalos, N. E., and Markopoulos, A. P. (2022). An optimization study on the surface texture and machining parameters of 60CrMoV18-5 steel by EDM. Materials 16, 3559. doi:10.3390/ma15103559
Kasdekar, D. K., and Parashar, V. (2015). MADM approach for optimization of multiple responses in EDM of En-353 steel. Int. J. Adv. Sci. Technol. 83, 59–70. doi:10.14257/ijast.2015.83.06
Keshavarz, G. M., Zavadskas, E. K., Olfat, L., and Turskis, Z. (2015). Multi-criteria inventory classification using a new method of evaluation based on distance from average solution (EDAS). Informatica 26, 435–451. doi:10.15388/informatica.2015.57
Kolahan, F., and Moghaddam, M. A. (2015). The use of Taguchi method with grey relational analysis to optimize the EDM process parameters with multiple quality characteristics. Sci. Iran. 22, 530–538.
Kolli, M., and Adepu, K. (2016). Optimization of the parameters for the surfactant-added EDM of a Ti-6Al-4V alloy using the GRA-Taguchi method. Mater. Technol. 50, 229–238. doi:10.17222/mit.2014.249
Kumar, A., and Rai, R. N. (2020). Grey-Taguchi and TOPSIS-Taguchi-based optimisation of performance parameters of spark EDM on heat-treated AA7050/5 B4C composite. J. Institution Eng. Ser. D 101, 71–79. doi:10.1007/s40033-020-00211-8
Kumar, A., Vivekananda, K., and Ram Prasad, A. V. S. (2022). Experimental investigation and parametric optimization during EDM of titanium grade 9 using MOORA-fuzzy integrated multi-objective genetic algorithm. Mater. Today Proc. 62, 4473–4479. doi:10.1016/j.matpr.2022.04.938
Kumar, D., and Mondal, S. (2020). Process parameters optimization of AISI M2 steel in EDM using Taguchi based TOPSIS and GRA. Mater. Today Proc. 26, 2477–2484. doi:10.1016/j.matpr.2020.02.527
Kumar, D., Singh, N. K., and Bajpai, V. (2020). Recent trends, opportunities and other aspects of micro-EDM for advanced manufacturing: a comprehensive review. J. Braz. Soc. Mech. Sci. Eng. 42, 222. doi:10.1007/s40430-020-02296-4
Kumar, J., Gautam, S., Rajput, S. K., and Soota, T. (2021b). Ultraviolet-B radiation stress-induced toxicity and alterations in proteome of deinococcus radiodurans. J. Eng. Res. Special Issue 31, 1–15. doi:10.1159/000512018
Kumar, J., and Soota, T. (2020). Multi-response optimization of machining parameter for Zircaloy by response surface methodology and grey relation analysis. Mater. Today Proc. 21, 1544–1550. doi:10.1016/j.matpr.2019.11.084
Kumar, K., Kumar, J., and Verma, R. K. (2021a). Experimental investigations in electric discharge machining of Inconel-X 750 using hybrid optimization approach. Int. J. Mod. Manuf. Technol. 13, 96–105.
Kumar, P., Barua, P. B., and Gaindhar, J. L. (2000). Quality optimization (multi-characteristics) through Taguchi’s technique and utility concept. Qual. Reliab. Eng. Int. 16, 475–485. doi:10.1002/1099-1638(200011/12)16:6<475::aid-qre342>3.0.co;2-0
Kumar, P., and Edwin, J. J. (2021). Experimental investigation on electric discharge machining of high carbon-high chromium tool steel. Int. J. Mech. Eng. 6, 426–433.
