- 1Research and Development Department, Alpine Advanced Materials, Dallas, TX, United States
- 2Department of Mechanical Engineering, Stanford University, Stanford, CA, United States
- 3School of Engineering and Sciences, Tecnologico de Monterrey, Monterrey, Mexico
- 4Department of Mechanical Engineering, University of North Texas, Denton, TX, United States
- 5Center for Information Technology Renato Archer (CTI), Campinas, Brazil
Recent developments in additive manufacturing (AM) have led to significant opportunities in the design and fabrication of implantable medical devices due to the advantages that AM offers compared to conventional manufacturing, such as high customizability, the ability to fabricate highly complex shapes, good dimensional accuracy, a clean build environment, and reduced material usage. The study of structural design optimization (SDO) involves techniques such as Topology Optimization (TO), Shape Optimization (SHO), and Size Optimization (SO) that determine specific parameters to achieve the best measurable performance in a defined design space under a given set of loads and constraints. Integration of SDO techniques with AM leads to utmost benefits in designing and fabricating optimized implantable medical devices with enhanced functional performance. Research and development of various lattice structures represents a powerful method for unleashing the full potential of additive manufacturing (AM) technologies in creating medical implants with improved surface roughness, biocompatibility, and mechanical properties. Furthermore, the integration of artificial intelligence (AI) and machine learning (ML) in structural optimization has expanded opportunities to improve device performance, adaptability, and durability. The review is meticulously divided into two main sections, reflecting the predictability of the implant’s internal structure: (a) unpredictable interior topology, which explores topology-based optimization techniques, and (b) predictable inner topology, concentrating on lattice structures. The analysis of the reviewed literature highlights a common focus on addressing issues such as stress shielding, osseointegration enhancement, customization to individual needs, programmable functionalities, and weight reduction in implant designs. It emphasizes significant advances in reducing stress shielding effects, promoting osseointegration, and facilitating personalized implant creation. The review provides a detailed classification of optimization methods, with each approach scrutinized for its unique contribution to overcoming specific challenges in medical implant design, thus leading to more advanced, effective, and patient-oriented implantable devices.
1 Introduction
Due to specific health conditions of many patients, medical devices need to be introduced to the human body to fulfill various functions related to biological structure, performance monitoring, or medication delivery. They can be placed temporarily or permanently in the body through surgical or nonsurgical procedures. The Food and Drug Administration (FDA) has classified medical devices as class I, class II, and class III based on the level of risk associated with the device to ensure safety and effectiveness for the patient (FDA, 2017). The risk posed to the user of medical devices categorized under Class I is the lowest, while Class III devices pose the highest risk to the user. A schematic classification system of implantable medical devices, based on functional interfaces, is shown in Figure 1A to complement traditional classifications. Each interface type—soft tissue, hard tissue, and sensorial transduction—presents distinct mechanical and biological considerations critical for the successful application of additive manufacturing processes and workflow validation. Figure 1B illustrates hard tissue interface devices example for structural support and replacement, introducing a parametric micro-architecture designed to exhibit a broad spectrum of mechanical properties, including both positive and negative Poisson’s ratios, to reduce load-induced interface fracture risk and post-surgery bone remodeling. This approach is tested on 3D titanium hip implants, showing a 64.0% improvement in bone remodeling and a 13.2% reduction in fracture risk compared to traditional implants (Garner et al., 2022). Figure 1C shows a case of soft tissue interface devices for functions like vessel patency restoration. The method presented develops a 2D stent unit cell through inverse homogenization and topology optimization, focusing on maximizing contact area and achieving specific mechanical properties. Some designs obtained volume fractions are in the range of 5%–7%, which are smaller compared to those of some commercially available stents (Carbonaro et al., 2023).
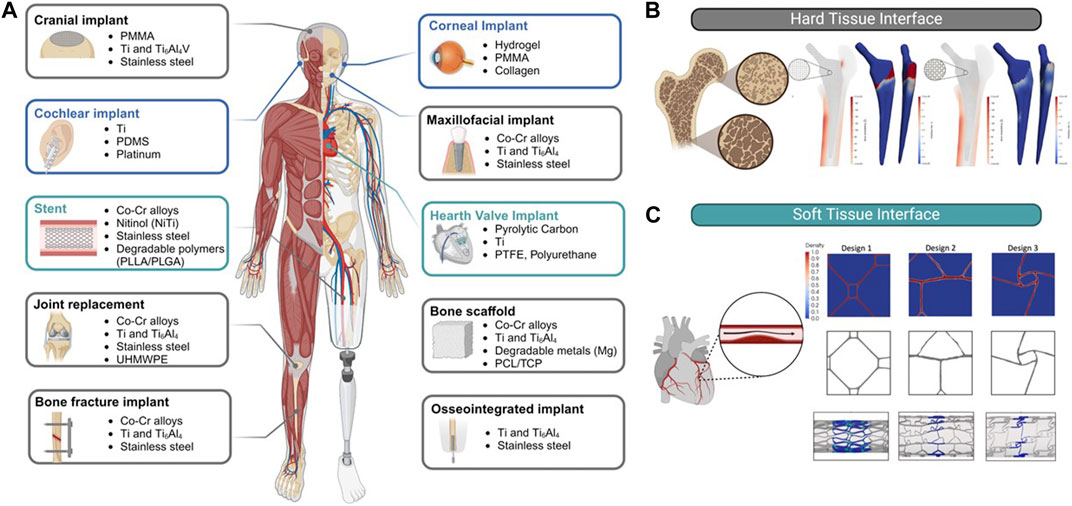
FIGURE 1. (A) Classification of implantable medical devices: soft tissue interface for regulating functions (cyan), hard tissue interface for structural support (black), and sensorial transduction environment interface for monitoring cues (blue). Examples of design optimization for medical implants using topology optimization and lattice structures include (B) an optimized hip implant using multi-objective design optimization and (C) self-expandable stents using inverse homogenization topology optimization. The illustrations were created with BioRender.com.
In general, medical implants, depending on their components, may consist of different biomaterials such as metals, composites, polymers, and ceramics. In addition to biocompatibility, the selection of a material for a medical implant is also based on physical properties, mechanical properties, and fabrication ability (Katti, 2004; Saini, 2015). Due to their superior mechanical properties, metals have been widely used as primary materials in numerous medical implants, especially in orthopedic implants. However, commercial metallic orthopedic implants exhibit higher stiffness, which is five to six times higher than bone (Katti, 2004), and according to Wolff’s law, bone can adapt to the mechanical stresses acting upon it, potentially leading to bone resorption or increased bone mass. If the loading on the bone increases, the bone will remodel itself over time, increasing its mass to become stronger, and vice versa (Ridzwan et al., 2007). Therefore, when a metallic implant is introduced to the bone, due to the metal-bone stiffness differences, a fraction of the loading will be carried by the implant and shielded from getting transferred to the bone. This is known as stress shielding phenomenon (Anguiano-Sanchez et al., 2016; Al-Tamimi et al., 2017), leading to various issues such as bone resorption, implant loosening, cracking, and discomfort during daily activities. Thus, research has been done on developing different strategies for developing new design concepts, cellular materials, and structural design optimization to find adequate approaches to reduce or avoid stress shielding at the bone-implant interface and to address other issues depending on the application. For example, Anguiano-Sanchez et al. (2016) proposed a new design concept to investigate reducing stress shielding by using a metallic stem, such as a titanium alloy, coated with polyether ether ketone (PEEK). The validation results showed that the use of PEEK coating contributed to a reduction of stress shielding by increasing the load transferred to the bone. However, additional considerations are needed for osseointegration of the PEEK coating in terms of porosity and surface roughness.
Therefore, optimization of implantable medical devices is necessary to accomplish robust designs that contribute to a better quality of life for patients. The most common optimization purposes of medical implants are related to the reduction or elimination of stress shielding problems, promotion of osseointegration, customization, and weight reduction. Based on a literature review, researchers have developed effective ways, such as incorporating cellular materials and structural design optimization techniques, to find adequate approaches that address the issues mentioned above. Structural design optimization (SDO) methods (Bendsøe and Sigmund, 2003), guided by gradient or non-gradient algorithms, provide the design variables that lead to optimal system performance under a given set of loadings, boundary conditions, and constraints. This goal is accomplished by utilizing various fields of study, such as calculus, mechanics, and programming. Topology optimization (TO), shape optimization (SHO), and size optimization (SO) are the three main categories of SDO methods, each referring to different aspects of the structure. The most widely used SDO method is topology optimization, since it not only modifies the inner topology of a structure but is also not restricted by the original boundary shapes (Yang and Zhao, 2015). Therefore, in general, it is mostly used prior to shape and size optimization. According to the literature, another way to optimize the structure of medical implants is by replacing solid volumes with lattice structures (Beyer and Figueroa, 2016; Jetté et al., 2018). This approach creates the potential to generate lighter medical components while offering appropriate levels of stiffness and energy absorption for various loadings. Furthermore, lattice structures have an impact on osseointegration due to their porosity, and they can be fabricated using AM technologies (Mendoza-Buenrostro et al., 2015; Thompson et al., 2016). Compared to SDO methods, lattice structures may offer robust solutions to problems with not fully known loading conditions and multiple objectives (Thompson et al., 2016). A special case is Topology Optimization (TO) and Load Sequence-Based Optimization (LBSO) are integral to advanced structural design. TO focuses on optimizing material layout within a design space, considering constraints and loads, to create efficient, lightweight structures ideal for manufacturing complexities. LBSO, in contrast, enhances design durability by considering the sequence and duration of load applications, crucial for dynamic environments.
In many cases, designs derived from topology or lattice-based optimization can be challenging to fabricate or even impossible using conventional manufacturing methods. Additive manufacturing, on the other hand, offers excellent opportunities for fabricating designs with highly complex shapes and lattice structures with tunable mechanical, biological, thermal, and optical properties (Thompson et al., 2016). Additive manufacturing of biomaterials (Saini, 2015; Bose et al., 2018) offers numerous advantages (Javaid and Haleem, 2017) compared to conventional manufacturing, and one of the most significant benefits is that AM offers a higher potential for achieving patient-specific designs with highly complex shapes. The most used AM processes for printing biomaterials (Bose et al., 2018) without any live cells are direct energy deposition (DED), powder bed fusion (PBF), material extrusion (ME), binder jetting (BJ), and vat polymerization (VP). Specifically, Metal Powder Bed Fusion (PBF), which primarily includes Selective Laser Melting (SLM) along with several similar technologies (such as EOS, SLM Solutions, Renishaw, Concept Laser - GE, etc.), and Electron Beam Melting (EBM) from Arcam - GE, are notable methods in this category.
Recently, significant interest has been devoted to the integration of structural design optimization and additive manufacturing (Zegard and Paulino, 2016). Despite the promising relationship between design optimization and AM in achieving improved medical implants, several constraints must be carefully considered throughout the process. Some of the constraints (Thompson et al., 2016) that designers must consider are related to computer-aided design and drafting, AM processes, the effect of AM on mechanical properties, and various challenges regarding quality control, environmental regulations, maintenance, etc. Several design tools have been developed for additive manufacturing-enabled theory and methodology (Yang and Zhao, 2015). Design for additive manufacturing (DfAM) (Thompson et al., 2016) has been used to take full advantage of AM processes in creating optimally complex and efficient designs featuring intricate geometries and lattice structures. The overall DfAM objective (Rosen, 2014) should be to maximize the performance of a component by integrating structural aspects, material content, and capabilities of additive manufacturing processes. AM-related structural design optimization can be categorized based on the optimization objective (Yang and Zhao, 2015), such as optimization of compliance, stiffness, strength, manufacturability. A comprehensive DfAM system is proposed by Rosen (2007) consisting of part and specification modeling, process planning, and manufacturing simulation. Tang et al. (2014), inspired by work of Rosen (2007), developed a multi-level design method where both topology optimization at the macro-level and lattice structure design at the meso-level are adapted sequentially. In addition, advances in the development of functional smart materials, flexible implantable electronics, and reconfigurable metamaterials have accelerated the adoption of machine learning, multiphysics and nonlinear simulation during various stages in the design of the next-generation of medical devices under real-world conditions (García-Ávila et al., 2021; 2022b). In fact, the FDA is increasingly interested in computer modeling and simulation as digital evidence and a valuable regulatory tool due to the potential for significant cost-savings in evaluating medical devices (Morrison et al., 2018).
This paper presents a comprehensive review of the current landscape in the optimization of additively manufactured medical implants. The narrative unfolds in two critical sections based on the interior structure predictability: Section 4 delves into the unpredictability of interior configurations through topological structural design optimization, while Section 5 explores the predictable inner topology with a focus on lattice-based optimization strategies. The review further investigates the impact of AI and ML in enhancing these optimization processes and incorporates the discussions on sustainable, safe, and smart materials from Section 3. Through an incisive analysis of contemporary research, the paper strives to elevate the reader’s grasp of the multifaceted challenges and burgeoning opportunities in crafting medical implants with AM technologies—highlighting a path towards more intelligent, personalized, and environmentally responsible medical solutions.
