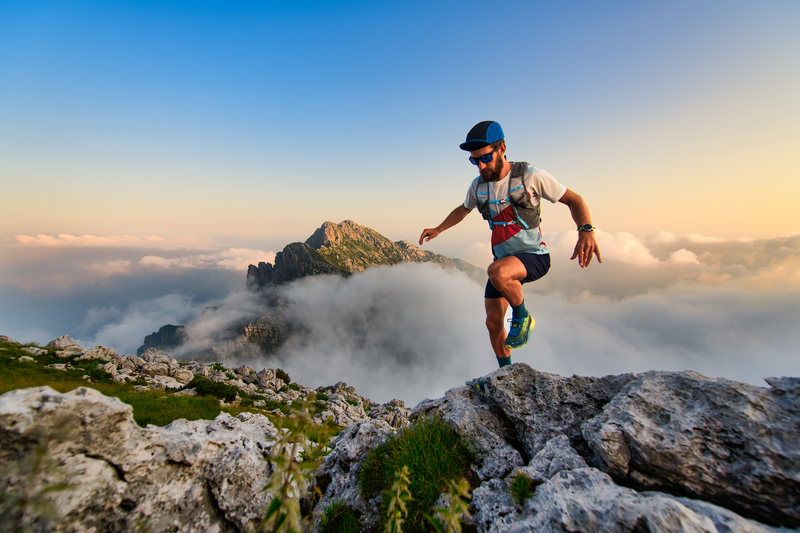
95% of researchers rate our articles as excellent or good
Learn more about the work of our research integrity team to safeguard the quality of each article we publish.
Find out more
REVIEW article
Front. Mech. Eng. , 01 June 2021
Sec. Engine and Automotive Engineering
Volume 7 - 2021 | https://doi.org/10.3389/fmech.2021.676853
This article is part of the Research Topic Smart Energy Systems View all 5 articles
The energy generation landscape is changing, pushed by stricter regulations for emissions control and green energy generation. The limitations of renewable energy sources, however, require flexible energy production sources to supplement them. Micro gas turbine based combined heat and power plants, which are used for domestic applications, can fill this gap if they become more reliable. This can be achieved with the use of an engine monitoring and diagnostics system: real-time engine condition monitoring and fault diagnostics results in reduced operating and maintenance costs and increased component and engine life. In order to allow the step change in the connection of small engines to the grid, a fleet monitoring system for micro gas turbines is required. A proposed framework combines a physics-based model and a data-driven model with machine learning capabilities for predicting system behavior, and includes a purpose-developed diagnostic tool for anomaly detection and classification for a multitude of engines. The framework has been implemented on a fleet of micro gas turbines and some of the lessons learned from the demonstration of the concept as well as key takeaways from the general literature are presented in this paper. The extension of fleet monitoring to optimal operation and production planning in relation to the needs of the grid will allow the micro gas turbines to fit in the future green energy system, connect to the grid, and trade in the energy market. The requirements on the system level for the widespread use of micro gas turbines in the energy system are addressed in the paper. A review of the current solutions in fleet monitoring and diagnostics, generally developed for larger engines, is included, with an outlook into a sustainable future.
When looking at energy generation, the share of renewable energy is continually increasing, and the European Union targets a 32% share of renewables along with an improvement of at least 32.5% in energy efficiency in 2030 compared to projections of future energy consumption, and a significant reduction of greenhouse gas emissions compared to 1990 levels (European Council, 2020), as depicted in Figure 1. The key targets for 2030 have been upgraded in comparison to those agreed in 2014 (European Council, 2014), with an updated proposal expected by June 2021 (European Council, 2020).
The use of wind and solar power whose generation is intermittent and unpredictable could result in stability issues for the grid and in a time lag between supply and demand of electricity. This results in a significant ramp of demand in the evening hours, graphically represented in what has become known as the “duck curve” (California Independent System Operator, 2016). The difference in supply and demand requires energy storage solutions; energy storage possibilities are now a hot topic of discussion. Pumped hydro storage accounts for the majority of energy stored around the world (US Department of Energy (DOE), 2021), but is typically limited to mountainous regions. The use of short term batteries at a large scale could provide a solution, and research on different battery technologies is conducted around the world (IEA International Energy Agency, 2014). Battery energy storage offers quick response times, but cost is still a major limiting factor for production. However, the demand for battery storage is expected to increase in the longer term (IEA International Energy Agency, 2014). The short to mid-term energy situation creates an opportunity for small combined heat and power (CHP) units, which can provide flexibility of energy production when connected to the grid. Distributed generation of heat and power can assist in the move toward cleaner energy by reducing transmission losses. In the longer term and with further technology development, batteries and gas turbines could co-exist in the future energy system to increase flexibility. As the world is moving toward fossil-free fuels, a fast way to reduce carbon emissions is to replace coal-fired plants with gas-powered gas turbines, as argued by Langston (2020). After all, gas turbine manufacturers have already investigated operating their engines with alternative fuels such as hydrogen, either pure or mixed with natural gas.
The reduction in energy consumption and the improvements in energy efficiency need to come from all parties involved. However, with buildings accounting for 40% of Europe’s energy consumption (European Commission, 2016), there is a significant margin for improvement. In fact, the role of buildings in the EU policy is expected to expand from energy savings to becoming active elements in future energy systems, allowing their occupants to use, supply and store energy in a more flexible and smarter way. The use of smart electricity meters in the EU (where it was expected to reach 100% implementation by 2020 in many countries, (European Commission, 2017) and worldwide allows real-time billing and can help in the reduction of electricity consumption. This needs to be done by engaging the consumers and providing incentives for them to reduce their electricity consumption. A number of studies in Sweden, where substantial practical experience has been gained through the full roll out of smart meters (first country to complete this, in 2009), showed the complexity of customer response to different incentives and highlighted that the potential for energy savings has not been fully exploited (Vassileva and Campillo, 2016). It was noted that a combination of environmental and economic incentives, taking into account the income, educational level and use patterns of the consumers is required in order to maximize energy savings (Vassileva and Campillo, 2014).
A grid based on distributed generation and small combined heat and power plants is a good solution to the problem of energy production flexibility. The key objectives of this paper are to identify the main requirements for micro gas turbines to fill the gap in energy production until the generation of energy can fully rely on renewable energy sources.
The section that follows discusses the historical development of micro gas turbines that have allowed them to become more energy efficient and a good option for distributed generation of energy as well as the progress to date in their operation with different fuels. The current state of the art in gas turbine monitoring for maintenance and diagnostics is then presented, as well as the enablers for the development of a framework for fleet monitoring that extends the better established diagnostics functionalities to optimal operation and production planning. The paper concludes with the discussion of the concept that will allow distributed micro gas turbines to connect to the smart grid and trade in the energy market, the challenges and opportunities for micro gas turbine fleet control and diagnostics, and the key elements of the proposed framework.