Kumar, R., Roy, S., Gunjan, P., Sahoo, A., Sarkar, D. D., and Das, R. K. (2018). Analysis of MRR and surface roughness in machining Ti-6Al-4V ELI titanium alloy using EDM process. Procedia Manuf. 20, 358–364. doi:10.1016/j.promfg.2018.02.052
Kumar, S., and Dhanabalan, S. (2020). Form tolerance analysis and multi-parametric optimization of meso deep square hole EDMed on Inconel-718 plate using GRA method. Grey Syst. Theory Appl. 11, 664–680. doi:10.1108/gs-07-2019-0022
Kumar, S., Uthayakumar, M., Kumaran, S. T., Parameswaran, P., and Mohandas, E. (2014). Electrical discharge machining of Al (6351)-5% SiC-10% B4C hybrid composite: a grey relational approach. Model. Simul. Eng. 2014, 1–7. doi:10.1155/2014/426718
Kumar, S. V., and Kumar, M. P. (2014). Optimization of cryogenic cooled EDM process parameters using grey relational analysis. J. Mech. Sci. Technol. 28, 3777–3784. doi:10.1007/s12206-014-0840-9
Kuo, Y., Yang, T., and Huang, G.-W. (2008). The use of grey relational analysis in solving multiple attribute decision-making problems. Comput. Industrial Eng. 55, 80–93. doi:10.1016/j.cie.2007.12.002
Laxman, J., and Raj, K. G. (2014). Optimization of EDM process parameters on titanium super alloys based on the grey relational analysis. Int. J. Eng. Res. 3, 344–348. doi:10.17950/ijer/v3s5/513
Leão, F. N., and Pashby, I. R. (2004). A review on the use of environmentally-friendly dielectric fluids in electrical discharge machining. J. Mater. Process. Technol. 149, 341–346. doi:10.1016/j.jmatprotec.2003.10.043
Liao, H. C., and Chen, Y. K. (2002). Optimizing multi-response problem in the Taguchi method by DEA based ranking method. Int. J. Qual. Reliab. Manag. 19, 825–837. doi:10.1108/02656710210434766
Liu, L., Thangaraj, M., Karmiris-Obratański, P., Zhou, Y., Annamalai, R., Machnik, R., et al. (2022). Optimization of wire EDM process parameters on cutting inconel 718 alloy with zinc-diffused coating brass wire electrode using Taguchi-DEAR technique. Coatings 12 (11), 1612. doi:10.3390/coatings12111612
Mandal, P., and Mondal, S. C. (2021). Multi-objective optimization of Cu-MWCNT composite electrode in electro discharge machining using MOPSO-TOPSIS. Measurement 169, 108347. doi:10.1016/j.measurement.2020.108347
Manivannan, R., and Kumar, M. P. (2016). Multi-response optimization of micro-EDM process parameters on AISI304 steel using TOPSIS. J. Mech. Sci. Technol. 30, 137–144. doi:10.1007/s12206-015-1217-4
Manivannan, R., and Kumar, P. (2013). Multi response optimization of process parameters in electrical discharge machining in AISI D2 tool steel using grey relational analysis. J. Manuf. Eng. 8, 22–27.
Maniya, K., and Bhatt, M. G. (2010). A selection of material using a novel type decision-making method: preference selection index method. Mater. Des. 31, 1785–1789. doi:10.1016/j.matdes.2009.11.020
Marichamy, S., Saravanan, M., Ravichandran, M., and Veerappan, G. (2016). Parametric optimization of electrical discharge machining process on α-β brass using grey relational analysis. J. Mater. Res. 31, 2531–2537. doi:10.1557/jmr.2016.213
Matharou, G. S., and Bhuyan, B. K. (2020). Parametric optimization of EDM processes for aluminum hybrid metal matrix composite using GRA-PCA approach. Int. J. Mech. Prod. Eng. Res. Dev. 10, 367–378. doi:10.24247/ijmperdjun202034
Mazarbhuiya, R. M., Choudhury, P. K., and Rahang, M. (2016). Taguchi-grey relational based multi-objective optimization of process parameters in electric discharge machining of aluminium with copper electrode. J. Basic Appl. Eng. Res. 3, 1169–1171.
Mazarbhuiya, R. M., and Rahang, M. (2020). Surface modification of aluminium and its parametric optimization using grey relational analysis. Mater. Today Proc. 26, 1594–1597. doi:10.1016/j.matpr.2020.02.327
Meena, V. K., Azad, M. S., Singh, S., and Singh, N. (2017). Micro-EDM multiple parameter optimization for Cp titanium. Int. J. Adv. Manuf. Technol. 89, 897–904. doi:10.1007/s00170-016-9130-2
Mhatre, M. S., Sapkal, S. U., and Pawade, R. S. (2014). Electro discharge machining characteristics of Ti-6Al-4V alloy: a grey relational optimization. Procedia Mater. Sci. 5, 2014–2022. doi:10.1016/j.mspro.2014.07.534
Miller, D. W., and Starr, M. K. (1969) Executive decisions and operations research. Englewood Cliffs, New Jersey: Prentice-Hall, Inc.,
Ming, W., Xie, Z., Ma, J., Du, J., Zhang, G., Cao, C., et al. (2021). Critical review on sustainable techniques in electrical discharge machining. J. Manuf. Process. 72, 375–399. doi:10.1016/j.jmapro.2021.10.035
Mishra, B. P., and Routara, B. C. (2017). An experimental investigation and optimisation of performance characteristics in EDM of EN-24 alloy steel using Taguchi method and grey relational analysis. Mater. Today Proc. 4, 7438–7447. doi:10.1016/j.matpr.2017.07.075
Mohanty, C. P., Satpathy, M. P., Mahapatra, S. S., and Singh, M. R. (2018). Optimization of cryo-treated EDM variables using TOPSIS-based TLBO algorithm. Sādhanā 43, 51. doi:10.1007/s12046-018-0829-7
Mohanty, U. K., and Rana, J. (2015). Parametric optimization of powder EDM process using grey relational analysis. J. Material Sci. Mech. Eng. 2, 248–253.