2 Advancements in additive manufacturing and optimization for medical devices
The use of 3D printing or additive manufacturing (AM) in the medical field has been rapidly evolving in the past two decades. From the initial introduction of anatomical models, surgical guides, and patient-matched implants, the technology has progressed to the development of 5S implants, which are customized implants that fit a patient’s unique anatomy (Figure 2A). Based on data from Scopus, it can be stated that the use of additive manufacturing in the development of medical implants is continuously increasing. Therefore, it is expected that there will be an increase in structural design optimization (SDO) to exploit the unique capabilities that AM offers. The research trends in SDO for additively manufactured medical implants are presented in Figure 2B. From 2006 to 2022, a total of 762 papers were published on the topology optimization of additively manufactured medical implants. In the year 2006, there was only one article published in this area, while in 2022 there are 234 articles published. The research on lattice structures in additively manufactured medical implants has also undergone significant changes, from three papers in 2010 to 706 papers in 2022. In addition, many researchers, referred to as the “Hybrid Optimization” type in Figure 2B, have combinated TO and lattice structures for the optimization and development of additively manufactured medical implants. A total of 454 papers are published using hybrid optimization, starting from one paper in 2011 to 148 papers in 2022. TO and lattice structures for AM medical implants are recent and ongoing research areas, and as seen from Figure 2B, there is a continuous trend over the last 10 years, with a significant growth particularly in the last 5 years. Finally, other manufacturing technologies including 4D printing and novel smart soft materials have drawn an increasing number of researchers to examine the development of next-generation implanted devices. From two articles in 2014 on topics related to 4D printing and implants to 456 articles published in 2022.
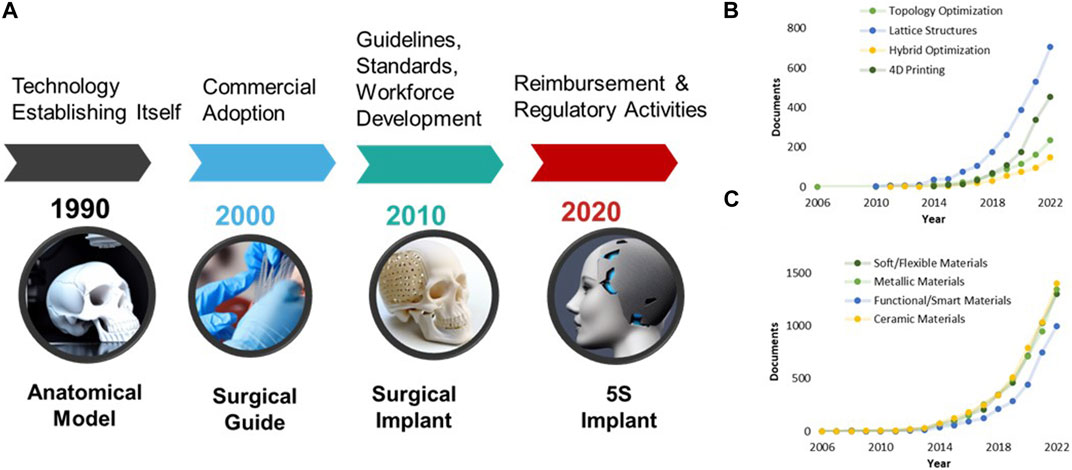
FIGURE 2. (A) 30 years of medical 3D printing, from the introduction of anatomical models, surgical guides, and patient-matched implants to the emergence of 5S implants (images generated by DALL-E 3). (B) Year wise publications based on Scopus for optimization of additive manufactured medical implants using (1) Topology optimization; (2) Lattice structures; (3) Hybrid optimization, which is a combination of TO and lattice structures: and (4) 4D printing. (C) Number of publications from 2006 to 2022 related to the use of various materials in medical 3D printing.
Research in materials for additive manufacturing and medical devices has been steadily increasing, with significant growth observed in soft/flexible, metallic, and functional/smart materials (Figure 2C). In 2022, soft/flexible materials had 1303 research papers, while metallic and ceramic materials had 1344 and 1400 publications respectively. Additionally, 1000 research papers were published on functional/smart materials in 2022. These findings indicate that researchers are continuously exploring new and innovative materials to meet the demands of the medical industry. In light of the tremendous evolution of additive manufacturing (AM), particularly over the last three decades, from replicating biological models to generating better implants, AM techniques have progressively been used in various applications within the biomedical field (Guo and Leu, 2013) such as scaffoldings for tissue engineering (Williams et al., 2005; Mendoza-Buenrostro et al., 2015); prosthetics (Subburaj et al., 2007; Liacouras et al., 2011); design and development of custom-made medical implants (Deshmukh et al., 2011; Van Noort, 2012); surgical planning, and medical education and training (Petzold et al., 1999; Sanghera et al., 2001; Faber et al., 2006; Sodian et al., 2007; Mori et al., 2009). Some of the reported findings include a reduction in operating time, increased safety of surgical procedures, faster patient recovery, enhanced resource utilization, improved efficiency in treatment planning and communication between patients and surgeons, and more accurate treatments, especially in maxillofacial/craniofacial cases. Bose et al. have presented a comprehensive review of AM techniques used for the fabrication of several biomedical devices (Bose et al., 2018). Based on the application, each AM technique (Groover, 2012) has its own advantages and disadvantages. For example, metal powder bed fusion (PBF) (Sun et al., 2016) processes are of special interest for fabrication of metallic medical devices (Bose et al., 2018) having great capabilities in fabrication of orthopedic implants (Tan et al., 2017) with porous structures due to offering high build resolution, appropriate dimensional accuracy and surface roughness, great customizability, less material usage, and clean build environment. Williams et al. demonstrated the potential of polycaprolactone (PCL) scaffolds fabricated via powder bed fusion (PBF) for replacement of skeletal tissues suitable for complex anatomic regions (Williams et al., 2005). It was shown that the bulk properties corresponded to the lower range of the human trabecular bone, and enhanced tissue in-growth was observed after 4 weeks of implementation. Similarly, many authors have utilized material extrusion (ME) processes for producing biodegradable scaffolds, a topic of research in tissue engineering for regenerative medicine (Hutmacher, 2000; Melchels et al., 2012).
Material extrusion (ME) and vat photopolymerization (VP) are among AM techniques that have been widely employed in applications that require polymeric biomaterials mainly for surgical planning, medical education and training in medicine and surgical guides for dental applications. Examples of additive manufacturing technologies for fabrication of prosthetics (Subburaj et al., 2007; Liacouras et al., 2011) and customized medical implants (Deshmukh et al., 2011; Van Noort, 2012) have shown excellent results in terms of aesthetics; high degree of accuracy for shape, size, and position of the prosthesis; and much faster and cheaper fabrication of the implants than conventional methods. Researchers have worked on developing methodologies and improving fabrication processes for medical devices. Mendoza-Buenrostro et al. (2015) developed a novel process for the fabrication of hybrid biodegradable scaffolds that can be used in various tissue engineering applications. The process combines the capabilities of material extrusion technology, electrospinning, and micro-milling. This manufacturing process has been demonstrated to fabricate various structural configurations of different scales and multi-materials. Medical devices must be sterilized before placement in the patient’s body to ensure safety. To ensure biocompatibility, the relationship between the fabrication process, material, and sterilization must be adequately established (Linares-Alvelais et al., 2018). Although most studies have focused on analyzing the impact of design parameters, fabrication processes, or materials for AM implants, fewer studies have examined the mechanical properties and biocompatibility of AM implants post-sterilization (Linares-Alvelais et al., 2018). Linares-Alvelais et al. have assessed the effects of sterilization on additively manufactured specimens. They employed the hydrostatic high-pressure sterilization method for samples printed using vat photopolymerization (VP) and material extrusion (ME) technologies. The proposed sterilization technique was found to enhance the mechanical properties of specimens compared to the standard autoclaving method. Therefore, they suggest employing the hydrostatic high-pressure technique to exploit the advantages that additive manufacturing offers in biomedical devices.
Additive manufacturing offers significant advantages by providing designs that are tailored to the specific requirements of patients, converting image data into 3D objects. Two conventional techniques used for capturing a patient’s data are computed tomography (CT) scans and magnetic resonance imaging (MRI). Additionally, though less common, methods such as Positron Emission Tomography (PET) and Single Photon Emission Computed Tomography (SPECT) are also viable options for obtaining patient-specific data. Salazar-Gamarra et al. demonstrated how monoscopic photogrammetry using mobile devices and free software can be utilized to obtain precise 3D models for creating facial prostheses, highlighting the feasibility of these technologies in the customization of medical treatments (Salazar-Gamarra et al., 2016). Transitioning from imaging to fabrication, in the field of additively manufactured medical implants, two primary approaches are generally considered in terms of implant temporality. The first is the biodegradable approach, as proposed by Ramirez-Cedillo et al. This method involves the development of a customized 3D barrier for guided bone regeneration, used in dental implant placements. Following this procedure, a personalized biodegradable 3D barrier for a trauma patient is designed and fabricated using Material Extrusion (ME) technology. This design is deemed optimal and is based on materials that degrade within an 8–10 months period, representing a scaffold approach that gradually dissolves in the patient’s body (Ramirez-Cedillo et al., 2017). The second approach, exemplified by the work of Melgoza et al., focuses on the design and fabrication of implants that are either permanent or temporary but do not degrade within the body, such as tracheal stents. Here, the strategy involves using an innovative open-source tool-path strategy, applied in the fabrication of a silicone tracheal stent using ME technology. Unlike the biodegradable 3D barriers, tracheal stents, while they may be temporary, are designed to remain stable and do not degrade in the body (L. Melgoza et al., 2014).
2.1 Process overview: AM medical implant development
The process for the development of AM medical implants consists of seven main steps which are: 3D modeling; topology optimization; model smoothing; preprocessing for AM; part fabrication using AM; post-processing of fabricated part; use of the implantable medical device. Depending on the case study, the 3D modeling of a medical device is based on design ideas, 2D images derived from CT scans, or 3D object for reverse engineering. Collected data are transformed into digital models’ various software packages (Thompson et al., 2016). The generated 3D model will be imported in an optimization software for performing TO which consists of many steps such as a specification of material properties, design variables, loadings, boundary conditions, objective function, constraints, sensitivity analysis, and other additional specifications needed for obtaining a converged TO solution. After accomplishing optimization, the optimized model is smoothened and converted into STL format. The preprocessing for AM is usually achieved with an AM-specific software such as Magics (Materialise, 2024). In the preprocessing phase, all possible data errors are corrected, support structures are added where they are needed, the model is sliced using a slicing algorithm, and the material filling pattern is defined. After the preprocessing step is completed, the medical device is fabricated using a relevant AM technology depending on the case study. In the post-processing phase of medical device manufacturing, it is critical to ensure that product requirements are thoroughly met. Sterilization is crucial, particularly for medical implants with lattice structures, which demand meticulous cleaning to eliminate all non-adhering powders, particles, or residues. The post-processing techniques used to enhance the quality of these medical devices vary based on application requirements and materials used. For orthopedic implants produced using Powder Bed Fusion (PBF), a range of methods can be employed, including shot peening, heat treatment, polishing techniques (Sing et al., 2016), acid attack, anodizing (de Damborenea et al., 2015), and other surface modification methods. The final step in this process involves the implantation of the medical device into the patient. This is performed through surgical or minimally invasive procedures, often employing appropriate or customized surgical instruments. Transitioning to regulatory aspects, it is pivotal to recognize the significant strides made in FDA-approved medical implants via AM. A notable example includes the FDA’s 510(k) clearance of Centinel Spine’s FLX Platform, consisting of 3D printed all-titanium fusion devices designed to stabilize vertebrae and enhance healing after spinal surgeries. Additionally, a significant milestone in this field is the FDA’s clearance of the first-ever 3D printed PEEK implant, the Inspire Porous PEEK Cervical Interbody Fusion System.
3 Structural, sustainable, small, safe, soft, and smart: the future of implantable medical device manufacturing
Classical structural design must quickly adapt to the trend of digital manufacturing transformation led by new micro-scale manufacturing technologies and the increased use of intelligent soft materials and reconfigurable metamaterials (architected materials) for implantable devices. Additionally, as conversations around sustainability become more significant in the healthcare sphere, companies that offer truly sustainable products will become more important. Medical devices themselves can be made more sustainable in various ways. For example, they can consume less energy, be made using sustainable low-emission production processes and sustainable materials, lower material uses or packaging, or have longer lifetimes. If sustainable materials are not safe or their performance is inadequate, they are not a viable alternative. Topology optimization helps reduce the overall dimensions or weight of the product to minimize material use, packaging, and distribution-related carbon emissions without neglecting the structural safety of the device. In general, medical devices are becoming smaller and smarter, which aids in addressing sustainability issues. The strategic involvement of AM in the healthcare industry will promote the mass customization of topologically optimized implantable devices and enhance the sustainability of process and product performance at the industrial, environmental, financial, resource, and end-user levels.