In the recent years, work in the field of micro gas turbines (particularly those with low power output levels of 1–100 kW) has focused on the development of components and engines with efficiency levels closer to those of larger gas turbines. The efficiency levels are limited by small-scale effects, such as low Reynolds numbers which result in high viscous losses, high tip clearances due to manufacturing tolerances, large area-to-volume ratio resulting in high heat losses, and relatively high auxiliary system losses due to the low power output level (Visser et al., 2011). Until recently, overall costs posed a limitation to the development of components, which is crucial for the increase of overall engine efficiency. However, the development of small turbochargers with efficiencies close to those of small gas turbines has allowed the development of micro gas turbines with practical efficiency levels, using off-the-shelf turbocharger parts from the automotive industry. State of the art component isentropic efficiencies for small turbocharger turbomachinery are at the level of 75% for compressors and 70% for turbines (Visser et al., 2011; Visser et al., 2012) with further room for improvement by optimizing the components for the particular application. In Visser et al. (2011), a conceptual design of a turbocharger-based microturbine for a heat demand driven micro combined heat and power (CHP) unit was proposed, with 3 kW of electric power, 15 kW for heating and hot water, and a target electrical efficiency of 16%. A competitive market for these small CHP units is domestic applications, with the units replacing conventional boilers in larger houses, as well as in small offices, where the output of the unit can cover daily needs. With an increase in electrical efficiency to 20% coming from design optimization and expected technology development, such systems can become even more competitive.
The micro gas turbines available in the market today cover a large range of power output. Some of the smallest commercial solutions for distributed power generation are the EnerTwin by MTT in the Netherlands which produces 3 kW electric power fueled by natural gas and the MGT by Bladon in the United Kingdom which produces 12 kW of electric power using diesel and/or kerosene as fuel. Larger commercial solutions for CHP have been provided by the market leaders Ansaldo Energia with the 100 kW AE-T100, Capstone with a range of gas turbines starting from 30 kW, FlexEnergy with the 333 kW GT333S. More recently, Aurelia Turbines in Finland has introduced the A400, a 400 kW model with a stated electrical efficiency of 40%, which is the highest in the market of small gas turbines.
The typical operating fuel for these engines has been natural gas, but alternative fuels have become more and more common in the recent years. In fact, much of the research has focused on fuel flexibility for micro gas turbines (Bo et al., 2018; Bower et al., 2020). Operation with a different fuel will often require the redesign of the combustor to meet the demands of lower grade fuels and some manufacturers opt for an externally fired configuration that can increase modularity. Commercially available gas turbines by Ansaldo Energia and Capstone can operate with biogas as well as with other fuels in an externally fired configuration. The Aurelia Turbines A400 can also run with a variety of fuels, including hydrogen (Aurelia Turbines, 2020). Units as small as the 3 kW micro gas turbine by MTT have been tested with fuel blends containing hydrogen and methane, with modifications to the combustor to allow for the faster flame characteristics and prevent flashbacks (du Toit et al., 2020).
The use of hydrogen has been advocated for its zero emissions and has long been suggested as an energy storage fuel (Turner, 2004). This pathway can take advantage of surplus renewable energy from wind and solar for electrolysis of water for the production of hydrogen that can be stored for later use. This can solve the energy storage problem. However, the use of hydrogen does not come without challenges. Running a gas turbine with hydrogen requires significant changes in its combustor and the fuel is less dense in terms of energy per unit volume compared to fossil fuels. The requirements for transport and storage of hydrogen imply that dedicated facilities are needed, which represents a major stumbling block in its widespread use. In a thorough review of the research in the field, Abe et al. (2019) conclude that compressed hydrogen in a gaseous or liquid form has multiple limitations, whereas solid-state storage solutions show potential but do not yet meet all the criteria for practical and economic hydrogen storage. However, hydrogen can already be used in existing pipelines mixed with natural gas (Langston, 2019).
Alternative fuels that can be produced from hydrogen can overcome some of these limitations, as they have higher energy density and are easier to store. Adding carbon captured from different sources to hydrogen can form a hydrocarbon with zero net greenhouse gas emissions. This synthetic fuel can then be used in different engines. Renewable synthetic fuels (e-fuels) generated exclusively with renewable sources are hailed as the route to sustainable transportation. Such fuels include e-methane, e-diesel, e-methanol. Another fuel option is ammonia, which can be produced through the same pathways, but is more toxic and has other disadvantages such as low flammability, high NOx emissions, and low radiation intensity (Kobayashi et al., 2019). Even though it was shown to have a narrow range of efficient combustion when used in fuel blends in gas turbines (Valera-Medina et al., 2015), research in ammonia-fuelled gas turbines has been increasing, with Kobayashi et al. (2019) achieving combustion of 100% ammonia. Mitsubishi Power is targeting the commercialization of the first ammonia-fuelled gas turbine in a size scale of 40 MW around 2025 (Mitsubishi, 2021). This route, however, is not without obstacles either. At present, the generation of e-fuels is relatively inefficient and expensive. The energy coming from renewable sources is becoming cheaper, but is still at a cost. The electrolysis process has its own limited efficiency and a high capital cost, which is a limiting factor to hydrogen production that can allow the synthesis of different fuels.
The production of alternative fuels from non-renewable sources can be a near-term solution, but the generation of carbon-neutral fuels will require that the energy input in the process is itself fully renewable. Fuels derived from biomass (biofuels), such as methanol, ethanol, or biodiesel, are considered renewable but their sustainability, especially when accounting for their ecological footprint, is a topic of debate (Stoeglehner and Narodoslawsky, 2009; Solomon, 2010). The need for biofuel sustainability criteria that are consistently applied worldwide is highlighted by Solomon (2010), as some pathways were found to be unsustainable whereas others have significant ecological and socio-economic impact.
E-fuels are a promising pathway to sustainable transportation, in particular for the aviation industry, with electric cars presenting a more promising alternative to ground transportation due to the higher efficiency of the entire chain and their lower cost (Searle, 2020). However, the use of electric vehicles is a few steps into the future, as it depends on consumer choices and material availability. In order to achieve net zero emissions, neither the transportation nor the power generation industry can rely on just one option; all different alternatives that offer cleaner energy generation need to be researched and employed in this journey.
These developments pave the way for the use of smaller and smaller engines for distributed generation of heat and power with cleaner fuels, something which is not too far into the future. The key element will then become the reliability of the engine in order to be able to compete with conventional systems.
In order to increase the reliability of gas turbines, a monitoring and diagnostics system is required. Monitoring the condition of a gas turbine, detecting anomalies in its operation and diagnosing faults aims to reduce engine life cycle costs, for both operation and maintenance, and to increase safety. This is done through avoiding unscheduled maintenance, detecting partial failures, improving repair schedules and increasing overhaul.
The detection of faults in the engine components is based on deviations in the values of component performance parameters from the baseline values of a “healthy” engine. This requires that the operating parameters of the engine are measured in real time and used to calculate key performance parameters. These can then be compared to those predicted by a model of the engine. A mathematical framework for this process, referred to as Gas Path Analysis (GPA), was provided by Urban (1973). This approach uses the measurable parameters in the gas path of the engine to detect the change in component health parameters. The main principle lies in the fact that hardware faults result in degraded component performance, producing changes in measurable parameters. The detection of these changes allows the isolation of the component whose performance has degraded and the correction of the faulty hardware.
Gas path analysis has been a key tool for engine diagnostics, initially employing a linear model for the gas turbine. Extensive research has followed in the field, with Stamatis et al. (1990) introducing a method to take into account the non-linearities in engine behavior and other studies proposing different techniques to counteract some of the drawbacks of these early models. The models themselves form an integral part of the diagnostic system, and can be physics-based, data-driven, or hybrid (a mixture of the two). Numerous papers have given an overview of different aspects of the vast literature in the field. As (Li, 2002) highlights, performance analysis has remained one of the most powerful tools for gas turbine condition monitoring and fault diagnostics. Engine gas-path performance monitoring represents the majority of engine health management related research (Jaw, 2005). An overview of the fundamental theory can be found in Volponi (2014) along with a timeline of past, present, and future trends.