Mohanty, U. K., Rana, J., and Sharma, A. (2017). Multi-objective optimization of electro-discharge machining (EDM) parameter for sustainable machining. Mater. Today Proc. 4, 9147–9157. doi:10.1016/j.matpr.2017.07.271
Moharana, B. R., and Patro, S. S. (2019). Multi objective optimization of machining parameters of EN-8 carbon steel in EDM process using GRA method. Int. J. Mod. Manuf. Technol. XI, 50–56.
Muthuramalingam, T., and Mohan, B. (2013). Taguchi-grey relational based multi response optimization of electrical process parameter in electrical discharge machining. Indian J. Eng. Material Sci. 20, 471–475.
Muthuramalingam, T., and Mohan, B. (2014). Application of Taguchi-grey multi responses optimization on process parameters in electro erosion. Measurement 58, 495–502. doi:10.1016/j.measurement.2014.09.029
Muthuramalingam, T., and Mohan, B. (2015). A review on influence of electrical process parameters in EDM process. Achieves Civ. Mech. Eng. 15, 87–94. doi:10.1016/j.acme.2014.02.009
Nadda, R., Kumar, R., Singh, T., Chauhan, R., Patnaik, A., and Gangil, B. (2018). Experimental investigation and optimization of cobalt bonded tungsten carbide composite by hybrid AHP-TOPSIS approach. Alexandria Eng. J. 57, 3419–3428. doi:10.1016/j.aej.2018.07.013
Nayak, S., and Routara, B. C. (2014). Optimization of multiple performance characteristics in electro discharge machining using grey relational analysis. Int. J. Sci. Technol. Res. 3, 116–121.
Nguyen, P. H., Banh, T. L., Mashood, K. A., Tran, D. Q., Pham, D. V., Muthuramalingam, T., et al. (2020). Application of TGRA-based optimisation for machinability of high-chromium tool steel in the EDM process. Arabian J. Sci. Eng. 45, 5555–5562. doi:10.1007/s13369-020-04456-z
Okponyia, K. O., and Oke, S. A. (2020). Exploring aluminum alloy metal matrix composites in EDM using coupled factor-level-present worth analysis and fuzzy analytic hierarchy process. Int. J. Industrial Eng. Eng. Manag. 2, 25–44. doi:10.24002/ijieem.v2i1.3781
Opricovic, S., and Tzeng, G. H. (2004). Compromise solution by MCDM methods: a comparative analysis of VIKOR and TOPSIS. Eur. J. Operational Res. 156 (2), 445–455. doi:10.1016/s0377-2217(03)00020-1
Palanisamy, A., Rekha, R., Sivasankaran, S., and Sathiya Narayanan, C. (2014). Multi-objective optimization of EDM parameters using grey relational analysis for titanium alloy (Ti-6Al-4V). Appl. Mech. Mater. 592-594, 540–544.