When considering examples of current commercial implants, the adopted structural shapes, topology design, porosity, stiffness, and other implant design aspects are subordinated to dynamic operating conditions while fulfilling the mechanical and biological interface functions (Figure 3A). Traditional implants are part of a broader family of surgical medical devices and other types of bioprostheses, including heart valves, endoscopic surgical guides, vascular stents, and membranes with tissue regenerative properties. With the development of novel biofabrication techniques, the structural design of implantable devices is increasingly focusing on soft and flexible devices capable of restoring active and passive functions of the human body (Figure 3B).
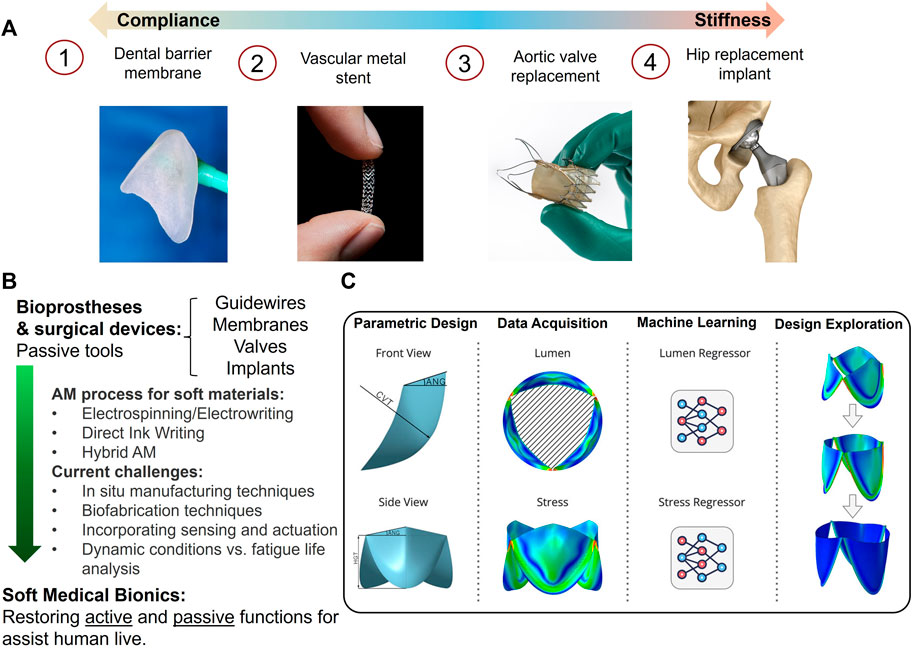
FIGURE 3. (A) Four examples of commercial implants in stiffness-compliance spectrum with different biological and mechanical interfaces; (B) Transition to the new generation of soft medical devices; (C) Overview of the proposed multistage generative approach of a prosthetic heart valve.
Researchers have been pioneering the integration of mechanical mimicry of the body’s tissues, such as muscle fibers and vascular networks, into the field of bioprinting and tissue replacement. This intricate task often uses reticular structures at various scales to emulate the body’s complex tissues, which is a key challenge in the field. The predominant techniques for devising and refining the geometry of medical devices traditionally rely on computer-aided designs coupled with the finite element method. However, this approach is often constrained by the limited range of geometries that can be explored and the extensive time required for design optimization. To overcome these limitations, there is a growing shift towards a generative design paradigm that leverages machine learning and optimization algorithms, as illustrated in Figure 3C (Danilov et al., 2023). This innovative method allows for a broader exploration of potential designs and a more efficient optimization process, paving the way for advancements in medical device fabrication. Another innovative perspective is the application of reticular structures in 4D printing. 4D printing has emerged as a collection of methods enabling the fabrication of 3D flexible structures with smart and sensitive materials that transform into new shapes upon exposure to stimuli such as temperature, light, humidity, or other environmental changes (Rahmatabadi et al., 2023a; 2023b; 2023c). The growing interest in 4D printing and metamaterials within medical devices has led to studies of responsive implants with shape-shifting properties. Moriwawa et al. fabricated a stretchable 2D donut-shaped kirigami device, as shown in Figure 4A, which can be transformed into a 3D cylindrical shape suitable for use in numerous spherically or columnar-shaped deformable biological tissues (Morikawa et al., 2019).
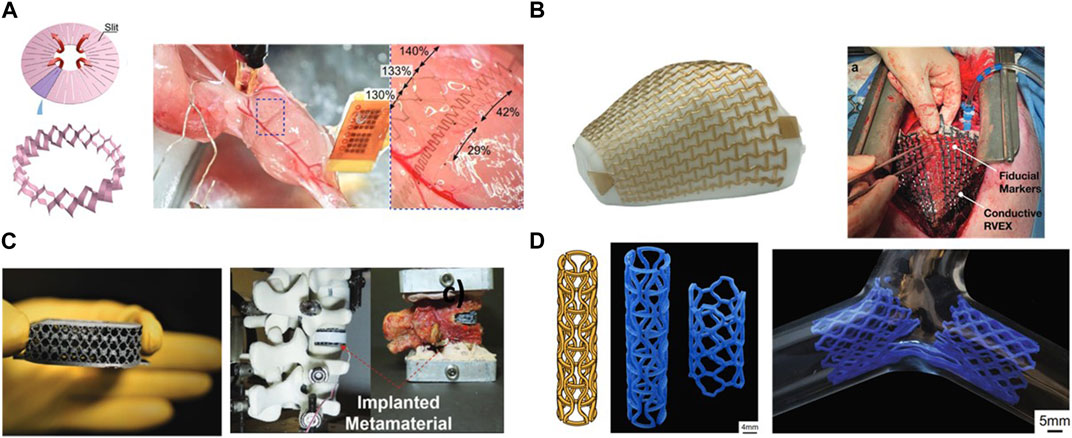
FIGURE 4. Four examples of 4D printing of reconfigurable lattice-based implants: (A) kirigami design, (B) auxetic design, (C) semicircular-shaped design, and (D) smooth re-entrant honeycomb design.
More flexibility, structural design, and compliance attributed to soft functional materials used in the fabrication of these devices make them ideal for delicate tasks in fragile in-body environments. For instance, an implantable elastic sleeve for right ventricular failure and monitoring of hemodynamic conditions was designed by Pirozzi et al. (Pirozzi et al., 2022), as seen in Figure 4B. However, the inherent nonlinearity of soft functional materials and their anisotropic actuation in compliant mechanisms present ongoing challenges in performance improvement. Topology optimization (TO), combined with four-dimensional (4D) printing, is a powerful digital tool used to achieve optimal internal architectures for implants. These implants can environmentally transform as required, offer “self-controllable functions,” extend product longevity, and facilitate greater structural complexity. The concept of “self-aware metamaterial implants” was introduced by Barri et al. (2022), creating a new generation of interbody fusion cage implants (see Figure 4C) with self-sensing, self-powering, and mechanical tunability functionalities. Similarly, the prototype of Teunis van Manen’s 4D printed bifurcation stents, as shown in Figure 4D, have shape-shifting properties but they are too large for clinical use, where a size 100 times smaller is required (van Manen et al., 2021). Whether under the rubric of space-time behavior or stimuli-responsiveness, these devices hold immense potential for decreasing the invasiveness of medical procedures and treatments. However, there are still structural issues to overcome, such as TO for multiscale structures versus high computational costs or dynamic loads versus fatigue life analysis (Mukherjee et al., 2021).
4 Structural design optimization (SDO) for medical implants
Structural design optimization (SDO) methods (Bendsøe and Sigmund, 2003) have been established to determine the design parameters that lead to the highest levels of operating performance for mechanical structures or systems. Numerous researchers have proposed optimized implant designs with the aim of mimicking bone properties by modifying the structure of existing implants, removing material in specific regions, or adding different features such as holes, ribs, grooves, etc. For instance, Figueroa et al. (2014) developed new optimized design concepts based on additive manufacturing for lumbar cages used in spinal surgeries. The proposed design concepts incorporated cavities to aid osseointegration. Furthermore, Figueroa-Cavazos et al. (2016) demonstrated the viability of additive manufactured new design concepts of intervertebral lumbar cages that were proposed, using material extrusion (ME) fabrication technology and biocompatible polycarbonate material. Robles-Linares et al. (2019) worked on AM-enabled parametric modeling to mimic the natural microstructure of cortical bone. They developed an innovative algorithm and successfully demonstrated its applicability by constructing additively manufactured samples with healthy and osteoporotic bone tissue microstructure.
Topology optimization (TO), shape optimization (SHO), and size optimization (SO) are three broad categories of structural design optimization that focus on different aspects of the structure (Müller and Klashorst, 2017). TO can realize any shape in the initially determined design space, while SHO and SO procedures are based on predefined configurations. Over the past decade, researchers have considered either one or a combination of these techniques in developing various optimization methodologies for medical implants. Usually, topology optimization is performed first, and the generated model can be fine-tuned by applying other SDO techniques. Problems of TO, SHO, and SO have their own characteristics and challenges. However, regardless of the problem optimization type, an SDO problem consists of three elements: objective function, design variables, and constraints. Optimization is an iterative process that involves either gradient-based mathematical techniques or non-gradient-based algorithms (Hare et al., 2013) such as genetic algorithm (GA) (Chen and Chiou, 2013), simulated annealing (SA), particle swarm optimization (PSO), etc. Few of the most used methods for solving SDO problems are level-set methods (LSM), solid isotropic microstructure with penalization (SIMP), evolutionary structural optimization (ESO) methods, soft-kill option (SKO), evolutionary algorithms (EA), etc. There are difficulties in solving an SDO problem associated with (Müller and Klashorst, 2017) many variables and the mixture of variable types (discrete, continuous, Boolean). Therefore, the aid of computational tools becomes crucial in solving an SDO problem. The assistance of finite element analysis is highly beneficial and essential in determining the satisfaction of constraints in the problem-solving process. There are commercial software packages available on the market such as Abaqus/Tosca, Hyperworks, etc., for performing structural design optimization.
4.1 Optimized design for additive manufacturing
Regardless of the exceptional evolution of AM, the progress on engineering design field has not fully exploited the vast opportunities offered by AM (Thompson et al., 2016). Furthermore, there are voids in managing the constraints that are associated with AM within the design process and sometimes designs that are generated from SDO are not friendly (Thompson et al., 2016). For example, a considerable amount of support structures required for the fabrication of an optimized design led to increase the fabrication and clean-up costs (Mirzendehdel and Suresh, 2016). Therefore, incorporating AM constraints into SDO techniques has drawn increasing attention (Wang and Kang, 2018) due to the promising benefits. Strategies that can be used to minimize support structures are classified into four groups (Mirzendehdel and Suresh, 2016). The first group is concerned with finding an optimal build position and orientation. The second group is related to achieving efficiency in support structures. The third group involves strategies that abide by design rules for AM. The fourth group contains strategies that go a step further, by optimizing design rules for AM.
Allaire et al. (2017) introduced a structural optimization with constraints such that overhang features were efficiently taken care in the SHO process. Li et al. (2015) presented a level set structural design optimization method accounting for extrusion constraints implementing a cross-section projection strategy. Liu and Ma (2018) worked on developing an innovative level set TO method with abilities to have control on the length scale of each material phase using multiple materials. Zhang and Zhou (2018), Johnson and Gaynor (2018), and Mezzadri et al. (2018) worked on developing TO methodologies for designing and generating self-supporting support structures for additive manufacturing. Adding manufacturing constraints into structural design optimization techniques is an active field of research to increase manufacturability, and optimal designs ready for AM can be produced with the aid of several commercial software packages present in the market.
More and more, researchers are working on developing tools for assisting design theories and methodologies that integrate with additive manufacturing technologies (Yang and Zhao, 2015). The assisting design tools for AM can be classified on four groups based on their focus (Yang and Zhao, 2015): (1) repair and manipulation; (2) solid modeling; (3) AM process-oriented design; and (4) structural design optimization, see Table 1. The first group of design tools is widely used in biomedical applications that such as the design of medical implants; reconstruction of medical images; customization of devices that require a perfect fit with the human body; and other applications that require reverse engineering. Even though the first three types of design tools enable review and processing of stereolithography (STL) files; meshing; 3D modeling; and generation of AM files, they have constraints in terms of memory usage, computational time, and internal structural configuration. The fourth group of AM design tools somehow conquered the limitations of other groups. It can be further divided into two subcategories based on the geometrical predictability of interior structures. For unpredictable interior structure, software packages that are based on finite element analysis and topology optimization technique are employed, such as Abaqus/Tosca (Dassult Systèmes), HyperWorks (Altair), Workbench (Ansys), etc. On the other hand, for predictable interior structures software packages that feature various cellular structures can be used such as nTopology, Netfabb, Within Enhance, etc.