While the research within the field of gas turbine diagnostics mainly evolved around exploring different variants of gas path analysis techniques, numerous studies were performed to explore methods for proper selection of measurement parameters. Traditionally, commercial gas turbines are equipped with a limited number of sensors that are essential for safe operation and control (Mathioudakis et al., 2004). However, to ensure high diagnostics accuracy, adequate instrumentation with acceptable quality might be needed. On the other hand, over-instrumentation does not necessarily guarantee better diagnostics; it can lead to increased cost due to installation and maintenance of redundant sensors. Hence measurement selection is essential for setting up gas path analysis based diagnostics. Ogaji et al. (2002) used a non-linear gas-path analysis model to select the required instrumentation set. The authors optimized the selection with respect to the number and type of sensors and their locations for the considered engine-faults. Jasmani et al. (2011) presented an analytical measurement selection method based on sensitivity and correlation analysis combined with a measurement subset concept for multiple component fault diagnosis. In this study, measurements with higher sensitivity and correlation are prioritized in the selection process. The measurement subset concept supplements the outcome of the sensitivity and correlation analyses with visualization and priority. In another study, Stenfelt et al. (2019) proposed a measurement selection method that automatically modifies the selection under sensor malfunction to ensure robust diagnostics. The authors emphasized that the use of correlated measurements in gas path diagnostics should be avoided. In all studies, the key parameters identified are the pressure and temperature at the exit of the components (compressor, combustor, turbine), the fuel flow rate, and the spool speed.
The basis for gas path analysis can be either a physics-based or a data-driven model. The two approaches, covered in a number of review articles (Li, 2002; Marinai et al., 2004; Stamatis, 2011; Fentaye et al., 2019) have their advantages and disadvantages. The outcome of these analyses, based on criteria such as model complexity, accuracy, reliability, computational efficiency, dealing with noise and limited numbers of measurements is that there is not one approach that outperforms the rest. Therefore, a hybrid scheme that combines physics-based and data-driven methods would be the best choice. In the area of modeling, where the target is that this is performed real time on-board for aero-engines, the main challenges identified are the need for dependable and reliable on-board models that cover safety-critical parameters, especially during transient operation (Wei et al., 2020).
The employment of gas turbine diagnostics has seen a significant increase in research in the field in the last 2 decades, as major engine manufacturers introduced remote monitoring and diagnostic services (Ozgur et al., 2000; Brummel et al., 2005; Therkorn, 2005). Developments in gas turbine diagnostics and diagnostics-oriented modeling have been covered in recent review articles which identified research trends and challenges. In gas path diagnostics, the main drivers for research have been the limited number of sensors, measurement uncertainty, engine non-linearity and dealing with simultaneous faults. To address this, Fentaye et al. (2019) suggests that researchers should converge toward a universally accepted definition of the gas path diagnostic problem as well as focus on the development of integrated platforms and hybrid methods to combine the advantages of different techniques and models. The fusion of information from different sources in order to address the limitations of different methods and enhance the capabilities of a decision support system has also been the focus of multiple research studies. It has been extensively used on the sensor level, but its use for decision support and diagnostics has been relatively limited (Zaccaria et al., 2019). However, since it offers benefits in dealing with incomplete data, it can be particularly beneficial for small and micro-scale gas turbines, allowing the development of an automated diagnostics and decision support system.
Earlier researchers have made significant contributions in improving gas turbine diagnostics methods. However, the majority of these studies mainly considered large scale gas turbines with only a few focusing on micro gas turbines. Performance-based diagnostics of a micro gas turbine posses additional challenges. To keep the manufacturing costs down, micro gas turbines are often equipped with only a few sensors. Moreover, the measurements often suffer from poor accuracy due to the use of low cost sensors. Rahman et al. (2018) presented a hybrid approach for micro gas turbine diagnostics by combining gas path analysis and multiple linear regression. The authors used five different measurements (i.e. compressor outlet pressure, recuperator outlet temperature, turbine outlet temperature, shaft speed and power) to diagnose seven different faults. The results showed that the proposed diagnostics approach performs satisfactorily even under measurement uncertainties.
Simultaneous monitoring of a set of assets has been the next step in the field. Activities ranging from engine monitoring, health tracking, and fault diagnosis to fleet health management, are performed by commercial airlines and power plant operators, original equipment manufacturers (OEMs) and independent maintenance facilities (Volponi, 2014). Fleetwide monitoring programs were initiated in the power generation industry (Plotts and Diatzikis, 2009; Johnson, 2014). Companies and customers value the information that can be provided to assist in troubleshooting or avoid an unplanned event and the effect this has on overall plant efficiency. Information from the fleet is then used to provide a baseline for engine performance and estimate the nominal range of various operating parameters.
Research studies have reflected this, starting to look into engine fleet monitoring and diagnostics, basing their approach on data-driven models. Aircraft fleet monitoring has been addressed by Chu et al. (2010), who constructed a data-driven model for aircraft operation using historical fleet data for anomaly detection. They then presented an approach for population-wide monitoring and detection of performance anomalies, performance shifts, and anomalous units in a fleet of aircraft (Chu et al., 2011). They pointed out that the method needs to be scalable in order to handle large scale fleet data and the model should be quickly computable. Different approaches were presented by Scheianu (2014) for diagnostics of an industrial gas turbine fleet based on performance monitoring and by Borguet et al. (2016) for data-driven modeling of a fleet of engines, applied to a virtual fleet of generic high bypass ratio turbofans. A framework for monitoring and diagnostics of a virtual fleet of aero-engines was presented by Zaccaria et al. (2018), pointing out the requirements for adaptive models in order to accurately detect and classify faults. Machine learning techniques are used more and more for anomaly detection in different applications with promising results, and lessons learned from these can also be transferred to fleet monitoring applications.
The ultimate goal in engine condition monitoring and health management is the prediction of the future state of the engine and its components (prognostics). This is linked to diagnostics and judges the impact of a specific fault on the engine or component. It can also take into account the degradation of the components and predict their remaining useful life. Knowledge of when a component might fail allows the shift from reactive to proactive maintenance, significantly reducing response time and costs. Discussion of prognostics has been on the table since the 2000s; Roemer and Kacprzynski (2000) presented an integrated set of health monitoring, diagnostic, and prognostic technologies for turbomachinery, highlighting the requirements for the prediction of remaining useful life. Many physics-based prognostic models have been presented in the literature, but their application in real systems is quite limited because they are generally complex and computationally expensive, defect-specific, and various parameters need to be determined for each system (Li et al., 2000; Oppenheimer and Loparo, 2002; Qiu et al., 2002). Data-driven models such as exponential smoothing, autoregressive models, and artificial neural networks have been widely employed (Wang et al., 2004; Orchard et al., 2005). A simple but effective regression method combined with statistical knowledge for prognostic analysis has been proposed by Li and Nilkitsaranont (2009). An extensive review of prognostic methods and approaches can be found in Heng et al. (2009).
The advances in instrumentation, communication techniques, and computer technology have allowed the development of advanced monitoring tools for a large range of machines and household devices. The use of monitoring and diagnostics is increasing rapidly across many industries and applications, as sensors are getting smaller, cheaper, smarter, and widespread. Computing is becoming exponentially cheaper and wireless visualization will enable wider deployment. Developments in artificial intelligence (AI), with the rise of cloud-based big data platforms and the success of deep learning, have made it easy to collect and analyze large volumes of data (Najafabadi et al., 2015). Therefore, the introduction of technology for the monitoring of machines can now provide significant payback.