Pandey, A. K., and Anas, M. (2022). Sustainability and recent trends in micro-electric discharge machining (μ-EDM): a state-of-the-art review. Mater. Today Proc. 57, 2049–2055. doi:10.1016/j.matpr.2021.11.250
Pandiyan, G. K., Prabaharan, T., Jafrey, D. J. D., and Sivalingam, V. (2022). Machinability analysis and optimization of electrical discharge machining in AA6061-T6/15wt.% SiC composite by the multi-criteria decision-making approach. J. Mater. Eng. Perform. 31, 3741–3752. doi:10.1007/s11665-021-06511-8
Patel, R. K., and Pradhan, M. K. (2021). Decision making on the machining parameters of electrical discharge machined AISI D2 tool steel by AHP and PROMETHEE method. Mater. Sci. Eng. 1104, 012011. doi:10.1088/1757-899x/1104/1/012011
Patnaik, P. K., Mishra, S. K., Swain, P. T. R., Purohit, A., Parija, S. K., and Panda, S. S. (2022). Multi-objective optimization and experimental analysis of electro-discharge machining parameters via gray-Taguchi, TOPSIS-Taguchi and PSI-Taguchi methods. Mater. Today Proc. 62, 6189–6198. doi:10.1016/j.matpr.2022.05.087
Paul, T. R., Saha, A., Majumder, H., Dey, V., and Dutta, P. (2019). Multi-objective optimization of some correlated process parameters in EDM of Inconel 800 using a hybrid approach. J. Braz. Soc. Mech. Sci. Eng. 41, 300. doi:10.1007/s40430-019-1805-9
Payal, H., Bharti, P. S., Maheshwari, S., and Agarwal, D. (2020). Machining characteristics and parametric optimisation of Inconel 825 during electric discharge machining. Teh. Vjesn. 27, 761–772. doi:10.17559/TV-20190214135509
Phan, N. H., Dong, P. V., Dung, H. T., Thien, N. V., Muthuramalingam, T., Shirguppikar, S., et al. (2021). Multi-object optimization of EDM by Taguchi-DEAR method using AlCrNi coated electrode. Int. J. Adv. Manuf. Technol. 116, 1429–1435. doi:10.1007/s00170-021-07032-3
Phan, N. H., Muthuramalingam, T., Minh, N. D., and Van Duc, N. (2022a). Enhancing surface morphology of machined SKD61 die steel in EDM process using DEAR approach based multi criteria decision making. Int. J. Interact. Des. Manuf. (IJIDeM) 16 (3), 1155–1161. doi:10.1007/s12008-022-00859-4
Phan, N. H., Vu, N. N., Shirguppikar, S., Ly, N. T., Tam, N. C., Tai, B. T., et al. (2022b). Multi-criteria decision making in electrical discharge machining with nickel coated aluminium electrode for titanium alloy using preferential selection index. Manuf. Rev. 9, 13–10. doi:10.1051/mfreview/2022010
Phimoolchat, J., and Muttamara, A. (2020). Multi-objective optimization of electrical discharge machining parameters for 2024 aluminum alloy using grey-Taguchi method. Mater. Sci. Forum 998, 55–60.
Prabhakar, M., Ranjith, R., and Venkatesan, S. (2021). Characterization of electric discharge machining of titanium alloy utilizing MEIOT technique for orthopedic implants. Mater. Res. Express 8, 086505. doi:10.1088/2053-1591/ac1a2d
Prabhu, S., and Vinayagam, B. K. (2015). Adaptive neuro fuzzy inference system modelling of multi-objective optimisation of electrical discharge machining process using single-wall carbon nanotubes. Aust. J. Mech. Eng. 13, 97–117. doi:10.7158/m13-074.2015.13.2
Prabhu, S., and Vinayagam, B. K. (2016). Multiresponse optimization of EDM process with nanofluids using TOPSIS method and genetic algorithm. Archive Mech. Eng. 63, 45–71. doi:10.1515/meceng-2016-0003
Pradhan, M. K. (2013a). Estimating the effect of process parameters on MRR, TWR and radial overcut of EDMed AISI D2 tool steel by RSM and GRA coupled with PCA. Int. J. Adv. Manuf. Technol. 68, 591–605. doi:10.1007/s00170-013-4780-9
Pradhan, M. K. (2013b). Estimating the effect of process parameters on surface integrity of EDMed AISI D2 tool steel by response surface methodology coupled with grey relational analysis. Int. J. Adv. Manuf. Technol. 67, 2051–2062. doi:10.1007/s00170-012-4630-1
Pradhan, M. K. (2018). Optimisation of EDM process for MRR, TWR and radial overcut of D2 steel: a hybrid RSM-GRA and entropy weight-based TOPSIS approach. Int. J. Industrial Syst. Eng. 29, 273–302. doi:10.1504/ijise.2018.10013960
Pragadish, N., Kaliappan, S., Subramanian, M., Natrayan, L., Satish Prakash, K., Ram Subbiah, R., et al. (2022). Optimization of cardanol oil dielectric-activated EDM process parameters in machining of silicon steel. Biomass Convers. Biorefnery 13, 14087–14096. doi:10.1007/s13399-021-02268-1
Prakash, V., Kumar, P., Singh, P. K., Hussain, M., Das, A. K., and S Chattopadhyaya, D. (2019). Micro-electrical discharge machining of difficult-to-machine materials: a review. Proc. Inst. Mech. Eng. Part B J. Eng. Manuf. 233, 339–370. doi:10.1177/0954405417718591
Pramanik, A., Basak, A. K., Littlefair, G., Debnath, S., Prakash, C., Singh, M. A., et al. (2020). Methods and variables in electrical discharge machining of titanium alloy - a review. Heliyon 6, e05554. doi:10.1016/j.heliyon.2020.e05554
Priyadarshini, M., and Pal, K. (2015). Grey-Taguchi based optimization of EDM process for titanium alloy. Mater. Today Proc. 2, 2472–2481. doi:10.1016/j.matpr.2015.07.188
Radhika, N., Chandran, G. K., Shivaram, P., and Karthik, K. T. (2015). Multi-objective optimization of EDM parameters using grey relation analysis. J. Eng. Sci. Technol. 10, 1–11.