4.2 Size optimization
Size optimization (SO) is a SDO method that is focused on the physical size of the members such as the thickness of a structure (Müller and Klashorst, 2017), without changing the overall shape and geometry of the structure. Therefore, SO can be beneficially employed for the optimization of struts in lattice structures. Few heuristic algorithms that have been effectively used for SO problems (Ahrari et al., 2015) are Genetic Algorithm, Simulated Annealing, Artificial Bee Colony, Particle Swarm Optimization, Harmony Search Algorithm, Reduced Space and Sequential Quadratic Programming, Big-Bang-Big Crunch Algorithm, etc. For example, Tang et al. (2015) to improve the performance of lattice structures, reconstructed the original bidirectional evolutionary SDO by developing a Bidirectional Evolutionary Structural Optimization (BESO) based SDO method to optimize thickness distribution of the lattice struts. The validation of the proposed method was done through a case study and nearly 75% weight reduction was achieved with the optimized lattice structure compared to the original design made from solid material. Sizing optimization can be very useful for optimization of lattice structures to create organic internal structures of implantable devices.
4.3 Shape optimization
Shape optimization (SHO) applies to the geometric layout (Müller and Klashorst, 2017), modifying the outer boundaries of structures in terms of CAD surfaces and curves (Cai et al., 2014). Therefore, in SHO problem, the domain itself becomes the design variable (Bendsøe and Sigmund, 2003). Under certain constraints related to geometry, stress, displacements, etc., SHO can accomplish mass minimization of a structure (Sun et al., 2018). Many researchers (Wall et al., 2008; Cai et al., 2014; Wang and Kang, 2018) to reduce local stress concentrations have developed new strategies by combining TO and SHO. Zhang et al. (Cai et al., 2014) established a technique by integrating B-spline finite cell method and level set function for stress constrained SHO and TO. Wang and Kang (2018) presented a level-set based method for TO and SHO of structures with coating layers. Wall et al. (2008) introduced a promising and highly efficient methodology for design shape optimization using non-uniform rational basis spline (NURBS).
Shape optimization is used widely in medical implants, particularly in hip implants. Katoozian and Davy (2000) applied shape optimization on a femoral component for defining the best geometrical design variables to evenly distribute the local stresses. They concluded that the most effective strategy in reducing all the equivalent stress criteria was to use a strain energy density criterion as the objective function. Virulsri et al. (2015) developed and proposed a multi-objective SHO procedure for designing an optimized femoral hip prosthesis that can also fit a Thai femur while considering safety. They showed through their study the significance of the prosthesis cross-section geometry on the development of the stresses around it. Nicolella et al. (2006) through a prosthesis shape optimization, reduced the tensile failure of prostheses-bone cement interface by 31.7% and reduced the probability of failure or all performance functions as well, without involving actual additive manufacturing in their study. Ruben et al. (2012) developed a multi-criteria shape optimization to obtain improved uncemented hip stems, considering the main causes of prosthesis failure such as stress shielding, normal contact stress, and tangential displacement. Based on their work they showed that optimization procedures are powerful tools to obtain new prosthesis design and help surgeons in decision-making process. Huiskes and Boeklagen (1989) introduced an iterative FE method for numerical shape optimization to achieve optimal prosthetics designs and minimize interface stresses. To maximize fatigue life of the cement in a hip prosthesis, Hedia et al. (1996) presented a method to maximize the fatigue life of cement in hip prostheses, not involving AM manufacturing. Their approach combined numerical shape optimization (SHO) with finite element (FE) modeling to optimize the longitudinal stem geometry (Hedia et al., 1996).
Besides hip prosthesis, SHO has been applied on other medical devices. Ribeiro et al. (2012) developed a SHO method to obtain an improved stent design with minimized stresses in the artery, contributing to the reduction of restenosis. In addition to that, Masoumi Khalil Abad et al. (2012) presented a design methodology based on shape optimization to improve the fatigue safety factor and increase the radial stiffness of Nitinol self-expandable stent-grafts. A study aimed to develop a fully automatic procedure enabling shape optimization and additive manufacturing of removable partial dentures (RPD), to maximize the uniformity of contact pressure distribution on the mucosa, thereby reducing associated clinical complications. A contact shape optimization algorithm was developed based on biometric data (Chen et al., 2015).
4.4 Topology optimization
Topology optimization (TO) is a SDO method that refers to the internal member configuration of a structure (Müller and Klashorst, 2017) without being limited by the nature of the original design. It is a method that identifies the locations where the material should be added or subtracted in a reference domain to achieve aimed functionalities for given loadings and boundary conditions that are applied to the structure (Thompson et al., 2016). This is the SDO method that has the most responsibility for the accomplishment of the final design. TO was introduced in 1988 in a seminal paper by Bendsøe and Kikuchi, and since then it has continuously evolved in many distinctive directions (Sigmund and Maute, 2013). According to Van Dijk et al. (2013) the most common TO approaches are density based and level-set based methods (Wang et al., 2003). In density-based methods such as homogenization methods, and solid isotropic microstructure with penalization (SIMP) methods (Li Y. Di et al., 2014), the geometry is described via a material distribution which is typically discretized using element-wise constant or nodal shape functions. Sigmund and Maute (Sigmund and Maute, 2013) presented a comparative and critical review of different TO approaches by discussing their strengths, weaknesses, similarities, and dissimilarities. They concluded that even though the differences between various TO approaches are small, the TO community seems to diverge creating separate individual research branches. Therefore, after identifying the nine main challenges of TO, they suggested the need for collective work in finding the “optimal optimization approach.”
SIMP is one of the most well-known and widely used FE-based TO method which is also found in the literature under different names “density method,” “power law,” etc. (Rozvany, 2008). In this method, the stiffness of the material is assumed to have a linear relationship with the material density of each element which is used as a design variable and normalized between zero (void) and one (solid). The converged algorithm of SIMP (Bendsøe and Sigmund, 2003) is controlled by a penalization parameter (P), which is increased from 1. The relation between the design function “density”
The density interpolates between material properties
Where,
Where F is a vector objective function over the feasible design space. Vector u contains design variables. The gj are referred to as constraints.
4.5 Case studies using structural design optimization
Structural design optimization techniques have been employed for the reduction of stress shielding and other purposes, see Table 2. Al-Tamimi et al. (2017) explored TO using SIMP method in order to minimize stress shielding and create lightweight orthopedic implants with reduced stiffness. The objective function of the TO problem is to decrease the strain energy. However, due to the high percentage of volume reduction, there was observed a decrease in equivalent stiffness resulting in the reduction of weight and stress shielding as well. Fraldi et al. (2010) worked on setting up guidelines for prosthesis design with maximized reliability. The strategy that was applied incorporated a maximum stiffness TO problem employed for a non-linear static FEA. He et al. (2018) proposed a methodology that was composed by topology and lattice optimization for designing a hip prosthesis with reduced stress shielding while meeting fatigue requirements. It achieved more than 50% stress shielding reduction while satisfying ISO ISO 7206-4: 2010 fatigue requirements and manufacturability via PBF. The proposed methodology can be further adapted for prosthesis design customization.
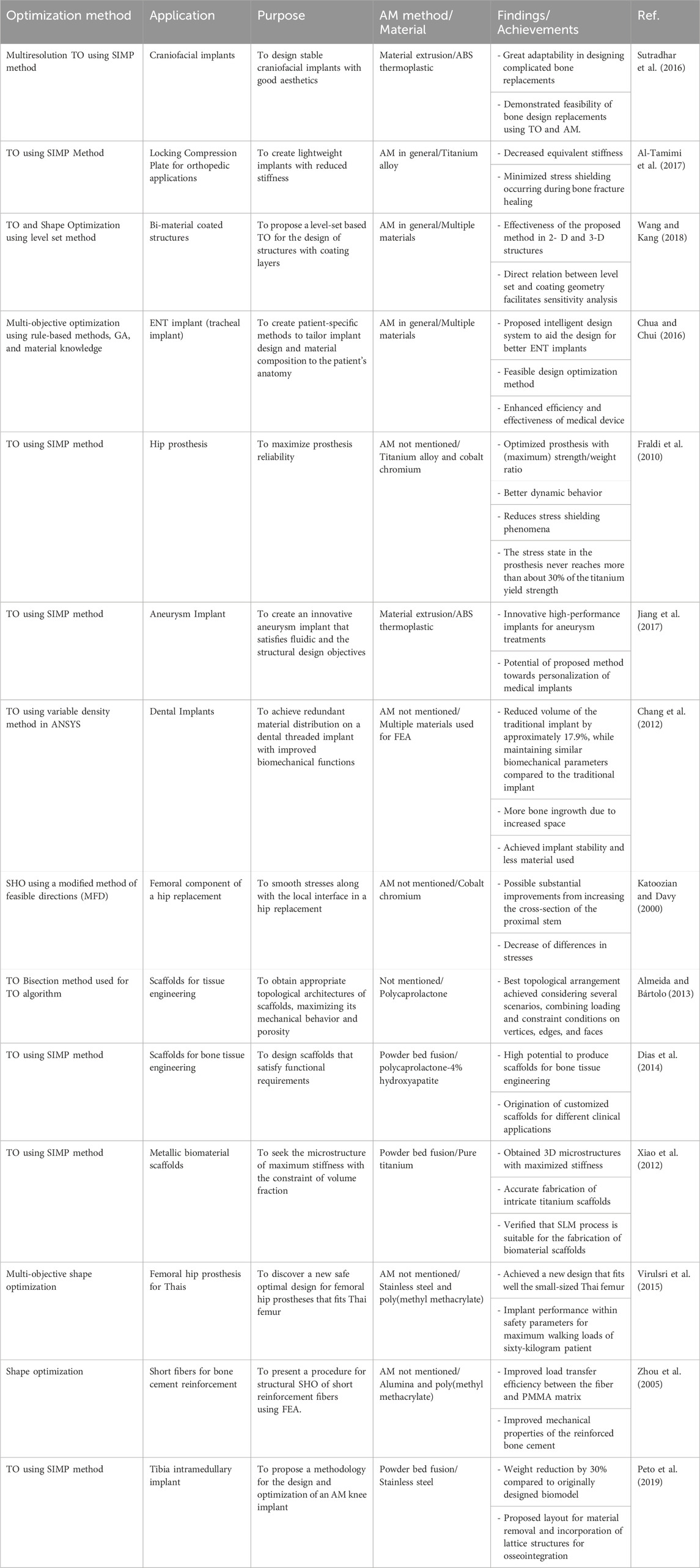
TABLE 2. Examples of case studies using structural design optimization for improvement of 3D printed medical implants.
Besides stress shielding reduction, stimulation of bone ingrowth is another important aspect that needs to be addressed when designing orthopedic implants. According to Chang et al. (2012) TO of dental implants leads to increasing of bone integration due to the additional concavities in the optimized structure. Therefore, TO influences sustaining implant stability, reducing implant displacement, and lowering implant material costs as well. Scaffolds used in bone tissue engineering must provide adequate structural support and diffusivity as well (Dias et al., 2014). Therefore, researchers have worked on proposing techniques using topology optimization to design scaffolds that meet specific requirements for mass transport and mechanical behavior (Xiao et al., 2012; Almeida and Bártolo, 2013; Dias et al., 2014) including some open-source tools for such design based on periodic equations (Dinis et al., 2014).
Personalization of medical implants is another significant accomplishment that comes from the integration of optimization techniques and AM. Chua and Chui (2016) investigated patient-based design methods to tailor ears, nose, and throat (ENT) implant design and material composition to the patient’s anatomy. The objective was achieved by developing an intelligent design system to perform multi-objective optimization for AM. Jiang et al. (2017) showed the potential of SIMP based TO toward the personalization of medical implants by creating an innovative design of an aneurysm implant while satisfying functional and structural requirements. In addition to that, Sutradhar et al. (2016) proposed a recent multiresolution TO method to achieve efficient and functional craniofacial implants with good aesthetics. Feasibility was successfully demonstrated via mechanical testing. Peto et al. (2019) proposed a methodology for the design and optimization of a tibia intramedullary implant for an 8-year-old osteosarcoma patient. Employing topology optimization achieved a weight reduction of the implant by 30% compared to the originally designed biomodel.