Ongoing trends in digitalization and automation show that consumer expectations have also changed. In order for a device to be competitive in the market, it needs to offer a diagnostics and decision support system. This is no longer limited to large gas turbines worth 100 million Euro, it is an expectation from almost all “top of the market” household appliances. Consumers expect to be able to monitor their appliances from their phones; these expectations and the wide use of this technology open up new opportunities for products with a lower acquisition cost that were unthinkable a few years ago. In order for the micro-CHP system to be attractive in the connected future, it needs to offer similar capabilities. If it is to be connected to an increasingly renewable-heavy energy system, it needs to provide even more advanced capabilities than today’s and tomorrow’s expectations. When energy dispatchability and grid stability becomes a key concern, an intelligent diagnostic and decision support system will be required. The engine of the future, referred to as the intelligent engine, uses engine health management for adaptive control based on the estimated state of health of the engine. This system conducts analysis on-board, allowing the estimation of deterioration, faults, and generating diagnostic and prognostic information to support line maintenance and overhaul logistics (Volponi, 2014). A key aspect of this is the construct of an accurate on-board model that is calibrated to the particular engine being monitored, the digital twin. As the cities get smarter, the energy consumption and overall efficiency and emission targets get stricter, and the grid can support more advanced technologies, a framework that allows systems to be connected needs to be in place.
Distributed generation of heat and power has changed the energy market in the last decades, offering many benefits, especially to their owners, but at the same time posing challenges mainly in terms of reliability and resilience when connected to the grid. These small electricity production units can be clustered together and monitored and controlled as a single entity, in a concept referred to as the virtual power plant (VPP). This is defined as the aggregation of load/generation/storage that can act as a single entity in the electricity grid and market (Pandzic et al., 2013; Oates and Melia, 2016). Research has been done on different aspects of a virtual power plant, mostly on improving the visibility, controllability in terms of electricity grid stability, and market functionality i.e. scheduling of distributed energy resources (Saboori et al., 2011; Ghavidel et al., 2016; Nosratabadi et al., 2017). With regards to a micro-CHP virtual power plant, research has focused on the technical feasibility, the economic potential, and the institutional environment.
A number of studies have addressed specific issues arising in the path of connecting micro gas turbines to the grid, mostly focusing on larger engines of the order of 100 kW. The production of a large number of engines will result in small variations in design parameters (production scatter) or operating parameters due to installation effects. These will have an impact on the performance parameters of the engine, which will in turn affect the optimal operation of the micro gas turbine. Paepe et al. (2019) suggested that robust optimization of the operation of the mGT by controlling the turbine outlet temperature and rpm can minimize this and maximize electrical efficiency and power output. This should be taken into account for modeling and production planning. An application study by di Gaeta et al. (2017) presented a scenario where a micro gas turbine is used to compensate the deficit in energy coming from renewable sources. They developed a simplified model of the MGT that can be coupled to the simulation and control of a hybrid energy grid operating with renewable energy sources and hydrogen storage, resulting in savings of 37–41% in fossil fuel consumption, depending on the level of hybridization of the grid.
Once the micro-CHPs are connected to the grid (be it on a neighborhood or larger scale) they can then trade energy in the market according to spot prices and hour by hour price variation for each day. An application study (Rist et al., 2017) analyzed the economic dispatch of a single gas turbine based micro-CHP unit in four cases (small and large hotel, restaurant, residential building), employing a comprehensive model for the engine, real demand profiles and electricity tariffs. They identified four different operation modes for the MGT: electricity driven, heat driven, maintenance cost driven, and revenue driven. Their analysis concluded that the connection of the micro-CHP to a smart grid can offer significant economic benefits in all four cases, highlighting that using the micro-CHP for electricity generation in residential buildings in times of peak demand has a large cost benefit compared to buying energy from the utility provider. In such situations, the unit can operate in electricity driven mode, covering the electricity needs of the building, or a revenue driven mode, selling energy to the grid. Their analysis, however, does not take into account the effect that variations in the load and frequent switching on and off of the engine will have on its lifetime.
In order for the units to be used for trade in the energy market, a framework for the management of micro CHP is essential. This framework will take care of control and diagnostics of the fleet and will also allow management of the fleet and result in the reduction of overall life cycle costs. This requires the use of historical data to predict the remaining lifetime of the engine. The framework will also include a cost function so then the system can make a decision on whether it is profitable or not to start the GT to provide electricity to the network. The concept for distributed generation of combined heat and power is schematically depicted in Figure 2.
FIGURE 2. The micro gas turbine fleet concept for distributed generation of combined heat and power (CHP).
The use of data from a large family of similar units has been proposed in the literature and applied in different fields. The main lessons learned from these applications that are applicable to the micro gas turbine case are the requirements for a scalable method and small computational times for the models. The models themselves should be adaptive in order to deal with production scatter and engine degradation. The framework should be automated in order to handle a large amount of data, and provide data processing, engine management, prediction of service and maintenance needs and include a visualization platform. The diagnostics and prognostics system should aim to collect and digitalize the experience obtained through maintenance, repair, and overhaul (MRO) and provide a tool that is user friendly and uses continuous learning techniques in order to build on the initial trained model and align the decisions of the tool to those of the experienced operators. In order for the operators to use the diagnostics and decision support tool, it should first gain their trust and show that it can make analyses that are not always possible by a human. If the tool can use data from thousands of engines in the fleet and analyze them to predict maintenance action requirements or predict component failure, the operators would start trusting the system and that would increase its applicability and use. This experience and trust can be instilled into the owners of the micro-CHP units by following a similar approach.
The shift from a large, centralized plant to a fleet of distributed micro-CHP engines raises a number of challenges for the control and diagnostics of the fleet and for the operation of the engines in the grid but also provides a number of opportunities due to the large number of “sister” engines in the fleet:
• There is significant variability between engines of the fleet, therefore a single engine model is not sufficient for fleet diagnostics, as the differences between the engines are not negligible (Niedler and Fiedler, 1999). A model of an average turbine needs to be combined with data-driven regression of every engine in “healthy” conditions to remove the effects of production scatter. The operating conditions of each machine should also be taken into consideration.
• Engine degradation will result in the deviation of the measurements from those of a healthy engine, and an adaptive model should be used to monitor the trend of the measurement data and predict component failure.
• The aim for low engine costs limits the number of sensors used much more than in larger engines, rendering the detection of sensor faults more difficult.
• The gas turbine community should understand the market in order to figure out what will make micro gas turbines more attractive to the customers.
Fleet monitoring applications in the currently deployed systems typically do not take advantage of the multitude of data from a fleet of similar engines to improve the diagnostics capabilities. This makes them essentially multiple single unit monitoring applications working individually in parallel and depicted on the same map.
As technology advancement has been reaching different parts of the industry at different times, many of the advanced functionalities that can enable the operation of assets in the most efficient and cost-effective way have been developed separately. However, in order to increase automation, process efficiency and reduce maintenance costs, these functionalities (modeling, diagnostics, control, and decision support) should be included in an integrated approach. A holistic system can help the industry move from a collection of sub-optimal solutions to individual problems to an optimal solution for the management of the entire fleet. This section presents a framework for the management of a fleet of units, and some of the lessons learned from its application to real engines, which highlight the requirements for the widespread use of micro gas turbines in the future smart energy system.