Raj, S., Rahul, S. H. R., Shivani, D. S., Guha, S., Aazim, B. D., Routara, B. C., et al. (2020). Optimization of surface topography of electro-discharge machined super alloy Inconel 825: using TOPSIS integrated with Taguchi philosophy. Mater. Today Proc. 24, 218–224. doi:10.1016/j.matpr.2020.04.270
Raj, S. O. N., and Prabhu, S. (2017). Analysis of multi objective optimisation using TOPSIS method in EDM process with CNT infused copper electrode. Int. J. Mach. Mach. Mater. 19, 76–94. doi:10.1504/ijmmm.2017.10002091
Rajamanickam, S., and Prasanna, J. (2019). Multi objective optimization during small hole electrical discharge machining (EDM) of Ti-6Al-4V using TOPSIS. Mater. Today Proc. 18, 3109–3115. doi:10.1016/j.matpr.2019.07.184
Rao, K. M., Kumar, D. V., Shekar, K. C., and Singaravel, B. (2021). Optimization of EDM process parameters using TOPSIS for machining AISI D2 steel material. Mater. Today Proc. 46, 701–706. doi:10.1016/j.matpr.2020.12.067
Rath, U. (2017). Parametric optimization of EDM on EN19 using grey-Taguchi analysis. Indian J. Sci. Technol. 10, 1–7. doi:10.17485/ijst/2017/v10i23/115565
Reddy, V. V., Krishna, P. V., Jawahar, M., and Shiva Kumar, B. (2018). Optimization of process parameters during EDM of SS304 using Taguchi-grey relational analysis. Mater. Today Proc. 5, 27065–27071. doi:10.1016/j.matpr.2018.09.011
Reddy, V. V., and Reddy, C. S. (2016). Multi response optimization of EDM of AA6082 material using Taguchi-DEAR method. Int. J. Sci. Eng. Res. 7, 215–219.
Routara, B. C., Das, D., Satpathy, M. P., Nanda, B. K., Sahoo, A. K., and Singh, S. S. (2020). Investigation on machining characteristics of T6-Al7075 during EDM with Cu tool in steady and rotary mode. Mater. Today Proc. 26, 2143–2150. doi:10.1016/j.matpr.2020.02.462
Roy, B., and Vincke, P. (1981). Multi-criteria analysis: survey and new directions. Eur. J. Operational Res. 8, 207–218. doi:10.1016/0377-2217(81)90168-5
Roy, T., and Dutta, R. K. (2019). Integrated fuzzy AHP and fuzzy TOPSIS methods for multi-objective optimization of electro discharge machining process. Soft Comput. 23, 5053–5063. doi:10.1007/s00500-018-3173-2
Sah, R. B., and Gangil, M. (2019). Optimization design of EDM machining parameter for carbon fibre nano composite. Res. J. Eng. Technol. Manag. 2, 1–6.