5 Lattice structures for AM medical implants
Cellular materials (Gibson et al., 2010) are used increasingly in biomedical applications due to their unique combination of properties, which depend directly on the shape and structure of cells in cellular solids (Gibson and Ashby, 1999). A key advantage offered by cellular materials is high strength accompanied by a relatively low mass. Furthermore, they can provide good energy absorption and good thermal and acoustic insulation properties (Rosen, 2007). Typically, cellular solids have a low relative density which allows large strains to happen before compression (Gibson and Ashby, 1999), and the unit cell geometry is modified to tailor the mechanical properties of the required component (Mahmoud and Elbestawi, 2017). Typical examples of natural cellular materials are foam, sponges, folded materials, honeycomb, and non-natural mathematical lattice structures. Cellular materials are employed in building scaffolds that are used in tissue engineering for purposes of substitution or regeneration of tissues in the human body (Gibson et al., 2010). They are typically classified based on their building unit cell order (stochastic or non-stochastic arrangement), based on level of anisotropy (cubic, orthotropic, etc.) (García-Ávila et al., 2022a), and their porosity type (open or closed) (Mahmoud and Elbestawi, 2017), with either 2-D or 3-D cell configuration.
Lattice structure is one type of cellular material (Mahmoud and Elbestawi, 2017), and its structure geometry can be modified to achieve the required levels of component performance (Beyer and Figueroa, 2016). Lattice structures have been widely implemented into the design of orthopedic implants (Mahmoud and Elbestawi, 2017; Jetté et al., 2018) mainly to achieve two approaches: to stimulate bone ingrowth and consequent osseointegration of bone-implant (Dinis et al., 2016) and also to reduce stress shielding in devices like plates for bone fracture consolidation. Lattice structures on meso-level can be divided into three main types based on the degree of an order (Tang et al., 2015). A disordered cellular structure contains various shapes and sizes of lattice unit cells that are arbitrary distributed within the structure. Periodic lattice structures are made of the same unit cell that is repeated throughout the structure. This category can be further divided into two subcategories based on the strut thickness variation. The two subcategories are homogeneous and spatially graded heterogeneous structures for uniform and non-uniform strut thickness respectively. In the third type, which is pseudo-periodic lattice structure, the size and shape of each lattice unit cell can be changed due to specific design requirements, while they all share the same general topology.
Optimization methods used for predictable internal topology, such as lattice structure, requires more human interference and knowledge to interpret objectives (Yang and Zhao, 2015). Since the optimized results depend much on the chosen patterns, researchers are focused on finding improved lattice patterns by optimizing design variables of lattice structures including strut thickness, cell topology, orientation, material, lattice skins, etc. According to research papers (Giannitelli et al., 2014; Wang et al., 2016; Mahmoud and Elbestawi, 2017) the lattice structures used in biomedical applications, such as tissue engineering and bone scaffolds, are classified by means of the unit cell design. Based on this classification, the main groups of unit cell designs are CAD-based design (Sun et al., 2005; Wettergreen et al., 2005; Kemmoku et al., 2010) image-based design (Feinberg et al., 2001; Hao et al., 2011) TO unit cells (Huang et al., 2011; Xiao et al., 2018) and implicit surface modeling (Rajagopalan and Robb, 2006). The CAD-based design group (Wang et al., 2016) consists of library of Platonic and Archimedean polyhedral solid (Li S. et al., 2014; Giannitelli et al., 2014) bio-inspired designs (Sun et al., 2005), and other designs generated using CAD software packages (Murr et al., 2010). Bucklen et al. (2008) proposed a library based on the assembly of regularly oriented unit primitives to aid in the development of scaffold design for orthopedic tissue engineering applications. Image-based design is generated by the intersection between 3D binary images (representing the shape of defect to be reproduced) and the stacking of a binary unit cell (3D patterning) (Giannitelli et al., 2014). Scaffold architectures via implicit surface modeling, are defined simply by using a single mathematical equation with the freedom to introduce different configuration features and pore shapes (Giannitelli et al., 2014). Topology optimization has been used by many researchers as an effective method in designing optimized unit cells that lead to several structural and functional improvements of an implant. A general computational flow chart of structural design optimization is shown in Figure 5.
5.1 Case studies using lattice-based structures
Lattice structures have been widely used by researchers for the improvement of medical implants, see Table 3. Jin et al. (2018) proposed a methodology for generating non-uniform lattice structures with improved stiffness while maintaining the same weight reduction ratio as the initial uniform lattice structure. Additionally, the methodology was ensured the manufacturability using powder bed fusion (PBF) technology. Huang et al. (2011) presented a new approach based on BESO techniques for designing periodic microstructures of cellular materials with maximum bulk or shear modulus. Compelling topological patterns resulted from the developed approach. Arabnejad Khanoki and Pasini (2012) designed an innovative implant with lattice microstructure and non-homogeneous material properties to address instability and bone resorption issues, by using a multiscale mechanics and design optimization technique. Hollister et al. (2002) demonstrated a successful image-based homogenization optimization approach for bone tissue engineering scaffolds which have conflicting design goals in terms of design requirements for mechanical support and enhancing tissue regeneration. Chen et al. (2011) explored the effect of scaffold design on the mechanobiological evolutions of tissue-scaffold systems by using the finite element-based homogenization technique and TO approach. Jetté et al. (2018) implemented lattice structures with diamond cubic unit cell configuration in a hip prosthesis to address stress shielding and bone tissue ingrowth aspects. It was demonstrated numerically and experimentally that the stiffness of the optimized porous stem model was 31% lower than the original fully dense model.
Several techniques have been employed for the fabrication of scaffolds. Based on the technique used the properties of the scaffolds vary since the porosity and the pore size changes from one technique to another. The development of additive manufacturing techniques facilitates the achievement of improved fabricated scaffolds with moduli varying from soft to hard tissues (Loh and Choong, 2013). Many researchers are combining different technologies to further improve the mechanical properties of scaffolds. Lara-Padilla et al. (2017) combined fused deposition modeling and electrospinning using a controlled cooling system to obtain improved scaffolds in terms of mechanical properties and delivery characteristics. The proposed bimodal scaffolds displayed better mechanical properties and appropriate morphology compared to polycaprolactone extruded strands (Lara-Padilla et al., 2017).
6 AI and machine learning in future research directions
The integration of artificial intelligence (AI) and machine learning (ML) techniques has significantly impacted the field of topology and lattice-based structural optimization for additively manufactured implantable medical devices (Nasiri and Khosravani, 2021). These techniques have enabled researchers to explore complex design spaces and develop highly optimized structures that can adapt to the unique requirements of individual patients, enhancing both the performance and durability of the devices. One notable application of AI and ML in this field is the use of generative algorithms, such as Genetic Algorithms (GA) and Generative Adversarial Networks (GAN), for the design of patient-specific lattice structures or automatic generation of implant geometry (Wu et al., 2022). These algorithms have been employed to optimize the mechanical properties of functionally graded porous Burch–Schneider cage (Xu et al., 2022), such as stiffness, strength, or specific anisotropic behaviors. Another significant development in the use of AI and ML techniques is the implementation of machine learning algorithms for predicting the performance and mechanical properties of additively manufactured lattice structures. These algorithms, such as Support Vector Machines (SVM) and Artificial Neural Networks (ANN), have been used to establish relationships between design parameters, manufacturing conditions, and the resulting mechanical properties, allowing for more effective AM optimization processes (Izonin et al., 2022).
Moreover, the combination of AI and ML techniques with multi-objective optimization algorithms has further expanded the potential for designing highly optimized implantable medical devices. These multi-objective optimization methods, such as the Non-dominated Sorting Genetic Algorithm II (NSGA-II) and the Technique for Order of Preference by Similarity (TOPSI), have been employed to simultaneously optimize multiple conflicting objectives during AM process, including build time and the surface roughness as the objective functions (Khodaygan and Golmohammadi, 2018). One study by Valiollah et al. (Panahizadeh et al., 2022) utilized the NSGA-II algorithm to Optimization of LB-PBF process parameters to achieve best relative density and surface roughness for Ti6Al4V samples. In conclusion, the integration of AI and ML techniques in topology and lattice-based structural optimization for additively manufactured implantable medical devices has opened new opportunities for improving the performance, adaptability, and durability of these devices, which can significantly enhance the quality of life for patients in need of such treatments.
6.1 Case study on data-driven design of architected metamaterial implants
The data-driven design process for creating metamaterials-based implants is a multifaceted approach that progresses through data acquisition, ML-guided design of unit cells, and multiscale design synthesis. A general case is shown in Figure 6A, underscoring the gyroid scaffolds enhanced mechanical properties when compared to cubic ones. This process is facilitated by a 3D Convolutional Neural Network, which predicts material properties, and a 3D convolutional autoencoder that enables efficient geometric reconstruction. The first stage of this process is generative architecture design (GAD), which utilizes the encoder-decoder structure of the neural network (autoencoder) to produce a set of architectures with previously unknown properties. By learning to represent high-dimensional data effectively in an unsupervised manner, the autoencoder simplifies the exploration of a vast design space into a more manageable one, a technique that has significantly advanced materials discovery. Following this is the multi-objective active learning loop (MALL), which assesses the generated dataset and iteratively selects high-performance architectures through the repeated application of the finite element method (FEM). The final stage involves 3D printing the ML-designed materials using laser powder bed fusion technology and experimentally validating their mechanical properties, thereby closing the loop from theoretical design to practical application. The practical application of this methodology was demonstrated with a large, irregular bone defect in a rabbit model in Figure 6B for bone grafting implants. The challenge of fitting a scaffold to such complex shapes is met by adapting an ML-designed cubic scaffold to the defect’s contours. FEM studies and experimental validation confirm that the ML-inspired designs offer significant improvements in load-bearing capacity, up to 20% over uniform designs, without sacrificing stiffness (Peng et al., 2023). This suggests the ML approach’s capability to deliver enhanced mechanical support in real-world clinical scenarios, proving the method’s effectiveness in creating large, intricate structures with greater load-bearing capacity for bone implant integration.
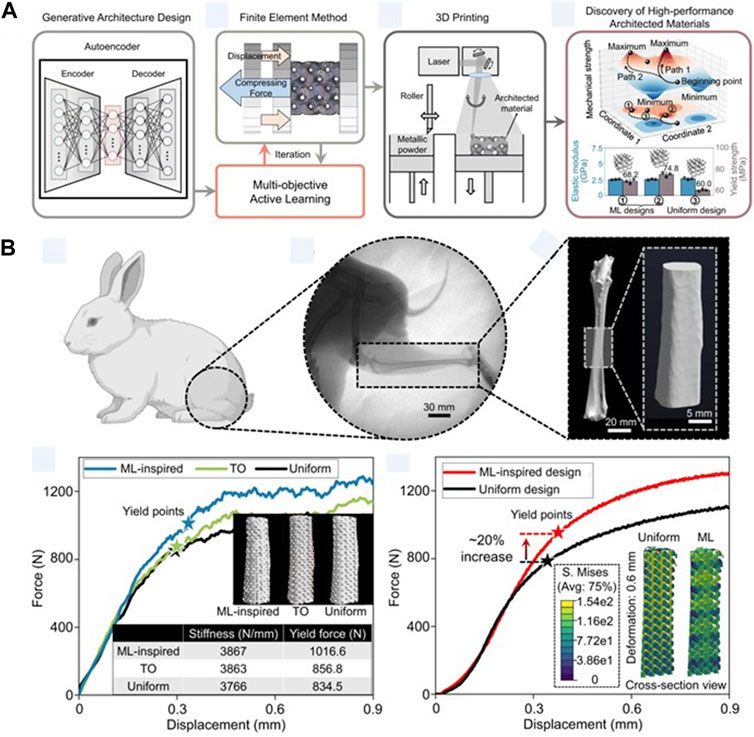
FIGURE 6. (A) Integrated design and fabrication workflow for high-performance architected materials, featuring a neural network proposing novel design candidates, an ML algorithm employing FEM for design refinement, a 3D printing technique for material fabrication, culminating in the discovery of superior architected materials, with statistical data indicated. (B) Bone implant design process for a significant tibial defect in a New Zealand rabbit, showcasing Micro-CT imaging for accurate modeling, FEM simulations to compare ML-inspired, topology-optimized, and uniform designs, and experimental validation highlighting the superior performance of the ML-inspired design with an inset of von Mises stress distribution under deformation.
7 Conclusion
This paper presented a literature review on topology and lattice-based structural design optimization for development of additively manufactured medical implants. In order to provide robust designs that contribute to better life quality for patients, it becomes necessary to perform structural optimization of medical implants for the reduction or elimination of stress shielding problems, promotion of osseointegration, customization, and weight reduction. The content of optimization techniques in this paper is organized in two main sections based on the interior structure predictability: (1) unpredictable interior topology (topology-based-optimization), and (2) predictable inner topology (lattice-based optimization). Prior to introducing structural optimization strategies that are used by different research works, a brief summary of findings and relevancy of additive manufacturing in medical areas is presented. Based on analysis of data generated from Scopus, it was found that the trend of the research in topology optimization and lattice structures have grown in order to fully exploit the capabilities that additive manufacturing offers for the fabrication of medical implants. TO and lattice structures for AM medical implants are recent and ongoing research areas, and there is a continuous increasing trend in the last 10 years, with a significant growth especially in the last 5 years. Combining topology optimization and lattice structures for improvement of additively manufactured medical implants is another strategy referred as “hybrid optimization” in this paper, which was implemented by many researchers starting from 2011.