The starting point for the proposed framework is the identification of a need for a modular architecture for a learning system that can be applied to existing automation platforms in the industry. This has been addressed in projects funded by the EU in the Sustainable Process Industry through Resource and Energy Efficiency program (SPIRE, 2021). An architecture for a generic learning system for process industry was proposed and developed in the FUDIPO (2021) project and is depicted in Figure 3 presented by Rahman et al. (2021). The main elements for this learning system that are relevant to the present application are:
1. Data assurance, the pre-processing of data that includes cleaning, smoothing and scaling.
2. Trend analysis, which essential for diagnostics and decision support, most commonly with the use of first and second derivatives.
3. Engine model, typically used for prediction and analysis.
4. Model predictive control to optimize the behavior of the units.
5. Anomaly detection, detection of abnormal conditions in the operation of the engine.
6. Fault diagnostics to provide a possible cause for the anomaly detected.
7. Sensors to collect data and store it in the database.
8. Decision support system, which analyses data and provides decisions for operation, planning and management.
9. Human-machine interface to present the data to the user or operator.
FIGURE 3. Learning system architecture (Rahman et al., 2021).
The implementation of this framework also brings specific challenges. The main one is the integration of highly heterogeneous models in a holistic system. This can be solved on the platform level, by decoupling model services and running them in containers that can be customized, thus enabling different models to run on the same platform (Lipenko et al., 2021). The framework needs to be implemented in a way that will not affect the calculation performance of models in order to allow them to be used for optimization of the process. The models themselves can be executed on premises or on the cloud, with each option offering advantages and disadvantages, discussed by Lipenko et al. (2021). The framework will also need to meet the requirements for dealing with large amounts of data identified by Morris et al. (2014): handle data produced by different sensors, which arrives rapidly, in large volumes, with noise and is varying in structure. Data must be available to operators and users in a convenient manner and data security must also be ensured.
As the production of power is shifted toward distributed micro-CHP units and the number of systems in a fleet increase from a few dozen to hundreds or thousands, the approach for monitoring and diagnostics needs to be extended to a wider framework. The main points of departure for this framework are:
• A model for each system would result in too many models once the number of systems increases, therefore a single model should be used for each engine in the fleet, with a unique set of tuning parameters for each system.
• The engine model needs to be simplified in order to run in real time and enable the unit to trade in the market.
• The presence of a multitude of engines can be utilized for diagnostics purposes, increasing the amount of information available for healthy engines. However, this requires a big amount of data to be collected, stored, and pre-processed to extract useful information.
• In the calculation of remaining useful lifetime, the usage profile needs to be taken into account, as the frequent start and stop decrease the remaining life of the engine. This can be done using historical data. The collection of data from multiple engines also increases the confidence of the calculation of remaining useful life.
• A unique cost function and weather forecast function for each engine is required to optimize the operation of the engine and allow the user to sell energy to the grid.
The learning system architecture presented by Rahman et al. (2021) can be adapted to fit the monitoring, diagnostics and production planning, as depicted in Figure 4. Each unit within the micro gas turbine fleet is connected to a PC, enabling remote monitoring and data collection through a web connection. A set of parameters from every unit is logged at 1 min frequency in the fleet historian. The historical and live data is used to monitor the status of the installed units in the condition monitoring, diagnostics, and prognostics module, which includes a model for the engine as well as anomaly detection and diagnostics functions. The module includes an engine prognostics function to predict the remaining life of each unit and its individual components that can enable predictive maintenance. The optimal operation and production planning module receives as input the weather forecast and the real-time electricity price in addition to the data from the fleet. This module performs a cost analysis taking also into account the output of the prognostics function, and includes a model predictive controller for optimal operation suggestions. These two modules provide the necessary input for the engine decision support tool that can make suggestions (or take actions once it has gained the trust of the user) for maintenance and optimal operation.
To enable automated fleet diagnostics capability, a data processing and model execution module is utilized. At first, the data from the fleet historian is collected and different data-filtering techniques are performed to remove outliers and noise. Trend analyses are conducted on the data to track the patterns of different parameters. In order to develop the monitoring and diagnostics functionality for the fleet, a physics-based micro gas turbine model has been developed. The physics-based model is also used to develop a component level diagnostic scheme. The diagnostic scheme is based on a hybrid multi-layer approach, shown in Figure 5, where in the first layer is based on gas path analysis and in the second layer a signature based multiple linear regression techniques is applied. This provides the locations and magnitudes of the possible faults. The outcome from the diagnostics tools is stored in the Condition monitoring and Diagnostics (CMD) database after data post-processing. These results are available in the decision support tool for further analysis and maintenance planning.
Managing a fleet operating on different fuels would add a level of complexity to the framework. It would need to include fuel characteristics to the tuning parameters of each engine model. The diagnostics model can be split into two levels, one sub-level that is fuel-dependent, with smaller families of engines, and one supervisory level that is collecting and comparing data from all engines in the fleet.
The management system for the micro-CHP takes into account historical usage data from the user, the weather forecast and the current electricity price in order to suggest the optimal operating strategy for the unit. This optimal strategy can be a trade-off between cost/unit life and comfort level at home and money savings from selling energy to the grid. The weather forecast function can help the user in determining the short term energy requirements to maintain the desired comfort level. An integrated framework and decision support system with a unique cost function and weather forecast function for each engine allows each user to decide if it is profitable to sell energy in the grid at any given time. When the spot price is high, the user could decide to turn on the engine or choose a lower comfort level at home in order to sell energy. Another operating option would be to run the engine continuously in higher or lower load and trade in the grid rather than turn it on and off more frequently. The decision support tool can advise the user, taking into account the health of the system and the degradation of the components. The optimal operation and production planning framework is depicted in Figure 4. With this framework in place, the micro gas turbines can be used as fast-responding load balancers in the grid, given the right incentives from the energy market.
The proposed framework was used to analyze the micro gas turbine fleet installed by Micro Turbine Technology BV (MTT), a company based in the Netherlands with systems installed throughout Europe. The diagnostics scheme can be used to analyze installation effects on engine performance, which differentiate the operation of different units and can also be used to monitor engine performance degradation over time. The proposed diagnostics scheme was tested on artificially simulated faults in Rahman et al. (2018). A data-driven approach for diagnostics was applied on real engine data collected from the field unit (Olsson et al., 2021). This can provide suggestions for maintenance of the different units, as seen in Figure 6. In the figure, the blue lines represent the historical data, the black lines the forecast for the next 5,000 h and the red lines the maintenance suggestion by the diagnostics algorithm. As shown by Olsson et al. (2021), the data-driven approach can provide an accurate forecast of the condition of the unit. However, as shown by this literature review, the data-driven and the physics-based approaches both have benefits. As such, a combined approach can be expected to provide even better predictions.
FIGURE 6. Engine degradation and maintenance suggestion for different micro-GT units (Olsson et al., 2021).
For the framework to be used for real-time decision support and production planning, it requires the addition of a cost function to the life prediction model, which can communicate with the grid and receive real-time information on the price of electricity and thermal energy and the weather forecast function to predict the short-term usage requirements. A smart system can then evaluate the optimal operating and maintenance strategies to minimize the cost function and maximize the economic return for the user.
As technology plays a bigger role in everyday life, with smart devices connected to smart grids, and automation is governing the operation of numerous small and large devices and machines, real-time monitoring and control is becoming more widespread. Furthermore, advances in computing power and sensor technology with lower costs propel the development of more advanced diagnostics and health prediction functions and yield new possibilities and applications for the monitoring of gas turbines. The technology has reached a level of maturity that allows the implementation of diagnostic functions in smaller and cheaper machines. Meanwhile, the advances in turbocharger technology have allowed the development of micro gas turbines with power output below 10 kW electric that are cost-effective and can be used for micro-CHP systems in domestic applications.