Sahoo, A. K., Pandey, P., and Mishra, D. R. (2021a). Multi-response optimization of EDM drilling parameters of the Nitinol SMA. J. Eng. Exact Sci. 7, 1–17. doi:10.18540/jcecvl7iss4pp13007-01-17e
Sahoo, S. K., Bara, A., Bhaskar, P., Sai, K. K., Rajiv, L. S., and Singh, S. L. (2021b). Optimization of process parameters based on RSM and GRA method for machining of Inconel-600 by electric discharge machining. Mater. Today Proc. 44, 2551–2555. doi:10.1016/j.matpr.2020.12.629
Sahu, A. K., and Mahapatra, S. S. (2020). Surface characteristics of EDMed titanium alloy and AISI 1040 steel workpieces using rapid tool electrode. Arabian J. Sci. Eng. 45, 699–718. doi:10.1007/s13369-019-04144-7
Sahu, A. K., and Mahapatra, S. S. (2021). Prediction and optimization of performance measures in electrical discharge machining using rapid prototyping tool electrodes. J. Intelligent Manuf. 32, 2125–2145. doi:10.1007/s10845-020-01624-8
Sahu, A. K., Mahapatra, S. S., Leite, M., and Goel, S. (2022). A hybrid grey-TOPSIS based quantum behaved particle swarm optimization for selection of electrode material to machine Ti6Al4V by electro-discharge machining. J. Braz. Soc. Mech. Sci. Eng. 44, 188. doi:10.1007/s40430-022-03494-y
Sameer, M. D., Birru, A. K., Srinu, G., and Naresh, C. (2021). Effect of process parameters during electric discharge machining of maraging steel and optimization using Taguchi-DEAR method. World J. Eng. 20, 409–417. doi:10.1108/WJE-07-2021-0434
Satpathy, A., Tripathy, S., Senapati, N. P., and Brahma, M. K. (2017). Optimization of EDM process parameters for AlSiC-20% SiC reinforced metal matrix composite with multi response using TOPSIS. Mater. Today Proc. 4, 3043–3052. doi:10.1016/j.matpr.2017.02.187
Seelan, K. J., and Rajesh, R. (2014). Optimization of EDM parameters using Taguchi method and grey relational analysis for aluminium titanium diboride (Al-TiB2). Int. J. Appl. Eng. Res. 9, 9358–9361.
Selvarajan, L., Manohar, M., and Dhinakaran, P. (2017). Modelling and experimental investigation of process parameters in EDM of Si3N4-TiN composites using GRA-RSM. J. Mech. Sci. Technol. 31, 111–122. doi:10.1007/s12206-016-1009-5
Selvarajan, L., Narayanan, C. S., and JeyaPaul, R. (2016). Optimization of EDM parameters on machining Si3N4-TiN composite for improving circularity, cylindricity and perpendicularity. Mater. Manuf. Process. 31, 405–412. doi:10.1080/10426914.2015.1058947
Senthil, P., Vinodh, S., and Singh, A. K. (2014). Parametric optimisation of EDM on Al-Cu/TiB2in-situ metal matrix composites using TOPSIS method. Int. J. Mach. Mach. Mater. 16, 80–94. doi:10.1504/ijmmm.2014.063922
Senthilkumar, T. S., and Muralikannan, R. (2019). Evaluation of recast layer and parametric optimization of EDM process on aluminium based HMMCs using grey relational analysis. Mater. Res. Express 6, 1065a6. doi:10.1088/2053-1591/ab3d73
Sharma, A., Kumar, V., Babbar, A., Dhawan, V., Kotecha, K., and Prakash, C. (2021). Experimental investigation and optimization of electric discharge machining process parameters using grey-fuzzy-based hybrid techniques. Materials 14, 5820. doi:10.3390/ma14195820
Sharma, A. K. (2021). EDM process parameters impact on MRR and TWR for titanium alloy [Ti6al4v] and their optimization using Taguchi and Topsis method - an experiential analysis. Mater. Sci. Eng. 1116, 012030. doi:10.1088/1757-899x/1116/1/012030
Sharma, R. K. (2020). Parametric optimization of process parameters for electric discharge machining of tungsten carbide (93% WC and 7% Co). Prod. Eng. Arch. 26, 154–161. doi:10.30657/pea.2020.26.28
Sharma, V., Misra, J. P., and Singhal, P. (2019). Optimization of process parameters on combustor material using Taguchi and MCDM method in electro-discharge machining (EDM). Mater. Today Proc. 18, 2672–2678. doi:10.1016/j.matpr.2019.07.128
Shastri, R. K., and Mohanty, C. P. (2021). Sustainable electrical discharge machining of Nimonic C263 superalloy. Arabian J. Sci. Eng. 46, 7273–7293. doi:10.1007/s13369-020-05211-0
Shastri, R. K., and Mohanty, C. P. (2022). A cost effective approach to explore the electrical discharge machined surface characteristics of Nimonic C263 superalloy. J. Mater. Eng. Perform. 31, 9748–9767. doi:10.1007/s11665-022-07048-0
Shukla, M., and Dhakad, S. K. (2018). Optimisation of electrical discharge machining of Al-LM-6/SiC/B4C composite: a grey relational approach. Mater. Today Proc. 5, 19147–19155. doi:10.1016/j.matpr.2018.06.269
Sidhu, S. S., Ablyaz, T. R., Bains, P. S., Muratov, K. R., Shlykov, E. S., and Shiryaev, V. V. (2021). Parametric optimization of electric discharge machining of metal matrix composites using analytic hierarchy process. Micromachines 12, 1289. doi:10.3390/mi12111289
Sidhu, S. S., Batish, A., and Kumar, S. (2014). ED machining of particulate reinforced MMC’s. Int. J. Industrial Manuf. Eng. 8 (3), 503–509.