Structural design optimization combined with additive manufacturing opens up a new option for the manufacturing of optimized and efficient medical devices. The topology-based optimization is an effective strategy in achieving medical implants with improved performance. The integration of topology optimization in the process chain for the development of AM medical implants is explained schematically in this paper. In summary, the main achievements presented from the reviewed research papers from applying TO and SHO for medical implants are related to minimization of stress shielding, weight reduction, customization, stimulation of bone ingrowth, material cost reduction, efficient bone replacements with good aesthetics and structural function, and increased feasibility of complicated bone replacement designs. In addition, based on papers that have used predictable inner topology strategies, it can be concluded that replacing solid volumes with lattice structures, also creates potential to generate lighter medical implants while offering suitable mechanical and supporting the flow of tissue fluids that carry nutrients. However, despite the benefits of these lightweight optimized medical implant designs, it is crucial to understand the constraints of AM and carefully consider them throughout the design process in order to fully exploit the advantages that AM offers.
Author contributions
MP: Conceptualization, Data curation, Formal Analysis, Investigation, Writing–original draft, Writing–review and editing. JG-Á: Conceptualization, Data curation, Formal Analysis, Investigation, Methodology, Software, Writing–original draft, Writing–review and editing. CR: Funding acquisition, Project administration, Resources, Supervision, Writing–review and editing. HS: Formal Analysis, Funding acquisition, Methodology, Project administration, Resources, Supervision, Writing–review and editing. JD: Conceptualization, Data curation, Investigation, Writing–review and editing. ER-C: Conceptualization, Formal Analysis, Investigation, Project administration, Supervision, Writing–original draft, Writing–review and editing.
Funding
The author(s) declare that financial support was received for the research, authorship, and/or publication of this article. The research was possible with the support of the College of Engineering of the University of North Texas, the Conacyt Mixed Scholarships Program and the Research Group in Advanced Manufacturing of Tecnologico de Monterrey.
Conflict of interest
Author MP was employed by Alpine Advanced Materials.
The remaining authors declare that the research was conducted in the absence of any commercial or financial relationships that could be construed as a potential conflict of interest.
Publisher’s note
All claims expressed in this article are solely those of the authors and do not necessarily represent those of their affiliated organizations, or those of the publisher, the editors and the reviewers. Any product that may be evaluated in this article, or claim that may be made by its manufacturer, is not guaranteed or endorsed by the publisher.
References
Ahrari, A., Atai, A. A., and Deb, K. (2015). Simultaneous topology, shape and size optimization of truss structures by fully stressed design based on evolution strategy. Eng. Optim. 47, 1063–1084. doi:10.1080/0305215X.2014.947972
Allaire, G., Dapogny, C., Estevez, R., Faure, A., and Michailidis, G. (2017). Structural optimization under overhang constraints imposed by additive manufacturing technologies. J. Comput. Phys. 351, 295–328. doi:10.1016/j.jcp.2017.09.041
Almeida, H. A., and Bártolo, P. J. (2013). Topological optimisation of scaffolds for tissue engineering. Procedia Eng. 59, 298–306. doi:10.1016/j.proeng.2013.05.125
Al-Tamimi, A. A., Peach, C., Fernandes, P. R., Cseke, A., and Bartolo, P. J. D. S. (2017). Topology optimization to reduce the stress shielding effect for orthopedic applications. Procedia CIRP 65, 202–206. doi:10.1016/j.procir.2017.04.032
Anguiano-Sanchez, J., Martinez-Romero, O., Siller, H. R., Diaz-Elizondo, J. A., Flores-Villalba, E., and Rodriguez, C. A. (2016). Influence of PEEK coating on hip implant stress shielding: a finite element analysis. Comput. Math. Methods Med. 2016, 1–10. doi:10.1155/2016/6183679
Arabnejad Khanoki, S., and Pasini, D. (2012). Multiscale design and multiobjective optimization of orthopedic hip implants with functionally graded cellular material. J. Biomech. Eng. 134, 031004. doi:10.1115/1.4006115
Barri, K., Zhang, Q., Swink, I., Aucie, Y., Holmberg, K., Sauber, R., et al. (2022). Patient-specific self-powered metamaterial implants for detecting bone healing progress. Adv. Funct. Mater 32, 2203533. doi:10.1002/ADFM.202203533
Bendsøe, M. P., and Sigmund, O. (2003). Topology optimization: theory, methods, and applications. Vasa 370. doi:10.1007/978-3-662-05086-6
Beyer, C., and Figueroa, D. (2016). Design and analysis of lattice structures for additive manufacturing. J. Manuf. Sci. Eng. 138, 121014. doi:10.1115/1.4033957
Bose, S., Ke, D., Sahasrabudhe, H., and Bandyopadhyay, A. (2018). Additive manufacturing of biomaterials. Prog. Mater Sci. 93, 45–111. doi:10.1016/J.PMATSCI.2017.08.003
Bucklen, B. S., Wettergreen, W. A., Yuksel, E., and Liebschner, M. A. K. (2008). Bone-derived CAD library for assembly of scaffolds in computer-aided tissue engineering. Virtual Phys. Prototyp. 3, 13–23. doi:10.1080/17452750801911352
Cai, S., Zhang, W., Zhu, J., and Gao, T. (2014). Stress constrained shape and topology optimization with fixed mesh: a B-spline finite cell method combined with level set function. Comput. Methods Appl. Mech. Eng. 278, 361–387. doi:10.1016/j.cma.2014.06.007
Carbonaro, D., Mezzadri, F., Ferro, N., De Nisco, G., Audenino, A. L., Gallo, D., et al. (2023). Design of innovative self-expandable femoral stents using inverse homogenization topology optimization. Comput. Methods Appl. Mech. Eng. 416, 116288. doi:10.1016/J.CMA.2023.116288
Chang, C. L., Chen, C. S., Huang, C. H., and Hsu, M. L. (2012). Finite element analysis of the dental implant using a topology optimization method. Med. Eng. Phys. 34, 999–1008. doi:10.1016/j.medengphy.2012.06.004
Chen, J., Ahmad, R., Suenaga, H., Li, W., Sasaki, K., Swain, M., et al. (2015). Shape optimization for additive manufacturing of removable partial dentures - a new paradigm for prosthetic CAD/CAM. PLoS One 10, e0132552. doi:10.1371/JOURNAL.PONE.0132552
Chen, T. Y., and Chiou, Y. H. (2013). Structural topology optimization using genetic algorithms. Lecture Notes in Engineering and Computer Science 3, 1933–1937.
Chen, Y., Zhou, S., and Li, Q. (2011). Microstructure design of biodegradable scaffold and its effect on tissue regeneration. Biomaterials 32, 5003–5014. doi:10.1016/j.biomaterials.2011.03.064
Chua, M. C. H., and Chui, C. K. (2016). Optimization of patient-specific design of medical implants for manufacturing. Procedia CIRP 40, 402–406. doi:10.1016/j.procir.2016.01.078
Danilov, V. V., Klyshnikov, K. Y., Onishenko, P. S., Proutski, A., Gankin, Y., Melgani, F., et al. (2023). Perfect prosthetic heart valve: generative design with machine learning, modeling, and optimization. Front. Bioeng. Biotechnol. 11, 1238130. doi:10.3389/fbioe.2023.1238130
de Damborenea, J. J., Larosa, M. A., Arenas, M. A., Hernández-López, J. M., Jardini, A. L., Ierardi, M. C. F., et al. (2015). Functionalization of Ti6Al4V scaffolds produced by direct metal laser for biomedical applications. Mater Des. 83, 6–13. doi:10.1016/J.MATDES.2015.05.078
Deshmukh, T. R., Kuthe, A. M., Chaware, S. M., Vaibhav, B., and Ingole, D. S. (2011). Rapid prototyping assisted fabrication of the customised temporomandibular joint implant: a case report. Rapid Prototyp. J. 17, 362–368. doi:10.1108/13552541111156487
Dias, M. R., Guedes, J. M., Flanagan, C. L., Hollister, S. J., and Fernandes, P. R. (2014). Optimization of scaffold design for bone tissue engineering: a computational and experimental study. Med. Eng. Phys. 36, 448–457. doi:10.1016/j.medengphy.2014.02.010
Dinis, J. C., Moraes, T. F., Amorim, P. H. J., Moreno, M. R., Nunes, A. A., and Silva, J. V. L. (2016). POMES: an open-source software tool to generate porous/roughness on surfaces. Procedia CIRP 49, 178–182. doi:10.1016/J.PROCIR.2015.07.085
Dinis, J. C., Morais, T. F., Amorim, P. H. J., Ruben, R. B., Almeida, H. A., Inforçati, P. N., et al. (2014). Open source software for the automatic design of scaffold structures for tissue engineering applications. Procedia Technol. 16, 1542–1547. doi:10.1016/J.PROTCY.2014.10.176
Faber, J., Berto, P. M., and Quaresma, M. (2006). Rapid prototyping as a tool for diagnosis and treatment planning for maxillary canine impaction. Am. J. Orthod. Dentofac. Orthop. 129, 583–589. doi:10.1016/j.ajodo.2005.12.015
FDA (2017). Learn if a medical device has been cleared by FDA for marketing. Available at: https://www.fda.gov/medical-devices/consumers-medical-devices/learn-if-medical-device-has-been-cleared-fda-marketing.
Feinberg, S. E., Hollister, S. J., Halloran, J. W., Chu, T. M. G., and Krebsbach, P. H. (2001). Image-based biomimetic approach to reconstruction of the temporomandibular joint. Cells Tissues Organs 169, 309–321. doi:10.1159/000047896
Figueroa, O., Rodríguez, C. A., Siller, H. R., Martínez-Romero, O., Flores-Villalba, E., Díaz-Elizondo, J. A., et al. (2014). “Lumbar cage design concepts based on additive manufacturing,” in 6th International Conference on Advanced Research in Virtual and Physical Prototyping, 603–607. VR@P 2013. doi:10.1201/b15961-110
Figueroa-Cavazos, J. O., Flores-Villalba, E., Diaz-Elizondo, J. A., Martínez-Romero, O., Rodríguez, C. A., and Siller, H. R. (2016). Design concepts of polycarbonate-based intervertebral lumbar cages: finite element analysis and compression testing. Appl. Bionics Biomech. 2016, 1–9. doi:10.1155/2016/7149182
Fraldi, M., Esposito, L., Perrella, G., Cutolo, A., and Cowin, S. C. (2010). Topological optimization in hip prosthesis design. Biomech. Model Mechanobiol. 9, 389–402. doi:10.1007/s10237-009-0183-0
García-Ávila, J., Cuan-Urquizo, E., Ramírez-Cedillo, E., Rodríguez, C. A., and Vargas-Martínez, A. (2022a). Novel porous structures with non-cubic symmetry: synthesis, elastic anisotropy, and fatigue life behavior. Math. Mech. Solids 28, 943–972. doi:10.1177/10812865221104236
García-Ávila, J., Rodríguez, C. A., Vargas-Martínez, A., Ramírez-Cedillo, E., and Israel Martínez-López, J. (2021). E-skin development and prototyping via soft tooling and composites with silicone rubber and carbon nanotubes. Materials 15, 256. doi:10.3390/MA15010256
García-Ávila, J., Torres Serrato, D. de J., Rodriguez, C. A., Martínez, A. V., Cedillo, E. R., and Martínez-López, J. I. (2022b). Predictive modeling of soft stretchable nanocomposites using recurrent neural networks. Polym. (Basel) 14, 5290. doi:10.3390/polym14235290
Garner, E., Wu, J., and Zadpoor, A. A. (2022). Multi-objective design optimization of 3D micro-architected implants. Comput. Methods Appl. Mech. Eng. 396, 115102. doi:10.1016/J.CMA.2022.115102
Giannitelli, S. M., Accoto, D., Trombetta, M., and Rainer, A. (2014). Current trends in the design of scaffolds for computer-aided tissue engineering. Acta Biomater. 10, 580–594. doi:10.1016/j.actbio.2013.10.024
Gibson, L. J., and Ashby, M. F. (1999). Cellular solids: structure and properties. Cambridge: Cambridge University Press.
Gibson, L. J., Ashby, M. F., and Harley, B. A. (2010). Cellular materials in nature and medicine. Cambridge: Cambridge University Press.
Groover, M. P. (2012). “Rapid prototyping and additive manufacturing,” in Fundamentals of modern manufacturing: materials, processes, and systems, 1128.