The monitoring, control, and diagnostics of individual engines is commonplace today. However, monitoring solutions for a fleet are not developed to the same extent and are not available in the public domain. The extension or multiplication of existing solutions will not take advantage of all the possibilities available with the presence of a multitude of engines of the same family. It will also not be able to deal with the limited number of sensors used in smaller systems, which is essential in order to limit cost and make them competitive in the market. This requires a purpose-designed system that will compare data from different engines to assist the process.
Fleetwide monitoring requires the processing of a large amount of data and specific models for each engine which are still fast enough to provide data real time. A simplified “mother” engine model with a set of tuning parameters for each engine that will take into account installation effects, operating conditions, and degradation can ease the process of running engine diagnostics and prognostics real time for the entire fleet and is a key component for an automated diagnostics system. This will also ensure that smaller engines will remain competitive by reducing maintenance costs.
The framework for decision support discussed in this paper extends the diagnostics framework to include optimal operation and production planning that will allow the micro-CHP units to connect to the grid and the energy market and provide the possibility to sell energy to the grid when it is cost effective. For this functionality, a cost function has to be included in the framework presented in the literature, which will communicate with the market and determine the more remunerative operating strategy taking into account the possible lifetime decrease due to high fired temperature operations or quick load cycles. The associated challenges are the need to take into account the usage profile of the engine and predict consumer behavior in order to accurately predict its remaining life. However, the extent of possible applications and the maturity of the technologies involved make this a worthwhile venture for the gas turbine community to secure its place in the future energy market.
Conceptualization, IA and KK; investigation, IA, MR, VZ; resources, IA, MR, VZ; writing—original draft preparation, IA, MR; supervision, KK; writing—review and editing, IA and KK.
The work presented in this paper was carried out in connection to the FUDIPO (FUture DIrections for Process industry Optimization) project. This project has received funding from the European Union's Horizon 2020 SPIRE-2 (Sustainable Process Industry Research) programme under Grant Agreement No 723523. The research was partially funded by the Swedish Knowledge Foundation under the project DIAGNOSIS.
The authors declare that the research was conducted in the absence of any commercial or financial relationships that could be construed as a potential conflict of interest.
The authors kindly acknowledge Mark Oostveen and Tomas Olsson for their advice. An earlier version of this work (Aslanidou et al., 2018) was presented at the 2018 Global Power and Propulsion Society (GPPS) Forum.
CHP, combined heat and power; CMD, condition monitoring and diagnostics; MGT, micro gas turbine
Abe, J. O., Popoola, A. P. I., Ajenifuja, E., and Popoola, O. M. (2019). Hydrogen Energy, Economy and Storage: Review and Recommendation. Int. J. Hydrogen Energ. 44, 15072–15086. doi:10.1016/j.ijhydene.2019.04.068
Aslanidou, I., Zaccaria, V., Rahman, M., Oostveen, M., Olsson, T., and Kyprianidis, K. (2018). Towards an Integrated Approach for Micro Gas Turbine Fleet Monitoring, Control, and Diagnostics. Zurich, Switzerland, Jan: Proceedings of Global Power and Propulsion Society Forum 2018, 10–12.
Aurelia Turbines (2020). Availableat: https://aureliaturbines.com/news/aurelia-turbines-commences-deliveries-of-its-gas-turbines. (Accessed 28 Jan 2021).
Bo, A., Giacomazzi, E., Messina, G., and Di Nardo, A. (2018). Analysis of a Fuel Flexible Micro Gas Turbine Combustor through Numerical Simulations. J. Eng. gas turbines Power, 140.
Borguet, S., Léonard, O., and Dewallef, P. (2016). Regression-Based Modeling of a Fleet of Gas Turbine Engines for Performance Trending. ASME. J. Eng. Gas Turbines Power 138, 021201. doi:10.1115/1.4031253
Bower, H. E., Schwärzle, A., Grimm, F., Zornek, T., and Kutne, P. (2020). Experimental Analysis of a Micro Gas Turbine Combustor Optimized for Flexible Operation with Various Gaseous Fuel Compositions. J. Eng. gas turbines Power, 142.
Brummel, H., LeMieux, D. H., Voigt, M., and Zombo, P. (2005). Online Monitoring of Gas Turbine Power Plants. Proceedings in PowerGen International. NV: Las Vegas.
California Independent System Operator (CAISO) (2016). What the Duck Curve Tells Us about Managing a green Grid. Available at: https://www.caiso.com/Documents/FlexibleResourcesHelpRenewables_FastFacts.pdf, (Accessed 27 Jan 2021)
Chu, E., Gorinevsky, D., and Boyd, S. (2010). Detecting Aircraft Performance Anomalies from Cruise Flight Data. Atlanta, GA: AIAA Infotech@Aerospace.doi:10.2514/6.2010-3307
Chu, E., Gorinevsky, D., and Boyd, S. (2011). Scalable Statistical Monitoring of Fleet Data, IFAC Proc. Volumes. 18th World IFAC Congress, Milan, Italy, Aug. 28–Sept. 44 , 13227–13232. doi:10.3182/20110828-6-it-1002.01620
di Gaeta, A., Reale, F., Chiariello, F., and Massoli, P. (2017). A Dynamic Model of a 100 Kw Micro Gas Turbine Fuelled with Natural Gas and Hydrogen Blends and its Application in a Hybrid Energy Grid. Energy 129, 299–320. doi:10.1016/j.energy.2017.03.173
du Toit, M., Engelbrecht, N., Oelofse, S. P., and Bessarabov, D. (2020). Performance Evaluation and Emissions Reduction of a Micro Gas Turbine via the Co-combustion of H2/ch4/co2 Fuel Blends. Sustainable Energ. Tech. Assessments 39, 100718. doi:10.1016/j.seta.2020.100718
European Commission (2016). Putting Energy Efficiency First: Consuming Better. Available at: https://ec.europa.eu/commission/presscorner/detail/en/MEMO_16_3986. (Accessed Jan 28, 2021).
European Commission (2017). Smart Metering Deployment in the european union. Available at: http://ses.jrc.ec.europa.eu/smart-metering-deployment-european-union.(Accessed Jan 28, 2021).
European Council, (2020). 2030 Climate and Energy Framework. Available at: https://ec.europa.eu/clima/policies/strategies/2030_en.(Accessed Jan 27, 2021).
European Council, (2014). 2030 Climate and Energy Policy Frameworkmeeting Conclusions, Euco 169/14. Available at: https://www.consilium.europa.eu/media/24561/145397.pdf.(Accessed Jan 27, 2021).
Fentaye, A., Baheta, A., Gilani, S., and Kyprianidis, K. (2019). A Review of Gas Turbine Gas-Path Diagnostics: State-Of-The-Art Methods, Challenges and Opportunities. Aerospace 6. doi:10.3390/aerospace6070083
Fudipo, (2021). Future Directions of Production Planning and Optimized Energy – and Process Industries. Available at: https://fudipo.eu/.(Accessed Feb 27, 2021).
Ghavidel, S., Li, L., Aghaei, J., Yu, T., and Zhu, J. (2016). A Review on the Virtual Power Plant: Components and Operation Systems. IEEE Int. Conf. Power Syst. Technology (Powercon), 1–6.