Singh, A., Ghadai, R. K., Kalita, K., Chatterjee, P., and Pamučar, D. (2020). EDM process parameter optimization for efficient machining of Inconel-718. Facta Univ. Mech. Eng. 18, 473–490. doi:10.22190/fume200406035s
Singh, B., Kasdekar, D. K., and Parashar, V. (2015). Application of GRA for optimal machining parameter selection in EDM. Int. J. Hybrid Inf. Technol. 8, 371–382. doi:10.14257/ijhit.2015.8.10.34
Singh, M., and Singh, S. (2021). Multiple response optimization of ultrasonic assisted electric discharge machining of Nimonic 75: a Taguchi-grey relational analysis approach. Mater. Today Proc. 45, 4731–4736. doi:10.1016/j.matpr.2021.01.173
Singh, N. K., Pandey, P. M., Singh, K. K., and Sharma, M. K. (2016). Steps towards green manufacturing through EDM process: a review. Cogent Eng. 3, 1272662. doi:10.1080/23311916.2016.1272662
Sivaraj, M., Rajkumar, S., and Muthuraman, S. (2021). Parametric optimization of electrical discharge machining on AI-TiC composites using grey relational analysis. Mater. Today Proc. 37, 1254–1263. doi:10.1016/j.matpr.2020.06.437
Soepangkat, B. O. P., Wahyudi, A., and Pramujati, B. (2014). Multi-responses optimization of Edm sinking process of Aisi D2 tool steel using Taguchi grey-fuzzy method. IPTEK J. Technol. Sci. 25, 34–40. doi:10.12962/j20882033.v25i2.528
Somasundaram, M., and Kumar, J. P. (2022). Multi response optimization of EDM process parameters for biodegradable AZ31 magnesium alloy using TOPSIS and grey relational analysis. Sādhanā 47, 136–214. doi:10.1007/s12046-022-01908-0
Somu, C., Ranjith, R., Giridharan, P. K., and Ramu, M. (2021). A novel Cu-Gr composite electrode development for electric discharge machining of Inconel 718 alloy. Surf. Topogr. Metrology Prop. 9, 035025–35115. doi:10.1088/2051-672x/ac1f80
Srikanth, R., Singaravel, B., Vinod, P., Aravind, S., and Subodh, D. (2021). Optimization of process parameters in electric discharge machining process of Ti-6Al-4V alloy using hybrid Taguchi based MOORA method. Mater. Sci. Eng. 1057, 012064. doi:10.1088/1757-899x/1057/1/012064
Srinivasan, V. P., Palani, P. K., and Balamurugan, S. (2021b). Experimental investigation on EDM of Si3N4-TiN using grey relational analysis coupled with teaching-learning-based optimization algorithm. Ceram. Int. 47, 19153–19168. doi:10.1016/j.ceramint.2021.03.262
Srinivasan, V. P., Palani, P. K., Dhayananthan, S., Gopi, S., Balamurugan, S., and Venkatesh, S. M. (2021a). A multi criteria decision making (MCDM) based on TOPSIS and RSM for process improvement in electrical discharge machining of silicon nitride-titanium nitride ceramic composites. Mater. Today Proc. 45, 1319–1327. doi:10.1016/j.matpr.2020.05.436
Stević, Ž., Pamučar, D., Puška, A., and Chatterjee, P. (2020). Sustainable supplier selection in healthcare industries using a new MCDM method: measurement of alternatives and ranking according to COmpromise solution (MARCOS). Comput. Industrial Eng. 140, 106231. doi:10.1016/j.cie.2019.106231
Tamang, S. K., Natarajan, N., and Chandrasekaran, M. (2017). Optimization of EDM process in machining micro holes for improvement of hole quality. J. Braz. Soc. Mech. Sci. Eng. 39, 1277–1287. doi:10.1007/s40430-016-0630-7
Thangaraj, M., Annamalai, R., Moiduddin, K., Alkindi, M., Ramalingam, S., and Alghamdi, O. (2020). Enhancing the surface quality of micro titanium alloy specimen in WEDM process by adopting TGRA-based optimization. Materials 13 (6), 1440. doi:10.3390/ma13061440