Guo, N., and Leu, M. C. (2013). Additive manufacturing: technology, applications and research needs. Front. Mech. Eng. 8, 215–243. doi:10.1007/s11465-013-0248-8
Hao, L., Raymont, D., Yan, C., Hussein, A., and Young, P. (2011). Design and additive manufacturing of cellular lattice structures. Innovative Dev. Virtual Phys. Prototyp. 2011, 249–254. doi:10.1201/b11341-40
Hare, W., Nutini, J., and Tesfamariam, S. (2013). A survey of non-gradient optimization methods in structural engineering. Adv. Eng. Softw. 59, 19–28. doi:10.1016/j.advengsoft.2013.03.001
He, Y., Durocher, D., Burkhalter, D., Gilbert, J. M., and Bone, W. (2018). “Solid-lattice hip prosthesis design: applying topology and lattice optimization to reduce stress shielding from hip implants,” in Proceedings of the 2018 Design of Medical Devices Conference, Minneapolis, Minnesota, USA, April 9–12, 2018, 1–5.
Hedia, H. S., Barton, D. C., Fisher, J., and Elmidany, T. T. (1996). A method for shape optimization of a hip prosthesis to maximize the fatigue life of the cement. Med. Eng. Phys. 18, 647–654. doi:10.1016/S1350-4533(96)00025-2
Hollister, S. J., Maddox, R. D., and Taboas, J. M. (2002). Optimal design and fabrication of scaffolds to mimic tissue properties and satisfy biological constraints. Biomaterials 23, 4095–4103. doi:10.1016/S0142-9612(02)00148-5
Huang, X., Radman, A., and Xie, Y. M. (2011). Topological design of microstructures of cellular materials for maximum bulk or shear modulus. Comput. Mater Sci. 50, 1861–1870. doi:10.1016/j.commatsci.2011.01.030
Huiskes, R., and Boeklagen, R. (1989). Mathematical shape optimization of hip prosthesis design. I Bwmrchunrcs 22, 793–804. doi:10.1016/0021-9290(89)90063-8
Hutmacher, D. W. (2000). Scaffolds in tissue engineering bone and cartilage. Biomaterials 21, 2529–2543. doi:10.1016/S0142-9612(00)00121-6
Izonin, I., Tkachenko, R., Gregus, M., Duriagina, Z., and Shakhovska, N. (2022). PNN-SVM approach of Ti-based powder’s properties evaluation for biomedical implants production. Comput. Mater. Continua 71, 5933–5947. doi:10.32604/CMC.2022.022582
Javaid, M., and Haleem, A. (2017). Additive manufacturing applications in medical cases: a literature based review. Alexandria J. Med. 54, 411–422. doi:10.1016/j.ajme.2017.09.003
Jetté, B., Brailovski, V., Dumas, M., Simoneau, C., and Terriault, P. (2018). Femoral stem incorporating a diamond cubic lattice structure: design, manufacture and testing. J. Mech. Behav. Biomed. Mater 77, 58–72. doi:10.1016/j.jmbbm.2017.08.034
Jiang, L., Chen, S., Sadasivan, C., and Jiao, X. (2017). “Structural topology optimization for generative design of personalized aneurysm implants: design, additive manufacturing, and experimental validation,” in 2017 IEEE Healthcare Innovations and Point of Care Technologies (HI-POCT), Bethesda, MD, USA, 06-08 November 2017, 9–13. doi:10.1109/HIC.2017.8227572
Jin, X., Li, G. X., and Zhang, M. (2018). Optimal design of three-dimensional non-uniform nylon lattice structures for selective laser sintering manufacturing. Adv. Mech. Eng. 10, 168781401879083. doi:10.1177/1687814018790833
Johnson, T. E., and Gaynor, A. T. (2018). Three-dimensional projection-based topology optimization for prescribed-angle self-supporting additively manufactured structures. Addit. Manuf. 24, 667–686. doi:10.1016/j.addma.2018.06.011
Katoozian, H., and Davy, D. T. (2000). Effects of loading conditions and objective function on three-dimensional shape optimization of femoral components of hip endoprostheses. Med. Eng. Phys. 22, 243–251. doi:10.1016/S1350-4533(00)00030-8
Katti, K. S. (2004). Biomaterials in total joint replacement. Colloids Surf. B Biointerfaces 39, 133–142. doi:10.1016/j.colsurfb.2003.12.002
Kemmoku, D. T., Noritomi, P. Y., Roland, F. G., and Da Silva, J. V. L. (2010). Use of BioCAD in the development of a growth compliant prosthetic device for cranioplasty of growing patients. Innovative Dev. Des. Manuf. 2010, 127–130. doi:10.1201/9780203859476.ch17
Khodaygan, S., and Golmohammadi, A. H. (2018). Multi-criteria optimization of the part build orientation (PBO) through a combined meta-modeling/NSGAII/TOPSIS method for additive manufacturing processes. Int. J. Interact. Des. Manuf. 12, 1071–1085. doi:10.1007/s12008-017-0443-7
Lara-Padilla, H., Mendoza-Buenrostro, C., Cardenas, D., Rodriguez-Garcia, A., and Rodriguez, C. A. (2017). Influence of controlled cooling in bimodal scaffold fabrication using polymers with different melting temperatures. Materials 10, 640–716. doi:10.3390/ma10060640
Li, H., Li, P., Gao, L., Zhang, L., and Wu, T. (2015). A level set method for topological shape optimization of 3D structures with extrusion constraints. Comput. Methods Appl. Mech. Eng. 283, 615–635. doi:10.1016/j.cma.2014.10.006
Li, S. J., Xu, Q. S., Wang, Z., Hou, W. T., Hao, Y. L., Yang, R., et al. (2014b). Influence of cell shape on mechanical properties of Ti-6Al-4V meshes fabricated by electron beam melting method. Acta Biomater. 10, 4537–4547. doi:10.1016/j.actbio.2014.06.010
Li, Y.D., Kuang, B., and Liu, J. (2014a). SIMP-based evolutionary structural optimization method for topology optimization. Appl. Mech. Mater. 651–653, 2237–2240. doi:10.4028/www.scientific.net/AMM.651-653.2237
Liacouras, P., Garnes, J., Roman, N., Petrich, A., and Grant, G. T. (2011). Designing and manufacturing an auricular prosthesis using computed tomography, 3-dimensional photographic imaging, and additive manufacturing: a clinical report. J. Prosthet. Dent. 105, 78–82. doi:10.1016/S0022-3913(11)60002-4
Linares-Alvelais, J., Figueroa-Cavazos, J., Chuck-Hernandez, C., Siller, H., Rodríguez, C., and Martínez-López, J. (2018). Hydrostatic high-pressure post-processing of specimens fabricated by DLP, SLA, and FDM: an alternative for the sterilization of polymer-based biomedical devices. Materials 11, 2540. doi:10.3390/ma11122540
Liu, J., and Ma, Y. (2018). A new multi-material level set topology optimization method with the length scale control capability. Comput. Methods Appl. Mech. Eng. 329, 444–463. doi:10.1016/j.cma.2017.10.011
Loh, Q. L., and Choong, C. (2013). Three-dimensional scaffolds for tissue engineering applications: role of porosity and pore size. Tissue Eng. Part B Rev. 19, 485–502. doi:10.1089/ten.teb.2012.0437
Mahmoud, D., and Elbestawi, M. (2017). Lattice structures and functionally graded materials applications in additive manufacturing of orthopedic implants: a review. J. Manuf. Mater. Process. 1, 13. doi:10.3390/jmmp1020013
Masoumi Khalil Abad, E., Pasini, D., and Cecere, R. (2012). Shape optimization of stress concentration-free lattice for self-expandable Nitinol stent-grafts. J. Biomech. 45, 1028–1035. doi:10.1016/j.jbiomech.2012.01.002
Materialise (2024). Magics. Available at: https://www.materialise.com/en/software/magics (Accessed November 16, 2018).
Melchels, F. P. W., Domingos, M. A. N., Klein, T. J., Malda, J., Bartolo, P. J., and Hutmacher, D. W. (2012). Additive manufacturing of tissues and organs. Prog. Polym. Sci. 37, 1079–1104. doi:10.1016/J.PROGPOLYMSCI.2011.11.007
Melgoza, L., Vallicrosa, G. E., Serenó, L., Ciurana, J., and Rodríguez, C. A. (2014). Rapid tooling using 3D printing system for manufacturing of customized tracheal stent. Rapid Prototyp. J. 20, 2–12. doi:10.1108/RPJ-01-2012-0003
Mendoza-Buenrostro, C., Lara, H., and Rodriguez, C. (2015). Hybrid fabrication of a 3D printed geometry embedded with PCL nanofibers for tissue engineering applications. Procedia Eng. 110, 128–134. doi:10.1016/j.proeng.2015.07.020
Mezzadri, F., Bouriakov, V., and Qian, X. (2018). Topology optimization of self-supporting support structures for additive manufacturing. Addit. Manuf. 21, 666–682. doi:10.1016/j.addma.2018.04.016
Mirzendehdel, A. M., and Suresh, K. (2016). Support structure constrained topology optimization for additive manufacturing. Cad. Comput. Aided Des. 81, 1–13. doi:10.1016/j.cad.2016.08.006
Mori, K., Yamamoto, T., Oyama, K., and Nakao, Y. (2009). Modification of three-dimensional prototype temporal bone model for training in skull-base surgery. Neurosurg. Rev. 32, 233–239. doi:10.1007/s10143-008-0177-x
Morikawa, Y., Yamagiwa, S., Sawahata, H., Numano, R., Koida, K., and Kawano, T. (2019). Donut-shaped stretchable kirigami: enabling electronics to integrate with the deformable muscle. Adv. Healthc. Mater 8, 1900939. doi:10.1002/ADHM.201900939
Morrison, T. M., Pathmanathan, P., Adwan, M., and Margerrison, E. (2018). Advancing regulatory science with computational modeling for medical devices at the FDA’s office of science and engineering laboratories. Front. Med. (Lausanne) 5, 241. doi:10.3389/FMED.2018.00241
Mukherjee, S., Lu, D., Raghavan, B., Breitkopf, P., Dutta, S., Xiao, M., et al. (2021). Accelerating large-scale topology optimization: state-of-the-art and challenges. Archives Comput. Methods Eng. 28, 4549–4571. doi:10.1007/S11831-021-09544-3
Müller, T. E., and Klashorst, E. van der (2017). A quantitative comparison between size, shape, topology and simultaneous optimization for truss structures. Lat. Am. J. Solids Struct. 14, 2221–2242. doi:10.1590/1679-78253900
Murr, L. E., Gaytan, S. M., Medina, F., Lopez, H., Martinez, E., MacHado, B. I., et al. (2010). Next-generation biomedical implants using additive manufacturing of complex cellular and functional mesh arrays. Philosophical Trans. R. Soc. A Math. Phys. Eng. Sci. 368, 1999–2032. doi:10.1098/rsta.2010.0010
Nasiri, S., and Khosravani, M. R. (2021). Machine learning in predicting mechanical behavior of additively manufactured parts. J. Mater. Res. Technol. 14, 1137–1153. doi:10.1016/J.JMRT.2021.07.004
Nicolella, D. P., Thacker, B. H., Katoozian, H., and Davy, D. T. (2006). The effect of three-dimensional shape optimization on the probabilistic response of a cemented femoral hip prosthesis. J. Biomech. 39, 1265–1278. doi:10.1016/j.jbiomech.2005.03.010
Panahizadeh, V., Ghasemi, A. H., Dadgar Asl, Y., and Davoudi, M. (2022). Optimization of LB-PBF process parameters to achieve best relative density and surface roughness for Ti6Al4V samples: using NSGA-II algorithm. Rapid Prototyp. J. 28, 1821–1833. doi:10.1108/rpj-09-2021-0238
Peng, B., Wei, Y., Qin, Y., Dai, J., Li, Y., Liu, A., et al. (2023). Machine learning-enabled constrained multi-objective design of architected materials. Nat. Commun. 14, 6630–6712. doi:10.1038/s41467-023-42415-y
Peto, M., Ramírez-cedillo, E., Hernández, A., and Siller, H. R. (2019). Structural design optimization of knee replacement implants for Additive Manufacturing. Procedia Manuf. 34, 574–583. doi:10.1016/j.promfg.2019.06.222
Petzold, R., Zeilhofer, H. F., and Kalender, W. a. (1999). Rapid prototyping technology in medicine—basics and applications. Comput. Med. Imaging Graph 23, 277–284. doi:10.1016/S0895-6111(99)00025-7
Pirozzi, I., Kight, A., Shad, R., Han, A. K., Dual, S. A., Fong, R., et al. (2022). RVEX: right ventricular external device for biomimetic support and monitoring of the right heart. Adv. Mater Technol. 7, 2101472. doi:10.1002/ADMT.202101472