Heng, A., Zhang, S., Tan, A. C. C., and Mathew, J. (2009). Rotating Machinery Prognostics: State of the Art, Challenges and Opportunities. Mech. Syst. Signal Process. 23, 724–739. doi:10.1016/j.ymssp.2008.06.009
IEA International Energy Agency (2014). The Role of Storage in Energy System Flexibility. Workshop summary report, 22–23. Berlin, Germany: IEA Expert’s group on RD priority setting and evaluation October.
Jasmani, M. S., Li, Y., and Ariffin, Z. (2011). Measurement Selections for Multicomponent Gas Path Diagnostics Using Analytical Approach and Measurement Subset Concept. J. Eng. Gas Turbines Power 133, 111701. doi:10.1115/1.4002348
Jaw, L. C. (2005). Recent Advancements in Aircraft Engine Health Management (EHM) Technologies and Recommendations for the Next Step. ASME Turbo Expo. Power Land, Sea, Air 1, 683–695. doi:10.1115/GT2005-68625
Johnson, P. (2014). Lessons Learned in Fleetwide Asset Monitoring of Gas Turbines and Supporting Equipment in Power Generation Applications. 2nd European Conference of the Prognostics and Health Management Society, Nantes, France: July 8-10.
Kobayashi, H., Hayakawa, A., Somarathne, K. D. K. A., and Okafor, E. C. (2019). Science and Technology of Ammonia Combustion. Proc. Combustion Inst. 37, 109–133. doi:10.1016/j.proci.2018.09.029
Langston, L. S. (2019). Hydrogen Fueled Gas Turbines. Mech. Eng. 141, 52–54. doi:10.1115/1.2019-MAR-6
Li, Y. G., and Nilkitsaranont, P. (2009). Gas Turbine Performance Prognostic for Condition-Based Maintenance. Appl. Energ. 86, 2152–2161. doi:10.1016/j.apenergy.2009.02.011
Li, Y. G. (2002). Performance-Analysis-Based Gas Turbine Diagnostics: A Review. Proc. Inst. Mech. Eng. A: J. Power Energ. 216, 363–377. doi:10.1243/095765002320877856
Li, Y., Kurfess, T. R., and Liang, S. Y. (2000). Stochastic Prognostics for Rolling Element Bearings. Mech. Syst. Signal Process. 14, 747–762. doi:10.1006/mssp.2000.1301
Lipenko, V., Nigl, S., Roither-Voigt, A., and David, Z. (2021). “Operationalizing Heterogeneous Data-Driven Process Models for Various Industrial Sectors through Microservice-Oriented Cloud-Based Architecture,” in AI and Learning Systems. Editors K. Kyprianidis, and E. Dahlquist (Rijeka: IntechOpen)). doi:10.5772/intechopen.92896
Marinai, L., Probert, D., and Singh, R. (2004). Prospects for Aero Gas-Turbine Diagnostics: a Review. Appl. Energ. 79, 109 – 126. doi:10.1016/j.apenergy.2003.10.005
Mathioudakis, K., Kamboukos, P., and Stamatis, A. (2004). Gas Turbine Component Fault Detection from a Limited Number of Measurements. Proc. Inst. Mech. Eng. Part A: J. Power Energ. 218, 609–618. doi:10.1243/0957650042584302
Mitsubishi, (2021). Mitsubishi Power Commences Development of World’s First Ammonia-Fired 40mw Class Gas Turbine System. Available at: https://power.mhi.com/news/20210301.html.
Morris, H., Ellis, S., Feblowitz, J., Knickle, K., and Torchia, M. (2014). A Software Platform for Operational Technology Innovation, 1–7. International Data Corporation.
Najafabadi, M., Villanustre, F., Khoshgoftaar, T., Seliya, N., Wald, R., and Muharemagic, E. (2015). Deep Learning Applications and Challenges in Big Data Analytics. J. Big Data 2. doi:10.1186/s40537-014-0007-7
Niedler, H. Z., and Fiedler, K. (1999). The Influence of Serial Performance Deviation of Military Jet Engines on the Diagnostic Quality of the Gas Path Analysis, Proceedings of 14th International Symposium on Air Breathing Engines. Florence, Italy. 5–10.
Nosratabadi, S. M., Hooshmand, R.-A., and Gholipour, E. (2017). A Comprehensive Review on Microgrid and Virtual Power Plant Concepts Employed for Distributed Energy Resources Scheduling in Power Systems. Renew. Sustainable Energ. Rev. 67, 341–363. doi:10.1016/j.rser.2016.09.025
Ogaji, S. O. T., Sampath, S., Singh, R., and Probert, S. D. (2002). Parameter Selection for Diagnosing a Gas-Turbine's Performance-Deterioration. Appl. Energ. 73, 25–46. doi:10.1016/s0306-2619(02)00042-9
Olsson, T., Ramentol, E., Rahman, M., Oostveen, M., and Kyprianidis, K. (2021). A Data-Driven Approach for Predicting Long-Term Degradation of a Fleet of Micro Gas Turbines. Energy and AI 4, 100064. doi:10.1016/j.egyai.2021.100064
Oppenheimer, C., and Loparo, K. (2002). Physically Based Diagnosis and Prognosis of Cracked Rotor Shafts. Proceedings of SPIE Component and Systems Diagnostics, Prognostics, and Health Management II. Bellingham, 122–132.
Orchard, M., Wu, B., and Vachtsevanos, G. (2005). A Particle Filter Framework for Failure Prognosis. Washington, DC: Proceedings of the World Tribology Congress. doi:10.1115/wtc2005-64005
Ozgur, D., Lakshminarasimha, A. N., Rucigay, R., Morjaria, M., and Sanborn, S. (2000). Remote Monitoring and Diagnostics System for GE Heavy Duty Gas Turbines, ASME Turbo Expo. Power Land, Sea, Air. , Vol. 3, V003T03A015. doi:10.1115/2000-GT-0314
Paepe, W. D., Coppitters, D., Abraham, S., Tsirikoglou, P., Ghorbaniasl, G., and Contino, F. (2019). Robust Operational Optimization of a Typical Micro Gas Turbine. Energ. Proced. 158, 5795–5803. doi:10.1016/j.egypro.2019.01.549
Pandzic, H., Morales, J., Conejo, A., and Kuzle, I. (2013). Offering Model for a Virtual Power Plant Based on Stochastic Programming. Appl. Energ. 105, 282–292.
Plotts, K., and Diatzikis, E. (2009). A Survey of New Technologies Used by Siemens Energy for the Monitoring and Diagnosis of a Global Fleet of Power Generation Systems, Proceedings of the ASME TURBO EXPO. Orlando, Florida, USA.paper number GT2009-59967
Qiu, J., Set, B., Liang, S., and Zhang, C. (2002). Damage Mechanics Approach for Bearing Lifetime Prognostics. Mech. Syst. Signal Process. 16, 817–829.
Rahman, M., Fentaye, A. D., Zaccaria, V., Aslanidou, I., Dahlquist, E., and Kyprianidis, K. (2021). “A Framework for Learning System for Complex Industrial Processes,” in AI and Learning Systems. Editors K. Kyprianidis, and E. Dahlquist (Rijeka: IntechOpen). doi:10.5772/intechopen.92899
Rahman, M., Zaccaria, V., Zhao, X., and Kyprianidis, K. (2018). Diagnostics-oriented Modelling of Micro Gas Turbines for Fleet Monitoring and Maintenance Optimization. Processes 6, 1–22.