Tharian, B. K., Jacob, E., Johnson, J., and Hari, V. (2019). Multi-objective parametric optimization in EDM using grey relational analysis. Mater. Today Proc. 16, 1013–1019. doi:10.1016/j.matpr.2019.05.190
Tiwari, M., Mausam, K., Sharma, K., and Singh, R. P. (2014). Investigate the optimal combination of process parameters for EDM by using a grey relational analysis. Procedia Mater. Sci. 5, 1736–1744. doi:10.1016/j.mspro.2014.07.363
Vaddi, V. R., Ch, S., Pogaku, V. K., and Bushaboina, S. K. (2018). Optimization of electrical discharge machining of titanium alloy (Ti-6Al-4V) using Taguchi-DEAR method. SAE Tech. Pap. 28 32. doi:10.4271/2018-28-0032
Vijayanand, M. S., and Ilangkumaran, M. (2017). Optimization of micro-EDM parameters using grey-based fuzzy logic coupled with the Taguchi method. Mater. Technol. 51, 989–995. doi:10.17222/mit.2017.048
Vikas, R. A. K., and Kumar, K. (2014). Effect and optimization of various machine process parameters on the surface roughness in EDM for an EN41 material using grey-Taguchi. Procedia Mater. Sci. 6, 383–390. doi:10.1016/j.mspro.2014.07.049
Vinoth Kumar, S., and Pradeep Kumar, M. (2017). Experimental investigation and optimization of machining process parameters in AISI D2 steel under conventional EDM and cryogenically cooled EDM process. Trans. Indian Inst. Metals 70, 2293–2301. doi:10.1007/s12666-017-1092-z
Xess, P. A., Biswas, S., and Masanta, M. (2014). Optimization of the EDM parameters on machining Ti-6Al-4V alloy with multiple quality characteristics. Appl. Mech. Mater. 619, 89–93. doi:10.4028/www.scientific.net/AMM.619.89
Youssef, H., and El-Hofy, H. A. G. (2020) Non-traditional and advanced machining technologies: machine tools and operations. USA: CRC Press.
Yuvaraj, T., and Suresh, P. (2019). Analysis of EDM process parameters on inconel 718 using the grey-taguchi and topsis method. J. Mech. Eng. 65, 557–564. doi:10.5545/sv-jme.2019.6194
Zeng, Y. P., Lin, C. L., Dai, H. M., Lin, Y. C., and Hung, J. C. (2021a). Multi-performance optimization in electrical discharge machining of Al2O3 ceramics using Taguchi base AHP weighted TOPSIS method. Processes 9, 1647. doi:10.3390/pr9091647
Zeng, Y. P., Lin, C. L., Hung, J. C., and Yang, C. F. (2021b). Optimization of electrical discharge machining processing for ceramics using grey-taguchi system. Mod. Phys. Lett. 35, 2141014. doi:10.1142/s0217984921410141
Glossary
Keywords: EDM process, optimization, MCDM, process parameter, response
Citation: Pendokhare D, Kalita K, Chakraborty S and Čep R (2024) A comprehensive review of parametric optimization of electrical discharge machining processes using multi-criteria decision-making techniques. Front. Mech. Eng 10:1404116. doi: 10.3389/fmech.2024.1404116
Received: 20 March 2024; Accepted: 23 April 2024;
Published: 09 May 2024.
Edited by:
Muhammad Jahan, Miami University, United StatesReviewed by:
Muthuramalingam T., SRM Institute of Science and Technology, IndiaTanveer Saleh, International Islamic University Malaysia, Malaysia
Copyright © 2024 Pendokhare, Kalita, Chakraborty and Čep. This is an open-access article distributed under the terms of the Creative Commons Attribution License (CC BY). The use, distribution or reproduction in other forums is permitted, provided the original author(s) and the copyright owner(s) are credited and that the original publication in this journal is cited, in accordance with accepted academic practice. No use, distribution or reproduction is permitted which does not comply with these terms.
*Correspondence: Kanak Kalita, a2FuYWtrYWxpdGEwMkBnbWFpbC5jb20=, ZHJrYW5ha2thbGl0YUB2ZWx0ZWNoLmVkdS5pbg==