Rahmatabadi, D., Aberoumand, M., Soltanmohammadi, K., Soleyman, E., Ghasemi, I., Baniassadi, M., et al. (2023a). Toughening PVC with biocompatible PCL softeners for supreme mechanical properties, morphology, shape memory effects, and FFF printability. Macromol. Mater Eng. 308, 2300114. doi:10.1002/MAME.202300114
Rahmatabadi, D., Ghasemi, I., Baniassadi, M., Abrinia, K., and Baghani, M. (2023b). 4D printing of PLA-TPU blends: effect of PLA concentration, loading mode, and programming temperature on the shape memory effect. J. Mater Sci. 58, 7227–7243. doi:10.1007/s10853-023-08460-0
Rahmatabadi, D., Soltanmohammadi, K., Pahlavani, M., Aberoumand, M., Soleyman, E., Ghasemi, I., et al. (2023c). Shape memory performance assessment of FDM 3D printed PLA-TPU composites by Box-Behnken response surface methodology. Int. J. Adv. Manuf. Technol. 127, 935–950. doi:10.1007/s00170-023-11571-2
Rajagopalan, S., and Robb, R. A. (2006). Schwarz meets Schwann: design and fabrication of biomorphic and durataxic tissue engineering scaffolds. Med. Image Anal. 10, 693–712. doi:10.1016/j.media.2006.06.001
Ramirez-Cedillo, E., Lara-Padilla, H., Zamudio-Peña, L. F., Rodriguez-Garcia, A., Ruiz-Huerta, L., Ruiz, A. C., et al. (2017). Process chain for the fabrication of a custom 3D barrier for guided bone regeneration. Procedia CIRP 65, 151–156. doi:10.1016/j.procir.2017.04.015
Ribeiro, N., Folgado, J., and Rodrigues, H. (2012). Shape optimization of a coronary stent. J. Biomech. 45, S642. doi:10.1016/S0021-9290(12)70643-7
Ridzwan, M. I. Z., Shuib, S., Hassan, A. Y., Shokri, A. A., and Mohammad Ibrahim, M. N. (2007). Problem of stress shielding and improvement to the hip implant designs: a review. J. Med. Sci. 7, 460–467. doi:10.3923/jms.2007.460.467
Robles-Linares, J., Ramírez-Cedillo, E., Siller, H., Rodríguez, C., and Martínez-López, J. (2019). Parametric modeling of biomimetic cortical bone microstructure for additive manufacturing. Materials 12, 913. doi:10.3390/ma12060913
Rosen, D. (2014). Design for additive manufacturing: past, present, and future directions. Mech. Des. 136. doi:10.1115/1.4028073
Rosen, D. W. (2007). Computer-aided design for additive manufacturing of cellular structures. Comput. Aided Des. Appl. 4, 585–594. doi:10.1080/16864360.2007.10738493
Rozvany, G. I. N. (2008). A critical review of established methods of structural topology optimization. Struct. Multidiscip. Optim. 37 (3), 217–237. doi:10.1007/S00158-007-0217-0
Ruben, R. B., Fernandes, P. R., and Folgado, J. (2012). On the optimal shape of hip implants. J. Biomech. 45, 239–246. doi:10.1016/j.jbiomech.2011.10.038
Saini, M. (2015). Implant biomaterials: a comprehensive review. World J. Clin. Cases 3, 52. doi:10.12998/wjcc.v3.i1.52
Salazar-Gamarra, R., Seelaus, R., Da Silva, J. V. L., Da Silva, A. M., and Dib, L. L. (2016). Monoscopic photogrammetry to obtain 3D models by a mobile device: a method for making facial prostheses. J. Otolaryngology 45, 33–13. doi:10.1186/s40463-016-0145-3
Sanghera, B., Naique, S., Papaharilaou, Y., and Amis, A. (2001). Preliminary study of rapid prototype medical models. Rapid Prototyp. J. 7, 275–284. doi:10.1108/13552540110410486
Sigmund, O., and Maute, K. (2013). Topology optimization approaches: a comparative review. Struct. Multidiscip. Optim. 48, 1031–1055. doi:10.1007/s00158-013-0978-6
Sing, S. L., An, J., Yeong, W. Y., and Wiria, F. E. (2016). Laser and electron-beam powder-bed additive manufacturing of metallic implants: a review on processes, materials and designs. J. Orthop. Res. 34, 369–385. doi:10.1002/jor.23075
Sodian, R., Weber, S., Markert, M., Rassoulian, D., Kaczmarek, I., Lueth, T. C., et al. (2007). Stereolithographic models for surgical planning in congenital heart surgery. Ann. Thorac. Surg. 83, 1854–1857. doi:10.1016/j.athoracsur.2006.12.004
Subburaj, K., Nair, C., Rajesh, S., Meshram, S. M., and Ravi, B. (2007). Rapid development of auricular prosthesis using CAD and rapid prototyping technologies. Int. J. Oral Maxillofac. Surg. 36, 938–943. doi:10.1016/j.ijom.2007.07.013
Sun, S., Brandt, M., and Easton, M. (2016). Powder bed fusion processes: an overview. Elsevier Ltd. doi:10.1016/B978-0-08-100433-3.00002-6
Sun, S. H., Yu, T. T., Nguyen, T. T., Atroshchenko, E., and Bui, T. Q. (2018). Structural shape optimization by IGABEM and particle swarm optimization algorithm. Eng. Anal. Bound Elem. 88, 26–40. doi:10.1016/j.enganabound.2017.12.007
Sun, W., Starly, B., Nam, J., and Darling, A. (2005). Bio-CAD modeling and its applications in computer-aided tissue engineering. Cad. Comput. Aided Des. 37, 1097–1114. doi:10.1016/j.cad.2005.02.002
Sutradhar, A., Park, J., Carrau, D., Nguyen, T. H., Miller, M. J., and Paulino, G. H. (2016). Designing patient-specific 3D printed craniofacial implants using a novel topology optimization method. Med. Biol. Eng. Comput. 54, 1123–1135. doi:10.1007/s11517-015-1418-0
Tan, X. P., Tan, Y. J., Chow, C. S. L., Tor, S. B., and Yeong, W. Y. (2017). Metallic powder-bed based 3D printing of cellular scaffolds for orthopaedic implants: a state-of-the-art review on manufacturing, topological design, mechanical properties and biocompatibility. Mater. Sci. Eng. C 76, 1328–1343. doi:10.1016/j.msec.2017.02.094
Tang, Y., Hascoet, J.-H., and Zhao, Y. F. (2014). “Integration of topological and functional optimization in design for additive manufacturing,” in ASME 2014 12th Biennial Conference on Engineering Systems Design and Analysis, Copenhagen, Denmark.
Tang, Y., Kurtz, A., and Zhao, Y. F. (2015). Bidirectional Evolutionary Structural Optimization (BESO) based design method for lattice structure to be fabricated by additive manufacturing. Cad. Comput. Aided Des. 69, 91–101. doi:10.1016/j.cad.2015.06.001
Thompson, M. K., Moroni, G., Vaneker, T., Fadel, G., Campbell, R. I., Gibson, I., et al. (2016). Design for additive manufacturing: trends, opportunities, considerations, and constraints. CIRP Annals 65, 737–760. doi:10.1016/j.cirp.2016.05.004
Van Dijk, N. P., Maute, K., Langelaar, M., and Van Keulen, F. (2013). Level-set methods for structural topology optimization: a review. Struct. Multidiscip. Optim. 48, 437–472. doi:10.1007/s00158-013-0912-y
van Manen, T., Janbaz, S., Jansen, K. M. B., and Zadpoor, A. A. (2021). 4D printing of reconfigurable metamaterials and devices. Commun. Mater. 2, 56–58. doi:10.1038/s43246-021-00165-8
Van Noort, R. (2012). The future of dental devices is digital. Dent. Mater. 28, 3–12. doi:10.1016/j.dental.2011.10.014
Virulsri, C., Tangpornprasert, P., and Romtrairat, P. (2015). Femoral hip prosthesis design for Thais using multi-objective shape optimization. Mater Des. 68, 1–7. doi:10.1016/j.matdes.2014.11.027
Wall, W. A., Frenzel, M. A., and Cyron, C. (2008). Isogeometric structural shape optimization. Comput. Methods Appl. Mech. Eng. 197, 2976–2988. doi:10.1016/J.CMA.2008.01.025
Wang, M. Y., Wang, X., and Guo, D. (2003). A level set method for structural topology optimization. Comput. Methods Appl. Mech. Eng. 192, 227–246. doi:10.1016/S0045-7825(02)00559-5
Wang, X., Xu, S., Zhou, S., Xu, W., Leary, M., Choong, P., et al. (2016). Topological design and additive manufacturing of porous metals for bone scaffolds and orthopaedic implants: a review. Biomaterials 83, 127–141. doi:10.1016/j.biomaterials.2016.01.012
Wang, Y., and Kang, Z. (2018). A level set method for shape and topology optimization of coated structures. Comput. Methods Appl. Mech. Engrg 329, 553–574. doi:10.1016/j.cma.2017.09.017
Wettergreen, M. A., Bucklen, B. S., Starly, B., Yuksel, E., Sun, W., and Liebschner, M. A. K. (2005). Creation of a unit block library of architectures for use in assembled scaffold engineering. Cad. Comput. Aided Des. 37, 1141–1149. doi:10.1016/j.cad.2005.02.005
Williams, J. M., Adewunmi, A., Schek, R. M., Flanagan, C. L., Krebsbach, P. H., Feinberg, S. E., et al. (2005). Bone tissue engineering using polycaprolactone scaffolds fabricated via selective laser sintering. Biomaterials 26, 4817–4827. doi:10.1016/j.biomaterials.2004.11.057
Wu, C. T., Yang, Y. H., and Chang, Y. Z. (2022). Three-dimensional deep learning to automatically generate cranial implant geometry. Sci. Rep. 12, 2683–2710. doi:10.1038/s41598-022-06606-9
Xiao, D. M., Yang, Y. Q., Su, X. B., Wang, D., and Luo, Z. Y. (2012). Topology optimization of microstructure and selective laser melting fabrication for metallic biomaterial scaffolds. Trans. Nonferrous Metals Soc. China 22, 2554–2561. doi:10.1016/S1003-6326(11)61500-8
Xiao, Z., Yang, Y., Xiao, R., Bai, Y., Song, C., and Wang, D. (2018). Evaluation of topology-optimized lattice structures manufactured via selective laser melting. Mater Des. 143, 27–37. doi:10.1016/j.matdes.2018.01.023
Xu, M., Zhang, Y., Wang, S., and Jiang, G. (2022). Genetic-based optimization of 3D burch–schneider cage with functionally graded lattice material. Front. Bioeng. Biotechnol. 10, 819005. doi:10.3389/fbioe.2022.819005
Yang, S., and Zhao, Y. F. (2015). Additive manufacturing-enabled design theory and methodology: a critical review. Int. J. Adv. Manuf. Technol. 80, 327–342. doi:10.1007/S00170-015-6994-5
Zegard, T., and Paulino, G. H. (2016). Bridging topology optimization and additive manufacturing. Struct. Multidiscip. Optim. 53, 175–192. doi:10.1007/s00158-015-1274-4
Zhang, W., and Zhou, L. (2018). Topology optimization of self-supporting structures with polygon features for additive manufacturing. Comput. Methods Appl. Mech. Eng. 334, 56–78. doi:10.1016/j.cma.2018.01.037
Keywords: structural design optimization, topology optimization, lattice structures, additive manufacturing, medical implants, lightweight design, architected materials
Citation: Peto M, García-Ávila J, Rodriguez CA, Siller HR, da Silva JVL and Ramírez-Cedillo E (2024) Review on structural optimization techniques for additively manufactured implantable medical devices. Front. Mech. Eng 10:1353108. doi: 10.3389/fmech.2024.1353108
Received: 09 December 2023; Accepted: 15 February 2024;
Published: 01 March 2024.
Edited by:
Kanak Kalita, Vel Tech Dr. RR & Dr. SR Technical University, IndiaReviewed by:
Davood Rahmatabadi, University of Tehran, IranMetin U. Salamci, Gazi University, Türkiye
Pradeep Jangir, Rajasthan Rajya Vidyut Prasaran Nigam Limited (RVPN), India
Copyright © 2024 Peto, García-Ávila, Rodriguez, Siller, da Silva and Ramírez-Cedillo. This is an open-access article distributed under the terms of the Creative Commons Attribution License (CC BY). The use, distribution or reproduction in other forums is permitted, provided the original author(s) and the copyright owner(s) are credited and that the original publication in this journal is cited, in accordance with accepted academic practice. No use, distribution or reproduction is permitted which does not comply with these terms.
*Correspondence: Erick Ramírez-Cedillo, ZXJpY2tyYW1jZUB0ZWMubXg=
†These authors have contributed equally to this work and share first authorship