Rist, J. F., Dias, M. F., Palman, M., Zelazo, D., and Cukurel, B. (2017). Economic Dispatch of a Single Micro-gas Turbine under Chp Operation. Appl. Energ. 200, 1–18. doi:10.1016/j.apenergy.2017.05.064
Roemer, M., and Kacprzynski, G. (2000). Advanced Diagnostics and Prognostics for Gas Turbine Engine Risk Assessment. IEEE Aerospace Conference Proceedings, 345–353. doi:10.1109/AERO.2000.877909
Saboori, H., Mohammadi, M., and Taghe, R. (2011). Virtual Power Plant (VPP), Definition, Concept, Components and Types. Power and Energy Engineering Conference (APPEEC), Asia-Pacific. IEEE, 1–4.
Scheianu, D. (2014). Methods and Results in Remote Monitoring and Diagnosing a Fleet of Industrial Gas Turbines, ASME Turbo Expo. Power Land, Sea, Air. Ceramics; Controls, Diagnostics and Instrumentation; Education; Manufacturing Materials and Metallurgy, Vol. 6, V006T06A014. doi:10.1115/GT2014-26068
Searle, S. (2020). E-fuels Won’t Save the Internal Combustion Engine. International Council on Clean TransportationAvailable at: https://theicct.org/blog/staff/e-fuels-will-not-save-ice.
SPIRE (2021). Sustainable Process Industry through Resource and Energy Efficiency. Available at: https://www.spire2030.eu/.(Accessed Feb 22, 2021).
Stamatis, A. G. (2011). Evaluation of Gas Path Analysis Methods for Gas Turbine Diagnosis. J. Mech. Sci. Technology 25, 469. doi:10.1007/s12206-010-1207-5
Stamatis, A., Mathioudakis, K., and Papailiou, K. (1990). Adaptive Simulation of Gas Turbine Performance. J. Eng. Gas Turbines Power 112, 168–175. doi:10.1115/1.2906157
Stenfelt, M., Zaccaria, V., and Kyprianidis, K. (2019). Automatic Gas Turbine Matching Scheme Adaptation for Robust GPA Diagnostics, Proc. ASME Turbo Expo Ceramics; Controls, Diagnostics, and Instrumentation; Education; Manufacturing Materials and Metallurgy, Vol. 621. Phoenix, Arizona, USA, 2019.
Stoeglehner, G., and Narodoslawsky, M. (2009). How Sustainable Are Biofuels? Answers and Further Questions Arising from an Ecological Footprint Perspective. Bioresour. Technology papers from the International Conference on Technologies and Strategic Management of Sustainable Biosystems. 100, 3825–3830. doi:10.1016/j.biortech.2009.01.059
Therkorn, D. (2005). Remote Monitoring and Diagnostic for Combined-Cycle Power Plants. ASME Turbo Expo. Power Land, Sea, Air, 1, 697–703. doi:10.1115/GT2005-68710
Turner, J. A. (2004). Sustainable Hydrogen Production. Science 305, 972–974. doi:10.1126/science.1103197
Urban, L. A. (1973). Gas Path Analysis Applied to Turbine Engine Condition Monitoring. J. Aircraft 10, 400–406. doi:10.2514/3.60240
US Department of Energy (DOE), (2021). Global Energy Storage Database. Available at: https://www.sandia.gov/ess-ssl/download/4999/.(Accessed Jan 27, 2021).
Valera-Medina, A., Morris, S., Runyon, J., Pugh, D., Marsh, R., Beasley, P., et al. (2015). Ammonia, Methane and Hydrogen for Gas Turbines. Energ. Proced. 75, 118–123. doi:10.1016/j.egypro.2015.07.205
Vassileva, I., and Campillo, J. (2016). Consumers’ Perspective on Full-Scale Adoption of Smart Meters: A Case Study in Västerȧs, Sweden. Resources 5.
Vassileva, I., and Campillo, J. (2014). Increasing Energy Efficiency in Low-Income Households through Targeting Awareness and Behavioral Change. Renew. Energ. 67, 59–63.
Visser, W., Shakariyants, A., Later, M. D., Ayed, A., and Kusterer, K. (2012). Performance Optimization of a 3KW Microturbine for CHP Applications ASME Turbo Expo 2012: Turbine Technical Conference and Exposition.
Visser, W., Shakariyants, A., and Oostveen, M. (2011). Development of a 3kW Microturbine for CHP Applications. J. Eng. Gas Turbines Power 133, 042301.
Volponi, A. (2014). Gas Turbine Engine Health Management: Past, Present, and Future Trends. J. Eng. Gas Turbines Power 136, 051201.
Wang, W., Golnaraghi, M., and Ismail, F. (2004). Prognosis of Machine Health Condition Using Neuro-Fuzzy Systems. Mech. Syst. Signal Process. 18, 813–831.
Wei, Z., Zhang, S., Jafari, S., and Nikolaidis, T. (2020). Gas Turbine Aero-Engines Real Time On-Board Modelling: A Review, Research Challenges, and Exploring the Future. Prog. Aerospace Sci. 121, 100693. doi:10.1016/j.paerosci.2020.100693
Zaccaria, V., Rahman, M., Aslanidou, I., and Kyprianidis, K. (2019). A Review of Information Fusion Methods for Gas Turbine Diagnostics. Sustainability 11. doi:10.3390/su11226202
Zaccaria, V., Stenfelt, M., Aslanidou, I., and Kyprianidis, K. (2018). Fleet Monitoring and Diagnostics Framework Based on Digital Twin of Aero-EnginesTurbomachinery Technical Conference and Exposition. Volume 6: Ceramics; Controls, Diagnostics, and Instrumentation; Education; Manufacturing Materials and Metallurgy. Oslo, Norway. Proc. ASME Turbo ExpoJune, 11– 15, V006T05A021. doi:10.1115/GT2018-76414
Keywords: micro gas turbine, fleet monitoring, diagnostics, smart energy system, production planning, automation, predictive maintenance
Citation: Aslanidou I, Rahman M, Zaccaria V and Kyprianidis KG (2021) Micro Gas Turbines in the Future Smart Energy System: Fleet Monitoring, Diagnostics, and System Level Requirements. Front. Mech. Eng 7:676853. doi: 10.3389/fmech.2021.676853
Received: 06 March 2021; Accepted: 11 May 2021;
Published: 01 June 2021.
Edited by:
Christine Mounaïm-Rousselle, Université d'Orléans, FranceReviewed by:
Georgios Mavropoulos, National Technical University of Athens, GreeceCopyright © 2021 Aslanidou, Rahman, Zaccaria and Kyprianidis. This is an open-access article distributed under the terms of the Creative Commons Attribution License (CC BY). The use, distribution or reproduction in other forums is permitted, provided the original author(s) and the copyright owner(s) are credited and that the original publication in this journal is cited, in accordance with accepted academic practice. No use, distribution or reproduction is permitted which does not comply with these terms.
*Correspondence: Ioanna Aslanidou, aW9hbm5hLmFzbGFuaWRvdUBtZGguc2U=
Disclaimer: All claims expressed in this article are solely those of the authors and do not necessarily represent those of their affiliated organizations, or those of the publisher, the editors and the reviewers. Any product that may be evaluated in this article or claim that may be made by its manufacturer is not guaranteed or endorsed by the publisher.
Research integrity at Frontiers
Learn more about the work of our research integrity team to safeguard the quality of each article we publish.